- 1Yangtze River Basin Ecological Environment Monitoring and Scientific Research Center, Yangtze River Basin Ecological Environment Supervision and Administration Bureau, Ministry of Ecology and Environment, Wuhan, China
- 2Department of Transdisciplinary Science and Engineering, Tokyo Institute of Technology, Tokyo, Japan
- 3State Key Laboratory of Freshwater Ecology and Biotechnology, Institute of Hydrobiology, Chinese Academy of Sciences, Wuhan, China
- 4College of Advanced Agricultural Sciences, University of Chinese Academy of Sciences, Beijing, China
1 Introduction
Biodiversity plays a crucial role in enhancing resource use efficiency, ecosystem functioning, and stability of ecological processes in the Anthropocene (Balvanera et al., 2006; Cardinale et al., 2006). However, human activities and biological invasions have led to widespread loss of biodiversity on Earth, posing threats to aspects such as food and medicine supply and ecosystem functioning regulation (McGeoch et al., 2010; Diaz et al., 2019). Therefore, accurately measuring biodiversity and predicting its temporal and spatial changes have been central research focuses in ecology (Solow and Polasky, 1994; Cardinale et al., 2012). Through continuous efforts by ecologists, methods for measuring biodiversity and analytical techniques have been constantly evolving and improving (Solow and Polasky, 1994; Legendre and Legendre, 2012). The development and refinement of these methods have enhanced our scientific understanding of life diversity on Earth and facilitated the conservation and management of biodiversity.
Among numerous methods for measuring and analyzing biodiversity, the dissimilarity-based models [DM; e.g., the Mantel tests (MT) and generalized dissimilarity model (GDM)] are the most commonly used for analyzing and predicting the spatiotemporal turnover patterns of communities (Xu et al., 2022). The Mantel test can assess the linear correlation of community changes with environmental variation (Mantel, 1967). Smouse et al. (1986) extended the statistical methods of MT, allowing for multivariate regression and correlation analysis, thus enhancing the applicability and flexibility of MT. Blanchet et al. (2008) discussed the use of forward selection in MT to identify the most important explanatory variables, improving the interpretability and explanatory power of MT results. GDM can explore the non-linear regression between community distance and ecological distance (e.g., environmental and geographic distances), detecting the extent of community changes along environmental gradients and informing us about the contribution of each important environmental variable (Ferrier et al., 2007). GDM’s applications in the analysis of biodiversity time series data (Dornelas et al., 2014) and the assessment of regional biodiversity (Ferrier et al., 2007) fully demonstrate its value in predicting spatiotemporal turnover patterns of community composition, enhancing our understanding of biodiversity patterns and processes. However, GDM may involve uncertainties in handling diversity data, which can arise from spatial autocorrelation, data gaps, or measurement errors (Woolley et al., 2017).
In this opinion article, we propose a conceptual framework by expanding the capabilities of DMs to incorporate mediators, aiming to overcome the intrinsic limitations that hinder the exploration of dynamic patterns in beta diversity with robust empirical support. While existing DMs excel at unveiling associations between community composition and environmental or spatial factors, they typically fall short in directly identifying and elucidating the roles of moderators and their underlying mechanisms. Researchers often rely on independent models for various moderating factors (e.g., different geographic regions, seasons, or biological assemblages), rather than integrating these moderating influences into a unified analytical model (Ferrier et al., 2007). Mere numerical comparisons among the outcomes of these independent DMs do not inherently convey the significance of the moderating factors. Therefore, our approach responds to the pressing need for models that align with our proposed conceptual framework, offering a comprehensive understanding of the intricate ecological mechanisms at play within beta diversity patterns and addressing this research frontier with rigor and innovation.
2 Integration of moderators into the model
The comprehensive DM workflow consists of six key steps (Figure 1). First, data collection (Step 1) entails the gathering of biodiversity data from various locations, including site-specific details and species composition information. Next, a dissimilarity matrix (Step 2) is generated, employing appropriate dissimilarity metrics like the Bray-Curtis distance to quantify the differences in species composition across these locations. To delve deeper into ecological patterns, environmental data (e.g., temperature, precipitation, and habitat characteristics) and geographic coordinates (latitude and longitude or other spatial identifiers) are gathered for constructing environmental and spatial distance matrices (Step 3), effectively measuring disparities in environmental factors and spatial distances. Subsequently, statistical modeling techniques (Step 4) are applied, employing DM methodologies. Once the modeling analysis is complete, model evaluation (Step 5) comes into play, encompassing the assessment of the constructed DM, which includes evaluating model fit, predictive performance, and potential biases or errors, thereby ensuring the reliability and integrity of the analysis. Finally, results are interpreted (Step 6) to decipher how environmental factors influence species composition disparities and how spatial distance shapes species distribution patterns.
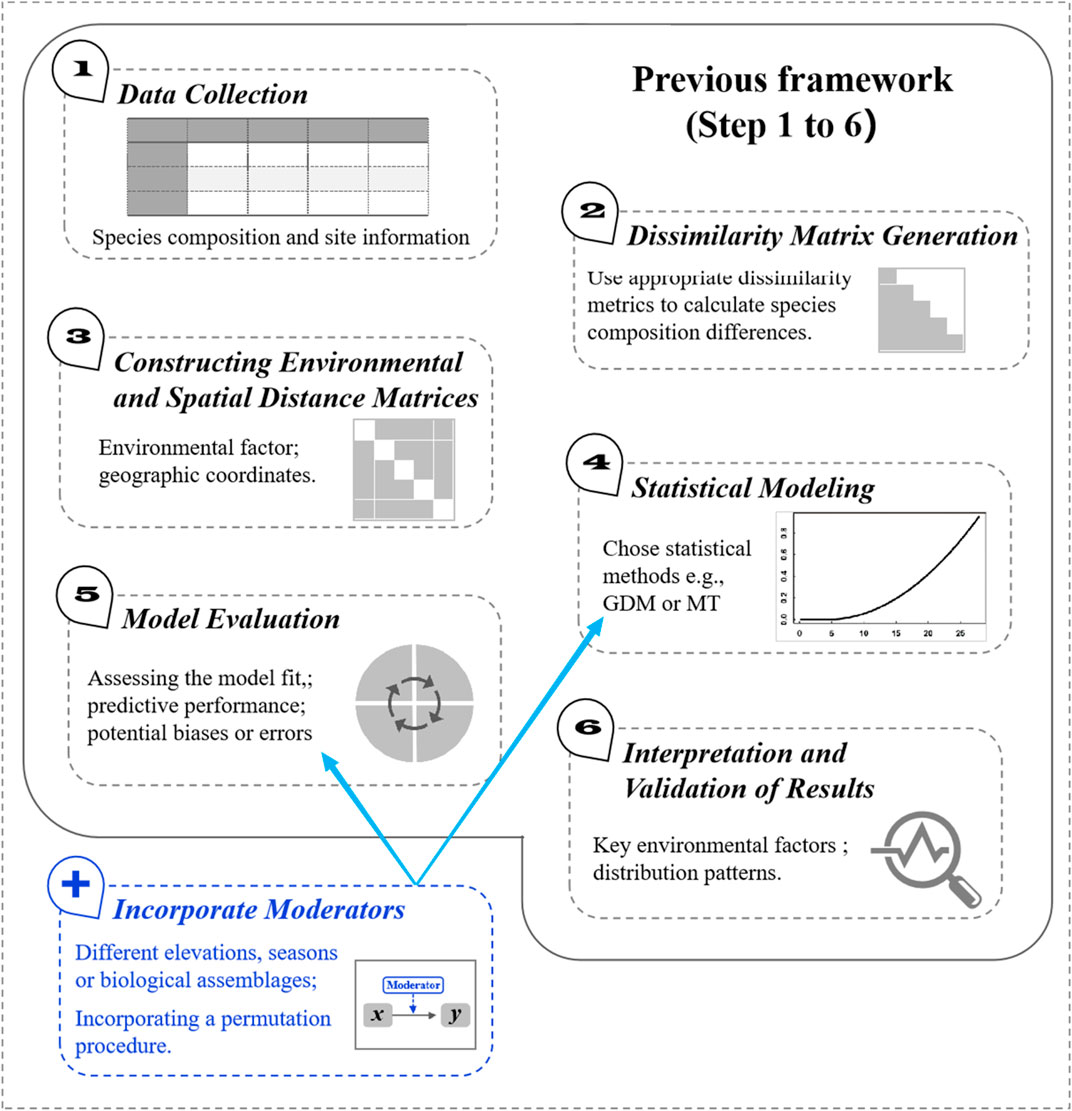
FIGURE 1. Flow chart to show the analytic procedures (Innovations in this study are marked in blue).
In the phase of applying the methodologies as they stand today, DMs construct the relationship between biological dissimilarity and environmental and/or spatial distance in the following manner:
where the function
Incorporating moderators into the DMs represents a significant advancement in our understanding of ecological relationships. In this context, the model formulates the intricate relationship between biological dissimilarity and environmental and/or spatial distance as follows:
where the function
Regarding the steps dedicated to model fitting and the critical phase of significance testing, our approach harmonizes seamlessly with the established framework. The process of model fitting remains consistent with the previous one when employing this framework. The model fitting can be achieved using the Iteratively Re-weighted Least Squares (IRLS) algorithm, However, it's in the domain of significance testing that our method introduces a valuable innovation.
While staying true to the core principles of the original DMs, we augment our approach by incorporating a permutation procedure that is different from the original procedure. To overcome the problem of lack of independence between site pairs, the original significance testing is performed using a random permutation procedure, which involves comparing the deviance explained by the fitted model with the deviance calculated when either individual or all predictor variables are randomly shuffled among the samples (Mokany et al., 2022). Rather than that, the procedure proposed in this article involves the systematic reshuffling of sample order, encompassing sites or time points, within a single matrix at each level of the moderator. For example, when taking elevation as a moderator, the samples could be re-permuted according to the elevation at which the samples were collected to obtain different matrices corresponding to each elevation. These matrices are then used to assess the significance of the effect of variables
3 Summary
Expanding upon our data collection methods, which encompass field surveys and remote sensing, our proposed framework plays a pivotal role in advancing our understanding of dynamic beta-diversity patterns in the Anthropocene. This progress is achieved by enhancing the capabilities of DMs through the incorporation of moderating variables, including but not limited to those related to spatiotemporal factors and biological traits. These moderating variables exert significant influence over the intricate interplay between environmental and biotic dissimilarities, ultimately shaping the dynamics of beta diversity. The innovation embedded within this conceptual framework not only amplifies the applicability of DMs but also propels scientific inquiry forward with a solid foundation of empirical evidence. Consequently, this advancement holds significant potential for strengthening the development of dependable management and conservation strategies in a rapidly changing world. In doing so, it contributes to the sustainability of our ecosystems and fosters a harmonious coexistence between humans and the environment.
Author contributions
SL: Conceptualization, Funding acquisition, Writing–original draft, Writing–review and editing. QL: Conceptualization, Funding acquisition, Writing–original draft, Writing–review and editing. MZ: Conceptualization, Writing–original draft. ZZ: Conceptualization, Writing–review and editing. RL: Conceptualization, Funding acquisition, Writing–original draft, Writing–review and editing.
Funding
The author(s) declare financial support was received for the research, authorship, and/or publication of this article. This research was funded by the National Key R&D Program of China (No. 2021YFC3200105), the National Natural Science Foundation of China (No. 32200173), and the China Scholarship Council (Nos 202203310007 and 202204910441).
Acknowledgments
We thank the editor and reviewer for their insightful comments and suggestions.
Conflict of interest
The authors declare that the research was conducted in the absence of any commercial or financial relationships that could be construed as a potential conflict of interest.
Publisher’s note
All claims expressed in this article are solely those of the authors and do not necessarily represent those of their affiliated organizations, or those of the publisher, the editors and the reviewers. Any product that may be evaluated in this article, or claim that may be made by its manufacturer, is not guaranteed or endorsed by the publisher.
References
Balvanera, P., Pfisterer, A. B., Buchmann, N., He, J. S., Nakashizuka, T., Raffaelli, D., et al. (2006). Quantifying the evidence for biodiversity effects on ecosystem functioning and services. Ecol. Lett. 9, 1146–1156. doi:10.1111/j.1461-0248.2006.00963.x
Blanchet, F. G., Legendre, P., and Borcard, D. (2008). Forward selection of explanatory variables. Ecology 89, 2623–2632. doi:10.1890/07-0986.1
Cardinale, B. J., Duffy, J. E., Gonzalez, A., Hooper, D. U., Perrings, C., Venail, P., et al. (2012). Biodiversity loss and its impact on humanity. Nature 486, 59–67. doi:10.1038/nature11148
Cardinale, B. J., Srivastava, D. S., Duffy, J. E., Wright, J. P., Downing, A. L., Sankaran, M., et al. (2006). Effects of biodiversity on the functioning of trophic groups and ecosystems. Nature 443, 989–992. doi:10.1038/nature05202
Diaz, S., Settele, J., Brondizio, E. S., Ngo, H. T., Agard, J., Arneth, A., et al. (2019). Pervasive human-driven decline of life on Earth points to the need for transformative change. Science 366, eaax3100. doi:10.1126/science.aax3100
Dornelas, M., Gotelli, N. J., McGill, B., Shimadzu, H., Moyes, F., Sievers, C., et al. (2014). Assemblage time series reveal biodiversity change but not systematic loss. Science 344, 296–299. doi:10.1126/science.1248484
Ferrier, S., Manion, G., Elith, J., and Richardson, K. (2007). Using generalized dissimilarity modelling to analyse and predict patterns of beta diversity in regional biodiversity assessment. Divers. Distributions 13, 252–264. doi:10.1111/j.1472-4642.2007.00341.x
Mantel, N. (1967). The detection of disease clustering and a generalized regression approach. Cancer Res. 27, 209–220.
McGeoch, M. A., Butchart, S. H. M., Spear, D., Marais, E., Kleynhans, E. J., Symes, A., et al. (2010). Global indicators of biological invasion: species numbers, biodiversity impact and policy responses. Divers. Distributions 16, 95–108. doi:10.1111/j.1472-4642.2009.00633.x
Mokany, K., Ware, C., Woolley, S. N. C., Ferrier, S., and Fitzpatrick, M. C. (2022). A working guide to harnessing generalized dissimilarity modelling for biodiversity analysis and conservation assessment. Glob. Ecol. Biogeogr. 31, 802–821. doi:10.1111/geb.13459
Smouse, P. E., Long, J. C., and Sokal, R. R. (1986). Multiple regression and correlation extensions of the Mantel test of matrix correspondence. Syst. Zool. 35, 627–632. doi:10.2307/2413122
Solow, A. R., and Polasky, S. (1994). Measuring biological diversity. Environ. Ecol. Statistics 1, 95–103. doi:10.1007/bf02426650
Woolley, S. N., Foster, S. D., O'Hara, T. D., Wintle, B. A., and Dunstan, P. K. (2017). Characterising uncertainty in generalised dissimilarity models. Methods Ecol. Evol. 8, 985–995. doi:10.1111/2041-210X.12710
Xu, Y. P., Xiang, Z. L., Rizo, E. Z., Naselli-Flores, L., and Han, B. P. (2022). Combination of linear and nonlinear multivariate approaches effectively uncover responses of phytoplankton communities to environmental changes at regional scale. J. Environ. Manag. 305, 114399. doi:10.1016/j.jenvman.2021.114399
Keywords: biodiversity, ecosystem functioning, ecological processes, generalized dissimilarity model, Mantel tests
Citation: Li S, Luo Q, Zhang M, Zhou Z and Li R (2023) Dynamic patterns of beta diversity in the Anthropocene: incorporating moderators into dissimilarity-based models. Front. Environ. Sci. 11:1302447. doi: 10.3389/fenvs.2023.1302447
Received: 26 September 2023; Accepted: 20 October 2023;
Published: 30 October 2023.
Edited by:
Jhih-Rong Liao, Tokyo Metropolitan University, JapanReviewed by:
Cheng Guojun, South-Central Minzu University, ChinaCopyright © 2023 Li, Luo, Zhang, Zhou and Li. This is an open-access article distributed under the terms of the Creative Commons Attribution License (CC BY). The use, distribution or reproduction in other forums is permitted, provided the original author(s) and the copyright owner(s) are credited and that the original publication in this journal is cited, in accordance with accepted academic practice. No use, distribution or reproduction is permitted which does not comply with these terms.
*Correspondence: Ruiwen Li, bHJ3MTk4NkAxMjYuY29t
†These authors have contributed equally to this work