- 1School of Economics and Management, Jiangxi Teachers College, Yingtan, China
- 2School of Economics and Management, East China Jiaotong University, Nanchang, China
The “14th Five-Year Plan” represents a crucial phase in enhancing financial inclusivity in China and addressing the challenge of “peak carbon emissions.” Given the digital economy’s rapid growth, China must urgently devise a model for high-quality development that combines digital finance with eco-friendly and low-carbon strategies. Uniquely focusing on the intersection of digital finance and sustainable growth, this paper fills a critical gap in the existing literature. To examine the impact of digital inclusive financing on carbon emissions from the vantage point of enhancing consumption structures, this study uses panel data covering the years 2014–2020 from 30 Chinese provinces. This research underscores the novel finding that digital inclusive finance can play a significant role in lowering carbon emissions by encouraging individuals to alter their consumption patterns. Variations across regions are evident in the impact, with the central and western areas experiencing a more pronounced decline in carbon emissions in comparison to the eastern region. Moreover, the influence of consumption structure upgrading varies across regions. Additionally, the study identifies a positive spatial spillover relationship between digital inclusive finance and carbon emissions. Through its pioneering exploration, this study offers fresh insights for boosting digital finance’s carbon-reduction impacts through consumption structure upgrades, strengthening the spatial interconnectedness of digital finance and consumption trends, and eventually supporting a path toward low-carbon, high-quality development.
1 Introduction
The transition to low-carbon and emission-reduction is a crucial strategic path for China’s future growth, and green and low-carbon development has emerged as a major strategic direction for China’s future development. In the context of industrial development and innovation, the balance between economic progress and environmental conservation has become critical. A recent study has shown an inverted U-shape relationship between economic growth and environmental degradation in 10 emerging countries, emphasizing the role of renewable energy, urbanization, and foreign direct investment in this dynamic (Awan et al., 2022). The Intergovernmental Panel on Climate Change (IPCC) predicts an increase of at least 1.5 °C in the average global surface temperature over the next two decades. Given the significance of this context, our research seeks to elucidate the interrelationship between digital inclusive finance and its ramifications on carbon emissions in China.
The transition to low-carbon and emission-reduction is a crucial strategic path for China’s future growth, and green and low-carbon development has emerged as a major strategic direction for China’s future development. Over the next two decades, the average global surface temperature is projected to increase by at least 1.5°C, according to the Intergovernmental Panel on Climate Change (IPCC). Even while other greenhouse gases and pollutants contribute to global warming, carbon dioxide is still the most important factor (Zhong et al., 2022). While previous studies have touched upon these subjects in isolation, our work’s novelty stems from intertwining the dynamics of digital inclusive finance, consumption structures, and their collective influence on carbon emissions.
In 2022, China’s carbon dioxide emissions are projected to be 11.477 billion tons, according to data from the International Energy Agency. This massive output accounts for about 31.19 percent of total global emissions, firmly establishing China as the world’s largest emitter of carbon dioxide (Cai and Song, 2022). The need to limit carbon emissions is now widely acknowledged by governments worldwide. The “30–60”dual-carbon targets were proposed by General Secretary Xi Jinping at his address to the 75th United Nations General Assembly in September of 2020. China aims to peak its carbon dioxide emissions by 2030 and achieve carbon neutrality by 2060. The State Council issued “Guiding Opinions on Accelerating the Establishment of a Green, Low-Carbon, and Circular Development Economic System” in 2021. This document underscores the importance of balancing economic growth with the effective management of greenhouse gas emissions. It highlights the imperative of steering economic and social development towards greener practices. Consequently, curbing the rise in carbon emissions has emerged as a pivotal concern within China’s pursuit of high-quality economic advancement.
As digital technology has become increasingly pervasive in the financial sector, digital inclusive finance (DIF) has emerged as a key driver of innovation in the sector. By 2021, the median value of China’s digital inclusive finance index across provinces is expected to be 363.61, a huge increase from 2011’s 33.58. In contrast to conventional finance, digital inclusive finance does away with the restrictions brought on by information asymmetry. It extends the reach of conventional banking by catering to the financing requirements of small and medium-sized businesses, an audience that conventional banks have historically struggled to reach (Sun et al., 2021). It plays a pivotal role in accelerating the green transformation of the economy and reducing carbon emissions, while also vastly improving the efficiency with which financial resources are allocated. As a result, does digital inclusive finance reduce carbon emissions? If this phenomenon is proven, what processes are at work? How do the unique characteristics and regional distributions of digital inclusive financing affect its carbon footprint? To address these inquiries, it becomes imperative to delve into the context of China’s modernization and undertake empirical investigations grounded in a comprehensive survey of pertinent literature. This approach not only allows for a thorough analysis but also opens avenues for incremental contributions within this paper.
Theoretical studies conducted in the present day suggest that the impact of the evolution of digital inclusive finance on reducing carbon emissions is complex. At the micro level, digital inclusive finance can affect carbon emissions through enterprise technological innovation (Zhang and Liu, 2022; Ma et al., 2022; Chu et al., 2023) and energy consumption reduction (Dong F. et al., 2022). Furthermore, through our comprehensive investigation, we aim to bridge the existing gap in understanding the potential spatial spillover effects, thereby enriching the discourse on the broader environmental implications of financial innovations.
At the meso level, through industrial structure adjustment (Wang Y. et al., 2022), industrial digital empowerment (Zhang L. et al., 2022), and regional innovation level (Zhang W. et al., 2022), Digital inclusive finance has the potential to improve energy resource accessibility while also increasing carbon emission efficiency, resulting in a reduction in the region’s overall carbon footprint. At the macro level, digital inclusive finance can enhance regional carbon emission reduction capacity through rural revitalization (Xue et al., 2022)], economic growth effects (Li et al., 2020), and population structure adjustment (Dong J. et al., 2022). There is a lack of in-depth theoretical analysis and empirical findings concerning the effects of digital inclusive finance on carbon emissions through the lens of improving the consumption structure, despite the fact that the prior research provides a valuable theoretical foundation and methodological perspectives.
Consumption, as one of the “Three Engines,” has progressively transformed into a driving force propelling China’s economic growth (Li et al., 2020), but it has also contributed to carbon emissions (Li et al., 2021). According to data from the Ministry of Ecology and Environment’s Publicity and Education Center in 2020, home consumption accounts for around 47.5 percent of all carbon emissions in China. Globally, household consumption accounts for as high as 72% of total carbon emissions. This underscores the substantial intervention possibilities within the consumption domain, with a pivotal role assigned to fostering environmentally-friendly shifts on the consumption front. Hence, Theoretically, Through the viewpoint of improving consumption patterns, we must learn how digitally inclusive finance affects carbon emissions. This approach aids in evaluating the societal and economic value engendered by digital inclusive finance’s advancement and enhancing comprehension of factors shaping carbon emission mitigation. Furthermore, unraveling the driving mechanisms underlying digital inclusive finance’s impact on carbon emissions carries tangible practical value. It aids in curbing carbon emissions from the consumption angle, facilitating ecological transitions in the economy, and fostering the growth of a low-carbon economic model.
As laws, technologies, and knowledge are shared, there is also a spillover effect of carbon emissions from one province to another (Yi et al., 2022). According to the theory of agglomeration economies, the spatial concentration of resources allows the reduction of transaction costs and increases the efficiency of resource usage. As a result, this arrangement leads to lower carbon emissions. Some scholars have engaged in both theoretical analysis and empirical research concerning this subject, such as Zhang and Liu (Zhang L. et al., 2022), and Yu and Zhu (Yu and Zhu, 2023), These professionals have all said on multiple occasions that the development of digital inclusive finance has a major adverse effect on local and regional carbon emissions. This observation underscores the existence of positive spatial spillover effects. However, there are also a few scholars who hold opposing views (Chen et al., 2023), suggesting that digital inclusive finance inhibits carbon emissions efficiency in neighboring regions. It is clear that a consensus on the spatial implications of digital inclusive financing on carbon emissions has not yet emerged. The interconnectivity of carbon emissions across areas will undoubtedly impact each province’s development of specialized emission reduction programs. As a result, investigating the impact of digital inclusive finance on carbon emissions within China’s provincial domains from a spatial standpoint becomes critical.
Unlike previous research, this work has the potential to make the following contributions: 1) It offers a unique approach by looking at the relationship between digital inclusive finance and carbon emissions reduction via the lens of consumption structure upgrading. While prior studies have separately delved into the relationships among digital inclusive finance, consumption structure improvement, and carbon emissions reduction, this paper uniquely amalgamates all three elements within a unified framework. 2) By employing a spatial Durbin model, this research uncovers a noteworthy pattern. It demonstrates that the advancement of digital inclusive finance facilitates the reduction of carbon emissions within a province, yet it simultaneously exhibits positive spillover effects. This means that as digital inclusive finance grows within a local area, it might inadvertently contribute to increased carbon emissions in neighboring regions. These distinctive contributions distinguish this paper’s significance within the existing scholarly landscape.
The paper’s structure unfolds as follows: In the second section, a theoretical analysis is presented alongside research hypotheses. The third section delineates the empirical design. The fourth section encompasses empirical result analysis, encompassing endogeneity tests, robustness assessments, and heterogeneity analysis. In the fifth section, special attention is given to spatial spillover effects. Ultimately, the paper concludes with recommendations.
2 Theoretical analysis and research hypotheses
2.1 Carbon emission effects of digital inclusive finance
Scholars from both the domestic and international arenas have recently actively participated in extensive theoretical discussions and empirical studies on the effects of digital inclusive finance development on carbon emissions (Ding R. et al., 2022; Wang et al., 2023a). Two main points of view have evolved from this discussion. According to the first point of view, digital inclusive finance has the ability to reduce carbon emissions. Shahbaz et al. and Boutabba suggest that financial development reduces energy-related emissions by facilitating trade openness, thereby achieving a reduction in CO2 emissions (Shahbaz et al., 2013; Boutabba, 2014). Dong et al. argue that digital inclusive finance primarily enhances carbon emissions efficiency by improving regional innovation levels and entrepreneurial activities (Dong. et al., 2022). Zhang et al. also support this viewpoint (Zhang W. et al., 2022).
The other perspective contends that digital inclusive finance inhibits carbon emissions reduction. For instance, Sadorsky highlights from a consumer perspective that financial development increases energy demand by boosting consumer credit demand, leading to higher carbon emissions (Pu and Fei, 2022). Perry Sadorsky reach similar conclusions (Sadorsky, 2010). Furthermore, Muhammad and Fatima and Sun and Chen find that bank-dominated financial institutions significantly increase carbon emissions and even impede technological progress in enterprises to a certain extent (Javid and Sharif, 2016; Sun and Chen, 2023). Within this study, we undertake an analysis of the influence of digital inclusive finance on carbon emissions, considering the following three distinct levels.
On the one hand, digital inclusive finance is based on digital platforms that digitize offline enterprises. According to transaction cost theory, online financial services significantly reduce high transaction costs and resource consumption resulting from transaction uncertainty and high transaction frequency (Yang et al., 2022), thereby promoting carbon emissions reduction. On the other hand, environmental service platforms in digital finance, such as “Ant Forest,” have increased public engagement in environmental activities, motivating individuals to adopt low-carbon lifestyles (Sun et al., 2021).
From the perspective of businesses, digital inclusive finance effectively reduces search costs and the number of interactions with financial institutions by conveying information to enterprises in need of loans through digital platforms. As a result, it lowers financing costs for companies and alleviates unnecessary resource consumption (Li et al., 2023). Furthermore, digital inclusive finance harnesses advanced digital technologies like big data and blockchain to delve into company-specific information, thereby amplifying information transparency (Li J. and Wang Q., 2022; Li Z. and Wang J., 2022; Fan and Feng, 2022). This reduces financing failures caused by information asymmetry, ultimately achieving emission reduction goals.
From the industrial level, first, as an emerging service sector, digital inclusive finance possesses attributes of minimal energy consumption, reduced pollution, and heightened efficiency. Consequently, its progression proves more favorable for advancing the reduction of carbon emissions in comparison to conventional industries (Qin et al., 2022; Wang et al., 2023b). Second, Through the availability of alternative funding routes, digital inclusive finance broadens the purview of financial services, shattering the financing barriers encountered by small and medium-sized firms. This facilitates optimal resource allocation, guides funds towards high-efficiency industries, promotes industrial upgrading, and supports green transformation (Zhang et al., 2023).
Based on the above analysis, we propose Hypothesis 1.
H1. The advancement of digital inclusive finance contributes greatly to the reduction of carbon emissions.
2.2 Mediating effect of consumption structure upgrading on carbon emission reduction through digital inclusive finance
Digital inclusive finance facilitates major consumption structure upgrading (Yu et al., 2022; Yan et al., 2023). Considering the “price effect,” digital inclusive finance’s impact is two-fold. Firstly, it mitigates financial limitations for businesses, facilitating access to funds for technological advancements and heightened production efficiency. This generates a consistent demand that leads to a decline in market equilibrium prices. Secondly, digital inclusive finance diminishes information disparities and trims consumer search expenses, further influencing a reduction in market equilibrium prices. This tandem effect fosters an increase in non-material consumption, thereby propelling the upgrading of consumption structure (Tian, 2022).
From the standpoint of the “income effect,” digital inclusive finance broadens the channels via which financial services are available and increases people’ potential for income growth. According to the theory of the consumption function, consumption is dependent on income. When the basic material needs of the public are met, they shift towards non-material consumption, achieving consumption structure upgrading (Yu et al., 2022).
Consumption structure upgrading significantly promotes carbon emission reduction (Dong J. et al., 2022; Xing and Ye, 2022; Hu et al., 2023). Upgrading the consumption structure leads to a shift from basic material consumption to non-material consumption, resulting in increased market share for the development and enjoyment-oriented sectors, primarily in the tertiary industry. Moreover, consumption structure upgrading often shapes new consumption concepts and high-quality consumption structure, such as advocating for green transportation and embracing low-carbon consumption (Dong F. et al., 2022). Therefore, consumption structure upgrading has a negative impact on carbon emissions.
Based on this, we propose Hypothesis 2.
H2. The effect of digital inclusive financing on carbon emission reduction is mediated by consumption structure upgrading.
2.3 Spillover effects
New economic geography suggests that everything has a certain spatial connection, and each region’s factors will have spillover effects on neighboring areas. Numerous research have confirmed the spatial consequences of digital inclusive finance in terms of carbon emission reduction (Wang X. et al., 2022; Chang and Li, 2022). Ding contends that digital inclusive finance adeptly transcends geographical constraints, amplifies the scope and depth of economic interactions among regions, and generates adverse spatial spillover consequences on regional economic growth (Ding X. et al., 2022). Similarly, due to the existence of technology spillovers and learning effects, a region can further improve its carbon emission efficiency by learning advanced emission reduction technologies and policies from nearby areas while improving its own carbon emission efficiency (Du et al., 2022). As a result, the impact of digital inclusive finance progress on carbon emission reduction should have similar spillover consequences.
Based on this, we propose Hypothesis 3.
H3. The growth of digital inclusive finance has an impact on carbon emission reduction.
This study combines the “dual-carbon” objectives with relevant theories to investigate the impact of digital inclusive finance on carbon emissions and the low-carbon development trajectory of digital inclusive finance. It investigates the methods through which digital inclusive finance influences changes in carbon emissions, as seen through the lens of improving consumption structure. (refer to Figure 1; Figure 2).
3 Research design
3.1 Model specification
3.1.1 Baseline regression model
To investigate the empirical relationship between digital inclusive finance and carbon emissions, we developed the following baseline regression model:
where
3.1.2 Mediation effect model
To investigate whether consumption structure upgrading acts as an intermediary variable in the relationship between digital inclusive finance and carbon emissions, we developed the following mediation effect model:
where
Eq. 1 represents the mechanism of how digital inclusive finance affects carbon emissions, where
The mediation effects are examined using the following methods: First, the total effect exists in Eq. 1 (i.e.,
3.1.3 Spatial econometric model
Given the volume of evidence available, it is clear that digital inclusive finance, consumption structure upgrades, and carbon emissions can have spatial implications. This study incorporates a spatial econometric model to more completely analyze the geographical spillover effects within these variables. The spatial econometric model that was developed is as follows:
In Eq. 4,
3.2 Variable selection
3.2.1 Dependent variable
In this study, the dependent variable is carbon emissions (lnC). The carbon emissions dataset, which spans each province and year, is obtained from the China Energy Statistical Yearbook. The natural logarithm of carbon emissions is used as the dependent variable to assist the regression analysis. It is important to note that, as of now, authoritative statistical data on carbon emissions in China has not been officially provided. Therefore, many scholars convert energy consumption data into carbon emissions. In this study, the calculation method proposed by Zhang and Zhang (Zhang and Zhang, 2015), as well as Dong et al. (Dong J. et al., 2022), is adopted, which uses the consumption of eight fossil energy sources: raw coal, coke, crude oil, fuel oil, gasoline, kerosene, diesel, and natural gas, to estimate carbon emissions.
Where
3.2.2 Core explanatory variable
The core independent variable under scrutiny in this study is inclusive digital finance (FIN). The degree of digitalization is considered as a proxy for inclusive digital finance. The Inclusive Digital Finance Index, produced by Peking University’s Digital Finance Research Center, emerges as the most comprehensive data source for tracking the advancement of digital finance across China’s provinces. This index factors in both the extensiveness and depth of digital financial services.
3.2.3 Mediating variable
The mediating variable in this study is consumption structure upgrading (CS). Following the method employed by Xing and Ye (Xing and Ye, 2022), this study utilizes the relative proportion of non-material consumption (comprising healthcare, transportation, communication, education, and entertainment) concerning total household consumption as a proxy variable for quantifying consumption structure upgrading.
3.2.4 Control variables
The following variables are included in the control variables: 1) GDP per capita (rgdp). Each province’s economic development level is reflected here. 2) GDP per capita quadratic term (rgdp2). Investigated in order to evaluate the Environmental Kuznets Curve. 3) Business framework (is). The ratio of secondary (including manufacturing and mining) to tertiary industry (services). 4) Technological advancement (lntec). The logarithm of patent applications and grants is included. 5) Structure of energy consumption (es). The fraction of coal usage in relation to overall energy consumption. 6) Level of urbanization (urb). The proportion of the urban population in relation to the overall population. 7) Density of population (lnpop). The logarithm of the ratio of resident population to provincial area is included. 8) Direct foreign investment (lnfdi). The logarithm of the amount of foreign direct investment is used. 9) Environmental laws and regulations (reg). The ratio of investment in industrial pollution control to secondary industry value added is used.
3.3 Data sources
This study gathered panel data from 30 Chinese provinces from 2014 to 2020, excluding Tibet, Hong Kong, Macau, and Taiwan. The China Energy Statistical Yearbook was used to obtain carbon emissions data for each province. The Peking University Inclusive Digital Finance Index was used to calculate inclusive digital finance data for each province. The China Statistical Yearbook was used to obtain consumption structure upgrading statistics for each province. The CSMAR and Wind databases were used to acquire control variable data. Instances of missing data were supplemented using respective provincial statistical yearbooks. Some variables underwent logarithmic transformation to alleviate heteroscedasticity. Table 1 showcases the descriptive statistics.
According to the findings in Table 1, there are considerable disparities in the minimum, standard deviation, and maximum values of carbon emissions (C) across provinces. Indicating distinct regional variations in carbon emissions. The inclusive digital finance coefficient (fin) and the consumption structure upgrading index (cs), as well as control variables such as foreign direct investment (fdi), technological innovation (tec), population density (pop), and urbanization level (urb), exhibit large differences between the maximum and minimum values, indicating imbalances in development among provinces.
4 Empirical analysis
4.1 Total effects of digital inclusive finance on carbon emissions
The overall impacts of digitally inclusive finance on carbon emissions are displayed in Table 2. With the help of the Hausman test, we can be confident that the fixed effects model is the best choice for this type of regression study. Notably, the coefficients in columns 1) and 2) related to digitally inclusive finance have negative signs and achieve statistical significance at the 1% and 5% levels, respectively. This indicates the positive effect that digitally inclusive finance has on decreasing carbon emissions. Hence, the first hypothesis is confirmed.
As digital inclusive finance continues to evolve, it innovates products and services, reduces service costs, and improves service efficiency, thereby influencing various stages of production and consumption and ultimately achieving carbon emission reduction. The median value of China’s digital inclusive finance index rose from 173.82 in 2014 (Sichuan Province) to 334.82 in 2020, an increase of 92.62 percent (Sichuan Province). During this period, China’s unit GDP energy consumption has decreased by about 20%. The average annual energy consumption growth of approximately 2.9% has driven a 6.2% growth in the national economy. This indicates that the advancement of digital inclusive finance contributes to improved energy use efficiency and, as a result, a reduction in carbon emissions.
Contrary to the inverted-U-shaped notion of the environmental Kuznets curve, the coefficients of per capita GDP (pgdp) and the squared term of per capita GDP (rgdp2) have opposing signs for the control variables. This discrepancy may be due to the data period used in this study, which covers the years 2014–2020 and does not include earlier data, thereby influencing the empirical results. Negative effects can be caused by factors such as industrial structure, population density, FDI, and environmental regulation. However, there are beneficial benefits from developments in technology, the structure of energy consumption, and the degree of urbanization.
4.2 The impact of digital inclusive finance on carbon emissions through consumer structure improvement
Through the viewpoint of consumer structure enhancement, Table 3 analyzes the impact of digital inclusive financing on carbon emissions. Data in column 1) indicates a positive and statistically significant (p.01) coefficient related to digital inclusive finance in relation to consumer structure upgrading. That’s why the expansion of consumer structure is boosted considerably by the advent of digitally inclusive finance. In short, as digital inclusive finance develops, a larger share of citizens’ spending goes toward experiences rather than things. Payment options are expanded, credit limits are eased, and non-materialistic consumption is promoted with the help of digitally inclusive finance.
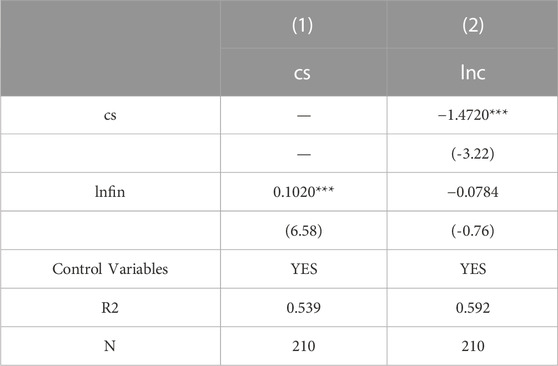
TABLE 3. Effects of digital inclusive finance on carbon emissions through consumer structure upgrading.
Column 2) investigates the mediating influence of consumer structure upgrading. The findings show that consumer structure upgrading is significantly and negatively correlated at the 1% level, indicating that it plays an important role in reducing carbon emissions. Simultaneously, the direct impact of digital inclusive finance on carbon emissions is insignificant, showing that this direct effect has little sway. As a result, consumer structure improvement emerges as a comprehensive intermediary bridging the gap between digital inclusive finance and carbon emissions.
4.3 Endogeneity test
A potential reverse causal relationship between digital inclusive finance and carbon emissions may exist, raising endogeneity concerns. On one hand, the growth of digital inclusive finance could impact consumer structure upgrading, adjustments in industrial composition, and enterprise innovation—factors that could subsequently influence carbon emissions. On the other hand, rising environmental consciousness among the public coupled with government-backed environmental policies might fuel innovation within businesses, producing an “innovation compensation” effect that drives digital inclusive finance development. To enhance result reliability, this study employs instrumental variable (IV) techniques to tackle this intricacy.
There are limited existing studies that discuss instrumental variables related to digital inclusive finance. For example, Fan et al. used internet penetration rate as an instrumental variable (Fan and Feng, 2022), while Yi et al. used mobile phone penetration rate and the number of mobile phone base stations (Yi and Zhou, 2018). In this study, the instrumental variable used for digital inclusive finance is mobile phone penetration rate, considering the following two perspectives. Firstly, digital inclusive finance relies heavily on information technology, and mobile phones serve as a medium through which consumers benefit from digitization. Moreover, if the mobile phone penetration rate is low in a certain province, it hinders the promotion of digital inclusive finance, indicating a strong correlation between the two variables that meets the relevance requirement. Secondly, the influence of mobile phone adoption on digital inclusive finance has no effect on carbon emissions intensity, satisfying the exogeneity criteria.
The outcomes of the two-stage instrumental variable regression are shown in Table 4. According to the results of the preliminary stage of regression, the coefficient associated with the mobile phone penetration rate is 0.0021 (first column). This suggests that an elevated mobile phone penetration rate corresponds to heightened digital inclusive finance development, and this coefficient holds statistical significance. In the subsequent column, the second-stage regression showcases that “phone” and “lnc” are negatively linked, reaching a 5% significance level. This demonstrates the carbon emission reduction effect of digital inclusive financing.
4.4 Robustness test
Robustness tests are integral to gauging the model’s resilience and its capability to offer plausible interpretations of real-world phenomena. A commonly employed approach to assess model stability is to modify key variables. The comprehensive digital inclusive finance index includes three sub-indices that, to some extent, represent the extent of digital inclusive finance development. Nevertheless, when considering the mediating factor of consumption structure upgrading, using the ratio of transportation, healthcare, education, and cultural expenditures in total consumption might not provide an accurate depiction of consumption structure upgrading. Thus, this study undertakes robustness tests by altering the measurement methodologies for both the explanatory and mediating variables.
4.4.1 Changing the explanatory variable
Broadness of coverage, depth of usage, and degree of digitalization are the three components that make up the digital inclusive finance index. Multiple indices—mobile, affordability, credit, and convenience—comprise the degree of digitalization. For the purpose of performing robustness tests, the degree to which inclusive finance has been digitalized has been substituted for the whole digital inclusive finance index. The outcomes are shown in Table 5.
There is a highly significant inverse relationship between the degree of digitalization of inclusive finance and the amount of financial inclusion, as shown by the data in the first column. This suggests an inverse association between digitization and carbon emissions, suggesting that carbon emissions can be lowered with the help of digitally inclusive financing. There is a positive correlation between the degree to which inclusive finance is digitized and the upgrading of the consumption structure, as shown in column (2), at the 5% level of statistical significance. Carbon emissions are greatly reduced when an improved consumption structure is introduced into the model, as indicated in column (3). At the same time, the more the use of digital tools in inclusive finance, the lower the resulting carbon emissions. Thus, the past empirical findings coincide with the mediating effect of upgrading consumption structure in the context of the link between digital inclusive finance and carbon emissions.
4.4.2 Changing the mediating variable
In the previous analysis, the level of consumption structure upgrading was measured using the proportion of non-material consumption, which is the proportion of transportation, healthcare, education, and cultural expenses in total consumption. To assess the robustness of the outcomes, this study employs two alternative methods as substitutes for the proxy variable denoting consumption structure upgrading.
First, referring to Tian for measuring consumption structure upgrading, expenditures are classified into three categories: subsistence consumption, development-oriented consumption, and enjoyment-oriented consumption, based on the classification of household consumption by the National Bureau of Statistics (Tian, 2022). These three categories are given weighted values, resulting in a composite consumption upgrading index. The following is how the consumption structure upgrading index is calculated: Consumption Structure Upgrading Index = 1 Proportion of Subsistence Consumption + 2 Development-Oriented Consumption + 3 Enjoyment-Oriented Consumption.
Second, referring to Liu and Hu (Liu and Hu, 2021), subsistence consumption is defined as expenditures on food, clothing, and housing. The consumption structure is measured by the proportion of total expenditures spent on food, clothes, and shelter (proportion of subsistence consumption). A decrease in this proportion indicates an upgrading of the consumption structure.
To sum up, through the lens of modified measurement methodologies for the mediating variable, it is evident that the impact of digital inclusive finance on carbon emissions maintains a mediating influence by way of consumption structure upgrading. This further bolsters the credibility of the prior empirical findings (Table 6).
4.5 Heterogeneity analysis
Given that China encompasses vast regional disparities in culture, development, geography, and other aspects—owing to its position as the third-largest country by land area—a deeper exploration of the effects and mechanisms of digital inclusive finance on carbon emissions within diverse regions becomes pertinent. To facilitate this analysis, the sampled provinces are categorized into three regions: East, Central, and West, based on the classification criteria of the National Bureau of Statistics. This division allows for distinct empirical investigations for each region. For the sake of comparative analysis, Table 7 integrates the findings for the entire sample, encompassing both the national and Eastern region perspectives, while Table 8 delves into the outcomes for the Central and Western regions.
The East, Central, and Western coefficients all share the national perspective of pessimism on the impact of digital inclusive finance on greenhouse gas emissions. Evidence that digitally inclusive finance can successfully curb greenhouse gas production. Specifically, at the 1 percent level, this effect is highly substantial in the Central and Western areas. When looking at the impact of digital inclusive finance on lowering carbon emissions, it appears that the Central and Western regions benefit more than the rest of the country as a whole. The Eastern region, in comparison, tends to yield insignificant findings. The quick economic growth, early adoption of green economy practices, and aggressive execution of low-carbon regulations that have been seen in the Eastern area may be to blame, since they may mitigate the benefits of digital inclusive finance in terms of lowering emissions and carbon intensity.
Consistent with the national trend, all three areas (the East, the Central, and the West) display positive outcomes when it comes to the impact of digital inclusive finance on upgrading the consumption structure. This suggests that digitally inclusive finance may help optimize the structure of consumer spending. The Central region feels the effects of this more than the Eastern region. The lack of economic and financial development in the Central Provinces has been mitigated, demonstrating the efficacy of digitally inclusive finance. Making available financial services in the Central Region boosts spending patterns that prioritize experiences over things. In the West, where inclusive digital money is still in its infancy, the impact has been minimal.
The overall effect of digital inclusive finance in the Central area is significantly unfavorable in terms of the mediating effect of improving the consumption structure. There is a negative correlation between improved consumption structure and carbon emissions, but digitally inclusive financing does promote consumption structure. The negative impact of digitally inclusive finance on greenhouse gas emissions is minimal. Instead, this effect is totally mediated by consumption structure, which in turn encourages lower carbon emissions. The influence of digital inclusive finance on carbon emissions is considerably negative in the Western region, demonstrating that upgrading consumption structures partially mediates the relationship between digital inclusive finance and carbon emissions.
4.6 Spatial spillover effects
Before estimating the parameters of the spatial econometric model, an apt model form needs to be selected, involving several sequential steps. To initiate, a Hausman test is administered to decide between fixed effects and random effects models. Following this, a LR (likelihood ratio) test is executed to ascertain the precise form of the fixed effects model. The LR test results indicate that the spatial Durbin model with both individual and time fixed effects is the best option. Furthermore, the spatial Durbin fixed effects model is better suitable than the spatial lag and spatial error fixed effects models. In the subsequent step, a Wald test is leveraged to assess the potential transformation of the model into a spatial lag or spatial error model. The Wald test results indicate a substantial dismissal of the null hypothesis for all models. In culmination, based on the course of these sequential steps, It is concluded that the spatial Durbin model with individual and time fixed effects is the best fit for the following investigation.
4.6.1 Results of spatial correlation test
An initial step in determining the presence of spatial correlation is to run a global spatial autocorrelation test using Moran’s I index. This indicator has values ranging from −1 to 1, with values closer to 1 indicating greater geographical connection. The positive or negative sign denotes positive or negative spatial correlation. Table 9 shows the results of the geographical correlation test for carbon emissions. The Moran’s I index, calculated using the economic distance weighting matrix, has a noticeably positive value at the 10% significance level for the period 2014–2020. This identifies the presence of a positive spatial connection in carbon emissions between provinces. Notably, the spatial correlation of digital inclusive finance shows incremental strengthening over time, whereas the spatial correlation of carbon emissions shows minor variations (Figure 3; Figure 4; Figure 5).
4.6.2 The total effect of digital inclusive finance on carbon emissions
Table 10 summarizes the overall impact of digital inclusive financing on carbon emissions. Columns 1 and 2 employ an economic-geographic matrix, whereas columns 3 and 4 employ an adjacency matrix. As demonstrated in Table 10, the coefficient of digital inclusive finance is negative at a 1% significance level when using the economic-geographic matrix and negative at a 5% significance level when using the adjacency matrix. This suggests that the province’s growth of digital inclusive finance contributes to lower carbon emissions. In essence, the progression of digital inclusive finance undeniably exerts a noteworthy restraining influence on carbon emissions, consequently corroborating the hypothesized relationship. This may be due to the fact that the development of digital inclusive finance can provide opportunities for reducing carbon emissions for low-income populations and underdeveloped regions through supporting energy efficiency, renewable energy, carbon reduction projects, and promoting a circular economy. By enacting these strategies, digital inclusive finance assumes a favorable role in curtailing carbon emissions and advancing sustainable development. The utilization of diverse matrices further substantiates the reliability and consistency of the empirical outcomes.
The coefficient associated with the lagging part of digitally inclusive finance is significantly positive at the 1% level when evaluated spatially and when other variables are held constant. Based on these findings, it is clear that digitally inclusive finance has far-reaching, beneficial spatial spillover effects. Geographical spillover effects from digital inclusive finance on carbon emissions can be understood as an uptick in emissions in neighboring provinces as a result of developments in digital inclusive finance within the original province.
Further investigation into the geographical effect mechanism of digital inclusive finance on carbon emissions reveals substantial direct, indirect, and total effects, as shown in Table 11. The direct effect is negative at the 5% significance level, proving that expanding access to digitally-enabled financial services leads to less pollution in the province as a whole. Provincial carbon emissions can be lowered by the introduction of a digitally inclusive funding structure. However, if the coefficient of the indirect effect is positive, then means that digital inclusive finance is having a beneficial spatial spillover effect. Carbon emissions from neighboring provinces rise when digital inclusive finance develops inside a jurisdiction.
Digital inclusive finance, when viewed through the lens of the mediating influence of consumption structure upgrading, has the ability to reduce time expenditures, support the sharing economy paradigm, and promote financial support for green energy consumption. These factors collectively guide the transition from traditional consumption structure towards a more sustainable structure. As consumption structure upgrading transpires within a province, a “siphon effect” ensues, driving consumption transference between regions. Consequently, digital inclusive finance exerts a restraining influence on the spatial spillover of consumption structure upgrading in neighboring provinces. Notably, while consumption structure upgrading contributes to carbon emission reduction, the consequence materializes in elevated carbon emissions in neighboring provinces.
5 Conclusion and recommendations
5.1 Conclusion
Using panel data from Chinese provinces from 2014 to 2020, this study combines fixed effects models and spatial Durbin models to thoroughly investigate the mechanisms and spatial spillover effects of digital inclusive finance on carbon emissions. The following are the conclusions:
Efficacious Carbon Emission Restraint: Digital inclusive finance has a tangible impact on carbon emissions. The advancement of digital inclusive finance coincides to the achievement of China’s twin carbon goals. Even after multiple rounds of thorough robustness testing, this assumption retains its robustness.
Consumption Structure Upgrading Mediation: The increased consumption structure intricately mediates the impact of digital inclusive finance on carbon emissions. Within this framework, digital inclusive finance acts as a catalyst for upgrading consumption structures, so contributing to carbon emission reductions.
Regional Heterogeneity Impact: An examination of regional disparities reveals that the carbon emission reduction impact of digital inclusive finance is significantly greater in the central and western regions when compared to the eastern region. Furthermore, in the context of the interaction between digital inclusive finance and carbon emissions, the mediating effect of consumption structure reveals full mediation in the central region, whereas partial mediation is observed in the western region.
Spatial Dynamics: With a geographical lens, it is clear that while the advancement of digital inclusive finance reduces carbon emissions inside the bounds of the originating province, it also has positive spatial spillover effects. This suggests that progress in digital inclusive finance in one province contributes to increased carbon emissions in other regions.
5.2 Recommendations
Based on the research findings, this study proposes the following policy recommendations.
(1) Local authorities must capitalize on the digital transformation trend, reinforce digital economic infrastructure development, and elevate digital inclusive finance in their regions to foster energy preservation and emissions reduction. With its broad reach embracing micro, small, and medium-sized firms (MSMEs), as well as low-income individuals formerly underserved by traditional financial intermediaries, digital inclusive finance provides services such as small-scale inclusive financing. This effectively mitigates the conventional credit’s risks and expenses, promoting a greener economic landscape. This helps local businesses improve their operational performance and enables individuals to improve their income status, thus reducing carbon emissions.
(2) China should aggressively develop digital inclusive finance to boost consumption levels and optimize consumption structure, ultimately injecting endogenous motivation to reduce carbon emissions. To encourage inhabitants to adopt low-carbon consumption habits, digital inclusive finance can offer services like as small-scale loans, payment settlements, insurance, and so on, leveraging digital financial tools to promote the upgrading and optimization of consumption levels and structure. Additionally, digital inclusive finance can provide advocacy and education on carbon emission reduction to residents through digital technology and information dissemination channels. Through mobile applications, online platforms, and other forms, residents can access information and guidance on energy conservation, carbon reduction techniques, and green lifestyles, motivating them to actively adopt environmentally friendly measures and reduce carbon emissions.
(3) Differentiated policy combinations or supplementary strategies need to be employed to propel both the advancement of digital inclusive finance and the execution of carbon emission management. Given the variance in digital inclusive finance’s growth stage and promotion degree across regions, it is vital to select appropriate policy blends and flexibly calibrate implementation intensity based on local developmental nuances and requisites. Region-specific policies can be shaped in accordance with distinct developmental characteristics and necessities. Factoring in the regional disparities in digital inclusive finance’s progress and promotion level, policy emphasis and focal points can be ascertained in line with particular circumstances.
(4) Achieve spatially balanced growth of inclusive finance. The government should adopt suitable policies and prioritize the development of digital inclusive finance in underdeveloped and disadvantaged areas, as well as provide more support and resources to achieve balanced development. Infrastructure such as digital payment systems and electronic banking outlets should be constructed in less developed areas to improve financial service accessibility and convenience. Additionally, efforts should be made to improve network coverage and communication infrastructure to guarantee that digital financial services are accessible. Promoting green finance and sustainable development, guiding financial institutions and users to choose green financial products, supporting financing for renewable energy and low-carbon projects, reducing reliance on high-carbon industries, and lowering carbon emissions should be prioritized in the development of digital inclusive finance.
6 Limitations and future research
First, while this study delves deeply into the impact of digital inclusive finance on carbon emissions from a consumption structure perspective, it overlooks the direct environmental costs associated with the growth of the digital economy itself. As digital technologies become more ubiquitous, so too does the consumption of energy to power them, especially in data centers and networking infrastructure. Future research should factor in the direct environmental footprint of the digital economy to provide a more holistic perspective on its impact on carbon emissions.
Second, our study’s regional focus, especially on Chinese provinces, might limit its generalizability to other nations or broader contexts. Different nations have unique socio-economic conditions, technological adoption rates, and regulatory environments which can significantly influence the interplay between digital finance, consumption structures, and carbon emissions. It would be intriguing to see a comparative study across various nations, especially juxtaposing developing and developed countries, to understand the broader dynamics of these variables.
Third, our study, while using an extensive dataset from 2014 to 2020, might not account for more recent technological innovations, regulatory changes, or shifts in consumption patterns. The digital landscape evolves rapidly, and what may have been relevant in 2020 could be outdated just a few years later. Moreover, the aftermath of the COVID-19 pandemic, which drastically accelerated digital adoption and altered consumption patterns, could introduce new variables into the equation. Future studies should consider an updated dataset, factor in post-pandemic behaviors, and assess the long-term sustainability and impact of pandemic-induced changes on the relationship between digital finance and carbon emissions.
Data availability statement
The datasets presented in this study can be found in online repositories. The names of the repository/repositories and accession number(s) can be found below: https://www.gtarsc.com/.
Author contributions
JY: Conceptualization, Supervision, Writing–review and editing. WX: Formal Analysis, Methodology, Software, Writing–original draft. LH: Data curation, Writing–original draft.
Funding
The author(s) declare financial support was received for the research, authorship, and/or publication of this article. This research was funded by the Jiangxi Province Social Science Planning Project “Study on the Mechanism and Countermeasures of Digital Economy Development for Carbon Emission Reduction in Jiangxi Province” (grant No. 22YJ27) and the Jiangxi University Humanities and Social Sciences Research Project “Research on the mechanism and path of environmental regulation driving high-quality economic development in Jiangxi” (grant No. JJ19101).
Conflict of interest
The authors declare that the research was conducted in the absence of any commercial or financial relationships that could be construed as a potential conflict of interest.
Publisher’s note
All claims expressed in this article are solely those of the authors and do not necessarily represent those of their affiliated organizations, or those of the publisher, the editors and the reviewers. Any product that may be evaluated in this article, or claim that may be made by its manufacturer, is not guaranteed or endorsed by the publisher.
References
Awan, A., Abbasi, K. R., Rej, S., Bandyopadhyay, A., and Lv, K. (2022). The impact of renewable energy, internet use and foreign direct investment on carbon dioxide emissions: A method of moments quantile analysis. Renew. Energy 189, 454–466. doi:10.1016/j.renene.2022.03.017
Boutabba, M. A. (2014). The impact of financial development, income, energy and trade on carbon emissions: Evidence from the Indian economy. Econ. Model 40, 33–41. doi:10.1016/j.econmod.2014.03.005
Cai, X., and Song, X. (2022). The nexus between digital finance and carbon emissions: Evidence from China. Front. Psychol. 13, 997692. doi:10.3389/fpsyg.2022.997692
Chang, X., and Li, J. (2022). Effects of the digital economy on carbon emissions in China: A spatial Durbin econometric analysis. Sustainability 14, 16624. doi:10.3390/su142416624
Chen, L., Lu, Y., Meng, Y., and Zhao, W. (2023). Research on the nexus between the digital economy and carbon emissions -evidence at China’s province level. J. Clean. Prod. 413, 137484. doi:10.1016/j.jclepro.2023.137484
Chu, H., Yu, H., Chong, Y., and Li, L. (2023). Does the development of digital finance curb carbon emissions? Evidence from county data in China. Environ. Sci. Pollut. Res. 30, 49237–49254. doi:10.1007/s11356-023-25659-5
Ding, R., Shi, F., and Hao, S. (2022a). Digital inclusive finance, environmental regulation, and regional economic growth: An empirical study based on spatial spillover effect and panel threshold effect. Sustainability 14, 4340. doi:10.3390/su14074340
Ding, X., Gao, L., Wang, G., and Nie, Y. (2022b). Can the development of digital financial inclusion curb carbon emissions? Empirical test from spatial perspective. Front. Environ. Sci. 10, 1045878. doi:10.3389/fenvs.2022.1045878
Dong, F., Hu, M., Gao, Y., Liu, Y., Zhu, J., and Pan, Y. (2022a). How does digital economy affect carbon emissions? Evidence from global 60 countries. Sci. Total Environ. 852, 158401. doi:10.1016/j.scitotenv.2022.158401
Dong, J., Zhang, M., and Cheng, G. (2022b). Impacts of upgrading of consumption structure and human capital level on carbon emissions-empirical evidence based on China’s provincial panel data. Sustainability 14, 12373. doi:10.3390/su141912373
Du, Q., Deng, Y., Zhou, J., Wu, J., and Pang, Q. (2022). Spatial spillover effect of carbon emission efficiency in the construction industry of China. Environ. Sci. Pollut. Res. 29, 2466–2479. doi:10.1007/s11356-021-15747-9
Fan, Q., Feng, S., Zhao, Z., Jiang, Y., and Lu, Y. (2022). Protective effect of Glutaredoxin 1 against oxidative stress in lens epithelial cells of age-related nuclear cataracts. China Popul. Resour. Environ. 32, 70–82.
Hu, D., Zhai, C., and Zhao, S. (2023). Does digital finance promote household consumption upgrading? An analysis based on data from the China family panel studies. Econ. Model. 125, 106377. doi:10.1016/j.econmod.2023.106377
Javid, M., and Sharif, F. (2016). Environmental Kuznets curve and financial development in Pakistan. Renew. Sust. Energy Rev. 54, 406–414. doi:10.1016/j.rser.2015.10.019
Li, J., and Wang, Q. (2022a). Impact of the digital economy on the carbon emissions of China’s logistics industry. Sustainability 14, 8641. doi:10.3390/su14148641
Li, J., Wu, Y., and Xiao, J. J. (2020). The impact of digital finance on household consumption: Evidence from China. Econ. Model. 86, 317–326. doi:10.1016/j.econmod.2019.09.027
Li, Y., Yang, X., Ran, Q., Wu, H., Irfan, M., and Ahmad, M. (2021). Energy structure, digital economy, and carbon emissions: Evidence from China. Environ. Sci. Pollut. Res. 28, 64606–64629. doi:10.1007/s11356-021-15304-4
Li, Z., Liu, W., and Wei, X. (2023). Retracted: The impact of digital finance development on carbon dioxide emissions: Evidence from households in China. Technol. Forecast. Soc. Chang. 190, 122364. doi:10.1016/j.techfore.2023.122364
Li, Z., and Wang, J. (2022b). The dynamic impact of digital economy on carbon emission reduction: Evidence city-level empirical data in China. J. Clean. Prod. 351, 131570. doi:10.1016/j.jclepro.2022.131570
Liu, M., and Hu, H. (2021). Carbon emissions, consumption structure upgrading, and high-quality economic development: Empirical evidence from China. J. Asia. Pac. Econ., 1–23. doi:10.1080/13547860.2021.2008099
Ma, Q., Tariq, M., Mahmood, H., and Khan, Z. (2022). The nexus between digital economy and carbon dioxide emissions in China: The moderating role of investments in research and development. Technol. Soc. 68, 101910. doi:10.1016/j.techsoc.2022.101910
Pu, Z., and Fei, J. (2022). The impact of digital finance on residential carbon emissions: Evidence from China. Struct. Change Econ. Dyn. 63, 515–527. doi:10.1016/j.strueco.2022.07.006
Qin, X., Wu, H., and Li, R. (2022). Digital finance and household carbon emissions in China. China Econ. Rev. 76, 101872. doi:10.1016/j.chieco.2022.101872
Sadorsky, P. (2010). The impact of financial development on energy consumption in emerging economies. Energy Policy 38, 2528–2535. doi:10.1016/j.enpol.2009.12.048
Shahbaz, M., Tiwari, A. K., and Nasir, M. (2013). The effects of financial development, economic growth, coal consumption and trade openness on CO2 emissions in South Africa. Energy Policy 61, 1452–1459. doi:10.1016/j.enpol.2013.07.006
Sun, J., and Chen, J. (2023). Digital economy, energy structure transformation, and regional carbon dioxide emissions. Sustainability 15, 8557. doi:10.3390/su15118557
Sun, Y., Zhang, T., Wang, X., Li, D., Wu, Q., He, H., et al. (2021). Taxonomy, virulence determinants and antimicrobial susceptibility of Aeromonas spp. isolated from bacteremia in southeastern China. J. Quant. Tech. Econ. 38, 43–59. doi:10.1186/s13756-021-00911-0
Tian, G. (2022). Influence of digital finance on household leverage ratio from the perspective of consumption effect and income effect. Sustainability 14, 16271. doi:10.3390/su142316271
Wang, C., Qi, F., Liu, P., Ibrahim, H., and Wang, X. (2023b). The effect of the digital economy on carbon emissions: An empirical study in China. Environ. Sci. Pollut. Res. 30, 75454–75468. doi:10.1007/s11356-023-27742-3
Wang, C., Zhang, R., Ibrahim, H., and Liu, P. (2023a). Can the digital economy enable carbon emission reduction: Analysis of mechanisms and China’s experience. Sustainability 15, 10368. doi:10.3390/su151310368
Wang, X., Sun, X., Zhang, H., and Ahmad, M. (2022b). Digital economy and environmental quality: Insights from the spatial Durbin model. Int. J. Environ. Res. Public Health 19, 16094. doi:10.3390/ijerph192316094
Wang, Y., Zhang, Y., and Li, J. (2022a). Digital finance and carbon emissions:an empirical test based on micro data and machine learning model. China Popul. Resour. Environ. 32, 1–11.
Xing, X., and Ye, A. (2022). Consumption upgrading and industrial structural change: A general equilibrium analysis and empirical test with low-carbon green transition constraints. Sustainability 14, 13645. doi:10.3390/su142013645
Xue, Q., Feng, S., Chen, K., and Li, M. (2022). Impact of digital finance on regional carbon emissions: An empirical study of sustainable development in China. Sustainability 14, 8340. doi:10.3390/su14148340
Yan, X., Deng, Y., Peng, L., and Jiang, Z. (2023). Study on the impact of digital economy development on carbon emission intensity of urban agglomerations and its mechanism. Environ. Sci. Pollut. Res. 30, 33142–33159. doi:10.1007/s11356-022-24557-6
Yang, G., Ding, Z., Wu, M., Gao, M., Yue, Z., and Wang, H. (2022). Can digital finance reduce carbon emission intensity? A perspective based on factor allocation distortions: Evidence from Chinese cities. Environ. Sci. Pollut. Res. 30, 38832–38852. doi:10.1007/s11356-022-24748-1
Yi, M., Liu, Y., Sheng, M. S., and Wen, L. (2022). Effects of digital economy on carbon emission reduction: New evidence from China. Energy Policy 171, 113271. doi:10.1016/j.enpol.2022.113271
Yi, X., and Zhou, L. (2018). Does digital financial inclusion significantly influence household consumption? Evidence from household survey data in China. J. Financ. Res., 47–67.
Yu, C., Jia, N., Li, W., and Wu, R. (2022). Digital inclusive finance and rural consumption structure - evidence from peking university digital inclusive financial index and China household finance survey. China Agric. Econ. Rev. 14, 165–183. doi:10.1108/CAER-10-2020-0255
Yu, H., and Zhu, Q. (2023). Impact and mechanism of digital economy on China’s carbon emissions: From the perspective of spatial heterogeneity. Environ. Sci. Pollut. Res. 30, 9642–9657. doi:10.1007/s11356-022-22552-5
Zhang, C., Zhang, Z., and Guo, X. f. (2015). Interaction between the polymorphisms of cyclooxygenase-2-1195G/A,MnSOD9Ala/val genes and the high-fat diets and its correlation with ulcerative colitis. China Popul. Resour. Environ. 25, 37–43. doi:10.3881/j.issn.1000-503X.2015.01.007
Zhang, L., Mu, R., Zhan, Y., Yu, J., Liu, L., Yu, Y., et al. (2022a). Digital economy, energy efficiency, and carbon emissions: Evidence from provincial panel data in China. Sci. Total Environ. 852, 158403. doi:10.1016/j.scitotenv.2022.158403
Zhang, M., and Liu, Y. (2022). Influence of digital finance and green technology innovation on China’s carbon emission efficiency: Empirical analysis based on spatial metrology. Sci. Total Environ. 838, 156463. doi:10.1016/j.scitotenv.2022.156463
Zhang, R., Wu, K., Cao, Y., and Sun, H. (2023). Digital inclusive finance and consumption-based embodied carbon emissions: A dual perspective of consumption and industry upgrading. J. Environ. Manage. 325, 116632. doi:10.1016/j.jenvman.2022.116632
Zhang, W., Liu, X., Wang, D., and Zhou, J. (2022b). Digital economy and carbon emission performance: Evidence at China’s city level. Energy Policy 165, 112927. doi:10.1016/j.enpol.2022.112927
Keywords: digital inclusive finance, consumption structure upgrading, carbon emissions, spatial spillover, panel data analysis
Citation: Ye J, Xu W and Hu L (2023) Digital inclusive finance, consumption structure upgrading and carbon emissions. Front. Environ. Sci. 11:1282784. doi: 10.3389/fenvs.2023.1282784
Received: 24 August 2023; Accepted: 13 September 2023;
Published: 25 September 2023.
Edited by:
Magdalena Ziolo, University of Szczecin, PolandCopyright © 2023 Ye, Xu and Hu. This is an open-access article distributed under the terms of the Creative Commons Attribution License (CC BY). The use, distribution or reproduction in other forums is permitted, provided the original author(s) and the copyright owner(s) are credited and that the original publication in this journal is cited, in accordance with accepted academic practice. No use, distribution or reproduction is permitted which does not comply with these terms.
*Correspondence: Wenhua Xu, MjQ5NkBlY2p0dS5lZHUuY24=