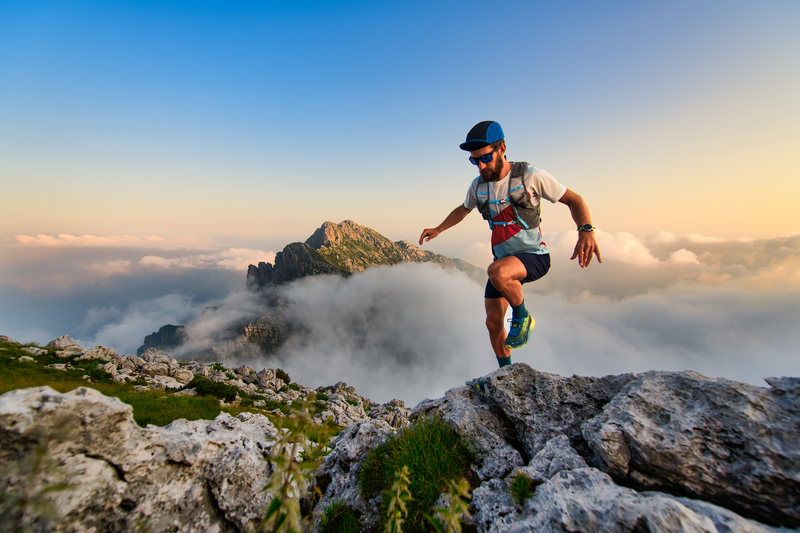
94% of researchers rate our articles as excellent or good
Learn more about the work of our research integrity team to safeguard the quality of each article we publish.
Find out more
EDITORIAL article
Front. Environ. Sci. , 13 September 2023
Sec. Atmosphere and Climate
Volume 11 - 2023 | https://doi.org/10.3389/fenvs.2023.1280733
This article is part of the Research Topic Machine-Learning in Studies of Atmospheric Environment and Climate Change View all 6 articles
Editorial on the Research Topic
Machine learning in studies of atmospheric environment and climate change
Human activities have emitted huge amounts of pollutants into the Earth system since the Industrial Revolution with the usage of fossil fuels (Crippa et al., 2018). Consequently, these emitted air pollutants deteriorate air quality and exert climate consequences (e.g., global warming and related devastating hazards), which poses a threat to human health and ecosystem balance on Earth (Kang et al., 2019). It is very challenging but extremely important to precisely predict air pollution and climate change under different mitigation scenarios, thereby providing scientific evidence for policymakers to make wise decisions and minimize losses due to unavoidable consequences. In recent years, data science has progressed rapidly to develop new understanding from data-driven approaches, and the advance in modern observations (such as satellite remote sensing and in situ observational network) provides large amount of data for the better understanding of atmospheric pollution (Chen et al., 2020) and climate change (Chen et al., 2022). The marriage of these two advances has attracted considerable attention as a powerful tool to tackle complex issues, and more applications have been developed in air pollution prediction and big data analysis in climate science (Jones, 2017). The aim of this Research Topic was to advance our understanding of air pollution and climate change using machine learning methods. Within this Research Topic, five articles with contribution from 32 authors are published. Key messages from each article are highlighted in the following paragraphs.
Ma et al. combined an ensemble machine learning model and satellite observations to generate a pollution distribution map for the electric power system in Yunnan Province, China. They found that this new method and remote sensing data are very helpful in identifying high-pollution areas and therefore could be useful for advising policymakers.
Wang et al. developed a deep learning-based emulator for a sophisticated gas-phase chemical scheme. This emulator is 300–750 times more efficient than the original scheme module. They coupled this emulator with an air quality model and showed a similar accuracy to that of the original module. Their work well demonstrates the high potential of machine learning in advancing process-based models.
Ke et al. developed a data science model to optimize the outcomes of an air quality numerical model. They found that this approach can largely improve model’s capability in predicting highly polluted events in China. Their work demonstrates a promising prospect of machine learning in environment forecast and pollution precautions.
Zhu et al. applied a deep learning approach to calibrating surface air temperature forecast in Xinjiang Province, China. They found that high forecast biases are mainly distributed over mountain regions, and the proposed approach is able to improve the forecast skill.
Yuan et al. used the periodical canonical correlation analysis method to analyse multi-angle simultaneous polarization observations of particulate matter in the atmosphere in Shanghai, China. They found that this advanced data analysis approach is better than traditional methods (such as locally weighted linear regression and autoregressive moving average) in predicting particulate matter concentrations in the atmosphere.
Overall, this Research Topic highlights new prospects that modern machine learning methods bring to us to address challenges in the complex atmospheric and climate system. We hope that more interdisciplinary studies will be carried out at the interface of data science and atmospheric science. Finally, we would like to thank all the authors and the reviewers for their contributions.
YC: conceptualization, funding acquisition, investigation, validation, visualization, writing—original draft, and writing—review and editing. WX: validation and writing—review and editing. JH: writing—review and editing. YW: validation and writing—review and editing.
The authors declare that the research was conducted in the absence of any commercial or financial relationships that could be construed as a potential conflict of interest.
All claims expressed in this article are solely those of the authors and do not necessarily represent those of their affiliated organizations, or those of the publisher, the editors, and the reviewers. Any product that may be evaluated in this article, or claim that may be made by its manufacturer, is not guaranteed or endorsed by the publisher.
Chen, Y., Haywood, J., Wang, Y., Malavelle, F., Jordan, G., Partridge, D., et al. (2022). Machine learning reveals climate forcing from aerosols is dominated by increased cloud cover. Nat. Geosci. 15, 609–614. doi:10.1038/s41561-022-00991-6
Chen, Y., Wild, O., Ryan, E., Sahu, S. K., Lowe, D., Archer-Nicholls, S., et al. (2020). Mitigation of PM<sub>2.5</sub> and ozone pollution in Delhi: a sensitivity study during the pre-monsoon period. Atmos. Chem. Phys. 20, 499–514. doi:10.5194/acp-20-499-2020
Crippa, M., Guizzardi, D., Muntean, M., Schaaf, E., Dentener, F., van Aardenne, J. A., et al. (2018). Gridded emissions of air pollutants for the period 1970–2012 within EDGAR v4.3.2. Earth Syst. Sci. Data 10, 1987–2013. doi:10.5194/essd-10-1987-2018
Jones, N. (2017). How machine learning could help to improve climate forecasts. Nature 548, 379. doi:10.1038/548379a
Keywords: atmospheric science, climate change, machine learning, data science, air pollution
Citation: Chen Y, Xu W, He J and Wang Y (2023) Editorial: Machine learning in studies of atmospheric environment and climate change. Front. Environ. Sci. 11:1280733. doi: 10.3389/fenvs.2023.1280733
Received: 21 August 2023; Accepted: 31 August 2023;
Published: 13 September 2023.
Edited and reviewed by:
Hong Liao, Nanjing University of Information Science and Technology, ChinaCopyright © 2023 Chen, Xu, He and Wang. This is an open-access article distributed under the terms of the Creative Commons Attribution License (CC BY). The use, distribution or reproduction in other forums is permitted, provided the original author(s) and the copyright owner(s) are credited and that the original publication in this journal is cited, in accordance with accepted academic practice. No use, distribution or reproduction is permitted which does not comply with these terms.
*Correspondence: Ying Chen, eS5jaGVuLjIxQGJoYW0uYWMudWs=
Disclaimer: All claims expressed in this article are solely those of the authors and do not necessarily represent those of their affiliated organizations, or those of the publisher, the editors and the reviewers. Any product that may be evaluated in this article or claim that may be made by its manufacturer is not guaranteed or endorsed by the publisher.
Research integrity at Frontiers
Learn more about the work of our research integrity team to safeguard the quality of each article we publish.