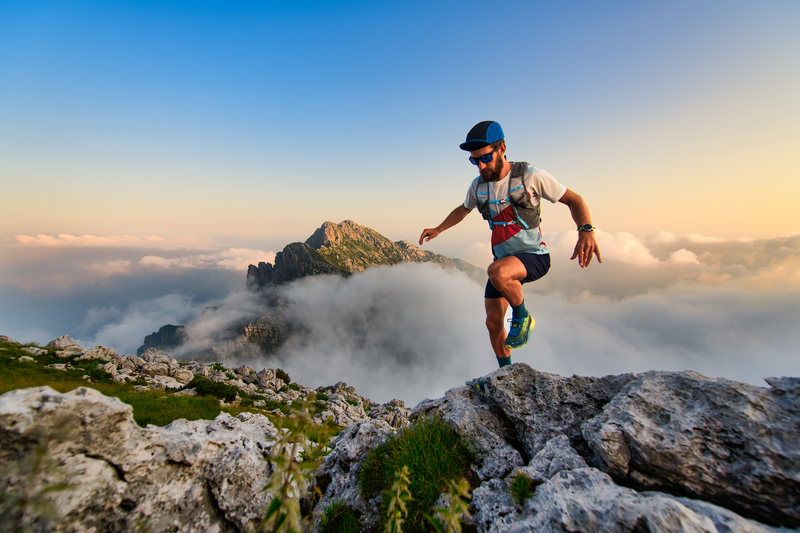
95% of researchers rate our articles as excellent or good
Learn more about the work of our research integrity team to safeguard the quality of each article we publish.
Find out more
ORIGINAL RESEARCH article
Front. Environ. Sci. , 23 November 2023
Sec. Environmental Informatics and Remote Sensing
Volume 11 - 2023 | https://doi.org/10.3389/fenvs.2023.1304845
This article is part of the Research Topic Remote Sensing for Environmental Monitoring View all 13 articles
Forecasting streamflow is important for managing future water resources and environmental needs under the impacts of climate change. Moreover, quantifying the combined effects of future climate variations and human-made infrastructures, e.g., dams and reservoirs, poses a significant challenge. In this study, we used the Soil and Water Assessment Tool (SWAT) for a case study in the Srepok River Basin—a tributary of the Mekong River Basin. Here, we aim to reveal the impacts of various climate change scenarios and the effects of reservoir operations in this region. Our findings indicate that 1) the projected annual streamflow is anticipated to increase by a minimum of 9.2% (2046–2065) and could peak at an increase of 14.9% (2080–2099) under the highest greenhouse gas emissions, 2) Srepok 4, Srepok 3, and Buon Kuop demonstrate a higher capability for mitigating flood peaks and managing seasonal flow in the downstream floodplain, whereas Buon Tua Srah shows the least performance, and 3) reservoirs operated with annual regulation have more pronounced impacts than those regulated on a daily schedule. Our work provides i) a scientific foundation for regional stakeholders and decision-makers to develop sustainable strategies that address the combined effects of reservoir operation and future climate, and ii) it supports national authorities and officials in resolving conflicts related to transboundary rivers within the Mekong River Basin.
Climate change, increasingly recognized as a major concern, has significant impacts on the quality and quantity of water resources (Shukla et al., 2020; Tran et al., 2021a; 2021b; Hussain et al., 2022). Previous studies have identified greenhouse gas emissions, partly resulted from urbanization (Nguyen et al., 2022), as one of the primary reasons causing global warming. These emissions are expected to lead to future increases in temperature and precipitation (IPCC, 2014; IPCC, 2019) resulting in more frequent extreme weather events (e.g., extreme heat waves, widespread floods, year-long droughts, and severe wildfires). The Representative Concentration Pathway (RCP) is a trajectory for greenhouse gas concentration developed by the IPCC (IPCC, 2019). Numerous studies have highlighted the effects of climate change on the natural water cycle (Bolch et al., 2012; Aryal et al., 2019; Bhatta et al., 2019; Roderick et al., 2019; Tran et al., 2023b). Floods caused by extreme rainfall are expected to become more frequent across the globe (Chattopadhyay et al., 2017; Vo et al., 2018; Ivanov et al., 2021; Tran et al., 2023b), and are likely to persist even more severely in the near future. Besides, Lehner et al. (2019) observed that the flow patterns of a third of the world’s 200 largest rivers have changed since the 1950s, with these alterations being directly attributable to climate change. Although it is challenging to obtain an accurate prediction of streamflow under the climate impacts, it remains a crucial undertaking for effective water resource planning, regional management, and mitigation of extreme events (Khoi and Suetsugi, 2012; Tran et al., 2022c; Tran et al., 2022e; Umar et al., 2022). In Vietnam, a number of studies have investigated the impacts of climate change at various scales (Khoi and Suetsugi, 2012; Ty et al., 2012; Huyen et al., 2017; Vo et al., 2018; Giang and Vy, 2021). However, the majority of these studies were carried out in previous decades with the neglect of important factors (e.g., man-made infrastructures), raising concerns about their reliability which is relevant to the current specifics of climate change in this region.
The Mekong River, an important transboundary river in East and Southeast Asia, flows through several countries, including China, Myanmar, Laos, Thailand, Cambodia, and Vietnam (Arias et al., 2014a) (Figure 1A). The Srepok River Basin (SRB), which is located in central Vietnam, is a significant tributary of the Mekong River Basin (MRB), annually contributing a substantial volume of water to the Mekong River (Arias et al., 2014b; Nuong et al., 2022; Chang et al., 2023) (Figure 1B). Alterations in water supply, particularly due to dam operations, can remarkably affect the region’s water resources (Giang et al., 2017; Du et al., 2022; Nguyen et al., 2023a; Nguyen et al., 2023b; Bui et al., 2023; Smigaj et al., 2023). Within SRB, such changes in the water cycle may affect the hydrology of the middle and lower sections of the MRB, influencing the lives of nearly 11,000 Cambodians residing along the river and at the basin’s outlet (International Rivers, 2010). Previous studies have enhanced our understanding of how climate change may impact the Srepok River as well as the 3S River (Srepok, Sesan, and Sekong) (Arias et al., 2014a; Cochrane et al., 2014; Piman et al., 2016; Huyen et al., 2017; Trang and Lakshmi, 2022; Bui et al., 2023). However, these studies have overlooked the impact of existing dams and reservoirs due to a lack of operational data, an oversight that should be considered in climate change studies. Furthermore, the critical role of the Krongbuk tributary has been largely ignored, meaning that total runoff at the SRB outlet—and by extension, regional volume assessments and disaster prevention strategies—may not be fully understood or accounted for. Furthermore, the reliability of their baseline models, which utilize historical data, is questionable due to the limited duration of the calibration and validation periods—factors that play a crucial role in deriving accurate parameters for projecting future climate scenarios. Also, the study by Piman et al. (2016), which constructed a baseline period between 1986 and 2006, or Arias et al. (2014a) from 1982 to 2005, Cochrane et al. (2014), Oeurng et al. (2016) from 1980 to 2008, and Shrestha et al. (2016) from the 1980s–2000s, have eliminated considerations of current dam operations for the SRB. This gap suggests that such studies may not fully capture the projected impacts of future climate change on this region, potentially providing decision-makers and regional planners with incomplete data.
FIGURE 1. (A) Location of SRB in the MRB (B) SRB and the distribution of reservoirs, and stream network.
The climate inputs for hydrological models, e.g., temperature and precipitation, can be derived using two primary methods: 1) revising records obtained from meteorological stations (Khoi and Suetsugi, 2012; Li and Fang, 2021; Raghavan et al., 2012), and 2) adjusting outputs from climate models (Vo et al., 2018; Tran et al., 2022c). General Circulation Models (GCMs), also known as Global Climate Models, are developed through assumptions and mathematical representations of the physical climate system’s processes (Li and Fang, 2021). An ensemble of GCMs, created by various global organizations and institutions, can provide more accurate predictions for water resources than a single GCM (Pierce et al., 2009; Ranger et al., 2011). Accordingly, we utilized datasets from the climate change scenarios released by the Vietnam Ministry of Natural Resources and Environment (2020) in this study. These scenarios cover two timeframes: the near future (2046–2065) and the far future (2080–2099) and the ensemble would be formed based on projections from six distinct Regional Climate Models (RCMs). These include: i) the Climate-WRF (CL-WRF) model from the United States (Fita et al., 2010); ii) the Providing REgional Climates for Impacts Studies (PRECIS) model from the United Kingdom (Moberg and Jones, 2004); iii) the Conformal Cubic Atmospheric Model (CCAM) from Australia (Her, 2014); iv) the Regional Climate Model (RegCM) from Italy (NCAR, 2017); v) the Meteorological Research Institute (MRI) for Atmospheric Climate Model (AGCM/MRI) from Japan (Mizuta et al., 2012); and vi) the Rossby Centre Regional Climate (RCA3) model from Sweden (Samuelsson et al., 2011).
This study aims to reveal the impacts of dam operation and future climate scenarios on the water resources of SRB by employing the SWAT hydrological model. In general, our findings would:
(1) Refine and update previous baseline models using recent and comprehensive datasets for accurate simulations of projected future runoff, especially at the SRB’s outlet;
(2) Analyze the impacts of climate change and the existing dams’ operation, an aspect often overlooked in previous works; and
(3) Quantify the contributions of the SRB’s main tributaries to the overall flow under different future climate conditions.
By fulfilling these objectives, our study aims to reduce the uncertainties currently associated with future hydrological projections in this region. The implications of our findings are critical for water resource management, with direct benefits for the local communities in Vietnam and Cambodia. Additionally, this work provides a solid scientific foundation for disaster prevention strategies in the lower MRB, thereby supporting stakeholders and regional authorities in making informed decisions.
The Srepok River, a major tributary of the Mekong, has its source in the highlands of Dak Lak Province, Vietnam. It flows through Ratanakiri and Stung Treng regions before joining the Mekong River (Figures 1A, B) with the total river’s length approximately 450 km. The river’s initial course covers 169 km within Vietnamese territory before traveling through 281 km in Cambodia. The SRB covers an area of approximately 18,200 km2 within Vietnam, primarily occupying the upper segment of the basin, which constitutes over 65% of the total area. This watershed is characterized by a diverse topography with the upper region’s elevation ranges from 200 to over 2,240 m, with average heights transitioning from 350 m in the northwest to about 1,000 m in the southeast (Figures 2A, B). Between 1998 and 2018, the average annual precipitation recorded at main hydrological stations of SRB (e.g., Giang Son, Duc Xuyen, Cau 14, and Ban Don) were approximately 1920 mm, 1937 mm, 1704 mm, and 1,601 mm, respectively. The majority of this precipitation, exceeding 70%, occurred during the wet season (June to November), with an estimated 41% of the annual precipitation contributing to the basin’s runoff. In this study, the Duc Xuyen and Giang Son regions are specifically highlighted due to their significant hydrological contributions to the SRB’s outflow (Ty et al., 2011; 2012).
On the other hand, Vietnam exhibits considerable hydropower potential, notably in the northern and central highland regions. In this study, we include five primary hydropower reservoirs and associated power plants subsequent to the initial phase of Hydropower Projects in the Lower MRB, including: Buon Tua Srah, Buon Kuop, Srepok 3, Srepok 4, and Srepok 4A (Figure 1B; Table 1). As mentioned, we incorporates these infrastructures (see section 2.3.2) to assess their implications on the SRB’s hydrological dynamics and to evaluate their operational performance under various climate change scenarios.
We used the SWAT hydrological model with the necessary inputs as presented in Table 1.
SWAT is a semi-distributed hydrological model developed and maintained by the U.S. Department of Agriculture (USDA) and the Agricultural Research Service (ARS) (Arnold et al., 2012a). In recent years, SWAT has gained popularity in both the United States and Europe, largely owing to its effectiveness in addressing a range of hydrological issues (Gassman et al., 2007; Tran et al., 2023a). Numerous studies have utilized the SWAT model to examine the impacts of various factors on streamflow and sediment loads (Ahmed et al., 2020). These factors include land use and land cover (LULC) changes (Anaba et al., 2017; Aryal et al., 2022; 2023; Tran and Lakshmi, 2022), the effects of climate change (Vo et al., 2018; Aslam et al., 2022; Shafeeque et al., 2023a; 2023b), improvements in ecosystem services (Ashrafi et al., 2022a; Arshad et al., 2022; Ashrafi et al., 2022b; Tapas et al., 2022), and the validation of satellite-based products (Arshad et al., 2021; Tran et al., 2022a; 2022b; Noor et al., 2023).
The calibration and validation of the SWAT model, as well as the assessment of parameter uncertainty using the Sequential Uncertainty Fitting procedure (SUFI-2) as the objective function, were carried out with the SWAT-CUP program (version 5.2.1) (https://www.2w2e.com/home/SwatCup), as detailed by Abbaspour et al. (2015) and Zhang et al. (2014). Model performance metrics are presented in Table 2. The objective function (Nash-Sutcliffe efficiency; NSE) was used in SWAT-CUP to calibrate and validate the SWAT model.
TABLE 2. Performance metrics for the model calibration and validation. Where Q is the streamflow (m3/s), m, s stand for measured and simulated, and d stands for deviation of it, i is the ith measured and simulated,
Where Q is the streamflow (m3/s), m, s stand for measured and simulated, and d stands for deviation of it, i is the
A 7-year warm-up period (1985–1991) was chosen within the 33-year simulation period (1985–2018), followed by 10 years (1992–2001) for model calibration and 17 years (2002–2018) for validation. The calibration and validation of the model were performed on a daily basis, with the ideal range for the number of iterations set between 300 and 500 to balance computational efficiency and time requirements.
This work initially focuses on the combined impacts of certain reservoirs—Buon Tua Srah, Buon Kuop, Srepok 3, and Srepok 4—on the SRB (Figure 1B). These reservoirs, previously overlooked in related studies, are included here due to their importance in assessing climate change effects. We will examine the operational impacts of each reservoir, particularly their influence on flood peaks and total runoff at the SRB outlet. To be specific, we compare a baseline scenario (natural flow without reservoirs) against four scenarios where each reservoir is independently operated, to isolate their individual impacts for better management and operational planning. The Srepok 4A reservoir, however, will not be considered in this study due to its limited capacity and primary function of serving irrigation needs for a small area, as reported by MRC (2017).
For the reservoir setup in the SWAT model, we selected the following parameters: the operational start date of the reservoir (MORES for month and IYRES for year), the reservoir’s surface area at emergency spillway capacity (RES_ESA; ha), and the corresponding volume (RES_EVOL; 104 m3). Additional parameters include the surface area at principal spillway capacity (RES_PSA; ha), the required volume to reach this capacity (RES_PVOL; 104 m3), the initial volume of water in the reservoir (RES_VOL; 104 m3), the initial sediment concentration (RES_SED; mg/L), evaporation coefficient (EVRSV), and the average daily discharge when overflowing (RES_RR; m3/s). We also considered the non-flood season duration (IFLOD1R and IFLOD2R for start and end months, respectively), the fraction of water removed from the reservoir during non-flood season (WURTNF; m3/s), the minimum outflow relative to principal spillway volume (OFLOWMN_FPS), and the target storage volume also relative to the principal spillway (STARG_FPS) (Arnold et al., 2012a).
Two parameters were identified as most sensitive for reservoir calibration: the hydraulic conductivity at the reservoir bottom (RES_K; mm/h) and the number of days required to reach the target storage from the current volume (NDTARGR; days). These were calibrated using methodologies described by Kim and Parajuli (2014) and Qiu et al. (2019). In addition, twenty-three parameters were chosen for model calibration, validation, and sensitivity analysis, based on p-value and t-Stat statistical methods as mentioned in Arnold et al. (2012b), Tuo et al. (2016), and Xu et al. (2016). These parameters’ descriptions and their calibrated values are listed in Table 4.
In this study, SWAT’s operating policies are based on monthly storage targets. The storage target operations are piecewise linear functions for each reservoir in the system. Each reservoir’s policy is defined by parameters: a target storage value for each month (STARG) and NDTARGR (days), which are constant across all months. Daily reservoir releases (
Where V is the volume of water stored in the reservoir,
Future climate data were extracted from the Vietnam Climate Change Scenario dataset, which was validated by the Vietnamese Government under resolution No. 93/NQ-CP, issued on 31 October 2016. This resolution affirmed Vietnam’s commitment to the Paris Agreement—a global pact within the United Nations Framework Convention on Climate Change—officially ratified on 20 July 2020. Summaries of the data from six different Regional Climate Models (RCMs), updated using the IPCC 2019 report and the 2018 Vietnam Meteorological datasets (e.g., 5 m resolution lidar DEM), are presented in Table 3. Our analysis employed the RCP4.5 scenario, which projects an average annual rainfall increase of 10%–20%, and the RCP8.5 scenario, which anticipates an average annual rainfall increase of up to 40%. These scenarios correspond to the near future and far future periods, as designated by MONRE (2016).
Figure 3 and Table 4 present the parameter ranking, method, description, range, and fitted value for parameter sensitivity analysis using SWAT model.
FIGURE 3. Sensitivity analysis using t-Stat and p-value for chosen SWAT-CUP parameters with their ranking. Parameters with a p-value ≤0.05 and a high t-Stat value—when |t-Stat| ≥ 1.96 were considered sensitive (Mosavi et al., 2021). This threshold indicates that, with 95% confidence, the variables have a significant effect.
TABLE 4. Summary of SWAT and reservoir parameters with their sensitivity ranked based on t-Stat values, as described in Mosavi et al. (2021).
The SCS runoff curve number (CN2) was found as the most sensitive parameter, followed by the base flow alpha factor for bank storage (ALPHA_BNK), the effective hydraulic conductivity in the main channel alluvium (CH_K2), Manning’s “n” value for overland flow (OV_N), the plant uptake compensation factor (EPCO), the calibration coefficient used to control the impact of the storage time constant from low flow (MSK_CO2), and the deep aquifer percolation fraction (RCHRG_DP) (Figure 3). These results indicate that SRB is significantly sensitive to surface runoff parameters (defined by CN2, CH_K2, and OV_N) and EPCO. This sensitivity can be attributed to the region’s dense vegetation cover. The findings are consistent with observations from previous studies by Bajracharya et al. (2018), Bhatta et al. (2019), and Li and Fang (2021). However, groundwater-related parameters, e.g., RCHRG_DP and GW_DELAY, were found to be non-sensitive in our study. This insensitivity may be due to extensive groundwater extraction for agricultural activities, which diminishes the interaction between surface and subsurface waters.
To achieve optimal calibration, we performed the model calibration and validation at multiple objective stations, including Krongbuk, Giang Son, and Duc Xuyen (Figure 1B). Figure 4 compares observed and simulated daily streamflow at A) Krongbuk B) Giang Son C) Duc Xuyen D) Cau 14, and E) Ban Don stations. A detailed summary of the model’s performance metrics is presented in Figure 5. We performed these different scenarios using the same model setup and set of calibrating parameters (Table 4). The Krongbuk station, chosen as the objective station for model calibration and validation, showed a good performance, particularly during the validation phase of the second period following the operation of five reservoirs (after 2009) (Ty et al., 2011) (Figure 4A). Our findings thus address a gap in previous analyses, highlighting the capability of the Krongbuk station for model calibration purposes.
FIGURE 4. Comparisons between observed and simulated streamflow (daily scale) using the objective station–Krongbuk, for calibration period (1992–2002), first validation (without dam) (2003–2008), and second validation (with dam) (2009–2018). The results were shown at (A) Krongbuk (B) Giang Son (C) Duc Xuyen (D) Cau 14, and (E) Ban Don.
FIGURE 5. Summary of the model’s calibration and validation (1992–2018) performed at different objective stations (Duc Xuyen, Krongbuk, and Giang Son).
Model calibration for the Duc Xuyen region (2009–2018), revealed low values of NSE and KGE (Figure 4C). This could be explained due to a range of local factors, including variations in terrain profile, agricultural practices, and water resource management policies. Specifically, the intensive use of both surface water and groundwater for agricultural purposes in the Duc Xuyen area, as opposed to practices in the Krongbuk region, may have contributed to these results, and aligned with findings from Arias et al. (2014b), Huyen et al. (2017), and Ty et al. (2011, 2012).
Figure 5 shows the calibration and validation scenarios conducted at the Duc Xuyen, Krongbuk, and Giang Son stations. While the scenario performed at Krongbuk provided the most accurate fit for the SRB (Table 2), Giang Son station’s performance was slightly less accurate, whereas the Duc Xuyen station had the least precise results. In particular, the Duc Xuyen station showed a range of KGE values from 0.45 to 0.80 across the stations of Ban Don, Cau 14, Duc Xuyen, Giang Son, and Krongbuk (Figure 5A). The least favorable outcomes were observed at the Giang Son station (NSE of 0.21 and RMSE of 96.15 m³/s), and at the Krongbuk station (NSE of 0.05 and RMSE of 12.84 m³/s). In addition, the calibration and validation processes at the Krongbuk station indicated improvements, particularly with respect to flood peak predictions and total streamflow volume assessment (Figure 5). The model achieved satisfactory scores here, enhancing its dependability for assessing the effects of RCPs, especially regarding the cumulative impacts from the regional reservoirs.
The streamflow contributions from the Giang Son and Duc Xuyen regions to the SRB outlet are quantitatively shown in Figure 6. To be specific, the Duc Xuyen region adds an extra 21.1% to the SRB’s total runoff compared to the Giang Son region.
FIGURE 6. Streamflow contributions from different regions to the SRB outlet, categorized as (A) annual (B) monthly (C) before and after reservoirs operated (D) seasons, and (E) among different periods.
The Giang Son region contributes between 32.9% and 39.8% of the total runoff volume at the SRB outlet (Figure 6B). During the wet season, the region is responsible for 30.2% of the total streamflow, which is less than the 43.1% provided by the Duc Xuyen region, underscoring the latter’s significant influence during this period (Figure 6C). The scenario changes in the dry season, where the contribution dynamics between the two regions reverse. Figure 6D shows that the Giang Son region’s share of the total streamflow increases to 39.2%, exceeding the 32.2% from the Duc Xuyen region. This indicates the variable influence of these regions on the SRB’s hydrology across different seasons.
Figure 6C shows a notable difference in the annual streamflow patterns corresponding to the period before (pre-2009; baseline scenario without dam) and after (post-2009) the commencement of dam operations. This provides insights into the changing hydrological response that could be explained due to anthropogenic intervention (Ty et al., 2012). These observations underscore the transformative impact of reservoirs on streamflow patterns, with implications for water resource management, flood control, and ecosystem services in the SRB. Besides, the streamflow patterns in the pre-2009 period are characterized by relative stability. However, during the post-2009, when the reservoirs became operational, the runoff demonstrates marked variability, indicating the direct influence of reservoir management on the hydrology of the region. In addition, SRB experienced a noticeable 25% reduction in total runoff in the Ban Don, Cau 14, and Duc Xuyen regions (Figure 6) between 2009 and 2018. This decline is attributed to the active period of dam operation and highlights the substantial role that these structures play in modifying natural streamflow regimes.
We conducted a comprehensive assessment to evaluate the influence of individual reservoirs on the cumulative runoff at the SRB outlet (Buon Tua Srah, Buon Kuop, Srepok 3, and Srepok 4) (Figure 1B). For this, we compared the flood peak, annual, and seasonal runoff at the SRB outlet under these experimental scenarios against a baseline scenario in which all reservoirs would be inactive (see section 2.3.2) (Figure 7).
FIGURE 7. Discharge in (A) annual (B) flood peak (C) monthly, and (D) seasonal (wet, dry). Each scenario is performed with the reservoir’s name, shown in the legend, corresponding to when it is activated.
Table 5 presents the impacts of each reservoir on the runoff of the SRB, showing an increasing influence from the downstream to the upstream regions. Notably, the capacity for flood reduction correlates directly with the active storage capacity of the reservoirs. In particular, Srepok 4 demonstrates the most effective capability for mitigating flood peaks in the downstream floodplain, followed by Srepok 3 and Buon Kuop, while Buon Tua Srah exhibits the least effectiveness (Figure 7B). The least performance of Buon Tua Srah can be attributed to its location; despite having a good design capacity, it is situated in the highland and upper region of the SRB (Figure 1B). Furthermore, our results also show that the increased volume of water released during the dry season contributes to a rise in the total runoff of the SRB when compared to the baseline scenario.
TABLE 5. The reservoir’s impact on streamflow at Duc Xuyen, Cau 14, and Ban Don stations, compared to the baseline (all reservoirs inactive) (2009–2018) on seasonal and annual scales.
Regarding the average annual runoff at the outlet of the SRB (2009–2018), the activation of each individual reservoir corresponds to a respective decrease in annual runoff: 0.26% for Buon Tua Srah, 0.13% for Buon Kuop, 0.24% for Srepok 3, and 0.08% for Srepok 4, when compared to the scenario where all these reservoirs are deactivated. Despite the overall reduction in runoff following reservoir activation, the Buon Tua Srah reservoir exhibits the most significant decrease in total runoff at the outlet of the SRB. While Buon Tua Srah is located upstream, allowing it to capture more discharge from the upper regions of the SRB, Srepok 3 is situated further downstream but possesses the highest design capacity, which allows it to store and subsequently release a substantial volume of water downstream. Further analysis revealed that the impacts of the reservoirs (Buon Kuop, Srepok 3 and 4) are particularly noticeable during the dry and wet seasons, as can be attributed to their locations in the mainstream of the Srepok River (Figure 1B; Figure 7; Table 5). Moreover, we found that reservoirs operated on an annual regulation (Buon Tua Srah, Buon Kuop, and Srepok 3) have a more pronounced and enduring impact on runoff patterns compared to those managed on a daily regulation (Srepok 4) (Table 5), and this finding therefore could be used to better regulate the runoff before and after flood and drought events.
Figure 8 shows the rainfall variations throughout the baseline period (1992–2018) and under two future scenarios, RCP 4.5 and 8.5. The peak rainfall occurs from July to October, with September experiencing the highest volume. During the wet season (June to November) (Nguyen and Nguyen, 2004), 63% of the annual rainfall is recorded, whereas the dry season sees a significant drop, contributing only 58% (243.45 mm) of the total accumulative volume (Figure 8A). Between 2046 and 2065, a slight increase in rainfall is projected, accounting for 3.39 mm (RCP 4.5) and 3.88 mm (RCP 8.5), compared to the baseline period (Figure 8A). In general, an upward trend rise in the annual rainfall (2080–2099) with approximately of 6.2%–7.1% increase for the 2046–2065 period and 7.7%–11.5% increase for the 2080–2099 period in rainfall compared to the historical record. We also found that these scenarios suggest average monthly rainfall increases of at least 4.21 mm–6.28 mm with the most significance expected during August and September (Figures 8B, C).
FIGURE 8. Baseline scenario for (A) Precipitation (D) Tmax (G) Tmin, and changes in monthly precipitation for (B) RCP4.5 (C) RCP8.5, monthly maximum temperature for (E) RCP4.5 (F) RCP8.5, and monthly minimum temperature for (H) RCP4.5, and (I) RCP8.5 in the 2046–2065 and 2080–2099 compared to the baseline (1992–2018). Dash line represents the mean value.
Figure 8 and Figure 9 show projected increases in maximum (Tmax), minimum (Tmin), and average (Tmean) temperatures under future RCPs. The baseline data reveals the warmest months to be between March and May, with temperatures decreasing during the wet season and reaching their lowest in October (Figure 9D; Figure 9E; Figure 9F). Under the future scenarios RCP 4.5 and RCP 8.5, modest temperature rises of 1.4 °C and 1.8 °C are expected, respectively, with RCP 8.5 presenting a more pronounced increase (Figure 9E, Figure 9F, Figure 9H, and Figure 9I). The trends suggest that seasonal and annual average temperatures will continue to increase, with the period from 2080 to 2099 under RCP 8.5 anticipating the highest temperature increase.
FIGURE 9. Mean changes in the dry season for (A) Precipitation (D) Tmax, and (G) Tmin, the wet season for (B) Precipitation (E) Tmax, and (H) Tmin, and the annual for (C) Precipitation (F) Tmax, and (I) Tmin in (F) 2046–2065 and (G) 2080–2099 compared to the baseline (1992–2018). Dash line represents the mean value.
The two RCPs predict an increase in streamflow across monthly, annual, and seasonal scales. For the period between 2046 and 2065, an increase in annual streamflow is anticipated, with a range of 9.14% for RCP 4.5%–9.8% for RCP 8.5 (Figure 10). A more significant jump of 2.1%–3.2% above these levels is projected for the later period of 2080–2099. The largest streamflow increases are expected from May to September compared to the baseline scenario, with the smallest rises occurring at the start of the year in January and February. These range from an increase of 5.1% under RCP 4.5 (2046–2065) to 7.74% under RCP 8.5 (2080–2099) (Figures 10A, B). The projected average streamflow increase for 2046–2065 is approximately 29.77 m³/s, corresponding to an increase of about 10.7% for both RCP 4.5 and RCP 8.5 from the baseline scenario (Figure 10A). Between 2080 and 2099, the rise is found at 12.10% (35.78 m³/s) for RCP 4.5% and 15.97% (47.21 m³/s) for RCP 8.5 (Figure 10B).
FIGURE 10. Changes in streamflow for (A) monthly (2046–2065) (B) monthly (2080–2099) (C) annual (D) wet season (E) dry season, and hydrological components during (F) 2046–2065, and (G) 2080–2099.
Figures 10C–E show variations in annual and seasonal streamflow, highlighting the SRB’s sensitivity to seasonal shifts under different RCPs. The wet season exhibits the most notable increases in streamflow, with rises of 10.13% (RCP 4.5) and 10.92% (RCP 8.5) between 2046 and 2065. These increases are projected to grow to 12.36% and 16.8% for the respective RCPs (2080–2099) compared to the baseline scenario (Figure 10D). Conversely, the dry season’s streamflow increase is more modest, at 6.81% and 7.1% for RCP 4.5 and RCP 8.5, respectively (2046–2065), and 8.3% and 10.5% (2080–2099), respectively (Figure 10E). These changes are confirmed by the monthly streamflow trends (Figures 10A, B) and align with the anticipated alterations in wet season rainfall resulted from the influences of RCP scenarios. Furthermore, average annual streamflow is projected to peak (2080–2099) under the RCP 8.5 scenario (14.9%), with the least significant rises noted at 9.2% under RCP 4.5% and 9.8% under RCP 8.5 during the 2046–2065 period (Figure 10C).
Figures 10F, G present a statistical analysis of hydrological components impacted by the RCP scenarios. Key hydrological elements, e.g., potential evapotranspiration (PET), actual evapotranspiration (ET), and groundwater levels, are all projected to trend upwards, though the differences between the RCP scenarios are not pronounced. However, the RCP 8.5 is anticipated to drive more considerable increases or decreases in streamflow and related hydrological effects.
We noticed that the typical historical severe drought in the Giang Son region (2012–2013), which resulted in a large disparity between the Duc Xuyen and Giang Son regions, highlights the need for robust water conservation and allocation practices to support the agricultural sector, which is vulnerable to water scarcity during such events. Specifically, while streamflow in the Duc Xuyen region was more stable across drought periods, streamflow in the Giang Son region varied dramatically. This shows the Giang Son region’s vulnerability to drought and indicates the critical need to focus on water resource management strategies, especially considering the dependency of local agriculture on reliable water supplies.
Since their commissioning (post-2009), the downstream reservoirs—Srepok 4, Srepok 3, and Buon Kuop—have demonstrated a robust capability to mitigate flood peaks in the downstream floodplain. This aligns with the operational design outlined by the MRC (2017), which specifies that mainstream reservoirs are designed with more flood prevention functions compared to upstream reservoirs (e.g., Buon Tua Srah) that are mainly used for irrigation and electricity production. The substantial impact of the Srepok 3 reservoir, with a 0.24% decrease in total runoff at the SRB’s outlet, is primarily attributed to its high design capacity, which allows for the release of more water to the downstream region (Table 5). Conversely, the impact of the Buon Tua Srah reservoir (0.26% decrease in total runoff) is attributed to its location in the upstream region, which helps to collect more discharge in the upper part of the SRB.
An upward trend in future climate projections indicates an approximate increase of 6.2%–7.1% for the 2046–2065 period and 7.7%–11.5% for the 2080–2099 period in rainfall, leading to an increase in the average annual streamflow by around 9.2%–11.2% and 9.8%–14.9%, under the RCP 4.5 and 8.5, respectively (Figures 8A–C, Figures 9A–C, and Figures 10A–E). To answer whether climate change impacts or reservoir operations will significantly affect the SRB’s outlet, we found that it depends on several factors, which require further consideration. First, the regulations of the reservoirs presented in this study are set as constant due to the limitations of SWAT configurations for reservoir module, which can only accept a fixed setup for the entire simulation (Arnold J. et al., 2012). This thus does not reflect the actual operation of these reservoirs in reality, which vary according to different factors (e.g., agricultural activities, irrigation, groundwater extraction, local usage, etc.). Furthermore, when considering the impacts of climate change and the role of reservoirs, it is clear that while the current chosen reservoirs have decreased flood intensity, however, the regular operation of reservoirs does not fully reflect each reservoir’s maximum flood prevention capacity. Additionally, the limitations arising from the SWAT configuration for the reservoir module mean that the findings in this study should be taken as a reference for future works using more complex numerical models, e.g., the Variable Infiltration Capacity (VIC) model (Liang et al., 1994) with more detailed experiments performed.
The two greenhouse gas emission scenarios (RCP 4.5 and 8.5) predict an average increase in precipitation, with the most significant rise expected in August and September (Figures 8B, C). The SRB is projected to experience the largest increase in annual streamflow volumes during the 2080–2099 period under the RCP 8.5 scenario. The smallest increase is anticipated under the RCP 4.5 scenario between 2046 and 2065, with the greatest increase projected under RCP 8.5 from 2080 to 2099 (Figure 10C). A higher greenhouse gas emission trajectory correlates with greater increases in weather intensity and hydrological responses in terms of volume. However, we also noted that the CMIP5 models may not fully capture the actual climate characteristics due to the neglect of socioeconomic activities and environmental factors that could influence the reliability of the models’ outcomes (Bourdeau-Goulet and Hassanzadeh, 2021; Chen et al., 2021). Our suggestion is to use the latest version of the CMIP model (CMIP6) which could help to decrease the uncertainty resulted from these mentioned factors.
Furthermore, various hydrological components, e.g., potential evapotranspiration (PET), actual evapotranspiration (ET), and groundwater levels, are anticipated to follow an upward trajectory. While the differences between the RCP scenarios are not markedly significant, the more intense RCP 8.5 is expected to lead to more pronounced fluctuations in streamflow and hydrological outcomes (Figures 10F, G).
In summary, the insights resulted from this study serve as an important scientific basis for stakeholders and authorities involved in water resource management and climate adaptation strategies in SRB. Strategic planning can involve the construction of additional water storage facilities or the implementation of water-saving technologies in agricultural practices. The efficacy of downstream reservoirs, e.g., Srepok 4, Srepok 3, and Buon Kuop, in mitigating flood peaks presents a case for continuing and expanding such infrastructure, particularly in the context of future projected increases in terms of precipitation and temperature. These infrastructures are not only critical for flood control and prevention but also for maintaining steady water supplies during dry periods. The clear advantage of downstream reservoirs in flood mitigation due to their design and capacity should guide future reservoir projects to maximize flood control benefits. The change in projected future precipitation and temperatures highlights the urgent need for updating regional flood risk maps, enhancing early warning systems, and preparing for more extreme weather events. Conversely, the increase in evapotranspiration and the fluctuations in groundwater levels will demand more complex agricultural and urban planning to ensure water sustainability.
Our findings suggest that proactive measures, informed by the predicted hydrological changes under various climate scenarios, are crucial for ensuring the resilience of the SRB and the communities that depend on its resources. Authorities are advised to integrate these findings into long-term planning and operational decision-making to mitigate the adverse impacts of climate variability and secure water for all uses.
In this study, we examined both the individual and combined effects of future climate change and reservoir operations on the SRB, with a focus on flood peaks and variations in seasonal and annual runoff for the near (2045–2065) and far future (2080–2099) periods. Additionally, we introduced a novel calibration approach that includes the Krongbuk region, which had been overlooked in previous research. Our key findings include:
(1) The wet season accounts for 63% of the annual precipitation, while the dry season receives 58% less precipitation. Future projections suggest an average annual increase in precipitation of about 6.2%–7.1% in the near future and up to 11.5% in the far future. Meanwhile, a maximum temperature increase of 1.8 °C is expected under the highest emission scenario compared to the historical period.
(2) Future average monthly streamflow is projected to increase by at least 10.7% (2046–2065) and could reach a maximum increase of 15.97% under the highest greenhouse gas emissions scenario (2080–2099). Variability in annual and seasonal streamflow has been indicated, highlighting the SRB’s high sensitivity to seasonal shifts under varying future climate conditions. The average annual streamflow is projected to increase by 9.2% in the near future under the RCP 4.5 scenario, with an additional increase of 0.6% expected in the far future. Under the RCP 8.5 scenario, the near future could see an increase of 11.2%, with the far future anticipating a rise of 14.2%. Key hydrological components, including potential evapotranspiration, actual evapotranspiration, and groundwater levels, are also expected to rise.
(3) Climate change is predicted to have a more substantial impact on downstream streamflow than dam operations, potentially leading to significant changes in flow regimes, especially in the downstream region of the SRB. Srepok 4, Srepok 3, and Buon Kuop have demonstrated superior capabilities for mitigating flood peaks in the downstream floodplain, whereas Buon Tua Srah has shown the least effectiveness. Regarding seasonal discharge, Buon Kuop, Srepok 3, and 4 significantly influence the flow, while reservoirs operated on an annual regulation (including Buon Tua Srah, Buon Kuop, and Srepok 3) have a more pronounced and sustained impact on runoff patterns compared to those managed on a daily regulation, such as Srepok 4.
Overall, although reservoirs play a role in mitigating the impacts of climate change within the study period, the influence of climate change is expected to significantly intensify extreme hydrological events, e.g., floods and droughts, especially in critical transboundary river basins such as the SRB. These findings are crucial for implementing effective water resource management strategies in the SRB and similar basins within the MRB, as well as for increasing local awareness of water resource utilization in agriculture.
We recognize that this study had certain limitations, primarily due to the unavailability of daily scale dam operation data as well as the poor performance of the SWAT reservoir module that can be used only with constant setup for long-term simulation. This resulted in uncertainties related to the dam’s operation during the SRB study periods and during extreme events. Besides, we did not consider the effects of deforestation caused by constructing these reservoirs that could affect the flow regimes. In addition, the climate change scenarios should be updated to CMIP6, which contains socioeconomic and human impacts. Besides, previous studies in this region have compared satellite-based precipitation with in-situ rain-gauge observations (Mohammed et al., 2018a; 2018b; Le et al., 2020); downscaled soil moisture (Dandridge et al., 2020); estimation of flooding using satellite data sets (Fayne et al., 2017) and droughts (Lakshmi et al., 2023); combination with socio-economic data (Tiwari et al., 2023); and land-use land cover (Spruce et al., 2020; Nguyen et al., 2022). Thus, it is necessary to implement these data sets to provide more precise results. Such improvements would improve the understanding of city and country officials concerning water resource management and hazard mitigation in the SRB and MRB.
The original contributions presented in the study are included in the article/Supplementary material, further inquiries can be directed to the corresponding authors.
T-N-DT: Conceptualization, Data curation, Formal Analysis, Funding acquisition, Investigation, Methodology, Resources, Software, Validation, Visualization, Writing–original draft, Writing–review and editing. BN: Conceptualization, Data curation, Formal Analysis, Investigation, Methodology, Resources, Software, Validation, Visualization, Writing–review and editing. MG-L: Funding acquisition, Project administration, Supervision, Writing–review and editing. GS: Funding acquisition, Project administration, Supervision, Writing–review and editing. VL: Project administration, Supervision, Writing–review and editing.
The author(s) declare financial support was received for the research, authorship, and/or publication of this article. This study is funded by the Faculty of Building Services, Hydro and Environmental Engineering, and Warsaw University of Technology, Warszawa, Poland, under the representative of MG-Ł (Grant ID: VAT 525-000-58-34).
We also would like to express our sincere gratitude to reviewers for their constructive comments to improve the quality of our manuscript. Special acknowledgment also goes to reviewers for their constructive comments and support.
The authors declare that the research was conducted in the absence of any commercial or financial relationships that could be construed as a potential conflict of interest.
All claims expressed in this article are solely those of the authors and do not necessarily represent those of their affiliated organizations, or those of the publisher, the editors and the reviewers. Any product that may be evaluated in this article, or claim that may be made by its manufacturer, is not guaranteed or endorsed by the publisher.
Abbaspour, K. C., Rouholahnejad, E., Vaghefi, S., Srinivasan, R., Yang, H., and Kløve, B. (2015). A continental-scale hydrology and water quality model for Europe: calibration and uncertainty of a high-resolution large-scale SWAT model. J. Hydrology 524, 733–752. doi:10.1016/j.jhydrol.2015.03.027
Ahmed, Z., Tran, T. N. D., and Nguyen, Q. B. (2020). “Applying semi distribution hydrological model SWAT to assess hydrological regime in Lai Giang catchment, Binh Dinh Province, Vietnam,” in Proceedings of the 2nd Conference on Sustainability in Civil Engineering (CSCE’20), Islamabad, Pakistan (Pakistan: Capital University of Science and Technology). https://csce.cust.edu.pk/archive/20-404.pdf.
Anaba, L. A., Banadda, N., Kiggundu, N., Wanyama, J., Engel, B., and Moriasi, D. (2017). Application of SWAT to assess the effects of land use change in the murchison bay catchment in Uganda. Comput. Water, Energy, Environ. Eng. 06 (01), 24–40. doi:10.4236/cweee.2017.61003
Arias, M. E., Cochrane, T. A., Kummu, M., Lauri, H., Holtgrieve, G. W., Koponen, J., et al. (2014a). Impacts of hydropower and climate change on drivers of ecological productivity of Southeast Asia’s most important wetland. Ecol. Model. 272, 252–263. doi:10.1016/j.ecolmodel.2013.10.015
Arias, M. E., Piman, T., Lauri, H., Cochrane, T. A., and Kummu, M. (2014b). Dams on Mekong tributaries as significant contributors of hydrological alterations to the Tonle Sap Floodplain in Cambodia. Hydrology Earth Syst. Sci. 18 (12), 5303–5315. doi:10.5194/hess-18-5303-2014
Arnold, J., Kiniry, J. R., Srinivasan, R., Williams, J. R., Haney, E., and Neitsch, S. (2012a). Swat input data. Res (Chapter 29). 393–406 https://swat.tamu.edu/docs/.
Arnold, J. G., Moriasi, D. N., Gassman, P. W., Abbaspour, K. C., White, M. J., Srinivasan, R., et al. (2012b). SWAT: model use, calibration, and validation. Trans. ASABE 55 (4), 1491–1508. doi:10.13031/2013.42256
Arshad, A., Mirchi, A., Samimi, M., and Ahmad, B. (2022). Combining downscaled-GRACE data with SWAT to improve the estimation of groundwater storage and depletion variations in the Irrigated Indus Basin (IIB). Sci. Total Environ. 838 (April), 156044. doi:10.1016/j.scitotenv.2022.156044
Arshad, A., Zhang, W., Zhang, Z., Wang, S., Zhang, B., Jehanzeb, M., et al. (2021). Reconstructing high-resolution gridded precipitation data using an improved downscaling approach over the high altitude mountain regions of Upper Indus Basin (UIB). Sci. Total Environ. 784, 147140. doi:10.1016/j.scitotenv.2021.147140
Aryal, A., Shrestha, S., and Babel, M. S. (2019). Quantifying the sources of uncertainty in an ensemble of hydrological climate-impact projections. Theor. Appl. Climatol. 135 (1–2), 193–209. doi:10.1007/s00704-017-2359-3
Aryal, A., Tran, T. N. D., Kim, K. Y., Rajaram, H., Lakshmi, V., and Venkat), (2022). Climate and land use/land cover change impacts on hydrological processes in the mountain watershed of gandaki River Basin, Nepal. Chicago: AGU Fall Meeting Abstracts, H52L.0615
Aryal, A., Tran, T. N. D., Kumar, B., and Lakshmi, V. (2023). Evaluation of satellite-derived precipitation products for streamflow simulation of a mountainous himalayan watershed: a study of myagdi khola in kali gandaki. Remote Sens. 15(19), 47–62. doi:10.3390/rs15194762
Ashrafi, S., Kerachian, R., Pourmoghim, P., Behboudian, M., and Motlaghzadeh, K. (2022a). Evaluating and improving the sustainability of ecosystem services in river basins under climate change. Sci. Total Environ. 806, 150702. doi:10.1016/j.scitotenv.2021.150702
Ashrafi, S., Khoie, M. M. M., Kerachian, R., and Shafiee-Jood, M. (2022b). Managing basin-wide ecosystem services using the bankruptcy theory. Sci. Total Environ. 842 (June), 156845. doi:10.1016/j.scitotenv.2022.156845
Aslam, R. A., Shrestha, S., Usman, M. N., Khan, S. N., Ali, S., Sharif, M. S., et al. (2022). Integrated SWAT-MODFLOW modeling-based groundwater adaptation policy guidelines for lahore, Pakistan under projected climate change, and human development scenarios. Atmosphere 13 (12), 2001. doi:10.3390/atmos13122001
Bajracharya, A. R., Bajracharya, S. R., Shrestha, A. B., and Maharjan, S. B. (2018). Climate change impact assessment on the hydrological regime of the Kaligandaki Basin, Nepal. Sci. Total Environ. 625, 837–848. doi:10.1016/j.scitotenv.2017.12.332
Bhatta, B., Shrestha, S., Shrestha, P. K., and Talchabhadel, R. (2019). Evaluation and application of a SWAT model to assess the climate change impact on the hydrology of the Himalayan River Basin. Catena 181, 104082. doi:10.1016/j.catena.2019.104082
Bolch, T., Kulkarni, A., Kääb, A., Huggel, C., Paul, F., Cogley, J. G., et al. (2012). The state and fate of himalayan glaciers. Science 336 (6079), 310–314. doi:10.1126/science.1215828
Bourdeau-Goulet, S. C., and Hassanzadeh, E. (2021). Comparisons between CMIP5 and CMIP6 models: simulations of climate indices influencing food security, infrastructure resilience, and human health in Canada. Earth’s Future 9 (5). doi:10.1029/2021EF001995
Bui, T. T. P., Kantoush, S., Kawamura, A., Du, T. L. T., Bui, N. T., Capell, R., et al. (2023). Reservoir operation impacts on streamflow and sediment dynamics in the transboundary river basin, Vietnam. Hydrol. Process. 37 (9). doi:10.1002/hyp.14994
Chang, C., Lee, H., Do, S. K., Du, T. L. T., Markert, K., Hossain, F., et al. (2023). Operational forecasting inundation extents using REOF analysis (FIER) over lower Mekong and its potential economic impact on agriculture. Environ. Model. Softw. 162 (August 2022), 105643. doi:10.1016/j.envsoft.2023.105643
Chattopadhyay, S., Edwards, D. R., Yu, Y., and Hamidisepehr, A. (2017). An assessment of climate change impacts on future water availability and droughts in the Kentucky River Basin. Environ. Process. 4 (3), 477–507. doi:10.1007/s40710-017-0259-2
Chen, C. A., Hsu, H. H., and Liang, H. C. (2021). Evaluation and comparison of CMIP6 and CMIP5 model performance in simulating the seasonal extreme precipitation in the Western North Pacific and East Asia. Weather Clim. Extrem. 31, 100303. doi:10.1016/j.wace.2021.100303
Cochrane, T. A., Arias, M. E., and Piman, T. (2014). Historical impact of water infrastructure on water levels of the Mekong River and the Tonle Sap system. Hydrology Earth Syst. Sci. 18 (11), 4529–4541. doi:10.5194/hess-18-4529-2014
Dandridge, C., Fang, B., and Lakshmi, V. (2020). Downscaling of SMAP soil moisture in the lower Mekong River Basin. Water 12, 56. doi:10.3390/w12010056
Du, T. L. T., Lee, H., Bui, D. D., Graham, L. P., Darby, S. D., Pechlivanidis, I. G., et al. (2022). Streamflow prediction in highly regulated, transboundary watersheds using multi-basin modeling and remote sensing imagery. Water Resour. Res. 58 (3), e2021WR031191–25. doi:10.1029/2021WR031191
Fayne, J., Bolten, J., Fuhrmann, S., Rice, M., Houser, P., and Lakshmi, V. (2017). Flood mapping in the lower mekong basin using MODIS observations. Int. J. Remote Sens. doi:10.1080/01431161.2017.1285503
Fita, L., Fernández, J., Fernández, F., and García-Díez, M. (2010). CLWRF: WRF modifications for regional climate simulation under future scenarios. http://www.meteo.unican.es/wiki/cordexwrf.
Gassman, P. W., Reyes, M. R., Green, C. H., and Arnold, J. G. (2007). The soil and water assessment Tool: historical development, applications, and future research directions. Trans. ASABE 50 (4), 1211–1250. doi:10.13031/2013.23637
Giang, N. T., Thao, H. T., Vinh, T. N., Duy, P., Binh, H., and Quan, V. D. (2017). Study on sediment regime changes in downstream of the Ba River, Vietnam under the impact of reservoirs system. VNU J. Sci. Earth Environ. Sci. 4, 127–134. doi:10.25073/2588-1094/vnuees.4217
Giang, P. Q., and Vy, T. T. (2021). Will climate change exacerbate the economic damage of flood to agricultural production? A case study of rice in ha tinh Province, vietnam. Front. Environ. Sci. 9 (June), 1–13. doi:10.3389/fenvs.2021.643947
Her, K., and Hawkins, E. (2014). Climate projections. Taiwan Rev. 64 (11), 38–60. doi:10.1079/9781780641973.0038
Hussain, A., Zaib, K., Khalil, J., Rahman, U., Shang, S., Ejaz, N., et al. (2022). Analyzing the impact of drought on agriculture: evidence from Pakistan using standardized precipitation evapotranspiration index. Nat. Hazards 115, 389–408. doi:10.1007/s11069-022-05559-6
Huyen, N. T., Tu, L. H., Tram, V. N. Q., Minh, D. N., Liem, N. D., and Loi, N. K. (2017). Assessing the impacts of climate change on water resources in the srepok watershed, central highland of Vietnam. J. Water Clim. Change 8 (3), 524–534. doi:10.2166/wcc.2017.135
International Rivers (2010). Srepok dams. https://archive.internationalrivers.org/campaigns/srepok-dams.
Ivanov, V. Y., Xu, D., Dwelle, M. C., Sargsyan, K., Wright, D. B., Katopodes, N., et al. (2021). Breaking down the computational barriers to real-time urban flood forecasting. Geophys. Res. Lett. 48, 1–12. doi:10.1029/2021GL093585
Khoi, D. N., and Suetsugi, T. (2012). Uncertainty in climate change impacts on streamflow in Be River Catchment, Vietnam. Vietnam. Water Environ. J. 26 (4), 530–539. doi:10.1111/j.1747-6593.2012.00314.x
Kim, H., and Parajuli, P. B. (2014). Impacts of reservoir outflow estimation methods in SWAT model calibration. Trans. ASABE 57 (4), 1029–1042. doi:10.13031/trans.57.10156
Lakshmi, V., Le, M.-H., Goffin, B., Besnier, J., Pham, H., Do, H., et al. (2023). Regional analysis of the 2015–16 Lower Mekong River basin drought using NASA satellite observations. J. Hydrology, Regional Stud. 46, 101362. doi:10.1016/j.ejrh.2023.101362
Le, M.-H., Lakshmi, V., Bolten, J., and Bui, D. (2020). Adequacy of satellite-derived precipitation estimate for hydrological modeling in vietnam basins. J. Hydrology 586, 124820. doi:10.1016/j.jhydrol.2020.124820
Lehner, F., Wood, A. W., Vano, J. A., Lawrence, D. M., Clark, M. P., and Mankin, J. S. (2019). The potential to reduce uncertainty in regional runoff projections from climate models. Nat. Clim. Change 9 (12), 926–933. doi:10.1038/s41558-019-0639-x
Liang, X., Lettenmaier, D. P., Wood, E. F., and Burges, S. J. (1994). A simple hydrologically based model of land surface water and energy fluxes for general circulation models. J. Geophys. Res. 99 (Issue D7), 14415–14428. doi:10.1029/94JD00483
Ministry of Natural Resources and Environment of The Socialist Republic of Vietnam. (2016). Approval of the Paris agreement to implement the united Nations framework convention on climate change 7, 1.
Mizuta, R., Yoshimura, H., Murakami, H., Matsueda, M., Endo, H., Ose, T., et al. (2012). Climate simulations using MRI-AGCM3.2 with 20-km grid. J. Meteorological Soc. Jpn. 90 (A), 233–258. doi:10.2151/jmsj.2012-A12
Moberg, A., and Jones, P. D. (2004). Regional climate model simulations of daily maximum and minimum near-surface temperatures across Europe compared with observed station data 1961-1990. Clim. Dyn. 23 (7–8), 695–715. doi:10.1007/s00382-004-0464-3
Mohammed, I., J Bolten, R. S., and Lakshmi, V. (2018a). Improved hydrological decision support system for the Lower Mekong River Basin using satellite-based earth observations. Remote Sens. 10, 885. doi:10.3390/rs10060885
Mohammed, I., J Bolten, R. S., and Lakshmi, V. (2018b). Satellite observations and modeling to understand the Lower Mekong River Basin streamflow variability. J. Hydrology 564, 559–573. doi:10.1016/j.jhydrol.2018.07.030
Mosavi, A., Golshan, M., Choubin, B., Ziegler, A. D., Sigaroodi, S. K., Zhang, F., et al. (2021). Fuzzy clustering and distributed model for streamflow estimation in ungauged watersheds. Sci. Rep. 11, 8243–8314. doi:10.1038/s41598-021-87691-0
MRC (2017). “The council study: the study on sustainable management and development of the Mekong River Basin, including impacts of mainstream hydropower projects,” in Thematic report on the positive and negative impacts of hydropower development on the social (Lao PDR: Environmen. Mrc), 107.
National Institute for Soils and Fertilizers (2002). The basic information of main soil units of Vietnam. Vietnam: The Gioi Publishers.
Nguyen, B. Q., Kantoush, S., Binh, D. V., Saber, M., Vo, D. N., and Sumi, T. (2023a). Quantifying the impacts of hydraulic infrastructure on tropical streamflows. Hydrol. Process. 37 (3). doi:10.1002/hyp.14834
Nguyen, B. Q., Kantoush, S., Binh, D. V., Saber, M., Vo, D. N., and Sumi, T. (2023b). Understanding the anthropogenic development impacts on long-term flow regimes in a tropical river basin, Central Vietnam. Hydrological Sci. J. 68, 341–354. doi:10.1080/02626667.2022.2153298
Nguyen, B. Q., Tran, T. N. D., Łukaszewska, M. G.-, Sinicyn, G., and Lakshmi, V. (2022). Assessment of urbanization-induced land-use change and its impact on temperature, evaporation, and humidity in central vietnam. Humidity Central Vietnam 14 (21), 3367. doi:10.3390/w14213367
Nguyen, D. N., and Nguyen, T. H. (2004). Climate and climate resources in Vietnam (in Vietnamese). Vietnam: Agricultural Publishing House.
Nguyen, M. D., and Nguyen, H. T. (2013). Drought and water depletion during the 2012-2013 dry season in the Central Highlands. Vietnam: Daklak News. https://baodaklak.vn/channel/3461/201304/han-han-va-can-kiet-nguon-nuoc-trong-mua-kho-nam-2012-2013-o-tay-nguyen-2229396/.
Nguyen, N. T., Du, T. L. T., Park, H., Chang, C.-H., Choi, S., Chae, H., et al. (2023). Estimating the impacts of ungauged reservoirs using publicly available streamflow simulations and satellite remote sensing. Remote Sens. 15 (18), 4563. doi:10.3390/rs15184563
Noor, R., Arshad, A., Shafeeque, M., Liu, J., Baig, A., Ali, S., et al. (2023). Combining APHRODITE rain gauges-based precipitation with downscaled-TRMM data to translate high-resolution precipitation estimates in the indus basin. Remote Sens. 15 (2), 318. doi:10.3390/rs15020318
Nuong, B. T., Linh, B. K., Trang, V. Q., Huyen, P. T., Thi, B., Thao, P., et al. (2022). Applying fuzzy analytical hierarchy process to establish environmental sustainability indicators for water resources Srepok River Basin, vietnam. VNU J. Sci. Earth Environ. Sci. 38 (4), 63–74. doi:10.25073/2588-1094/vnuees.4877
Pathak, S., Ojha, C. S. P., Shukla, A. K., and Garg, R. D. (2019). Assessment of annual water-balance models for diverse Indian watersheds. J. Sustain. Water Built Environ. 5 (3). doi:10.1061/jswbay.0000881
Pierce, D. W., Barnett, T. P., Santer, B. D., and Gleckler, P. J. (2009). Selecting global climate models for regional climate change studies. Proc. Natl. Acad. Sci. U. S. A. 106 (21), 8441–8446. doi:10.1073/pnas.0900094106
Piman, T., Cochrane, T. A., and Arias, M. E. (2016). Effect of proposed large dams on water flows and hydropower production in the Sekong, sesan and srepok rivers of the mekong basin. River Res. Appl. 32 (10), 2095–2108. doi:10.1002/rra.3045
Piman, T., Lennaerts, T., and Southalack, P. (2013). Assessment of hydrological changes in the lower mekong basin from basin-wide development scenarios. Hydrol. Process. 27 (15), 2115–2125. doi:10.1002/hyp.9764
Pokhrel, Y., Tiwari, A., Kramer, D., Akhter, T., Tang, Q., Liu, J., et al. (2023). A synthesis of hydroclimatic, ecological, and socioeconomic data for transdisciplinary research in the Mekong. Sci. Data 10 (1), 283. doi:10.1038/s41597-023-02193-0
Qiu, J., Yang, Q., Zhang, X., Huang, M., Adam, J. C., and Malek, K. (2019). Implications of water management representations for watershed hydrologic modeling in the Yakima River basin. Hydrology Earth Syst. Sci. 23 (1), 35–49. doi:10.5194/hess-23-35-2019
Raghavan, S. V., Vu, M. T., and Liong, S. Y. (2012). Assessment of future stream flow over the sesan catchment of the lower mekong basin in vietnam. Hydrol. Process. 26 (24), 3661–3668. doi:10.1002/hyp.8452
Ranger, N., Hallegatte, S., Bhattacharya, S., Bachu, M., Priya, S., Dhore, K., et al. (2011). An assessment of the potential impact of climate change on flood risk in Mumbai. Clim. Change 104 (1), 139–167. doi:10.1007/s10584-010-9979-2
Roderick, T. P., Wasko, C., and Sharma, A. (2019). Atmospheric moisture measurements explain increases in tropical rainfall extremes. Geophys. Res. Lett. 46 (3), 1375–1382. doi:10.1029/2018GL080833
Samuelsson, P., Jones, C. G., Willén, U., Ullerstig, A., Gollvik, S., Hansson, U., et al. (2011). The Rossby Centre regional climate model RCA3: model description and performance. Tellus, Ser. A Dyn. Meteorology Oceanogr. 63 (1), 4–23. doi:10.1111/j.1600-0870.2010.00478.x
Shafeeque, M., Hafeez, M., Sarwar, A., Arshad, A., Khurshid, T., Asim, M. I., et al. (2023a). Quantifying future water - saving potential under climate change and groundwater recharge scenarios in Lower Chenab Canal, Indus River Basin. Theor. Appl. Climatol. doi:10.1007/s00704-023-04621-y
Shafeeque, M., Luo, Y., Arshad, A., Muhammad, S., Ashraf, M., and Pham, Q. B. (2023b). Assessment of climate change impacts on glacio - hydrological processes and their variations within critical zone. Nat. Hazards 115 (3), 2721–2748. doi:10.1007/s11069-022-05661-9
Shukla, A. K., Ojha, C. S. P., Garg, R. D., Shukla, S., and Pal, L. (2020). Influence of spatial urbanization on hydrological components of the upper ganga River Basin, India. J. Hazard. Toxic, Radioact. Waste 24 (4). doi:10.1061/(asce)hz.2153-5515.0000508
Smigaj, M., Hackney, C. R., Kieu, P., Dang, V. P., Ngoc, N. T., Du, D., et al. (2023). Monitoring riverine traffic from space: the untapped potential of remote sensing for measuring human footprint on inland waterways. Sci. Total Environ. 860 (September 2022), 160363. doi:10.1016/j.scitotenv.2022.160363
Spruce, J., Bolten, J., Mohammed, I., Srinivasan, R., and Lakshmi, V. (2020). Mapping land use land cover change in the lower mekong basin from 1997 to 2010. Front. Environ. Sci. Sect. Land Use Dyn. 8. doi:10.3389/fenvs.2020.00021
Tapas, M., Etheridge, J. R., Howard, G., Lakshmi, V. V., and Tran, T. N. D. (2022). Development of a socio-hydrological model for a coastal watershed: using stakeholders’ perceptions. Chicago: AGU Fall Meeting Abstracts, H22O–H0996.
Tran, T., Nguyen, Q. B., Tam, D., Le, L., Nguyen, T. D., Vo, N. D., et al. (2022d). Evaluate the influence of groynes system on the hydraulic regime in the ha thanh river, binh dinh Province, vietnam. In Advances in hydroinformatics, 241–254. doi:10.1007/978-981-19-1600-7_15
Tran, T.-N.-D., and Lakshmi, V. (2022). The land use changes impacts on socio-economic drivers and simulation of surface and groundwater in the Eastern Shore of Virginia, the United States. Chicago: AGU Fall Meeting Abstracts, H42D–H1270.
Tran, T.-N.-D., Le, M.-H., Zhang, R., Nguyen, B. Q., Bolten, J. D., and Lakshmi, V. (2023a). Robustness of gridded precipitation products for vietnam basins using the comprehensive assessment framework of rainfall. Atmos. Res. 293 (15), 106923. doi:10.1016/j.atmosres.2023.106923
Tran, T. N. D., Nguyen, B. Q., Le, M.-H., Lakshmi, V., Venkat), , Bolten, J. D., et al. (2022a). Robustness of gridded precipitation products in hydrological assessment for Vietnam river basins. Chicago: AGU Fall Meeting Abstracts, H22M–H07.
Tran, T. N. D., Nguyen, Q. B., Nguyen, T. T., Vo, N. D., Nguyen, C. P., and Gourbesville, P. (2022c). “Operational methodology for the assessment of typhoon waves characteristics. Application to ninh thuan Province, vietnam,” in Advances in hydroinformatics, 887–902. doi:10.1007/978-981-19-1600-7_55
Tran, T. N. D., Nguyen, Q. B., Vo, N. D., Le, M. H., Nguyen, Q. D., Lakshmi, V., et al. (2023c). Quantification of global Digital Elevation Model (DEM) – a case study of the newly released NASADEM for a river basin in Central Vietnam. J. Hydrology Regional Stud. 45 (October 2022), 101282. doi:10.1016/j.ejrh.2022.101282
Tran, T. N. D., Nguyen, Q. B., Vo, N. D., Marshall, R., and Gourbesville, P. (2022b). Assessment of terrain scenario impacts on hydrological simulation with SWAT model. Application to lai Giang catchment, vietnam. Adv. Hydroinformatics, 1205–1222. doi:10.1007/978-981-19-1600-7_77
Tran, T. N. D., Nguyen, Q. B., and Zeeshan, A. (2021a). Application of plaxis for calculating the construction stability and soft embankment in protecting ha thanh. 2nd conference on sustainability in Civil engineering (CSCE) 2020, 202–210. https://csce.cust.edu.pk/archive/20-613.pdf.
Tran, T.-N.-D., Nguyen, Q. B., Zhang, R., Aryal, A., Łukaszewska, M.-G., Sinicyn, G., et al. (2023b). Quantification of gridded precipitation products for the streamflow simulation on the Mekong River Basin using rainfall assessment framework: a case study for the Srepok River subbasin, central highland vietnam. Remote Sens. 15 (4), 10–30. doi:10.3390/rs15041030
Tran, T. N. D., Zeeshan, A., and Vo, N. D. (2021b). “Application of hydrodynamic modelling to assess the efficiency of hurricane protection measure at xom ro dike,” in PHU YEN PROVINCE, VIETNAM. 2nd Conference on Sustainability in Civil Engineering (CSCE). https://csce.cust.edu.pk/archive/20-406.pdf.
Tuo, Y., Duan, Z., Disse, M., and Chiogna, G. (2016). Evaluation of precipitation input for SWAT modeling in Alpine catchment: a case study in the Adige river basin (Italy). Sci. Total Environ. 573, 66–82. doi:10.1016/j.scitotenv.2016.08.034
Ty, T. V., Sunada, K., and Ichikawa, Y. (2011). A spatial impact assessment of human-induced intervention on hydrological regimes: a case study in the upper Srepok river basin, central highlands of vietnam. Int. J. River Basin Manag. 9 (2), 103–116. doi:10.1080/15715124.2011.595720
Ty, T. V., Sunada, K., Ichikawa, Y., and Oishi, S. (2012). Scenario-based impact assessment of land use/cover and climate changes on water resources and demand: a case study in the Srepok River Basin, vietnam-Cambodia. Water Resour. Manag. 26 (5), 1387–1407. doi:10.1007/s11269-011-9964-1
Umar, M., Nasir, S., Arfan, K., Ammar, R., Hafiz, A., Safdar, M., et al. (2022). A modified approach to quantify aquifer vulnerability to pollution towards sustainable groundwater management in Irrigated Indus Basin. Environ. Sci. Pollut. Res. 29, 27257–27278. doi:10.1007/s11356-021-17882-9
Vo, N. D., Nguyen, T. H., Vu, H. C., Gourbesville, P., and Nguyen, Q. B. (2018). Effects of climate change on streamflow in kon – ha thanh river watershed. Vietnam 3 (September), 2233–2224. doi:10.29007/1hrc
Xu, F., Dong, G., Wang, Q., Liu, L., Yu, W., Men, C., et al. (2016). Impacts of DEM uncertainties on critical source areas identification for non-point source pollution control based on SWAT model. J. Hydrology 540, 355–367. doi:10.1016/j.jhydrol.2016.06.019
Keywords: climate change, reservoir, SWAT, Srepok River basin, Mekong River basin
Citation: Tran T-N-D, Nguyen BQ, Grodzka-Łukaszewska M, Sinicyn G and Lakshmi V (2023) The role of reservoirs under the impacts of climate change on the Srepok River basin, Central Highlands of Vietnam. Front. Environ. Sci. 11:1304845. doi: 10.3389/fenvs.2023.1304845
Received: 30 September 2023; Accepted: 13 November 2023;
Published: 23 November 2023.
Edited by:
Prof-Maged Marghany, Syiah Kuala University, IndonesiaReviewed by:
Anoop Kumar Shukla, Manipal Academy of Higher Education, IndiaCopyright © 2023 Tran, Nguyen, Grodzka-Łukaszewska, Sinicyn and Lakshmi. This is an open-access article distributed under the terms of the Creative Commons Attribution License (CC BY). The use, distribution or reproduction in other forums is permitted, provided the original author(s) and the copyright owner(s) are credited and that the original publication in this journal is cited, in accordance with accepted academic practice. No use, distribution or reproduction is permitted which does not comply with these terms.
*Correspondence: Thanh-Nhan-Duc Tran, c3l1M2NzQHZpcmdpbmlhLmVkdQ==; Binh Quang Nguyen, bnFiaW5oQGR1dC51ZG4udm4=
Disclaimer: All claims expressed in this article are solely those of the authors and do not necessarily represent those of their affiliated organizations, or those of the publisher, the editors and the reviewers. Any product that may be evaluated in this article or claim that may be made by its manufacturer is not guaranteed or endorsed by the publisher.
Research integrity at Frontiers
Learn more about the work of our research integrity team to safeguard the quality of each article we publish.