- 1Center for Research on Biodiversity Dynamics and Climate Change, Phenology Lab, Department of Biodiversity, Biosciences Institute, São Paulo State University (UNESP), Rio Claro, Brazil
- 2Instituto Tecnológico Vale, Belém, Brazil
- 3Departamento de Ciências Atmosféricas, Instituto de Astronomia, Geofísica e Ciências Atmosféricas, Universidade de São Paulo, São Paulo, Brazil
- 4Center for Ecosystem Science and Society, School of Informatics, Computing, and Cyber Systems, Northern Arizona University, Flagstaff, AZ, United States
- 5Empresa Brasileira de Pesquisa Agropecuária, Embrapa Semiárido, Petrolina, Brazil
- 6Department of ICT and Natural Sciences, NTNU—Norwegian University of Science and Technology, Ålesund, Norway
- 7Wageningen Data Competence Center, Wageningen University and Research, Wageningen, Netherlands
Introduction: The interplay of water and light, regarded as the main driver of tropical plant dynamics, determines leaf phenology and ecosystem productivity. Leaf phenology has been discussed as a key variable to explain photosynthetic seasonality in evergreen tropical forests, but the question is still open for seasonally tropical ecosystems. In the search for implementing long-term phenology monitoring in the tropics, phenocameras have proven to be an accurate method to estimate vegetative phenology in tropical communities. Here, we investigated the temporal patterns of leaf phenology and their relation to gross primary productivity (GPP) in a comparative study across three contrasting tropical biomes: dry forest (caatinga), woodland savanna (cerrado), and rainforest (Atlantic Forest).
Methods: We monitored leaf phenology (phenocameras) and estimated gross primary productivity (eddy-covariance) continuously over time at three study sites. We investigated the main drivers controlling leaf phenology and tested the performance of abiotic (climate) and biotic (phenology) factors to explain gross primary productivity across sites.
Results: We found that camera-derived indices presented the best relationships with gross primary productivity across all sites. Gross primary productivity seasonality was controlled by a gradient of water vs. light, where caatinga dry forest was water-limited, cerrado vegetation responded to water seasonality and light, and rainforest was mainly controlled by light availability. Vegetation phenology was tightly associated with productivity in the driest ecosystem (caatinga), where productivity was limited to the wet season, and the camera-derived index (Gcc) was the best proxy for gross primary productivity.
Discussion: Leaf phenology increased their relative importance over gross primary productivity seasonality at less seasonal sites (cerrado and rainforest), where multiple leafing strategies influenced carbon exchanges. Our multi-site comparison, along with fine-scale temporal observations of leaf phenology and gross primary productivity patterns, uncovered the relationship between leafing and productivity across tropical ecosystems under distinct water constraints.
1 Introduction
Tropical plant communities are responsible for up to 50% of all terrestrial photosynthesis and dominate precipitation recycling rates, strongly contributing to the regulation of regional and global climate (Field et al., 1998; Malhi, 2010). Leaf phenology is related to plant physiology, temporal variations of biomass, leaf area index (LAI), leaf age, deciduousness, length of the growing season (LOS) and, consequently, it is a key driver of the photosynthetic metabolism and carbon ecosystem exchange (Polgar and Primack, 2011; Restrepo-Coupe et al., 2013; Richardson et al., 2013; Wu et al., 2016). Whilst leaf phenology patterns have recently been discussed as a key variable to explain photosynthetic seasonality for temperate and some tropical plant communities (Migliavacca et al., 2011; Restrepo-Coupe et al., 2013; Toomey et al., 2015; Wu et al., 2016, 2017), the question is still opened for seasonally dry tropical ecosystems.
Leaf exchange patterns define the seasonality of tropical plant communities, which is evident in the proportion of deciduous and evergreen species (Reich, 1995; Morellato et al., 2000; Camargo et al., 2018). Light and water are key factors controlling leaf growth and primary production in the tropics (Wright and van Schaik, 1994; Huete et al., 2006; Vico et al., 2017). Water stress is considered the main limiting of dry season productivity in tropical plant communities, both globally (Guan et al., 2015; Wagner et al., 2016) and across biomes in South America (da Rocha et al., 2009; Restrepo-Coupe et al., 2013; Christoffersen et al., 2014; Vico et al., 2015). Indeed, seasonal water deficit tailored ecosystem boundaries and transition zones between humid and dry lands (e.g.,: Olson et al., 2001), with mean annual precipitation of 2,000 mm yr-1 proposed as a threshold of water-limited ecosystems to others responsive to day length or biotic cues (Guan et al., 2015). However, most studies have focused on evergreen Amazonian tropical forests, where an increase of leaf flush in the driest season drives the vegetation greening (Huete et al., 2006; Lopes et al., 2016; Saleska et al., 2016). The knowledge of seasonally dry tropical ecosystems remains elusive (Mendes et al., 2020; Costa et al., 2022a).
Recent studies show the seasonal dynamics of climate, vegetation indices, evapotranspiration, carbon, and energy fluxes at deciduous ecosystems in Brazil (Campos et al., 2019; Mendes et al., 2020; Costa et al., 2022a; Costa et al., 2022b). Ecosystem gross primary productivity (GPP) from satellite remotely sensed in the Amazon, Cerrado (savanna), Pantanal and Caatinga biomes, was weak related with eddy covariance (EC) GPP observed data, with different behaviour in each biome (Costa et al., 2022a). This study demonstrates the importance of investigating specific local environmental drivers and its influence on the ecosystem response captured by in situ and remote sensors. Studies mostly represent phenology by NDVI and/or EVI from satellite using medium to large pixel size products, and 10–16 days of temporal resolution. Nonetheless, seasonally dry tropical forest in Brazil use to synchronize leaf flushing a few days after the first rainfall events (Alberton et al., 2019). Then, satellite-derived indices do not have the capability to track ecosystem responses so fast. It is important to use remote sensed products that combine spatial and temporal resolution to better represent the ecosystem phenology and productivity.
A comparative perspective of tropical phenology may shed light on mechanisms controlling ecosystem processes and how they respond to distinct seasonal environments. Phenological data acquisition is limited in the tropics, with scarce long-term temporal series and uncertainties regarding what triggers plant life-cycle events (Morellato et al., 2016; Abernethy et al., 2018). The use of repeated photographs taken by digital cameras (phenocameras) is an accurate method to monitor leaf phenology over time simultaneously in different plant communities (Morisette et al., 2009; Richardson et al., 2018; Alberton et al., 2017, 2019; Nezval et al., 2020). The camera-derived Gcc (green) index characterizes changes in canopy leaf phenology and has also been related to ecosystem gross primary productivity (GPP) temporal curves estimated from local eddy flux measurements in temperate forests and grasslands (Migliavacca et al., 2011; Toomey et al., 2015), and the drylands (Yan et al., 2019). The process of senescence, represented by the camera-derived Rcc (red) index, has recently demonstrated good performance in tracking canopy photosynthesis as well, especially for evergreen forests (Liu et al., 2020). Despite the successful application of phenocameras to track leafing seasonality in tropical sites (e.g.,: Alberton et al., 2014, 2019; Nagai et al., 2016; Lopes et al., 2016), further evaluation is needed to ascertain if Gcc is a proxy of ecosystem productivity across tropical plant communities.
Here, we investigated the temporal dynamics of leaf phenology and GPP patterns, and how they affect tropical ecosystem productivity across three tropical sites under contrasting seasonality (Guan et al., 2015, Figure 1): a light-limited evergreen Atlantic Forest; a mesic seasonal woodland cerrado with a marked dry season; and the Caatinga, a xeric vegetation representing a water-limited, seasonally dry tropical forest (Supplementary Figure S1) (Morellato et al., 2000; Olson et al., 2001; Camargo et al., 2018; Alberton et al., 2019). We simultaneously monitored the top-canopy phenology using camera-derived repeated digital images, extracting the vegetation indices (Gcc and Rcc). Cameras were at the top of eddy covariance (EC) towers, providing meteorological variables and EC-derived estimates of GPP. Specifically, we asked: i) What are the main abiotic factors related to leafing community responses at each vegetation site? We expect to observe a water-light interplay along the vegetation responses where highly seasonal sites would be more constrained by water limitation towards an increase light-control on leafing patterns at less seasonal environments. ii) What is the relative importance between biotic (leaf phenology) and abiotic (climate) factors controlling ecosystem productivity? We expect that GPP would be explained by the biological process of leaf flushing and senescence, partly in combination with a light and temperature response in the rainforest and partly with an increasing water-limitation response in the cerrado and caatinga; and iii) What are the best proxies of GPP seasonality across the three tropical contrasting biomes? We expect to find more synchronicity between the camera-derived phenology and GPP curves at high seasonal sites since they would be responding to a strong common driver, while towards less seasonal sites curves asynchronies would be expected due to divergent responses between leafing and carbon phenology.
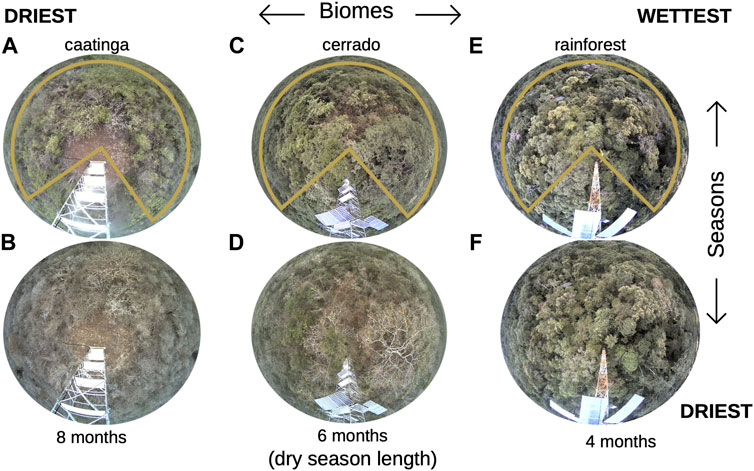
FIGURE 1. Hemispherical images showing the original image captured in the field in each biome type and the region of interest selected to represent a community profile (orange polygons). From left to right, driest, and longer DSL (dry season length) to the wettest and shorter DSL sites; upper and lower images show the vegetation during the wet versus dry season, respectively. (A,B) caatinga; (C,D) cerrado; (E,F) rainforest.
2 Materials and methods
2.1 Sites description
The study sites represent distinct vegetation types of three main tropical biomes (Veloso et al., 1991; Olson et al., 2001): the Caatinga (or the desert and xeric shrubland biome), represented by a seasonally dry tropical forest, the Cerrado (or the grasslands, savannas, and shrublands biome), represented by a woodland cerrado, and the tropical rainforest (or the moist broadleaf forests biome), represented by the Atlantic rainforest (Supplementary Figure S1), hereafter named as caatinga, cerrado, and rainforest, respectively. The sites show contrasting climate regimes reflected in their water availability throughout the year and in the length and intensity of the dry season (Table 1). The research was conducted at all three sites from January 2013 to December 2015.
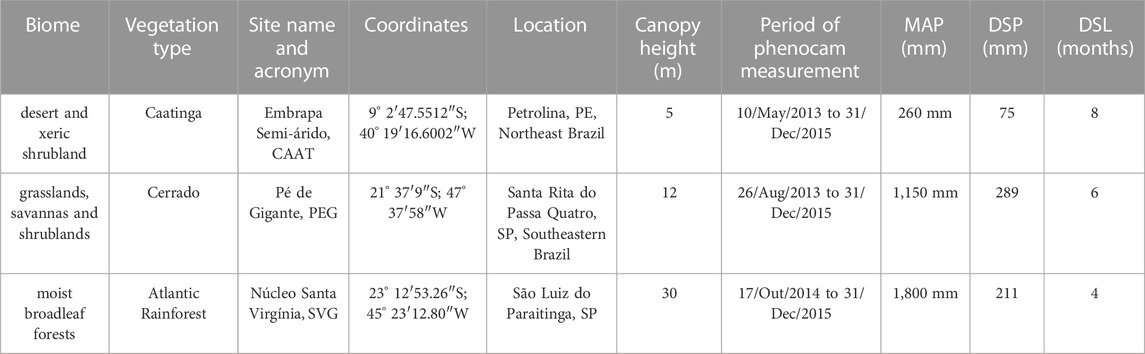
TABLE 1. Site descriptions including: biome (according to Olson et al., 2001), vegetation type, site name and acronym, coordinates, location, canopy height, period of phenocam measurement, mean total annual precipitation (MAP, mm), dry season precipitation (DSP, mm), and length of dry season (DSL) in months. Eddy covariance measurements in all sites from 1 January 2013, to 31 December 2015.
2.1.1 Caatinga site
It is located at the Reserva Legal of Empresa Brasileira de Pesquisa Agropecuária (Embrapa Semiárido), Pernambuco State, Northeast Brazil. It is a typical Brazilian xeric sclerophyllous vegetation from the semi-arid (caatinga) region, in the northeastern region of Brazil, characterized as a seasonally dry tropical forest (Supplementary Figure S1, for more details, see (Alberton et al., 2019). According to 30 years of climatic data (1960–1990) compiled from the WorldClim database v.1.0, the mean temperature is 26°C, with a minimum of 22 °C and a maximum temperature of 32.9°C, and the mean annual precipitation is 566 mm. The dry season extends for 8 months (May to December), and the wet season is usually from January to April, when total monthly precipitation exceeds 100 mm (Supplementary Figure S1). The three-year monthly average of local climatic data (2013–2015) for the caatinga vegetation was hotter and drier, with 260 mm annual total mean precipitation and an annual mean temperature of 27,05 °C (Figure 2A).
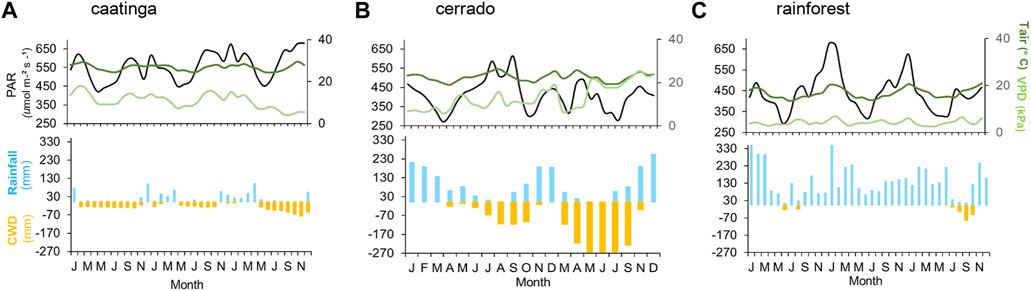
FIGURE 2. Mean monthly climate variables during the interval of January 2013 to December 2015 for (A) caatinga, (B) cerrado, and (C) rainforest sites. Upper panel: photosynthetic active radiation (PAR µmol m−2 s−1), mean air temperature (Tair °C), and vapor pressure deficit (VPD kPa); lower panel: monthly rainfall (Rainfall), monthly evapotranspiration (ET), and cumulative water deficit (CWD) all in mm month-1.
2.1.2 Cerrado site
The cerrado woodland is the dominant physiognomy of the study site located at the Reserva Ecológica Pé-de-Gigante (PEG) at 649 m a.s.l (for more details see Alberton et al., 2019), São Paulo State, southeastern Brazil (Supplementary Figure S1). According to the 30-year climatic data (1960–1990), the mean annual temperature is 21.1°C, with minimum and maximum means of 16 °C and 29.0°C, respectively. The mean total annual precipitation is 1421 mm, with a wet season from October to March (precipitation above 100 mm) and a dry season from April to September (Supplementary Figure S1). During our investigation (2013–2015), the mean annual temperature was 22.5 °C and the average annual precipitation of 1150 mm (Figure 2B). The year 2014 was atypical, with a dry summer: rainfall of 152 mm was registered between January to February, substantially lower than the values registered for the same period in 2013 and 2015 (374 mm and 403 mm, respectively).
2.1.3 Rainforest site
It is located at the Núcleo Santa Virginia (SVG) at 1,056 m a.s.l, within the Parque Estadual da Serra do Mar, São Paulo State, southeastern Brazil (Supplementary Figure S1). The vegetation of the field site is characterized as a cloud forest. The 30-year climatic data (1960–1990) showed a mean annual temperature of 16°C, with a minimum of 12 °C and a maximum of 23.8°C, and a mean total annual precipitation of 1,692 mm (Supplementary Figure S1). The site, despite under the proposed threshold of 2,000 mm of MAP, presents an 8-month wet season that extends from September to April and a small 4-month dry season from May to August, which is drier and colder, but a constant mist occurs daily from 9h00–10h00 in the morning and from 16h00–17h00 in the afternoon. The local climate between 2013 and 2015 showed an expected mean annual temperature of 16.7°C, and a slightly higher total annual precipitation of 1,800 mm; 2014 was the wettest year with 1,965 mm of total precipitation, but the distribution of rainfall was atypical (Figure 2C).
2.2 Measurements of atmospheric fluxes and environmental drivers
Measures with an automatic weather station and atmospheric turbulent fluxes with eddy covariance (EC) method (sensible heat flux, latent heat flux - evapotranspiration E), momentum, and CO2 flux) were taken from January 2013 to December 2015 (Table 1). CO2 and water vapor concentration and the three components of wind speed were measured at high frequency (10 Hz) by an open path infrared gas analyzer (LI-7500, Li-Cor, Lincoln, NE, United States of America) and by a three-dimensional sonic anemometer (CSAT3, Campbell Scientific, Logan, UT, United States of America) at all sites. Means, variances, and covariances at 30 min intervals were computed with the high-frequency raw EC data using EddyPro® software (v.6.2.1) (LI-COR Biosciences, Lincoln, Nebraska, United States of America). The anemometer tilt was compensated by double rotating method (Wilczak et al., 2001); corrections for air density fluctuations were applied according to Webb-Pearman-Leuning (Webb et al., 1980); and the spectral corrections following (Moncrieff et al., 2005).
A quality data check was performed. Spurious values due to sensor failures or field maintenance/occurrences were removed, which includes fluxes values measured during rainfall events. Values outside the physical and/or biologically plausible ranges were also removed from the dataset (Papale et al., 2006) and the friction velocity (u*) threshold varied seasonally for each site (caatinga—0.16 < u* < 0.18 m s-1; cerrado - 0.25 < u* < 0.35 m s-1; rainforest - 0.17 < u* < 0.18 m s-1). It was used the quality control flags (“0” for best quality fluxes, “1” for fluxes suitable, and “2” for fluxes with poor quality) provided by EddyPro software, and the fluxes marked with flag 2 were discarded from the results (Foken et al., 2005). For all these reasons, we found that 35.8%, 14.5%, and 31.6% of the NEE data was unavailable on the data set, removed, and therefore filled, respectively for caatinga, cerrado, and rainforest sites.
The marginal distribution sampling (MDS) method is included in the REddyProc R package (Wutzler et al., 2018) and was applied to fill gaps in the following variables: net ecosystem exchange (NEE), air temperature (Tair), and vapor pressure deficit (VPD). Finally, gross primary productivity (GPP) was estimated by partitioning net ecosystem exchange (NEE, in mol CO2 m−2 s-1) into ecosystem respiration (Reco) and GPP, where GPP = Reco -NEE. Reco was approximated by following the nighttime approach using the Loyd-Taylor function (Reichstein et al., 2005) implemented in the REddyProc Package. Further information can be found at Souza et al. (2015), Carvalho et al. (2018) for caatinga; Rocha et al. (2002), da Rocha et al. (2009) for cerrado, and Freitas (2012) for the rainforest.
The following meteorological variables were monitored using a datalogger and the measured data was averaged by 30-min: photosynthetically active radiation (PAR, LI-190SA, Li-Cor, Nebraska, United States of America); air temperature and relative humidity (HMP45C, Campbell Scientific, Inc., Logan, UT, United States of America), and rainfall (CS700-L Hydrological Services Rain Gauge, Liverpool, Australia). The vapor pressure defict (VPD) was computed by the method described in Allen et al. (1998). To evaluate dry season intensity, we calculated the cumulative water deficit (CWD) based on the cumulative difference of ET-P (Murray-Tortarolo et al., 2016; Alberton et al., 2019). Day length was used to represent the photoperiod seasonality and was calculated based on the latitude of each location (geosphere package in R, (Hijmans et al., 2022). Environmental variables were summarized into weekly values of mean air temperature (Tair), rainfall, photosynthetically active radiation (PAR), vapor pressure deficit (VPD), and photoperiod.
2.3 Temporal patterns of leaf phenology
At each site, a digital hemispherical lens camera Mobotix Q 24 (Mobotix AG—Germany) was set up at the top of the tower using settings as (Alberton et al., 2017, 2019) and at different dates (see Table 1). At the rainforest site, we used the images in the interval October 2014—December 2015 to minimize gaps. We filled sequence gaps (more than 7 days with no images recorded) using the StructTS algorithm with a structure model fitted by maximum likelihood (see Hyndman and Khandakar, 2008) with the package imputeTS in R (R Core Team 2017).
Regions of interest (ROI) were defined for each camera image as described by (Richardson et al., 2009; Alberton et al., 2014). We defined a community-level image ROI from the original image to extract the relative brightness of the green, red, and blue color channels (RGB), and then calculate the vegetation indices of leaf color changes: green-up (Gcc) and green-down (Rcc), which represent flushing and senescence events (Woebbecke et al., 1995; Richardson et al., 2009; Alberton et al., 2017). We calculated the normalized indexes of the green and red color channels according to the formulas:
Gcc and Rcc were calculated separately for each of the five hourly images taken each day. Daily Gcc and Rcc time series were taken as the 90th percentile of all daytime data (adapted from (Sonnentag et al., 2012) and eventually aggregated as a weekly moving average to the analyses.
2.4 Data analysis
To answer questions i) and ii), we started investigating the variables with the highest correlations related to leaf phenology and GPP. A set of climatic variables (rainfall, Tair, CWD, VPD, and photoperiod) were correlated to the temporal patterns of leaf flushing and senescence, represented by the camera-derived indices of Gcc and Rcc, respectively. Equally, the same analysis was conducted with GPP, testing the highest correlated variables using the same set of climatic factors in addition to the phenology variables of Gcc and Rcc. To do so, we applied a canonical correspondence analysis (CCA). Canonical Correspondence Analysis (CCA) is a statistical tool to find linear combinations in a lower-dimensional space that maximizes the correlation between two multivariate datasets (Härdle and Simar 2007). It was used to encounter the relative importance of multivariate features to predict leaf phenology and GPP values, while simultaneously identifying the temporal lag at which maximum correlation occurs.
The vectors projected in the lower-dimensional space are called canonical vectors (CVs, or component scores) and are linear combinations of the original dataset variables and canonical weights (or component loadings):
where a and b are the canonical weight of the original datasets X (length n) and Y (length m), respectively, and k is the covariate number on the lower-dimensional space (k<=the smallest dimensionality of the two datasets). Here Dataset X is either composed solely of climatic variables or climatic and biotic variables, while Dataset Y is composed of the Gcc, Rcc, or GPP time series. Initially, the algorithm finds the canonical weight for k = 1 that maximizes the correlation between CVx1 and CVy1, then the step is repeated to create k CV couples. The weight magnitude indicates the combined strength of the original variables in the pattern captured by the CV. Before the CCA, both datasets were normalized to values between 0 and 1.
Then, to answer question iii), we evaluated GPP best predictors by performing Generalized Additive Mixed Models (GAMM), using as dependent variable Y) the temporal patterns of GPP, and as explanatory variables X) the CVx canonical weights with the highest correlation. We used GAMMs as they can capture nonlinear relationships, handle with time series structural in ecological studies, and have been previously used in phenology analyses (Polansky and Robbins, 2013; Alberton et al., 2019). To find the subset of variables that resulted in the best-performing models (i.e., the one that lowers predictive errors), we applied an automatic term selection (Marra and Wood, 2011; Alberton et al., 2019) to identify terms that are not statistically significant (significance with p values < 0.001) and remove them altogether from the model (Marra and Wood, 2011). The variable selection was carried using the double penalty approach, described in (Marra and Wood, 2011) and available in the mgcv R package as null space penalization (Wood, 2017). The method constructs an additional penalty for each smooth term, penalizing only functions of the null space of the original penalty. If all the smoothing parameters for a term tend to infinity, the term will be selected out of the model. Finally, to understand how each term is interacting with GPP, we plotted the partial fits of each smoother parameter and evaluated F-tests of model outcomes.
3 Results
3.1 Temporal patterns of leaf phenology and GPP
Leaf flushing and senescence patterns were seasonal for all sites regardless of the intensity of the drought period. The flush of new leaves was restricted to the rainy months for the caatinga, while for cerrado and rainforest, leaf onset began earlier, still during the dry season (Figure 3). In general, leaf senescence was higher in the dry season (Figure 3). Similarly, GPP time series were seasonal for all sites. For the caatinga and cerrado, there was a marked increase and peaking within the wet season (Figure 3 a-b). Growing season in the caatinga was restricted to the rainy season, with GPP following an intermittent pattern according to the pulses of precipitation. Cerrado growing season presented a more subtle decay entering the dry season. The rainforest site demonstrated a smoother pattern of increase and decrease of GPP (Figure 3C).
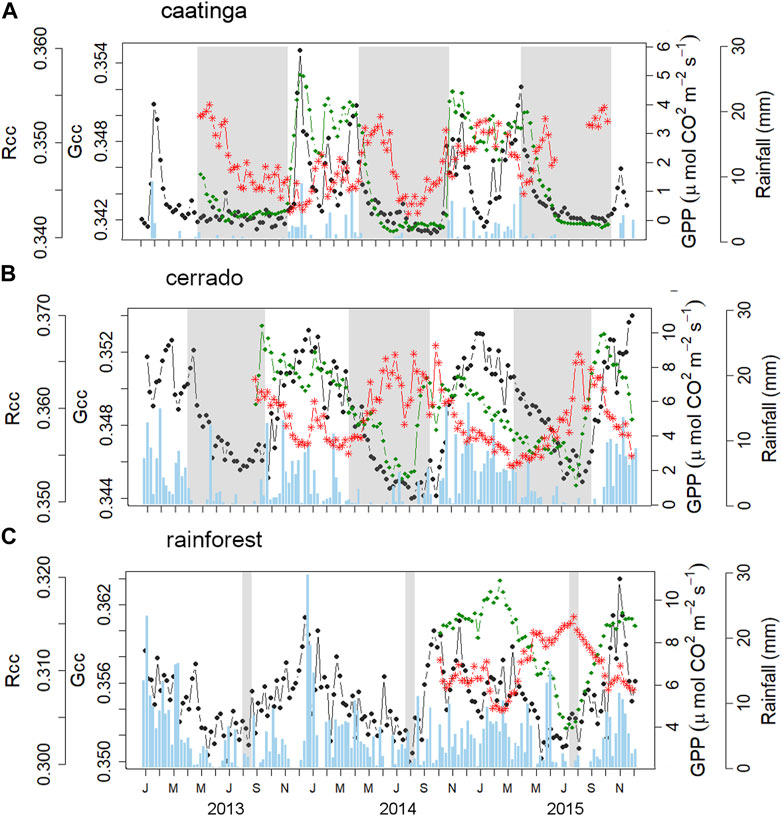
FIGURE 3. Time series (7 days composite) of the camera-derived color indices: Gcc (green dotted line), and Rcc (red dotted line); and EC estimates of gross primary productivity (GPP, black dotted line), for each vegetation type—(A) caatinga; (B) cerrado; and (C) rainforest. Blue bars refer to the weekly cumulative precipitation time series (mm); gray shaded areas indicate the dry season.
Green up for the caatinga was very sharp (within 2–4 weeks), starting at the very early rainy season and quickly declining with suppression of rainfall (Figure 3A). The measures of Gcc were concurrent to GPP year around and especially in the intra-seasonal variability during the growing season. There was a degree of greenness during the caatinga dry season (Figure 3A), related to the Cactaceae Pilosocereus pachycladus F. Ritter (Facheiro). This species is dark green year around and likely one of the species responsible for keeping Gcc steady during the dry season. Rcc peaked at the beginning of the dry season, when the senescence process is intense for the entire plant community, followed by a decrease towards the mid-to-end of the driest months (May to November), when almost all plants are already leafless (Figure 3A). GPP and Gcc curves were highly synchronic with correspondent peaks and troughs, although there were observational dates where Gcc did not follow GPP decreases to zero values.
The cerrado green-up was notably marked at the late dry season, peaking after 4–7 weeks, through the early-middle wet season, that thereafter declined smoothly until the next year’s flushing (Figure 3B). GPP start recovering around the peaking of Gcc, although the GPP peak was 4 weeks later than Gcc peak. Cerrado green-down and Rcc increasing began at the late wet season and extended continuously until the late dry season (August) (Figure 3B), with Rcc and Gcc peaking nearly together in August. Rcc demonstrated an opposite pattern in relation to GPP, while Gcc and GPP curves presented an evident temporal lag at the beginning of the growing season. GPP and Gcc asynchronies were also noticed during the GPP decay at the second growing season cycle (Figure 3B).
The rainforest green-up started during August, at the end of the coolest and driest season, thereafter Gcc increased through the early wet season, peaking at about 4–6 weeks later (Figure 3C). Green-down started in the middle of the wet season and declining smoothly towards the dry season. GPP and Gcc increased and decreased together, although Gcc presented a slighter more seasonal dynamic compared to GPP, which demonstrated a day-to-day oscillation (Figure 3C). The Rcc pattern peaked in August, the driest month when Gcc scores started to increase (Figure 3C).
3.2 Factors controlling leaf phenology and GPP
The relative importance of factors related to leaf phenology and GPP were evaluated based on the canonical loading values (Figure 4; 5, respectively). Regarding leaf phenology, in the Caatinga site, Rainfall and Tair presented the highest loading values related to the leaf flushing index Gcc (Figure 4A), while Rainfall and VPD were related to Rcc (Figure 4B). In the Cerrado, photoperiod was the highest factor, followed by Tair controlling Gcc patterns (Figure 4C). Rainfall exhibited the highest importance related to the cerrado senescence process (Rcc), followed by photoperiod (Figure 4D). In the Rainforest site, photoperiod followed by Tair demonstrated similar relative importance controlling Gcc and Rcc patterns (Figures 4E, F).
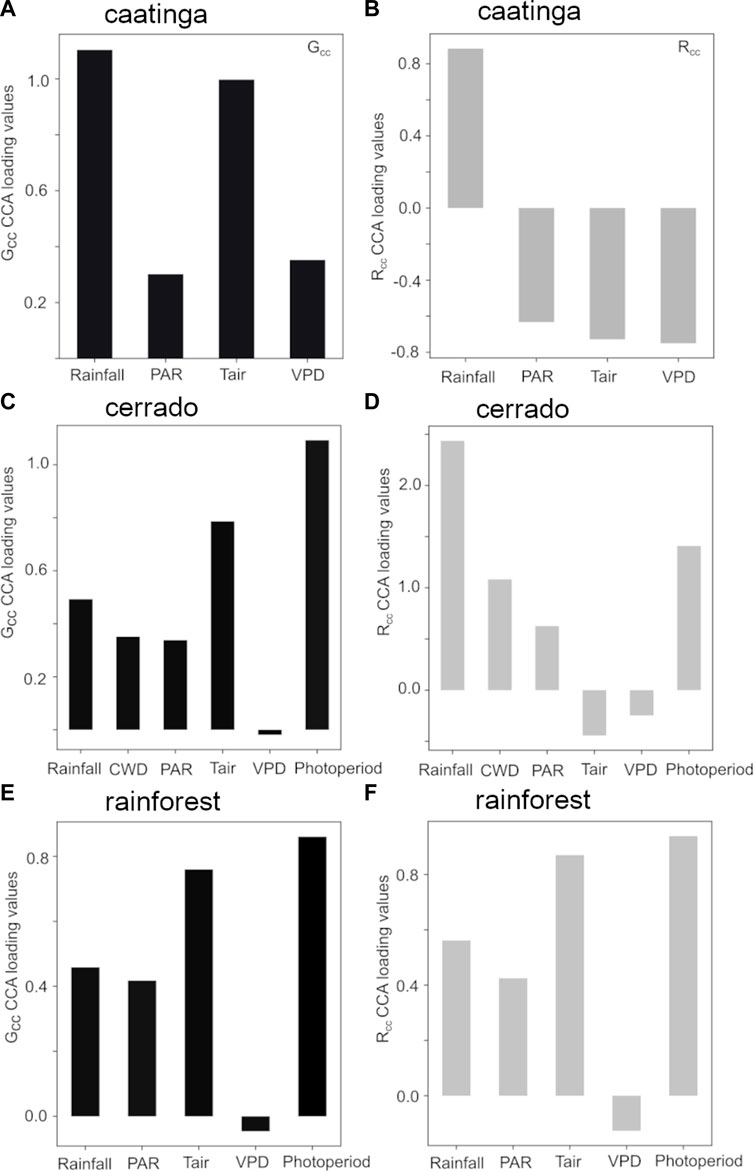
FIGURE 4. Canonical weights (or component loadings) representing the relative importance of each abiotic variable correlated with the camera-derived phenological indices of Gcc (black bars) and Rcc (gray bars) at each study site: (A,B) caatinga; (C,D) cerrado; and (E,F) rainforest.
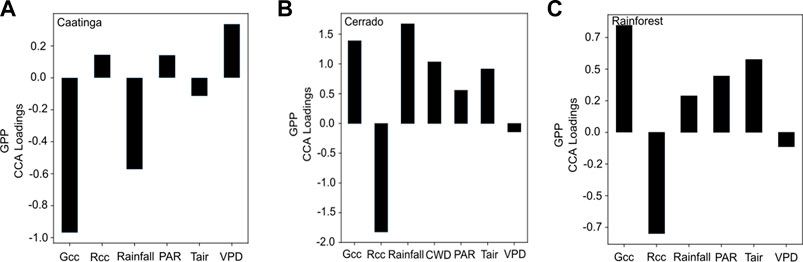
FIGURE 5. Canonical weights (or component loadings) representing the relative importance of each variable correlated with GPP (black bars) at each study site: (A) caatinga; (B) cerrado; and (C) rainforest.
By integrating both climatic and phenological variables, we tested the canonical loadings to evaluate the highest correlated variables with the temporal patterns of GPP (Figure 5). In the caatinga, Gcc index demonstrated the highest correlation with GPP followed by the Rainfall and VPD (Figure 5A). In the Cerrado site, Rcc and Rainfall presented the highest loading values, followed by the Gcc index (Figure 5B). Gcc and Rcc were the main variables correlated to GPP, followed by Tair, in the rainforest site (Figure 5C).
3.3 GPP model predictions
Predictor variables were selected as smooth terms in most of the models. Only Rcc presented linear relationship when modeled against GPP in the Cerrado vegetation site. In general, the GAMMs produced models with medium to high explanation power (R2 from 0.46 to 0.79). Camera-derived phenology (Gcc and Rcc) were recurrent predictors in all sites, although other variables were significant in explaining GPP (Table 2).
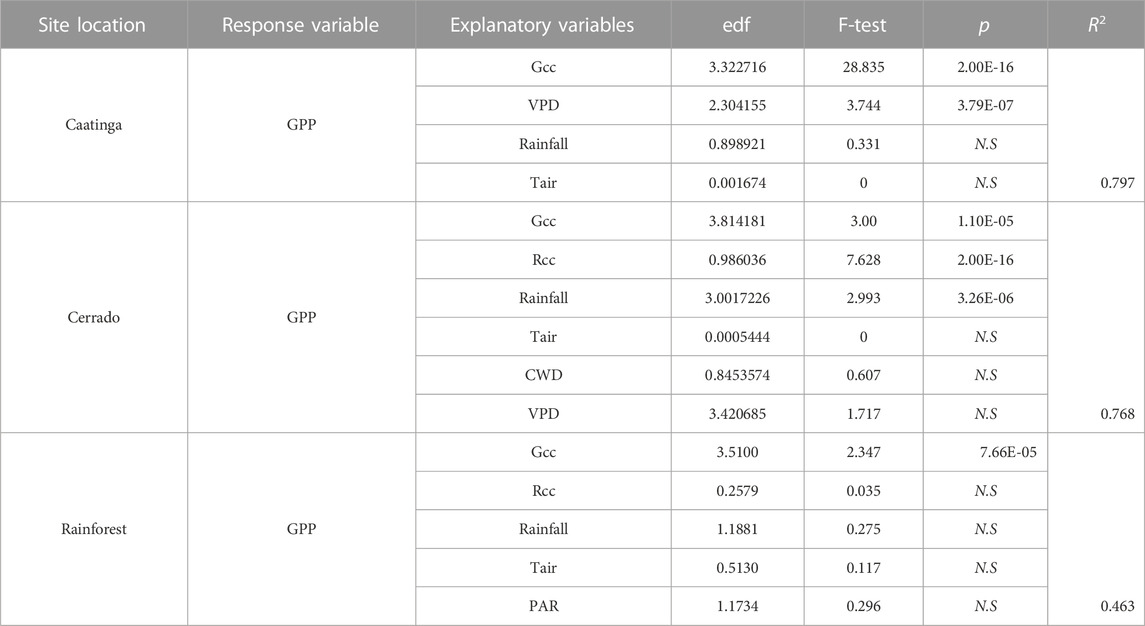
TABLE 2. Approximate significance of the smooth terms used in the GAMMs. Effective degrees of freedom (edf), F-test and p values are given for each of the following variables: green chromatic coordinate (Gcc), red chromatic coordinate (Rcc), Rainfall (mm), vapor pressure deficit (VPD), Air temperature (Tair), and cumulative water deficit (CWD). The coefficient of determination (R2) of each model is also shown. All variables were significant, with p values < 0.001.
For caatinga, Gcc and VPD were the variables that best explained GPP. The partial fit between predictors and the response variable demonstrated that GPP started to be sensitive within an interval of values between 0.345 and 0.350 of Gcc (Figure 6A), while presented a negative relationship with VPD, tending to reach zero values above a VPD of 10 (Figure 6B).
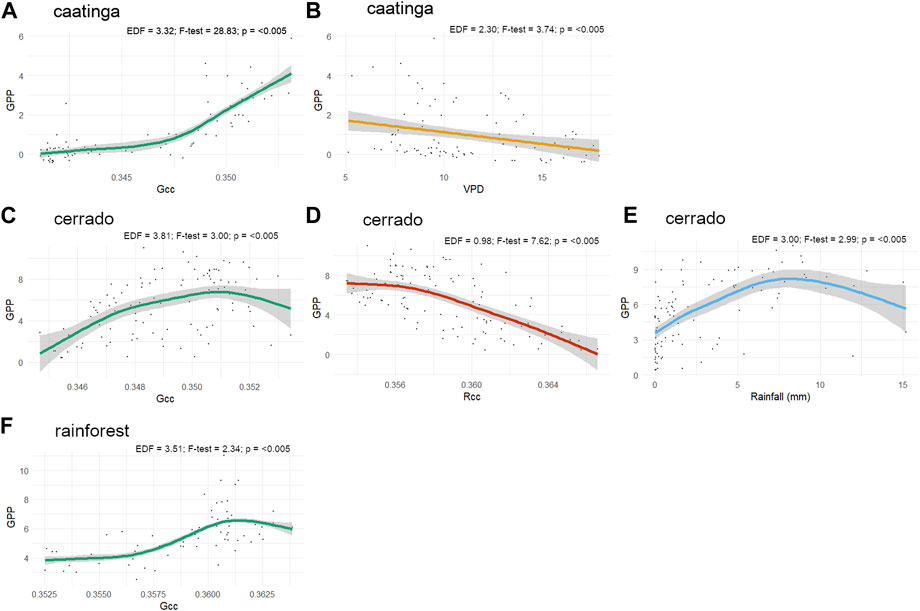
FIGURE 6. Partial fits of the temporal additive mixed models from the best relationships between GPP and biotic and abiotic variables. (A,B) caatinga GPP versus Gcc and VPD, respectively; (C–E) cerrado GPP versus Gcc, Rcc and rainfall, respectively; and (F) rainforest GPP versus Gcc.
In the cerrado site, Rcc index demonstrated to be highly significant in model predictions, with a negative relationship controlling GPP decay and the end of the growing season (Figure 6C). Rainfall and Gcc index were also significant variables showing a similar relationship with GPP increase according to their partial fits (Figure 6D-E). The rainforest GPP estimates were significantly related solely to the Gcc index, demonstrating a logistic function relationship (Figure 6F).
4 Discussion
Our study disentangled the factors driving phenology and GPP across contrasting tropical biomes and the influence of water availability. Leaf flushing drivers differed among biomes from Rainfall and Tair (caatinga), photoperiod and Tair (cerrado) to daylength and Tair (rainforest), reflecting as expected, reduced water constraints affecting leafing temporal patterns towards humid vegetation (Figure 1). On the other hand, leaf senescence drivers tended to be primarily constrained by water availability as demonstrated in the caatinga and cerrado sites. Our analysis partitioned the relative importance of biotic (phenology) and abiotic (environmental) factors, demonstrating that leaf phenology increased its relative importance over GPP towards less seasonal sites. We argue that biotic factors increase control of carbon-water interactions from driest to wettest vegetation sites, although camera-derived time series performed as a reliable proxy of GPP especially in harsh seasonal ecosystems, as the caatinga dry forest, when both leafing and GPP respond quickly to the common driver of rainfall.
4.1 Leaf phenology in tropical ecosystems
The harsh seasonal condition of the caatinga explains the observed dominance of species with a deciduous leaf strategy (Murphy and Lugo, 1986; Machado et al., 1997; Singh and Kushwaha, 2005; Alberton et al., 2019). In fact, rainfall was the main parameter affecting our caatinga Gcc and Rcc predictions. We argue that the high interannual variability of precipitation rates and the long, severe drought, selected species that are drought-avoiders, losing all leaves, and with leafing constrained to the short periods of water availability (Vico et al., 2015; Alberton et al., 2019). In fact, the interannual variation in annual rainfall distribution influenced the length of the growing season and its amplitude in a satellite-derived monitoring in the Caatinga (Medeiros et al., 2022). This scenario also explained the fast response to rainfall, usually within a few days to a couple of weeks after the first rain events, as detectable by the phenocamera daily monitoring (Alberton et al., 2019; Paloschi et al., 2021).
Leaf onset in the cerrado was concentrated in the late-dry season, with old leaves and leafless crowns concentrated in the dry season. Even though the Cerrado is below the proposed annual 2000 mm threshold (Guan et al., 2015), our cerrado woody species produced new leaves still in the dry season, indicating no water-limitation to leaf onset (Rossatto, Hoffmann, and Franco, 2009; Rossatto et al., 2012; Camargo et al., 2018). Cerrado woody plants, like other wood savanna species, present adaptations to maximize their carbon gains, such as water storage and deep root systems that access belowground water sources (Borchert, 1998; Eamus, 1999; Fabian G. Scholz et al., 2002; F. G. Scholz et al., 2008; Rossatto et al., 2012; Camargo et al., 2018), allowing start leaf production yet in the dry season. As suggested by (Dalmolin et al., 2015) leaf emergence associated with branching during the dry season may maximize carbon gain by increasing rates of C assimilation by plants at the onset of the rainy season. For those species flushing at the start of the wet period, water availability may play a key role as a driver of leaf expansion (Lenza and Klink, 2006; Silvério and Lenza, 2010; Camargo et al., 2018; Alberton et al., 2019). Also, cerrado plants responded to a consistent cue, the day length, for the beginning and synchronization of leaf flushing (Camargo et al., 2018). Synchronous, anticipated leaf flushing may also be an adaptation mechanism to avoid herbivory (Aide, 1988; Camargo et al., 2018), a matter that needs further study associating phenology and leaf defense traits (da Silva and Batalha, 2011).
The low range of variation in the rainforest Gcc index suggests the dominant presence of evergreen species. Studies reporting camera-derived phenology from high diverse tropical rainforest have also demonstrated low variation in the Gcc index when considering the whole community ROI (Nagai et al., 2016). Leaf green up and brown down (Gcc and Rcc) were mainly driven by photoperiod in the rainforest plant community, as suggested in a previous phenology study across Atlantic rainforest sites (Morellato et al., 2000) and further demonstrated by Borchert et al. (2005). Older tropical rainforest formations, as the Monsoon forests from South-East Asia, subject to the heterogeneity of climate variability in the rainfall distribution, may demonstrate intriguing patterns of phenological activity (Suepa et al., 2016). Rainforest evergreen species may show asynchronous activity throughout the year, which is likely related to the environmental conditions allowing growth and reproduction year-round (Fenner, 1998; Morellato et al., 2000).
4.2 The role of plant phenology and environment in determining GPP patterns across tropical ecosystems
Our models demonstrated a partitioned effect of biotic (phenology) and abiotic (environment) variables on GPP, with biotic and abiotic covariables contributing to the caatinga and cerrado, and a dominant contribution of the biotic factors in the rainforest. Then, GPP was controlled by a gradient of water vs. light, where caatinga dry forest was water-limited, cerrado responded to water and light seasonality, and less seasonal rainforest was mainly controlled by light availability, corroborating our initial predictions of a hydroclimate control of tropical ecosystem productivity (Guan et al., 2015).
The caatinga showed the strongest, fast-leafing response to the first rains, with GPP peaking early in the wet season with more than 90% decline during the dry season. The caatinga corroborated the prediction that under an annual rainfall threshold of 2,000 mm, seasonal plant communities might not be able to surpass the water stress imposed by the dry season, reducing productivity (Guan et al., 2015; Wagner et al., 2016; Vico et al., 2017). There are still few records of productivity rates from the caatinga derived from MODIS (da Silva et al., 2013; Brito Morais et al., 2017), but recent studies (Mendes et al., 2020; Paloschi et al., 2021) showed similar patterns, characterizing the caatinga fast-leafing response to water pulses.
The highest synchronicity of caatinga trees reinforced the length and intensity of the dry season as a major control of GPP, with precipitation triggering and synchronizing leaf onset, enabling species to recover their water status at the beginning of the wet season (Machado, Barros, and Sampaio, 1997; Alberton et al., 2019), a pattern similar found for other tropical dry forest (Christian et al., 2015). High interannual variability in precipitation rates contributes to the variability and intensity of leaf flushing onset in the community at a given year (Machado, Barros, and Sampaio, 1997; Gutiérrez et al., 2014; Medeiros et al., 2022). Thus, climatic constraints and leaf phenology were tightly coupled in the caatinga, explaining the high correlation between phenology and GPP. The camera-derived Gcc index demonstrated to be a reliable proxy of GPP for harsh seasonal environments, where the highly synchronized leafing responds quickly to rainfall.
In the cerrado, leaf senescence (Rcc) was the best parameter predicting GPP, along with Gcc and precipitation. Based on the rainfall contribution in our models, the senescence process in the cerrado, although not pervasive as in the dominant deciduous caatinga, could be a response to water stress. Our findings agree with the general suggestion that mild seasonal savanna ecosystems would present a complex interaction of climatic and phenological factors (Devi Kanniah, Beringer, and Hutley, 2010; Whitley et al., 2011; Ma et al., 2014; Moore et al., 2017) and that the overall semideciduous vegetation, incorporates complex and multiple leaf-exchange strategies (Rossatto, Hoffmann, and Franco, 2009; Alberton et al., 2014; Camargo et al., 2018). Hence, the strategy of trees to produce new leaves in the dry season is especially important for cerrado carbon-water interactions (Vico et al., 2015) since early leaf flushing may contribute to major carbon gains. Species anticipating leaf emergence, increase carbon assimilation rates when the rainy season begins, thus extending the growing season (Dalmolin et al., 2015). Based on that, different drives may control the onset of leaf flushing and productivity in the cerrado, explaining the observed asynchrony between Gcc and GPP curves.
We suggest that incorporating leaf senescence, rather than only greenness indices, will improve predictions of productivity in the tropics. The effects of foliage senescence on ecosystem processes and their main drivers are still neglected in productivity models (Cueva et al., 2021). However, recent studies are incorporating leaf senescence as a parameter explaining dryland productivity (Cueva et al., 2021) and plant response to climate change in temperate zones (Zani et al., 2020). Based on our visual imagery analysis, the Rcc index is mainly related to leaf senescence and the decrease of green biomass. However, it also may be linked to the many colorful, reddish, new leaves produced at the beginning of the cerrado leaf flushing.
Different from the two seasonally dry sites, as expected, rainforest productivity was the least affected by water throughout the year. Rainforest GPP was mainly controlled by phenology, with the Gcc as the dominant selected variable in the GPP model, despite presenting the lowest explanation power among all sites. The low mean air temperature, although not selected in the GPP model, may be limiting GPP by reducing photosynthetic activity (Vieira et al., 2011; Marchiori et al., 2016; Malhi et al., 2017), most likely due to the elevated altitude of the study site (1056 m a.s.l). Other factors, such as the mechanisms of leaf demography associated with leaf ontogeny are also relevant to control photosynthesis seasonality as suggested for a slightly seasonal evergreen Amazon Forest that is not water-limited (Wu et al., 2016, 2017). Also, Gcc index is sensitive to the onset of new leaves production (Lopes et al., 2016), which may be responsible to cause curves asynchronies between Gcc and GPP towards less seasonal vegetation sites. That is a subject deserving further study combining species synchronicity analysis within and among biomes in a comparative approach.
Our study demonstrated how temporal dynamics of leaf phenology swings according to the dryness intensity imposed in the environment. There was an interplay of water and light triggering plant community responses, indicated by the decrease of water constraining on community leaf flushing and senescence. The multi-site comparison, along with fine-scale temporal observations of leaf phenology and GPP patterns, uncovered the relationship between leafing and productivity across tropical ecosystems under distinct water constraints. Camera-derived indices showed to be reliable in predicting GPP seasonality for high seasonal constraint environments.
Leaf phenology interannual variations were yet detected in our results, but with the use of longer time series, we can further investigate the consequences that extreme climate events may have on ecosystem metabolism. We highlight the likely impacts that future changes on water regimes, mostly related to climate change, may have on plant phenology and productivity of tropical ecosystems (Vico et al., 2015; Morellato et al., 2016; Restrepo-Coupe et al., 2017) especially considering the drying trends presented in the last IPCC report (IPCC, 2021). Integrated methods are expected to significantly improve our understanding of patterns and drivers of tropical phenology and ecosystem metabolism across scales (Miura et al., 2023; Shin et al., 2023; Wang et al., 2023). Cross-scale monitoring phenology, linking phenocameras with high resolution orbital sensors, is promising to effectively track large-scale phenology with a fine individual tree resolution and the response of tropical vegetation to climate change (Wang et al., 2023).
Data availability statement
The raw data supporting the conclusion of this article will be made available by the authors, without undue reservation.
Author contributions
BA, AR, RT, and LM conceived and designed the study; BA processed and analyzed imagery, climatic and fluxes data; TM performed CCA models; BA, MM, HR, and LM analyzed and discussed the results; BA led the manuscript writing with significant input from all authors. All authors contributed to the article and approved the submitted version.
Funding
The present study was supported by the São Paulo Research Foundation-FAPESP (grants FAPESP-Microsoft Research Institute #2010/52113-5, #2013/50155-0, #2021/ 10639-5, and #2019/11835-2 to LM, and grants #2009/54208-6, #2015/50488-5), the National Council for Scientific and Technological Development (CNPq), the Coordination for the Improvement of Higher Education Personnel (CAPES) Finance, and the Fundação de Amparo à Ciência e Tecnologia do Estado de Pernambuco-FACEPE funding to the project Caatinga-FLUX (APQ 0062-1.07/15). BA received a fellowship from FAPESP (#2014/00215-0 and #2016/01413-5), a Pos-doctoral fellowship from CAPES Print Program (#88887.512218/2020-00) at UNESP and from FADESP (#2161/2022) at ITV. LM (#428055/2018-4), RT, and HR receive research productivity fellowships from CNPq. HR acknowledges FAPESP grants #2019/23853-5 and #2021/11762-5.
Acknowledgments
We thank the Instituto Florestal for the permissions to work at the Cerrado and Atlantic rainforest sites, and Embrapa for all the support and collaboration at the Caatinga site.
Conflict of interest
The authors declare that the research was conducted in the absence of any commercial or financial relationships that could be construed as a potential conflict of interest.
Publisher’s note
All claims expressed in this article are solely those of the authors and do not necessarily represent those of their affiliated organizations, or those of the publisher, the editors and the reviewers. Any product that may be evaluated in this article, or claim that may be made by its manufacturer, is not guaranteed or endorsed by the publisher.
Supplementary material
The Supplementary Material for this article can be found online at: https://www.frontiersin.org/articles/10.3389/fenvs.2023.1223219/full#supplementary-material
References
Abernethy, Katharine, Bush, Emma R., Forget, Pierre-Michel, Mendoza, Irene, and Morellato, Leonor Patricia C. (2018). Current issues in tropical phenology: A synthesis. Biotropica 50 (3), 477–482. doi:10.1111/btp.12558
Aide, T. Mitchell (1988). Herbivory as a selective agent on the timing of leaf production in a tropical understory community. Nature 336 (6199), 574–575. doi:10.1038/336574a0
Alberton, Bruna, Almeida, Jurandy, Helm, Raimund, Torres, Ricardo da S., and Menzel, AnnetteLeonor Patricia Cerdeira Morellato (2014). Using phenological cameras to track the green up in a cerrado savanna and its on-the-ground validation. Ecol. Inf. 19, 62–70. January. doi:10.1016/j.ecoinf.2013.12.011
Alberton, Bruna, Torres, Ricardo da S., Cancian, Leonardo F., Borges, Bruno D., Almeida, Jurandy, Mariano, Greice C., et al. (2017). Introducing digital cameras to monitor plant phenology in the tropics: Applications for conservation. Perspect. Ecol. Conservation 15 (2), 82–90. doi:10.1016/j.pecon.2017.06.004
Alberton, Bruna, Torres, Ricardo da Silva, Thiago Sanna Freire Silva, , Humberto, Rda Rocha, MagnaMoura, S. B., and Leonor Patricia Cerdeira Morellato, (2019). Leafing patterns and drivers across seasonally dry tropical communities. Remote Sens. 11 (19), 2267. doi:10.3390/rs11192267
Allen, R., Pereira, L., and Smith, M. (1998). Crop evapotranspiration guidelines for computing crop requirements. FAO irrig. Drain. Report modeling and application. J. Hydrol. 285, 19–40. January.
Borchert, R., Renner, S. S., Calle, Z., Navarrete, D., Tye, A., Gautier, L., et al. (2005). Photoperiodic induction of synchronous flowering near the equator. Nature 433 (7026), 627–629. doi:10.1038/nature03259
Borchert, Rolf (1998). “Responses of tropical trees to rainfall seasonality and its long-term changes,” in Potential impacts of climate change on tropical forest ecosystems. Editor Markham Adam (Dordrecht: Springer Netherlands), 241–253. doi:10.1007/978-94-017-2730-3_10
CamargoGabriela Gutierrez de, Maria, Gustavo Henrique de Carvalho, , de Costa Alberton, Bruna, Reys, Paula, and Leonor Patrícia Cerdeira Morellato, (2018). Leafing patterns and leaf exchange strategies of a cerrado woody community. Biotropica 50 (3), 442–454. doi:10.1111/btp.12552
Campos, Suany, Mendes, Keila R., da Silva, Lindenberg L., Mutti, Pedro R., Medeiros, Salomão S., Amorim, Laerte B., et al. (2019). Closure and partitioning of the energy balance in a preserved area of a Brazilian seasonally dry tropical forest. Agric. For. Meteorology 271, 398–412. June. doi:10.1016/j.agrformet.2019.03.018
Carvalho, H. F. S., Soelma Beserra de Moura, M., and George Freire da Silva, T. (2018). Fluxos de Radiação e Energia em Caatinga Preservada e Cana-de-Açúcar no Semiárido. Rev. Bras. Meteorol. 33, 452–458. September. doi:10.1590/0102-7786333005
Christian, Binal, Joshi, Nikita, Saini, Manjit, Mehta, Nirav, Goroshi, Sheshakumar, Rao Nidamanuri, Rama, et al. (2015). Seasonal variations in phenology and productivity of a tropical dry deciduous forest from MODIS and hyperion. Agric. For. Meteorology 214–215, 91–105. December. doi:10.1016/j.agrformet.2015.08.246
Christoffersen, Bradley O., Natalia Restrepo-Coupe, M. Altaf Arain, Baker, Ian T., Cestaro, Bruno P., Ciais, Phillippe, Fisher, Joshua B., et al. (2014). Mechanisms of water supply and vegetation demand govern the seasonality and magnitude of evapotranspiration in amazonia and cerrado. Agric. For. Meteorology 191, 33–50. June. doi:10.1016/j.agrformet.2014.02.008
Costa, G. B., Mendes, K. R., Branches Viana, L., Almeida, G. V., Rodrigues Mutti, P., et al. (2022a). Seasonal ecosystem productivity in a seasonally dry tropical forest (caatinga) using flux tower measurements and remote sensing data. Remote Sens. 14 (16), 3955. doi:10.3390/rs14163955
Costa, G. B., Mendes, K. R., Branches Viana, L., Almeida, G. V., Rodrigues Mutti, P., Santos e Silva, C. M., et al. (2022b). WUE and CO2 estimations by Eddy covariance and remote sensing in different tropical biomes. Remote Sens. 14 (14), 3241. doi:10.3390/rs14163955
Cueva, Alejandro, Bullock, Stephen H., Méndez-Alonzo, Rodrigo, López-Reyes, Eulogio, and Vargas, Rodrigo (2021). Foliage senescence as a key parameter for modeling gross primary productivity in a mediterranean shrubland. J. Geophys. Res. Biogeosciences 126 (1), e2020JG005839. doi:10.1029/2020JG005839
Dalmolin, Â. C., de Almeida Lobo, F., George, V., Russani Silva, P., José Dalmagro, H., Antunes, M. Z., et al. (2015). Is the dry season an important driver of phenology and growth for two Brazilian savanna tree species with contrasting leaf habits? Plant Ecol. 216 (3), 407–417. doi:10.1007/s11258-014-0445-5
Eamus, Derek (1999). Ecophysiological traits of deciduous and evergreen woody species in the seasonally dry tropics. Trends Ecol. Evol. 14 (1), 11–16. doi:10.1016/S0169-5347(98)01532-8
Fenner, Michael (1998). The phenology of growth and reproduction in plants. Perspect. Plant Ecol. Evol. Syst. 1 (1), 78–91. doi:10.1078/1433-8319-00053
Field, Christopher B., Behrenfeld, Michael J., Randerson, James T., and Paul, Falkowski. (1998). Primary production of the biosphere: Integrating terrestrial and oceanic components. Science 281 (5374), 237–240. doi:10.1126/science.281.5374.237
Foken, Thomas, Göockede, Mathias, Mauder, Matthias, Mahrt, Larry, Amiro, Brian, and Munger, William (2005). “Post-field data quality control,” in Handbook of micrometeorology: A guide for surface flux measurement and analysis. Editors Xuhui Lee, William Massman, and Beverly Law (Dordrecht: Springer Netherlands), 181–208. Atmospheric and Oceanographic Sciences Library. doi:10.1007/1-4020-2265-4_9
Freitas, Helber Custódio de (2012). A influência dos transportes advectivos na estimatitiva do balanço de CO2 do ecossistema: Um estudo de caso para a mata atlântica com uso de técnicas micrometeorológicas. Brazil: Universidade de São Paulo. Text. doi:10.11606/T.91.2012.tde-17042012-145144
Guan, Kaiyu, Pan, Ming, Li, Haibin, Adam, Wolf, Wu, Jin, Medvigy, David, et al. (2015). Photosynthetic seasonality of global tropical forests constrained by hydroclimate. Nat. Geosci. 8 (4), 284–289. doi:10.1038/ngeo2382
Gutiérrez, A. P. A., Engle, N. L., De Nys, E., Molejón, C., and Martins, E. S. (2014). “Drought preparedness in Brazil” weather and climate extremes. High Level Meet. Natl. Drought Policy 3, 95–106. doi:10.1016/j.wace.2013.12.001
Wolfgang Härdle, and Léopold Simar (Editors) (2007). “Canonical correlation analysis,” Applied multivariate statistical analysis (Berlin, Heidelberg: Springer), 321–330. doi:10.1007/978-3-540-72244-1_14
Hijmans, Robert J., and Charles Karney (GeographicLib), (2022). “Spherical trigonometry for geographic applications,” in Geosphere: Spherical trigonometry. Editors Williams, and Chris Vennes. Available at: https://cran.r-project.org/web/packages/geosphere/index.html.
Huete, Alfredo R., Kamel, Didan, Shimabukuro, Yosio E., Ratana, Piyachat, Saleska, Scott R., HutyraWenze Yang, Lucy R., et al. (2006). Amazon rainforests green-up with sunlight in dry season. Geophys. Res. Lett. 33 (6), L06405. doi:10.1029/2005GL025583
Kanniah, Devi, Kasturi, Jason Beringer, and Hutley, Lindsay B. (2010). The comparative role of key environmental factors in determining savanna productivity and carbon fluxes: A review, with special reference to northern Australia. Prog. Phys. Geogr. Earth Environ. 34 (4), 459–490. doi:10.1177/0309133310364933
Lenza, Eddie, and Augusto Klink, Carlos (2006). Comportamento fenológico de espécies lenhosas em um cerrado sentido restrito de Brasília, DF. Braz. J. Bot. 29, 627–638. December. doi:10.1590/S0100-84042006000400013
Liu, Ying, Wu, Chaoyang, Oliver, Sonnentag, Desai, Ankur R., and Wang, Jian (2020). Using the red chromatic coordinate to characterize the phenology of forest canopy photosynthesis. Agric. For. Meteorology 285–286, 107910. May. doi:10.1016/j.agrformet.2020.107910
Lopes, A. P., Nelson, B. W., Wu, J., Graça, P. M. L., Valentim Tavares, J., Prohaska, N., et al. (2016). Leaf flush drives dry season green-up of the central Amazon. Remote Sens. Environ. 182, 90–98. September. doi:10.1016/j.rse.2016.05.009
Ma, Xuanlong, Huete, Alfredo, Yu, Qiang, Restrepo-Coupe, Natalia, Beringer, Jason, Hutley, Lindsay B., et al. (2014). Parameterization of an ecosystem light-use-efficiency model for predicting savanna GPP using MODIS EVI. Remote Sens. Environ. 154, 253–271. November. doi:10.1016/j.rse.2014.08.025
Machado, Isabel C. S., Barros, Luiz M., and EverardoSampaio, V. S. B. (1997). Phenology of caatinga species at Serra talhada, PE, northeastern Brazil. Biotropica 29 (1), 57–68. doi:10.1111/j.1744-7429.1997.tb00006.x
Malhi, Yadvinder (2010). The carbon balance of tropical forest regions, 1990–2005. Curr. Opin. Environ. Sustain. 2 (4), 237–244. doi:10.1016/j.cosust.2010.08.002
Marra, Giampiero, and Wood, Simon N. (2011). Practical variable selection for generalized additive models. Comput. Statistics Data Analysis 55 (7), 2372–2387. doi:10.1016/j.csda.2011.02.004
Medeiros, Rodolpho, Andrade, João, Ramos, Desirée, Moura, Magna, Pérez-Marin, Aldrin, Carlos Dos Santos, , et al. (2022). Remote sensing phenology of the Brazilian caatinga and its environmental drivers. Remote Sens. 14 (11), 2637. doi:10.3390/rs14112637
Mendes, Keila R., Campos, Suany, da Silva, Lindenberg L., Mutti, Pedro R., Ferreira, Rosaria R., Medeiros, Salomão S., et al. (2020). Seasonal variation in net ecosystem CO2 exchange of a Brazilian seasonally dry tropical forest. Sci. Rep. 10 (1), 9454. doi:10.1038/s41598-020-66415-w
Migliavacca, Mirco, Galvagno, Marta, Cremonese, Edoardo, Rossini, Micol, Meroni, Michele, Oliver, Sonnentag, et al. (2011). Using digital repeat photography and eddy covariance data to model grassland phenology and photosynthetic CO2 uptake. Agric. For. Meteorology 151 (10), 1325–1337. doi:10.1016/j.agrformet.2011.05.012
Miura, Tomoaki, Tokumoto, Yuji, Shin, Nagai, Shimizu, Kentaro K., Runi Anak Sylvester Pungga, , and Ichie, Tomoaki (2023). Utility of commercial high-resolution satellite imagery for monitoring general flowering in sarawak, borneo. Ecol. Res. 38 (3), 386–402. doi:10.1111/1440-1703.12382
Moncrieff, John, Clement, Robert, Finnigan, John, and Meyers, Tilden (2005). “Averaging, detrending, and filtering of eddy covariance time series,” in Handbook of micrometeorology: A guide for surface flux measurement and analysis. Editors Xuhui Lee, William Massman, and Beverly Law (Dordrecht: Springer Netherlands), 7–31. Atmospheric and Oceanographic Sciences Library. doi:10.1007/1-4020-2265-4_2
Moore, Caitlin E., Beringer, Jason, Evans, Bradley, Hutley, Lindsay B., and Tapper, Nigel J. (2017). Tree–grass phenology information improves light use efficiency modelling of gross primary productivity for an Australian tropical savanna. Biogeosciences 14 (1), 111–129. doi:10.5194/bg-14-111-2017
Morais, Brito, Cristiano, Ygor, Maria do Socorro Bezerra de Araújo, , Soelma Beserra de Moura, Magna, Josiclêda Domiciano Galvíncio, , and Miranda, Rodrigo de Queiroga (2017). Análise do Sequestro de Carbono em Áreas de Caatinga do Semiárido Pernambucano. Rev. Bras. Meteorol. 32, 585–599. December. doi:10.1590/0102-7786324007
Morellato, Leonor Patrícia Cerdeira, Alberton, Bruna, Alvarado, Swanni T., Borges, Bruno, Buisson, Elise, Camargo, Maria Gabriela G., et al. (2016). Linking plant phenology to conservation biology. Biol. Conserv. 195, 60–72. March. doi:10.1016/j.biocon.2015.12.033
Morellato, L., Patnicia, C., Talora, Daniela C., Takahasi, Adrians, Bencke, Cinara C., Romera, Eliane C., et al. (2000). Phenology of atlantic rain forest trees: A comparative Study1. Biotropica 32 (4), 811–823. doi:10.1111/j.1744-7429.2000.tb00620.x
Morisette, Jeffrey T., Richardson, Andrew D., Knapp, Alan K., JeremyFisher, I., Graham, Eric A., Abatzoglou, John., et al. (2009). Tracking the rhythm of the seasons in the face of global change: Phenological research in the 21st century. Front. Ecol. Environ. 7 (5), 253–260. doi:10.1890/070217
Murphy, P. G., and Lugo, A. E. (1986). Ecology of tropical dry forest. Annu. Rev. Ecol. Syst. 17 (1), 67–88. doi:10.1146/annurev.es.17.110186.000435
Murray-Tortarolo, G., Friedlingstein, P., Sitch, S., Seneviratne, S. I., Fletcher, I., Mueller, B., et al. (2016). The dry season intensity as a key driver of NPP trends. Geophys. Res. Lett. 43 (6), 2632–2639. doi:10.1002/2016GL068240
Nagai, Shin, Ichie, Tomoaki, Yoneyama, Aogu, Kobayashi, Hideki, Inoue, Tomoharu, Ishii, Reiichiro, et al. (2016). Usability of time-lapse digital camera images to detect characteristics of tree phenology in a tropical rainforest. Ecol. Inf. 32, 91–106. March. doi:10.1016/j.ecoinf.2016.01.006
Nagai, Shin, Katsumata, Chifuyu, Miura, Tomoaki, Tsutsumida, Narumasa, Ichie, Tomoaki, Kotani, Ayumi, et al. (2023). Perspective: Improving the accuracy of plant phenology observations and land-cover and land-use detection by optical satellite remote-sensing in the asian tropics. Front. For. Glob. Change 6. doi:10.3389/ffgc.2023.1106723
Olson, David M., Wikramanayake, Eric D., Burgess, Neil D., Powell, George V. N., Underwood, Emma C., D’amico, Jennifer A., et al. (2001). Terrestrial ecoregions of the world: A new map of life on earth. BioScience 51 (11), 933–938. doi:10.1641/0006-3568(2001)051[0933:TEOTWA]2.0.CO;2
Paloschi, Rennan A., Ramos, Desirée Marques, Ventura, Dione J., Souza, Rodolfo, Souza, Eduardo, Leonor Patrícia Cerdeira Morellato, , et al. (2021). Environmental drivers of water use for caatinga woody plant species: Combining remote sensing phenology and sap flow measurements. Remote Sens. 13 (1), 75. doi:10.3390/rs13010075
Papale, D., Reichstein, M., Aubinet, M., Canfora, E., Bernhofer, C., Kutsch, W., et al. (2006). Towards a standardized processing of net ecosystem exchange measured with eddy covariance technique: Algorithms and uncertainty estimation. Biogeosciences 3 (4), 571–583. doi:10.5194/bg-3-571-2006
Polansky, Leo, and Robbins, Martha M. (2013). Generalized additive mixed models for disentangling long-term trends, local anomalies, and seasonality in fruit tree phenology. Ecol. Evol. 3 (9), 3141–3151. doi:10.1002/ece3.707
Polgar, Caroline A., and Primack, Richard B. (2011). Leaf-out phenology of temperate woody plants: From trees to ecosystems. New Phytol. 191 (4), 926–941. doi:10.1111/j.1469-8137.2011.03803.x
Reich, P. B. (1995). Phenology of tropical forests: Patterns, causes, and consequences. Can. J. Bot. 73 (2), 164–174. doi:10.1139/b95-020
Reichstein, Markus, Falge, Eva, Baldocchi, Dennis, Papale, Dario, Aubinet, Marc, Paul, Berbigier, et al. (2005). On the separation of net ecosystem exchange into assimilation and ecosystem respiration: Review and improved algorithm. Glob. Change Biol. 11 (9), 1424–1439. doi:10.1111/j.1365-2486.2005.001002.x
Restrepo-Coupe, N., Rocha, H. R. da, Hutyra, L. R., da Araujo, A. C., Borma, L. S., Bradley, C., et al. (2013). What drives the seasonality of photosynthesis across the Amazon basin? A cross-site analysis of eddy flux tower measurements from the brasil flux network. Agric. For. Meteorology 182, 128–144. December. doi:10.1016/j.agrformet.2013.04.031
Richardson, A. D., Bobby, H., Braswell, D. Y. H., Julian, P. J., and Ollinger, S. V. (2009). Near-surface remote sensing of spatial and temporal variation in canopy phenology. Ecol. Appl. A Publ. Ecol. Soc. Am. 19 (6), 1417–1428. doi:10.1890/08-2022.1
Richardson, Andrew D., Hufkens, Koen, Milliman, Tom, and Steve, Frolking (2018). Intercomparison of phenological transition dates derived from the PhenoCam dataset V1.0 and MODIS satellite remote sensing. Sci. Rep. 8 (1), 5679. doi:10.1038/s41598-018-23804-6
Richardson, A. D., Keenan, T. F., Migliavacca, M., Ryu, Y., Oliver, S., and Toomey, M. (2013). Climate change, phenology, and phenological control of vegetation feedbacks to the climate system. Agric. For. Meteorology 169, 156–173. doi:10.1016/j.agrformet.2012.09.012
Rocha, Humberto R. da, Freitas, Helber C., Rosolem, Rafael, Juárez, Robinson I. N., Tannus, Rafael N., Ligo, Marcos A., et al. (2002). Measurements of CO2 exchange over a woodland savanna (cerrado sensu stricto) in southeast brasil. Biota Neotropica 2, 1–11. doi:10.1590/S1676-06032002000100009
Rocha, Humberto R. da, Manzi, Antonio O., Cabral, Osvaldo M., Miller, Scott D., Goulden, Michael L., Saleska, Scott R., et al. (2009). Patterns of water and heat flux across a biome gradient from tropical forest to savanna in Brazil. J. Geophys. Res. Biogeosciences 114. G1. doi:10.1029/2007JG000640
Rossatto, Davi Rodrigo, Hoffmann, William Arthur, and Franco, Augusto César (2009). Differences in growth patterns between Co-occurring forest and savanna trees affect the forest–savanna boundary. Funct. Ecol. 23 (4), 689–698. doi:10.1111/j.1365-2435.2009.01568.x
Rossatto, Davi Rodrigo, LucasRamos Silva, de Carvalho, Villalobos-Vega, Randoll, Leonel da Silveira Lobo Sternberg, , and Franco, Augusto César (2012). Depth of water uptake in woody plants relates to groundwater level and vegetation structure along a topographic gradient in a neotropical savanna. Environ. Exp. Bot. 77, 259–266. April. doi:10.1016/j.envexpbot.2011.11.025
Saleska, Scott R., Wu, Jin, Guan, Kaiyu, Araujo, Alessandro C., Huete, Alfredo, Nobre, Antonio D., et al. (2016). Dry-season greening of Amazon forests. Nature 531 (7594), E4–E5. doi:10.1038/nature16457
Scholz, Fabian G., Bucci, Sandra J., Goldstein, Guillermo, Meinzer, F. C., and Franco, A. C. (2002). Hydraulic redistribution of soil water by neotropical savanna trees. Tree Physiol. 22, 603–612. doi:10.1093/treephys/22.9.603
Scholz, F. G., Bucci, S. J., Goldstein, G., Moreira, M. Z., Meinzer, F. C., Domec, J.-C., et al. (2008). Biophysical and life-history determinants of hydraulic lift in neotropical savanna trees. Funct. Ecol. 22 (5), 773–786. doi:10.1111/j.1365-2435.2008.01452.x
Silva, Bernardo Barbosa da, Josiclêda Domiciano Galvíncio, , Suzana Maria Gico Lima Montenegro, , Célia Cristina Clemente Machado, , Leidjane Maria Maciel de Oliveira, , and Soelma Beserra de Moura, Magna (2013). Determinação por sensoriamento remoto da produtividade primária bruta do perímetro irrigado São Gonçalo - PB. Rev. Bras. Meteorol. 28, 57–64. March. doi:10.1590/S0102-77862013000100006
Silva, Danilo Muniz da, and Batalha, Marco Antônio (2011). Defense syndromes against herbivory in a cerrado plant community. Plant Ecol. 212 (2), 181–193. doi:10.1007/s11258-010-9813-y
Silvério, Divino Vicente, and Lenza, Eddie (2010). Fenologia de espécies lenhosas em um cerrado típico no Parque Municipal do Bacaba, Nova Xavantina, Mato Grosso, Brasil. Biota Neotropica 10, 205–216. September. doi:10.1590/S1676-06032010000300024
Singh, K. P., and Kushwaha, C. P. (2005). Emerging paradigms of tree phenology in dry tropics. Curr. Sci. 89 (6), 964–975.
Sonnentag, Oliver, Hufkens, Koen, Teshera-Sterne, Cory, Young, Adam M., Friedl, Mark, Braswell, Bobby H., et al. (2012). Digital repeat photography for phenological research in forest ecosystems. Agric. For. Meteorology 152, 159–177. January. doi:10.1016/j.agrformet.2011.09.009
Souza, Luciana Sandra Bastos de, Magna Soelma Beserra de Moura, , Gilberto Chohaku Sediyama, , and George Freire da Silva, Thieres (2015). Balanço de energia e controle biofísico da evapotranspiração na Caatinga em condições de seca intensa. Pesqui. Agropecuária Bras. 50, 627–636. August. doi:10.1590/S0100-204X2015000800001
Suepa, Tanita, Qi, Jiaguo, Lawawirojwong, Siam, and Joseph, P. Messina (2016). Understanding spatio-temporal variation of vegetation phenology and rainfall seasonality in the Monsoon southeast Asia. Environ. Res. 147, 621–629. May. doi:10.1016/j.envres.2016.02.005
Toomey, M., Friedl, M. A., Steve, F., Hufkens, K., Klosterman, S., Oliver, S., et al. (2015). Greenness indices from digital cameras predict the timing and seasonal dynamics of canopy-scale photosynthesis. Ecol. Appl. 25 (1), 99–115. doi:10.1890/14-0005.1
Veloso, H. P., Rangel-Filho, A. L. R., and Lima, J. C. A. (1991). Classificação da vegetação brasileira, adaptada a um sistema universal. Rio de Janeiro, Brazil: IBGE. Available at: http://jbb.ibict.br//handle/1/397.
Vico, G., Dralle, D., Xue, F., Thompson, S., and Manzoni, S. (2017). How competitive is drought deciduousness in tropical forests? A combined eco-hydrological and eco-evolutionary approach. Environ. Res. Lett. 12 (6), 065006. doi:10.1088/1748-9326/aa6f1b
Vico, Giulia, Thompson, Sally E., Manzoni, Stefano, Molini, Annalisa, Albertson, John D., JarcileneAlmeida-Cortez, S., et al. (2015). Climatic, ecophysiological, and phenological controls on plant ecohydrological strategies in seasonally dry ecosystems. Ecohydrology 8 (4), 660–681. doi:10.1002/eco.1533
Wagner, Fabien H., Bruno, Hérault, Bonal, Damien, Stahl, Clément, Anderson, Liana O. Timothy R. Baker, Gabriel Sebastian Becker, , et al. (2016). Climate seasonality limits leaf carbon assimilation and wood productivity in tropical forests. Biogeosciences 13 (8), 2537–2562. doi:10.5194/bg-13-2537-2016
Wang, Jing, Song, Guangqin, Liddell, Michael, Morellato, Patricia, CalvinLee, K. F., Yang, Dedi, et al. (2023). An ecologically-constrained deep learning model for tropical leaf phenology monitoring using PlanetScope satellites. Remote Sens. Environ. 286, 113429. March. doi:10.1016/j.rse.2022.113429
Webb, E. K., Pearman, G. I., and Leuning, R. (1980). Correction of flux measurements for density effects due to heat and water vapour transfer. Q. J. R. Meteorological Soc. 106 (447), 85–100. doi:10.1002/qj.49710644707
Whitley, Rhys J., CatrionaMacinnis-Ng, M. O., Hutley, Lindsay B., Beringer, Jason, Zeppel, Melanie, Williams, Mathew, et al. (2011). Is productivity of mesic savannas light limited or water limited? Results of a simulation study. Glob. Change Biol. 17 (10), 3130–3149. doi:10.1111/j.1365-2486.2011.02425.x
Wilczak, James M., Oncley, Steven P., and Stage, Steven A. (2001). Sonic anemometer tilt correction algorithms. Boundary-Layer Meteorol. 99 (1), 127–150. doi:10.1023/A:1018966204465
Woebbecke, D. M., Meyer, G. E., Von Bargen, K., and Mortensen, D. A. (1995). Color indices for weed identification under various soil, residue, and lighting conditions. Trans. ASABE 38 (1), 259–269. doi:10.13031/2013.27838
Wood, Simon N. (2017). Generalized additive models: An introduction with R. 2. Boca Raton: CRC Press/Taylor and Francis Group. Chapman and Hall/CRC Texts in Statistical Science.
Wright, S. Joseph, and van Schaik, Carel P. (1994). Light and the phenology of tropical trees. Am. Nat. 143 (1), 192–199. doi:10.1086/285600
Wu, J., Albert, L. P., Lopes, A. P., Restrepo-Coupe, N., Hayek, M., Wiedemann, K. T., et al. (2016). Leaf development and demography explain photosynthetic seasonality in Amazon evergreen forests. Science 351 (6276), 972–976. doi:10.1126/science.aad5068
Wu, J., Guan, K., Hayek, M., Restrepo-Coupe, N., Wiedemann, K. T., Xu, X., et al. (2017). Partitioning controls on Amazon forest photosynthesis between environmental and biotic factors at hourly to interannual timescales. Glob. Change Biol. 23 (3), 1240–1257. doi:10.1111/gcb.13509
Wutzler, Thomas, Lucas-Moffat, Antje, Migliavacca, Mirco, Knauer, Jürgen, Sickel, Kerstin, Šigut, Ladislav, et al. (2018). Basic and extensible post-processing of eddy covariance flux data with REddyProc. Biogeosciences 15 (16), 5015–5030. doi:10.5194/bg-15-5015-2018
Yan, D., Scott, R. L., Moore, D. J. P., Biederman, J. A., and Smith, W. K. (2019). Understanding the relationship between vegetation greenness and productivity across dryland ecosystems through the integration of PhenoCam, satellite, and eddy covariance data. Remote Sens. Environ. 223, 50–62. March. doi:10.1016/j.rse.2018.12.029
Keywords: leaf phenology, drivers, productivity, GPP, phenocameras, caatinga, cerrado, atlantic forest
Citation: Alberton B, Martin TCM, Da Rocha HR, Richardson AD, Moura MSB, Torres RS and Morellato LPC (2023) Relationship between tropical leaf phenology and ecosystem productivity using phenocameras. Front. Environ. Sci. 11:1223219. doi: 10.3389/fenvs.2023.1223219
Received: 15 May 2023; Accepted: 21 August 2023;
Published: 14 September 2023.
Edited by:
Monica Rivas Casado, Cranfield University, United KingdomReviewed by:
Nagai Shin, Japan Agency for Marine-Earth Science and Technology, JapanJing Wang, Sun Yet san University, China
Guangqin Song, The University of Hong Kong, Pokfulam, Hong Kong, SAR China in collaboration with reviewer JW
Copyright © 2023 Alberton, Martin, Da Rocha, Richardson, Moura, Torres and Morellato. This is an open-access article distributed under the terms of the Creative Commons Attribution License (CC BY). The use, distribution or reproduction in other forums is permitted, provided the original author(s) and the copyright owner(s) are credited and that the original publication in this journal is cited, in accordance with accepted academic practice. No use, distribution or reproduction is permitted which does not comply with these terms.
*Correspondence: Bruna Alberton, YnJ1LmFsYmVydG9uQGdtYWlsLmNvbQ==