- 1Research Institute on Terrestrial Ecosystems, National Research Council, Porano, Italy
- 2National Biodiversity Future Center, Palermo, Italy
- 3Department of Veterinary Medicine—University of Bari Aldo Moro, Valenzano, Italy
- 4Agenzia Regionale Protezione Ambiente del Lazio, Rome, Italy
- 5Department of Chemistry, University of Rome, La Sapienza, Roma, Italy
- 6Agro-Technology Institute, Peoples Friendship University of Russia, Moscow, Russia
- 7Institute of North Industrial Ecology Problems—Subdivision of the Federal Research Centre, Kola Science Centre of Russian Academy of Science, Apatity, Russia
Introduction: Biogenic fraction of airborne PM10 dominated by bacteria and fungi, has been recognized as serious environmental and human health issues in cities.
Methods: In the present study, we combined a high-throughput amplicon sequencing of the bacterial 16S rRNA gene and the fungal internal transcribed spacer (ITS) region, with elemental analysis of airborne particulate matter (PM10) to investigate the community compositions and structures of PM10-associated bacteria and fungi across four different seasons in three urban sites of Rome with differential pollution rate.
Results: In this study, а clear seasonal shift of bacterial and fungal community structure driven by PM10 mass concentrations and environmental factors, such as temperature and precipitations, has been identified. In addition, the seasonal impact of local sources and long-range transported air masses on the community structures of the microbes has been also postulated. Our data revealed that the lack of precipitation and the subsequent resuspension of dust produced by vehicular traffic might contribute to the maximum abundance of soil-associated microbes in winter and summer. However, the increase of PM10 concentrations favoured also by climatic conditions, domestic heating and dust advection event from African desert further shaped the community structure of winter. Across three seasons, the pollutant removal-hydrogen oxidation bacteria and the opportunist-human pathogenic fungi progressively increased with pollution levels, in the sequence from green to residential and/or polluted area close to the traffic roads, with highest fraction during winter.
Discussion: Hence, our results highlight a close interrelationship between pollution, climatic factors and abundance of certain bacterial and fungal predicted functional groups also with potential implications for human health.
Introduction
Particulate matter (PM) is a common air pollutant, recognized as serious environmental and human health issues in cities and mega cities. PM10 is considered harmful to human health due to small dimensions determining high inhalability and lung penetration capacity. PM10 consists of a complex and heterogeneous mixture of biotic and abiotic particles, variable in time and space. Bacteria and fungi propagules constitute a dominant fraction of the total concentration of PM10 organic matter which includes other viable and not-viable material such as viruses, green algae, pollen, plant debris and microbial fragments (Fröhlich-Nowoisky et al., 2016). Once aerosolized from different equally diverse environments, including soils, aquatic environments, plant communities and animals, airborne microorganisms have a relative long atmospheric persistence due to their small size, ubiquity, and adaptable metabolic pathways (Cao et al., 2014). They can be also transported across continents by air masses prior to both wet and dry deposition (Cáliz et al., 2018; Maki et al., 2019). While their persistence in the atmosphere influences hydrogeological cycle and climate by acting like ice nuclei and promoting cloud formation (Morris et al., 2014), once deposited or inhalated, the airborne microbes can have harmful effects on plant, animal, and human health (Fröhlich-Nowoisky et al., 2016). Airborne pathogenic microorganisms are of considerable interest since they can induce diseases such as skin and internal organs infections, cardiovascular diseases, and respiratory problems (Chen et al., 2020; Moelling, and Broecker, 2020).
Although considerable efforts have been made in last decades to characterize the elemental composition of PM10 and identify its natural and anthropogenic sources in different environments and geographic areas (Werner et al., 2014; Padoan et al., 2016; Massimi et al., 2020), it wasn't until fairly recently that the development of next-generation sequencing techniques coupled to DNA metabarcoding analysis provided culture-independent robust tools for a fine-scale molecular characterization of airborne microbial communities (Dommergue et al., 2019; Ferguson et al., 2019). In this context, studies on the PM-associated microbial taxa in urban environments are still geographically scattered and conducted over a short timescale, leading to a lack of general conclusions about their dynamics, sources, composition and functions (Ruiz-Gil et al., 2020). The urbanization and the invariability of land use can promote the homogenization of the airborne microbiota in urban areas as well as lead to a decrease of species richness (Bowers et al., 2011; Liu et al., 2019). However, multiple and site-specific factors might have great influence on the airborne microbial community structure of metropolitan areas such as degree of urbanization, topography, industrial activities (Bowers et al., 2011; Stewart et al., 2020), climate (Uetake et al., 2019), short- and long-range transport of air masses (Liu et al., 2019) as well as adventitial events including dust storms (Gat et al., 2017) and peaks of pollution (Yan et al., 2018). The relationships of these variables with prokaryotic and eukaryotic diversity have been also attested in PM10 samples collected in one of the most polluted and urbanized areas of Northern Italy (the Po Valley, Franzetti et al., 2011; Innocente et al., 2017) as well as in suburban coastal site of Southeastern Italy (Lecce, Romano et al., 2020; Fragola et al., 2021). A strong seasonal shift of airborne microbial communities has been detected partially depending on microorganisms trophic mode and synchronization with life cycles of higher organisms such as plant growth and decay (Tignat-Perrier et al., 2020). Hence, the process of understanding atmospheric microbial diversity in urban areas is complex and needs more location-specific data to support policy decisions related to air quality and human health.
In a preliminary study we investigated the microbial community variations and the source-sink relationships associated to PM10, and their main local sources in the surrounding environment (paved road surfaces and leaf surfaces of Quercus ilex) in three urban sites of Rome, characterized by differential pollution rate during fall 2020 (Pollegioni et al., 2022). It was highlighted that an increase of extremotolerant microbes and opportunistic human pathogenicity in fungal communities was related to an increase of pollution levels. However, up to now, an overview of seasonal variations of airborne microbial communities are still missing in Rome. Similarly, information about the influence of air mass movements and local sources on microbial structure thought the year is lacking. In this study, we collected PM10 samples in the same urban macro-areas of Rome: urban park, residential area and an area polluted by traffic. We combined an marker gene (ie 16S or ITS) metabarcoding approach with air pollution analysis in order to 1) investigate the community compositions and structures of PM10-associated bacteria and fungi across four different seasonal periods for a one-year comparison, 2) analyze the inferred ecological functions of the microbial taxa in the airborne samples, 3) evaluate the impact of air mass transport and other potential drivers on seasonal community structures/functions of microbes, and 4) highlight putative variations in bacterial and fungal functional compositions across a gradient of urbanization and traffic density in Rome.
Material and methods
Sites description
In this study, the PM10 samples were collected in Rome (Italy) from 6 to 12 October 2020 (fall), 23 February to 3 March 2021 (winter), 25 to 31 May 2021 (spring) and 23 to 30 July 2021 (summer). As described in Pollegioni et al. (2022), three functional sites, different in terms of the anthropogenic load, have been selected: a) South-Western Road Traffic zone (Enrico Fermi Square, 41.864025 Long. 12.469712) characterized by dense population and intense traffic, b) Central Residential zone (Arenula street, Lat. Lat. 41.87184 Long. 12.46953) situated in a residential restricted traffic area and near to a small public garden, c) North-Eastern Urban Park zone (Villa Ada Park, Lat. 41.933001.12 Long. 12.507532) the second largest urban Park in the city (Figure 1A). Continuous monitoring of PM10 by the Regional Agency for Environmental Protection (ARPA Lazio) in each site corroborated the differences in terms of anthropogenic load among them (Figure 1B).
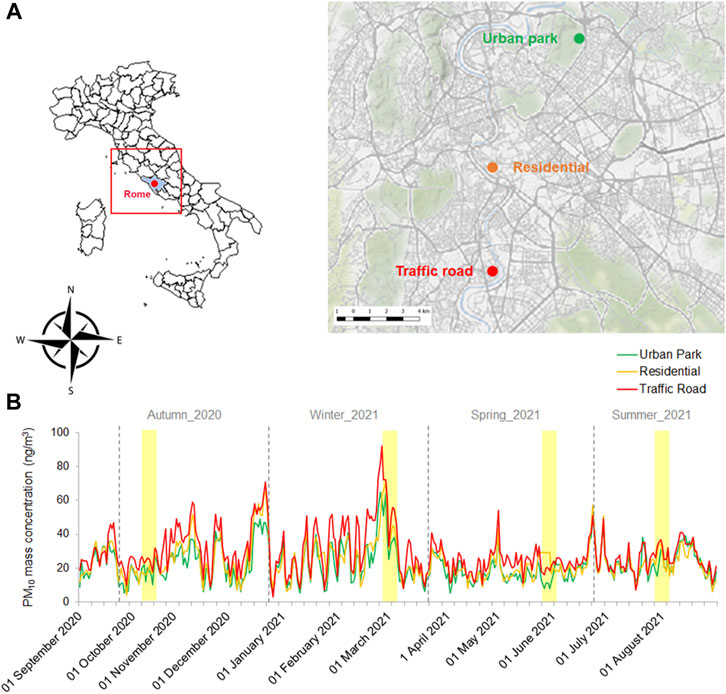
FIGURE 1. Geographic location of the three urban sites (Urban Park, Residential and Road Traffic) selected in Rome (A) and their PM10 mass concentration recorded from 01 September 2020 to 31 August 2021 (B). Four sampling campaigns of Early Autumn 2020, Late Winter 2021, Late Spring 2021 and Mid-Summer 2021 were reported as yellow shaded areas.
Meteorological data including wind speed and direction, temperature, relative humidity, and rainfall level were also provided by ARPA Lazio by using a weather station (Lat 41.90850, Long 12.49356), located at roof level in the city center and approximately at the same distance (∼4–6 km) from the three urban sites (Supplementary Figures S1, S2). In total accordance with the global description of the climate, three meteorological variables, temperature, relative humidity, and rainfall, were also monitored by ARPA Lazio at the three functional sites of Rome during the sampling campaigns. Finally, the air masses reaching Rome at 100 m and 500 m above the ground level at 12:00 UTC were determined by six-day analytical back-trajectory analysis, with the HYSPLIT (Hybrid Single Particle Lagrangian Integrated Trajectory) model (http://ready.arl.noaa.gov) for each week of sampling. All samples were categorized as either continental meaning of Eurasian continent origin, Pacific or African based on their latitude/longitude location 144 h prior to arriving at Rome. This methodology allowed us to identify the possible influence of long-range transported air masses and North African dust outbreaks on the levels of PM10 and on their chemical compositions.
Sample collection
PM10 membrane filters have been used as matrix for metabarcoding and chemical analysis. In each urban site, two active PM10 samplers were installed 3 m above the ground within an environmental monitoring station (property of ARPA Lazio). As fully reported in Pollegioni et al. (2022), for chemical analysis, PM10 sample collection was carried out with a HSRS (High Spatial Resolution Sampler, Fai Instruments, Rome, Italy) at 2 L/min flow rate on Polytetrafluoroethylene (PTFE) membrane filters (47 mm diameter, 0.45 μm pore size). In order to get sufficient genetic material because of low environmental concentration of bio-aerosols, UV-sterilized polycarbonate filters (47 mm diameter, 0.8 μm pore size) were used to collect PM10 with an Impact Sampler (SKC, Dorset, England) at 10 L/min flow rate (Ferguson et al., 2019). To improve the DNA recovering efficiency, each polycarbonate filter was changed every 48 h at each sampling period. Once collected, filters were transported and immediately processed in the laboratory. In total, three polycarbonate filters and 1 PTFE filter were available from each urban site and for each season.
DNA extraction and 16S/ITS metabarcoding
The three polycarbonate filters were aseptically pooled per each urban site for each season and used for the DNA extraction with DNeasy PowerWater Kit (Qiagen) as previously described in Pollegioni et al. (2022). The extracted DNA was amplified via PCR using universal primer pairs: Forward: 341F 5′-CCTACGGGNGGCWGCAG-3′ and Reverse: 805R 5′-GACTACHVGGGTATCTAATCC-3′which target the hypervariable V3-V4 regions of the bacterial 16S rRNA gene (Klindworth et al., 2013) and Forward: ITS1 5′-TCCGTAGGTGAACCTGCGG-3′ and Reverse: ITS2 5′- GCTGCGTTCTTCATCGATGC -3′ targeting the ITS1-5.8S region of the fungal rRNA gene (White et al., 2013). In order to integrate relevant flow-cell binding domains and unique indices, dual indexed libraries were generated using Nextera XT library preparation technology according to manufacturer’s protocol (Illumina, San Diego, CA, United States). Eighteen equimolar single-stranded DNA libraries were pooled and then pair-ended sequenced (2 × 300 cycles) on Illumina MiSeq platform by Sequentia Biotech company (Barcelona, Spain).
Bioinformatic analysis
Demultiplexed Illumina paired-end raw reads of the two amplicons libraries (16S and ITS1) were pre-processed to generate Amplicon Sequence Variants (ASVs) using DADA2 R package (Callahan et al., 2016). Briefly, primers were removed, sequences forward and reverse trimmed based on the Quality score plots (forward 280 bp, reverse 270 for 16S and forward 250 bp, reverse 200 for ITS1). The filtered reads were then used to train the error model using machine learning approach. Forward and reverse reads were dereplicated to generate unique sequences and denoised (collapsed) in amplicon sequence variants (ASVs) applying the trained error model. Finally, forward and reverse reads were merged and checked for chimera sequences. Representative sequences for each ASV were taxonomic assigned using the naive Bayesian classifier (Wang et al., 2007) against SILVA database (version 132) for 16S amplicons and against UNITE ITS database (Kõljalg et al., 2013) for ITS amplicon. ASVs assigned to chloroplast, mitochondria or “unknown” (i.e., that could not be classified at the kingdom level) were removed and excluded from analyses.
Analyses of chemical components
PTFE membrane filters were used for the gravimetric assessment of PM10 mass concentration, using an automated microbalance (Sartorius R180D Analytical Balances, Sartorius AG, Göttingen, Germany). Separation and sub-sequent chemical analysis of both the water-soluble and insoluble fraction of PM10 were performed following the protocols of Canepari et al. (2006a); Canepari et al. (2006b). This two-step chemical analytical method is highly efficient for increasing the selectivity of PM10 elemental components as specific source tracers, for both natural and anthropogenic emission sources (Massimi et al., 2020). Content of 38 elements in PM10 (Al, As, B, Ba, Be, Bi, Ca, Cd, Ce, Co, Cr, Cs, Cu, Fe, Ga, K, La, Li, Mg, Mn, Mo, Na, Nb, Ni, Pb, Rb, Sb, Se, Sn, Sr, Te, Ti, Tl, U, V, W, Zn, and Zr) in the two solubility fractions was estimated by analysing water-extracted and acid-digested solutions with inductively coupled plasma mass spectrometry (ICP-MS, Bruker 820-MS, Billerica, MA, United States), The limits of detection (LODs; Supplementary Tables S9–S11) were set at 3 times the standard deviation (SD) of 5 replicate blank determinations. Further details on the preparation of external standards for the calibration and internal standards to control the nebulizer efficiency are reported in Massimi et al. (2020).
Statistical analysis
All the exploratory statistical analyses were carried out using R v3.6.0 programming language (R Development Core 2019) and the cited associated packages.
In order to mitigate the impact of differences in the sequence count on the species richness evaluation, ASV tables were normalized to the second lowest number of reads, 13,925 for 16S and 46,859 for ITS region, using the function rarefy from the vegan R package (Oksanen et al., 2015). Significant seasonal differences in percentage of relative abundance of Phyla, Classes and Orders were inferred by non-parametric Kruskal-Wallis test for equal medians and subsequent Mann-Whitney pairwise test. Three alpha diversity descriptors, Species Richness, Chao1 and Shannon entropy index, were calculated on the rarefied dataset for bacterial and fungal communities using phyloseq v1.32 package (McMurdie and Holmes, 2013). Significant seasonal differences in the values of alpha diversity estimators, PM10 content, temperature, relative humidity, and precipitation were tested by using non-parametric Kruskal-Wallis analysis, and then post-hoc Dunn tests with package agricolae (de Mendiburu, 2021). Correlation matrices between multiple variables, including alpha diversities, PM10 mass concentration, the three meteorological data and 18 selected pollution tracers (Al_ins, Cr_ins, Cs_sol, Cs_ins, Cu_ins, Fe_ins, Li_ins, Mg_sol, Mn_ins, Mo_ins, Na_sol, Pb_ins, Rb_sol, Rb_ins, Sb_ins, Ti_ins, Tl_sol, and Tl_in), were generated by computation of Spearman’s correlation index with Bonferroni correction using the ggcorrplot package (Kassambara, 2019).
Beta-diversity was used to evaluate changes in bacterial and fungal community composition across seasons and urban sites. Normalized datasets were Hellinger transformed then a hierarchical cluster analysis of samples based on pairwise Bray-Curtis dissimilarity matrix were performed with the hclust function (average distance) implemented in vegan. Bootstrap support for each node was determined by resampling 1,000 times. Principal Coordinate Analysis (PCoA) was also used to display the Bray-Curtis dissimilarities in the microbial composition between samples. Likewise, to test for dissimilarity differences between microbial communities based on two factors, season and type of urban sites, we applied a non-parametric two-way ANOSIM test implemented in the vegan package (anosim function), with the number of permutations set to 9,999. Finally, the most abundant taxa (at the genus level) of each community were correlated to the ordinations of PCoA, and statistically significant correlations between them were computed with envfit function implemented in the vegan package.
The relationships between ASV relative abundances in microbial communities and environmental metadata (PM10 content, temperature, relative humidity, and precipitation) were explored by Bray-Curtis distance-based redundancy analysis (dbRDA). However, predictors were preliminary investigated to understand if they were collinear and then redundant. Linear dependencies between environmental variables were assessed i) using function vif.cca and ii) considering Spearman’s correlation index. In this way, we excluded relative humidity from further analysis based on its strong negative correlation with temperature. The significance of the model was confirmed with an analysis of variance (ANOVA) for the dbRDA and variables that were significantly associated to community variation were detected (anova.cca function for “terms”). Statistically significant variance (adjusted R2) explained by each predictor was computed applying model selection with function ordiR2step and anova.cca in the vegan package. In addition, Principal Component Analysis was also performed with R-package ggbiplot (Vu, 2011) to explore the seasonal and urban site-specific patterns of selected chemical components in the aerosol samples. The variables were selected depending on their ability to selectively trace PM10 emission sources (Massimi et al., 2020).
Finally, two-step functional classification was also introduced to describe the predominant habitats/ecological function profiles of the microbial species identified in the aerosol samples. First, as described by Romano et al. (2020), we classified the bacterial phyla based on their main habitats: terrestrial and aquatic environments, soil, marine and fresh water, human, animal, and insect, and other. Then, metabolic or other ecologically relevant functions of microbial taxa were predicted using Functional Annotation of Prokaryotic Taxa (FAPROTAX) database (http://www.loucalab.com/archive/FAPROTAX/lib/php/index.php?section=Home), as implemented in microeco R package (Liu et al., 2021), which extrapolates putative functional profiles based on the literature of cultured bacterial taxa. Fungal functional traits were predicted using the FUNGuild (Nguyen et al., 2016) and FungalTraits (Põlme et al., 2020) databases. As an additional functional group, the human opportunistic-pathogenic fungi were also identified following the classification scheme found in de Hoog et al. (2020). The 27 most abundant functional groups (relative abundance ≥1%) in bacteria and fungi at species-level were shown by a bubble diagram using ggplot2 and reshape2 (Francis, 2017) R packages. Finally, Cluster Analysis based on Euclidean distance and Complete linkage was performed to investigate the relationships between the PM10 content, the chemical components used as pollution tracers, and the predominant habitats/ecological function profiles of the microbes detected in our samples using ClustOfVar R package (Chavent et al., 2012).
Results
Mass concentrations, meteorological parameters and air masses
The PM10 mass concentration varied among the three sampling sites across each season, progressively increasing from the Urban Park to Residential and Road Traffic area (Figure 1B). Although the PM10 mass concentrations were also characterized by overall significant seasonal variations, the Dunn-test results revealed little difference in terms of PM10 between fall, spring and summer. On the contrary, the PM10 content in winter was significantly higher compared to other seasons, reaching the maximum values of 53.9″ μg m-3″, 55.5″ μg m-3″, and 79.7″ μg m-3″, at Urban Park, Residential and Traffic Road, respectively (Table 1).
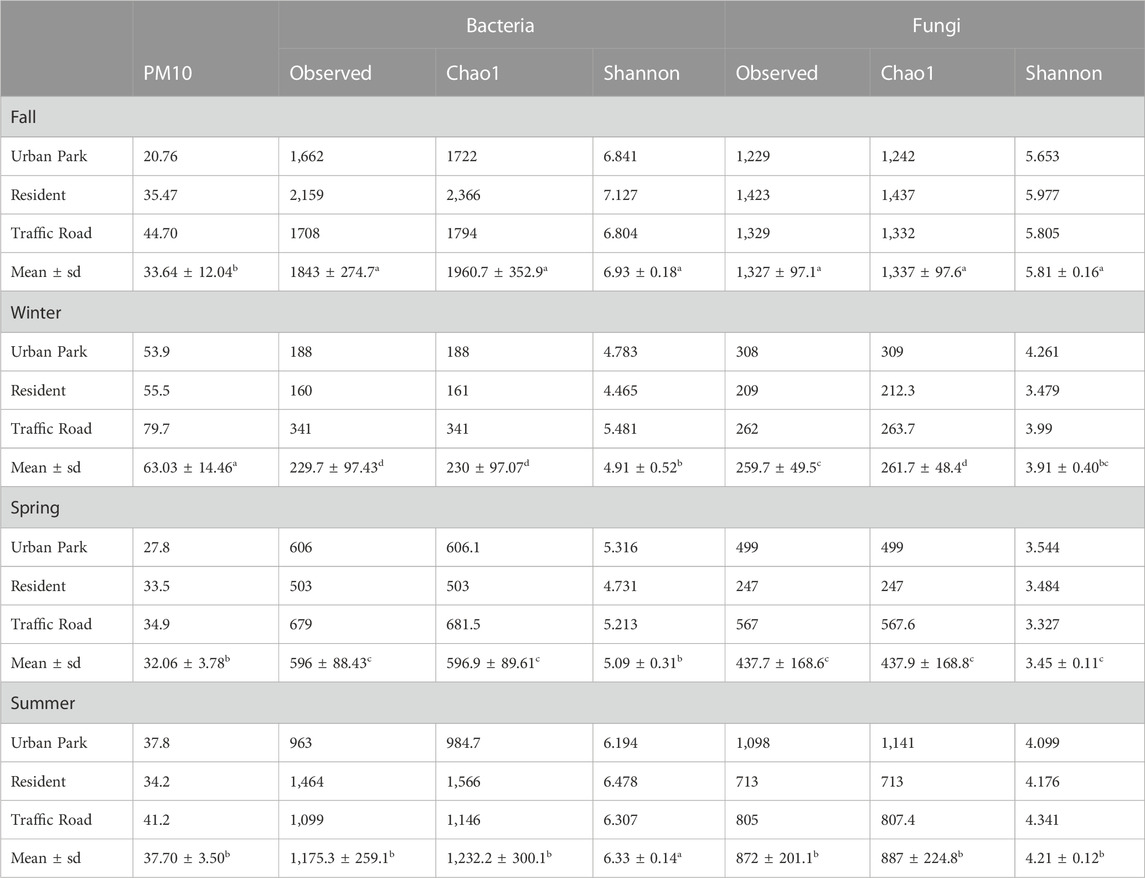
TABLE 1. PM10 mass concentration (ug/m3), and alpha-diversity parameters for all bacterial and fungal ASVs found at species-level in the aerosol samples collected in three urban sites of Rome (Urban Park, Residential and Road Traffic) during the sampling campaign of Autumn 2020, Winter 2021, Spring 2021 and Summer 2021. Observed Species Richness, Chao1 index and Shannon_H index calculated on data rarefied to the second smallest library size were reported. For comparisons between the seasons, mean values showing different letters were significantly different at P ˂ 0.05 according to the non-parametric post-hoc multiple Dunn test after providing significance within the Kruskal-Wallis analysis.
Meteorological data including mean wind speed and direction, temperature, relative humidity, and rainfall level were recorded from September 2020 and August 2021 (Supplementary Figures S1, S2). In particular, the minimum average atmospheric temperature in the center of Rome was recorded during winter sampling (12.69°C ± 0.90°C) and average maximum during summer sampling (29.21°C ± 0.82°C), corresponding to a seasonal decline of atmospheric humidity (HR) from winter to summer, with an average maximum value of 70.63% ± 4.04% and average minimum of 43.38% ± 4.14%, respectively (Supplementary Table S1). In correspondence with a Mediterranean weather, the rainy seasons were fall and spring. All sampling campaigns were conducted in the periods characterized by low precipitation with a highest sum of 14.47 ± 6.11 mm during fall. Two predominant clusters of local airflows were observed during sampling: southwest (SW) and northeast (NE) (Supplementary Table S1; Supplementary Figure S2). The prevailing wind direction was north-east in winter, while it was south-west in spring and summer with wind lowest mean speed value registered in winter. The backward trajectory model indicated that the source regions of air masses shifted from Pacific to Continental twice during sampling campaigns, from fall to winter, and from spring to summer, with temporary dust intrusions from Saharan regions observed in Rome from 24th to 27th February 2021 (Supplementary Figures S3).
Alpha diversity of bacterial and fungal communities
After sequence preprocessing, a total of 8,274 and 4,858 amplicon sequence variants (ASVs) were obtained for bacteria and fungi, respectively. The rarefied values of the alpha-diversity estimators for bacteria and fungi are summarized in the Table 1. In the three urban locations (Urban Park, Residential and Road Traffic), no significant differences were detected for the alpha-diversity estimators among sites, either for bacteria (Kruskal-Wallis tests p ≥ 0.92) or fungi (Kruskal-Wallis tests p ≥ 0.79) (Figure 2). Seasonal patterns were characterized by significantly higher average values of Chao1 for both bacteria and fungi in autumn (Kruskal-Wallis tests p ≤ 0.05), While for the winter season, Chao1 recorded the lowest value. (Table 1; Figure 2). For bacteria, winter and spring periods were characterized by significant lower Shannon-diversity than in summer and fall samples. On the contrary, mean Shannon-diversity estimates of fungal communities were relatively low in all seasons except for fall where the highest mean value of Shannon index was observed (Table 1; Figure 2). Alpha diversity seasonal variation of bacterial and fungal communities wasn’t significantly associated (Spearman Correlations, p > 0.05) to variation in either meteorological or PM10 mass concentrations (Supplementary Figure S4). Despite the lack of the overall correlation, the microbial communities of Rome sampled during the cold season showed the lowest diversity estimators as well as the highest PM10 content at each urban site (Table 1).
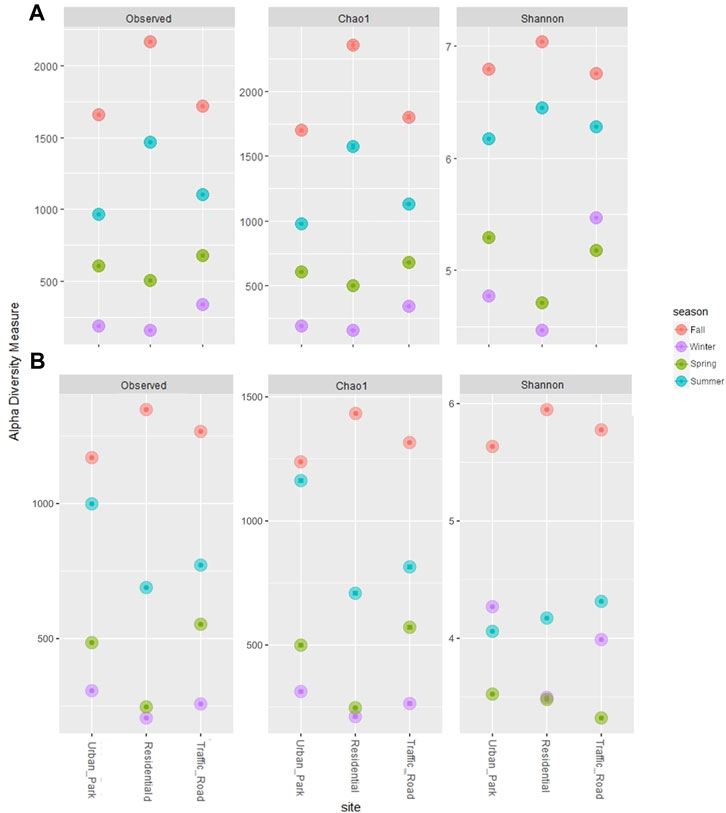
FIGURE 2. Three alpha diversity estimators (Observed Species Richness, Chao1 index and Shannon_H index) of bacterial (A) and fungal (B) communities of PM10 samples collected in three urban sites of Rome across four seasons (Fall, Winter, Spring and Summer).
Taxonomic composition and structure of microbial communities
Bacterial communities
Bacterial communities included 40 phyla, 78 classes and 182 orders (Supplementary Table S2). The predominant phyla in the total dataset included the Proteobacteria (29.88% ± 10.93%), followed by Actinobacteria (24.48% ± 7.27%), Firmicutes (24.39% ± 14.77%), Bacteroidetes (8.37% ± 2.59%), Synergistetes (2.41% ± 4.91%), Thermotogae (2.24% ± 4.80%), and Chloroflexi (1.69% ± 1.01%), with a relative abundance greater than 1% (Figure 3A). The composition of the community structure varied across seasons, with Proteobacteria (fall: 43.49 ± 6.17%a, summer: 30.22 ± 4.72%b, winter: 28.29 ± 5.67%b, spring: 17.51 ± 5.60%c, Mann-Whitney pairwise test p < 0.05), Firmicutes (spring: 45.49 ± 10.47%a, summer: 21.13 ± 6.95%b, winter: 20.16 ± 5.06%b, fall: 10.79 ± 2.86%c, Mann-Whitney pairwise test p < 0.05) and Actinobacteria (summer: 32.78 ± 4.73%a, fall: 26.01 ± 2.29%b, winter: 20.62 ± 6.30%c, spring: 18.52 ± 4.84%c, Mann-Whitney pairwise test p < 0.05) as the predominant phylum for fall, spring and summer, respectively. In addition, Synergistetes (winter: 9.53 ± 5.0%a, spring: 0.06 ± 0.08%b, summer: 0.02 ± 0.04%b, fall: 0.02 ± 0.01%b, Mann-Whitney pairwise test p < 0.05) and Thermotogae (winter: 8.96 ± 6.03%a, spring: 0.00%b, summer: 0.00%b, fall: 0.00%b, Mann-Whitney pairwise test p < 0.05) were abundant only in winter (Figure 3A). Seasonal changes at class (Supplementary Figure S5) and order levels (Figure 3C) showed, during fall, the most abundance of Sphingomonadales (8.74% ± 1.84%), Rhodobacterales (7.19% ± 4.02%), Rhizobiales (5.19% ± 1.27%), Betaproteobacteriales (5.21% ± 1.42%) and Pseudomonadales (3.33% ± 0.80%) which were also present on summer and winter samples barely comprising ∼25% and ∼17% of reads on average, respectively. However, four orders, Clostridiales, Lactobacillales, Selenomonadales and Bacteroidales accounted for ∼52% of relative abundance in the spring communities. Micrococcales (summer: 15.87 ± 1.24%a, fall: 9.96 ± 1.49%b, winter: 8.55 ± 1.34%c, spring: 6.90 ± 2.28%c, Mann-Whitney pairwise test p < 0.05) was substantially enriched in summer microbes. Instead, Synergistales (winter: 9.53 ± 5.59%a, spring: 0.06 ± 0.07%b, summer: 0.02% ± 0.03% b, fall: 0.02% ± 0.01% b, Mann-Whitney pairwise test p < 0.05) and Petrotogales (winter: 8.96 ± 6.03%a, spring: 0.00%b, summer: 0.00%b, fall: 0.00%b, Mann-Whitney pairwise test p < 0.05) bacterial orders were detected only in microbiome isolated from winter samples (Figure 3C).
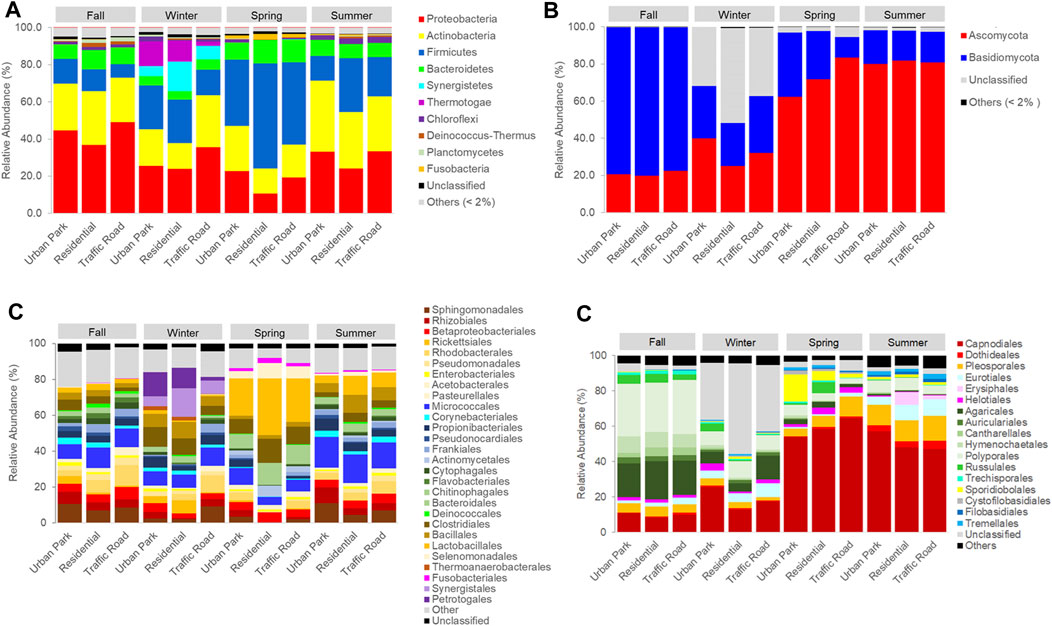
FIGURE 3. The most abundant (frequency ≥2%) bacterial and fungal phyla (A,B) and orders (C,D) in each PM10 sample collected in three urban sites of Rome across four seasons (Fall, Winter, Spring and Summer).
Standing on the Bray-Curtis dissimilarity matrix calculation, bacterial communities of airborne PM10 grouped only by season (Figures 4A, B). In particular, the composition of bacteria in winter was quite different from that in other seasons. Venn diagram highlighted that 22.6% of ASVs was shared among all bacterial samples with the large percentage of private ASVs detected in winter and fall (14.8% and 12.5%) (Supplementary Figure S6A). In agreement, statistical differences among samples were detected by season (R = 0.77, p < 0.01, ANOSIM) but not for sampling location (R = 0.25, p = 0.29, ANOSIM). To find which taxa are mainly responsible for the observed seasonal gradients, we fitted the most abundant genera to the ordinations. Taxa with most significant correlations with sample ordinations are shown as correlation arrows in Supplementary Figure S7A. Out of 12 most abundant genera accounting for a total relative abundance of ≥20%, ten were mainly responsible for the observed seasonal gradients of airborne samples such as Petrotoga and Acetomicrobium for winter (Supplementary Figure S7A). Fall and summer samples were also especially enriched in Sphingomonas, Methylobacterium, Massilia, Rubellimicrobium, Paracoccus, Blastococcus, Nocardioides, and Kocuria. The differential abundance of Streptococcus and Actinomyces accounted for the main divergence of spring samples from the remaining microbial communities. Finally, despite this sharp seasonal repartition of airborne communities, Spearman’s rank correlation between the PM10 mass and the relative abundance of the top functional groups highlight that Paracoccus (e.g., P. contaminans) gradually increase in the airborne communities passing from Urban Park, to Residential and Traffic Road in three sampling seasons, fall, winter and summer (Supplementary Figure S8).
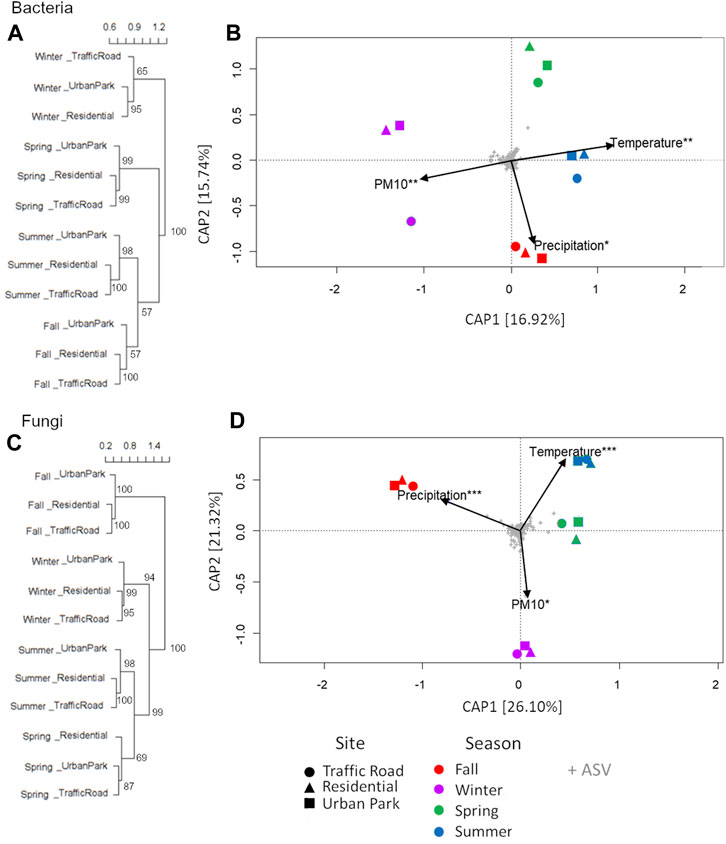
FIGURE 4. Clustering analysis (A,C) and distance-based redundancy analysis (db-RDA) (B,D) of airborne microbial community composition over four consecutive seasons at three urban sites of Rome (Urban Park, Residential and Traffic Road). Clusters and db-RDA plots are constructed from a Bray-Curtis distance matrix calculated from Hellinger-transformed ASV counts data for bacteria and fungi. Constrained ordinations of samples grouped by seasonal period with environmental factors are shown. The total variance (in percent) explained by each axis is indicated in parentheses. Asterisks represent significance of the environmental variables under permutation tests (1,000 permutations): ***p < 0.001; **p < 0.01; *p < 0.05.
Fungal communities
Fungal communities were composed by 9 phyla, 33 classes and 99 orders (Supplementary Table S3). The predominant fungal phyla were Ascomycota and Basidiomycota across all samples (Figure 3B). However, the average relative abundance of Basidiomycota was 78.80 ± 1.36%a in the fall communities, higher (Mann-Whitney pairwise tests p < 0.001) than in winter (27.21 ± 3.86%b), spring (23.80% ± 10.56% bc) and summer samples (16.96% ± 1.04% c). Fungal taxa clearly showed an equally strong seasonal pattern at order levels. The most abundant fungal classes of fall samples were Agaricomycetes (77.43% ± 1.48%) followed by Dothideomycetes (15.89% ± 1.03%), and Eurotiomycetes (2.46% ± 1.22%), in contrast with spring and summer samples mostly composed by Dothideomycetes (∼67%), followed by Agaricomycetes (8.34%–11.29%), Eurotiomycetes (1.61%–8.27%), Tremellomycetes (3.22%–4.02%), Microbotryomycetes (1.55%–7.66%) and Leotiomycetes (∼3%), with winter samples showing intermediate microbial profiles (Supplementary Figure S5). The fungal orders most responsible for these marked differences included the Polyporales (fall: 29.35 ± 1.23%a, winter: 8.40 ± 0.78%b, summer: 6.12 ± 1.10%c, spring: 4.17 ± 1.36%d, Mann-Whitney pairwise test p < 0.01), Agaricales (fall: 20.05 ± 1.23%a, winter: 8.12 ± 4.11%b, spring: 2.36 ± 0.68%c, summer: 0.57 ± 0.24%d, Mann-Whitney pairwise test p < 0.05), Cantharellales (fall: 3.93 ± 1.03%a, winter: 0.58 ± 0.28%b, summer: 0.25 ± 0.19%bc, spring: 0.24 ± %c, Mann-Whitney pairwise test p < 0.05), Hymenochaetales (fall: 8.59 ± 0.91%a, winter: 1.15 ± 0.25%b, spring: 0.78 ± 0.47%b, summer: 0.24 ± 0.06%c, Mann-Whitney pairwise test p < 0.05), Russulales (fall: 4.34 ± 0.82%a, spring: 2.56 ± 3.07%b, winter: 1.81 ± 1.98%b, summer: 0.16 ± 0.12%b, Mann-Whitney pairwise test p < 0.05), and Auriculariales (fall: 3.19 ± 0.25%a, winter: 1.74 ± 0.35%b, spring: 0.32 ± 0.25%c, summer: 0.30 ± 0.06%c, Mann-Whitney pairwise test p < 0.05), highly enriched in the fall microbes and Capnodiales (spring: 58.93 ± 4.69%a, summer: 52.3 ± 5.45%a, winter: 18.52 ± 5.83%c fall: 9.67 ± 1.05%d, Mann-Whitney pairwise test p < 0.05), and Pleosporales (summer: 12.21 ± 1.06%a, spring: 7.21 ± 3.16%b, fall: 5.27 ± 0.47%b winter 3.17 ± 0.94%c, Mann-Whitney pairwise test p < 0.05) predominant in spring and summer communities (Figure 3D). Clustering analysis of ASVs confirmed that the three fungal communities collected in Urban Park, Residential and Traffic Road were seasonally grouped, with fall and winter samples strongly differentiated from those of spring and summer (Figures 4C, D). In agreement with bacterial community structure, statistically significant differences were detected among different seasons (R = 0.85, p = 0.02, ANOSIM) but not for sampling location (R = 0.08, p = 0.65, ANOSIM). Taxa with most significant correlations with sample ordinations are shown as correlation arrows in Supplementary Figure S7B Similar to bacterial microbiome, up to 12 most abundant fungal genera accounting for a total relative abundance of ≥30%, appeared as robust indicator taxa of the sampling periods (Supplementary Figure S7B). In particular, Mycosphaerella, Cladosporium, and Alternaria were assigned to Ascomycota phylum and made up a remarkable fraction of eukaryotic communities across spring and summer. Conversely, Ceripodia, Xylodon and Phlebia belonging to Basidiomycota were prevalent during fall as well as Trametes, Bjerkandera, Aspergillum and Penicillum in winter (Supplementary Figure S7B). None of most abundant fungal genera has been shown to gradually increase passing from Urban Park, to Residential and Traffic Road during each sampling season.
Impact of environmental and pollution variables on airborne microbial structure
The environmental variables (temperature, relative humidity, and precipitation) and PM10 mass concentrations at three urban sites of Rome were not all independent. Temperature showed a significant negative correction with relative humidity (r = −0.96. p > 0.001) as expected from the climatic Mediterranean conditions of Rome. To assess the impact of different meteorological and pollution parameters on seasonal changes in community composition, we applied the dbRDA. Three explanatory variables, temperature, precipitation and PM10, were selected based on their lack of collinearity. As reported in Supplementary Table S4 and showed in Figure 4B, seasonal trends in bacterial community diversity were significantly explained by the temperature gradient (6.87% of the explained variance), followed by concentration of PM10 (6.70% of the explained variance) and precipitation level (6.00% of the explained variance). Temperature was also a dominant environmental factor explaining seasonal variations in fungal communities (17.03% of the explained variance). Similarly, the composition of fungal communities was strongly influenced by rain levels (17.44% of the explained variance) followed by PM10 mass concentration (6.13% of the explained variance) (Figure 4D, Supplementary Table S4).
Impact of PM10 elemental components on airborne microbial community function
The Principal Component Analysis (PCA) applied to the mean elemental concentrations of PM10 revealed that the winter samples were mostly characterized by a prevalence of the insoluble fractions of elements which are known as tracers of anthropogenic emission sources (Figure 5A) (Massimi et al., 2020). Among these elemental components, such as lead (Pb_Ins), antimonium (Sb_Ins), iron (Fe_ins), and manganese (Mn_Ins), are tracers of the non-exhaust emission of vehicular traffic, being components of brake pads ‘wear, disks and tires (Namgung et al., 2016). The soluble fraction of three main tracers of biomass burning emissions, cadmium (Tl_sol), rubidium (Rb_Sol) and cesium (Cs_Sol), were clearly detectable in the PM10 of all urban sites at high levels during winter. In the same latter season as well as in summer, high concentrations of the insoluble fractions of allumium (Al_Ins), rubidium (Rb_Ins), lithium (Li_Ins), cesium (Cs_Ins), tallium (Tl_Ins) and titanium (Ti_Ins) were also found. These elements are constituents of soils and often attributed to the removal and resuspension of deposited material from the ground to the atmosphere, both for natural (i.e., wind) and anthropogenic activities (i.e., vehicular traffic) (Massimi et al., 2021). Two main tracers of sea salt source, magnesium (Mg_Sol) and sodium (Na_Sol), were mostly recorded in the fall and spring airborne PM10 samples (Figure 5A; Supplementary Tables S5–S8).
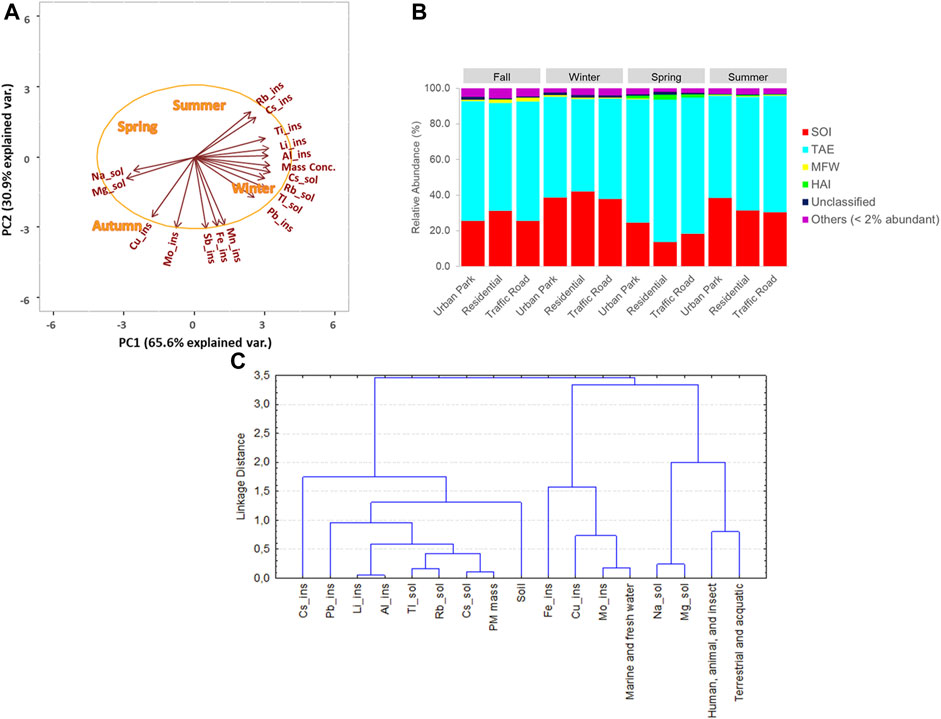
FIGURE 5. Principal Component Analysis of the selected elemental components of PM10 mass (A), percentage contribution of the habitat-types, terrestrial and aquatic environments (TAE), soil (SOI), marine and fresh water, human (MFW), animal, and insect and other (HAI) associated with the bacterial phyla detected in the aerosol PM10 samples in four seasons (B), and the hierarchical cluster analysis between selected elemental components of PM10 mass and the percentage contribution of habitat types based on Euclidean distance and Complete linkage (C) were reported.
Most of the bacterial taxa at the phylum level were also classified as likely coming from terrestrial and aquatic habitats, followed by soil, human, animal, and insect origin and marine-fresh water environments (Figure 5B; Supplementary Table S9). Bacterial phyla with soil origin, negatively correlated to seasonal precipitation (Spearman Index = −0.65, p = 0.022), were found in greater proportion in winter (39.50% ± 2.27%) and summer (33.42% ± 4.35%) than in spring (18.80% ± 5.48%) and fall (27.46% ± 3.20%). In contrast, terrestrial and aquatic phyla, positively impacted by seasonal precipitation (Spearman Index = 0.63, p = 0.029), were substantially enriched on average in bacteria isolated during fall (64.96% ± 3.73%) and spring (75.24% ± 5.43%) in comparison to winter (54.98% ± 2.57%) and summer (61.16% ± 4.12%). Cluster analysis of PM10 content, pollution tracers, and the predominant functional habitats of bacteria identified two main groups of variables (Figure 5C). The three main tracers of biomass burning emissions, cadmium (Tl_sol), rubidium (Rb_Sol) and cesium (Cs_Sol), and the insoluble fractions of lead (Pb_Ins), lithium (Li_Ins), cesium (Cs_Ins), tallium (Tl_Ins) and titanium (Ti_Ins), constituents of soils, tend to cluster together with PM10 content and the relative abundance of bacteria showing soil origin (Figure 5C). The second cluster included the remaining chemical tracers such as two main tracers of sea salt source, magnesium (Mg_Sol) and sodium (Na_Sol), and the percentage of bacterial taxa likely coming from terrestrial and aquatic habitats, human, animal, and insect origin and marine-fresh water environments (Figure 5C).
In addition, functional prediction revealed the presence of 74 predicted functions for bacterial community. Chemoheterotrophy (53.17% ± 13.76%; aaerobic 32.30% ± 14.04% and anaerobic 20.87% ± 17.54%), fermentation (21.08% ± 18.82%), and animal parasites or symbionts (8.37% ± 5.66%), attributed to C cycling and were the most abundant groups (Figure 6A, Supplementary Tables S10, S11). At seasonal level, anaerobic chemoheterotrophy (47.81% ± 10.70%), fermentation (50.32% ± 11.06%), and animal parasites or symbionts (16.74% ± 4.21%) were the predominant groups in spring. Within the S cycling functional group, bacteria involved in respiration of sulfur compounds and sulfate respiration were preferentially recorded in winter. It is worth noting that Spearman’s rank correlation between the PM10 mass and the relative abundance of the top functional groups highlight that bacteria with the functional capacity for hydrogen oxidation and nitrogen cycling showed an increasing trend in terms of relative abundance, transitioning from the Urban Park to the most polluted site, Traffic Road, during three sampling seasons (fall, winter and summer). (Figure 6A; Supplementary Figure S8). The Cluster Analysis based on Euclidean distance indicated that bacteria belonging to hydrogen_oxidation function group or involved in the nitrogen and sulfur cycles were linked to the levels of PM10, soluble cadmium (Tl_sol), rubidium (Rb_Sol) and cesium (Cs_Sol), the insoluble lead (Pb_Ins), lithium (Li_Ins), cesium (Cs_Ins), tallium (Tl_Ins) and titanium (Ti_Ins) detected in Rome across four seasons (Figure 7A).
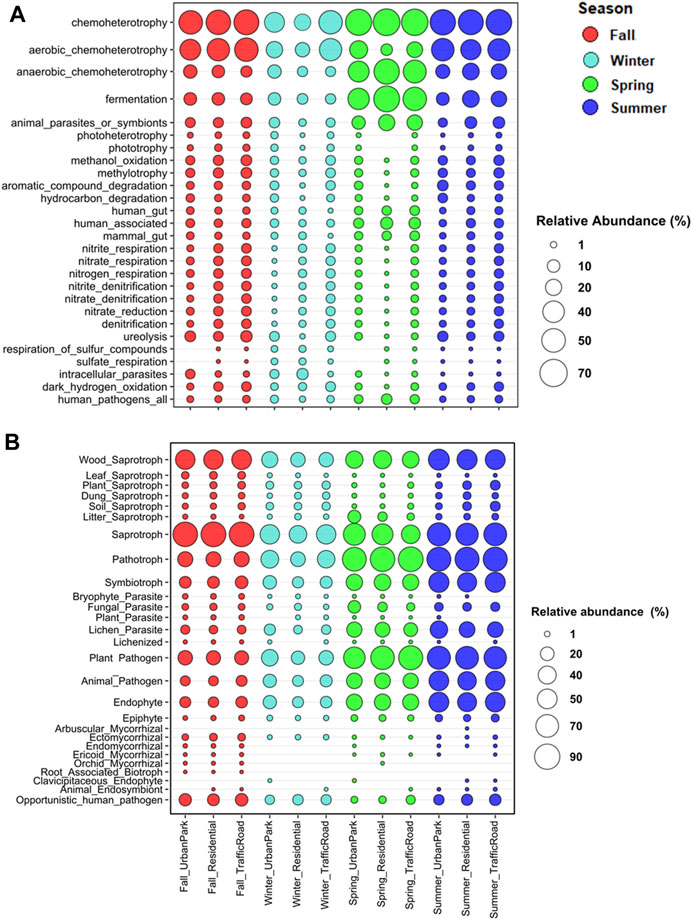
FIGURE 6. Relative abundance of ASVs (%) assigned to the top 27 functional groups in the four seasonal samples of airborne bacteria (A) and fungi (B) at three urban sites of Rome (Urban Park, Residential and Traffic Road) based on the FAPROTAX, FUNGuild, and FungalTraits databases.
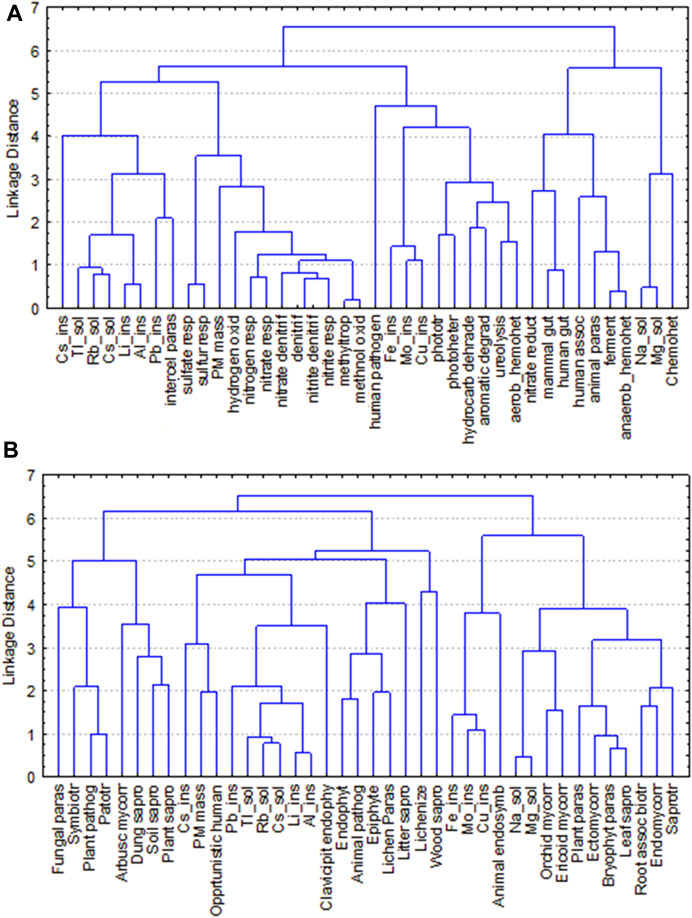
FIGURE 7. Hierarchical Cluster Analysis based on Euclidean distance and Complete linkage of the PM10 content, the chemical components used as pollution tracers, and the top 27 functional groups of airborne bacteria (A) and fungi (B) at three urban sites of Rome (Urban Park, Residential and Traffic Road) from Fall 2020 to Summer 2021.
Fungal communities were dominated by taxa of soil origin and to slightly less extent by fungi frequently associated to plants, with a clear seasonal trend (Figure 6B; Supplementary Tables S12–S13). The majority of the identified taxa were saprotrophs in fall (85.80% ± 1.02%) and in summer (70.79% ± 2.96%), as it is the case for many fungi found in soil. Especially frequent in this group were taxa related to wood decay in both seasons, fall (49.56% ± 0.85%) and summer (54.30% ± 3.49%). Many of the fungal taxa were also identified as plant pathogens and endophytes, especially in spring (pathogens: 73.39% ± 9.90%; endophytes: 31.09% ± 0.70%) and summer (pathogens:71.19% ± 2.40%; endophytes: 49.38% ± 2.83%), with similar frequency distributions across sampling sites (Figure 6B; Supplementary Table S12). Cluster analysis did not reveal any sharp agglomeration between the PM10 content, the chemical components used as pollution tracers, and the predominant ecological function profiles of fungi (Figure 7B).
Potentially human pathogens taxa
Although 70 ASVs (0.85%) was assigned to human-pathogens group (Figure 6A), the distribution of human-pathogenic bacteria is homogenous across seasons and in different urban sites (Supplementary Figure S8A, and Supplementary Tables S10, S11). Although potential entero-pathogens such as, Acinetobacter lwoffii, Alcaligenes faecalis, Salmonella enterica and Escherichia coli were mostly found in fall and winter, their presence was scattered into few samples and with low abundance. Conversely, a total of 95 opportunistic fungi from 59 genera belonging to 42 families, 23 orders, 13 classes and 4 divisions were found and classified as human-pathogenic fungi showing distinct seasonal and site-specific patterns (Figure 6B; Supplementary Figure S8B; Supplementary Tables S12, S13). The percentage of pathogenic fungi was higher in winter (11.00%–13.74%) than in spring (4.41%–7.23%), summer (4.46%–7.57%) and fall (2.52%–3.09%). Among them, members of the genera Alternaria (A. alternata, A. angustiovoidea, A. armoraciae, A. chlamydospore, A. metachromatica) showed the highest relative abundance across seasons, especially in summer (6.80%–7.88%) and spring (1.64%–4.98%), together with two Basidiomycota species, Bjerkandera adusta for winter (4.67%–1.44%), and Ceriporia lacerata for fall (12.02%–8.98%). Furthermore, the detection of positive significant Spearman’s rank correlation between the PM10 mass and the relative abundance of the human-pathogenic fungi indicated that human-pathogenic fungi tended to progressively increase in terms of fraction and relative abundance passing from the Urban Park to the most polluted site Traffic Road in fall, spring and summer (Figure 6B; Supplementary Figures S9, S10; Supplementary Tables S12, S13).
Discussion
Influence of seasonal features on airborne microbial communities
Seasonal shifts of airborne microbes have been detected in different urban environments of Eurasia (Ruiz-Gil et al., 2020). Innocente et al. (2017), and Romano et al. (2019) identified the meteorological conditions, physicochemical factors such as concentrations of particulate matter and chemical pollutants, and long-range transported air masses, as key factors affecting the diversity and dispersion of airborne microbial communities in the atmosphere across seasons in Southern and Northern Italy. However, a clear identification of the environmental factors that actually affect the structure of airborne communities is challenging, since their variations are often tightly related to seasonality. Disentangling the effects of each environmental factor is in fact difficult to perform. In agreement, our data confirmed that structure and composition of airborne microbial communities of Rome can be in part influenced by seasonal variation of temperature and precipitation, air masses origin as well as PM10 chemical compositional pattern.
Rome exhibits typical Mediterranean climate conditions, characterized by hot and dry summers, cold and dry winters, and demi-seasons with more rainfall. In agreement with Massimi et al. (2021), winter is the season of Rome with the highest concentrations of particular matter (PM10). The analysis of PM10 elemental components confirmed that the seasonal PM10 increment partially derived from biomass burning, vehicle exhaust and fuel combustion activities that can promote haze events. These anthropogenic sources, together with stationary atmospheric layers related to winter temperature decrease, lack of precipitation and high humidity, maintained high levels of pollutants in the urban macro areas of Rome (Innocente et al., 2017; Massimi et al., 2021). The winter period was also characterized by significantly lower species richness and diversity for airborne samples in comparison to warmer summer/early fall airs hosting more diverse bacterial and fungal communities. Du et al. (2018) observed that air pollution in hazy weather decreased the species richness and community diversity of bacteria in Beijing. Atmospheric temperature and precipitation can strongly influence the airborne seasonal communities across the urban Mediterranean areas (Genitsaris et al., 2017; Romano et al., 2019, Núñez et al., 2021). Similarly, to what had been detected for Northern Italy (Gandolfi et al., 2015), exposure to high levels of chemical pollutants at low temperatures might have affected the microbial growth and reduced the species diversity of microbial communities of Rome in winter. Indeed, high temperature can directly accelerate metabolic rates promoting the microbial proliferation (Zhou et al., 2016) and also contribute to physical detachment and dispersion of fungi (Jones and Harrison, 2004) and bacteria (Genitsaris et al., 2017) from soil surfaces in summer season. Likewise, precipitation can play a role altering the structure of the soil communities (Shi et al., 2020), promoting production of conidia or spore released by fungi (Jones and Harrison, 2004) and favoring the activity and survival of airborne bacterial concentrations, mostly in the rainy season such as early fall (Uetake et al., 2019).
Seasonal variations were mainly manifested as changes in the abundance of the most representative taxa, some of which are especially adapted to particular environmental conditions. Airborne communities in the atmosphere mainly comprised of Proteobacteria, Actinobateria, Firmicutes and Bacteroidetes as prokariotic phyla and as Ascomycota and Basidiomycota as eukaryotic phyla. In agreement with previous studies performed in Spain (Núñez et al., 2021) and Italy (Franzetti et al., 2011; Innocente et al., 2017; Romano et al., 2020), few taxa were dominant and served as seasonal indicators. In particular, gram-positive members of Actinobacteria which can be found in soil (Delgado-Baquerizo et al., 2018), were predominant indicators for the summer season. Summer samples of Rome were in fact enriched in Nocardiodes, Kocuria, and Blastococcus, usually resistant to high temperature, irradiation and dryness. Similarly, in this study three core fungal genera all assigned to Ascomycota, Mycosphaerella, Cladosporium and Alternaria, were mostly abundant in summer and correlated to high temperature. The highest concentrations of Alternaria and Cladosporium spores frequently occurred from June to September in many parts of Europe (Grinn-Gofron and Bosiacka, 2015), including the Mediterranean Basin (Sakiyan and Inceoglu, 2003). These data corroborated the theory that temperature has a strong effect on both fungal and bacterial composition of airborne samples during the warmest season of Rome (Núñez et al., 2021).
When we explored the community structure of PM10 winter samples, we noted that taxonomic composition of bacteria varied considerably at phylum level, with high relative abundance of Proteobacteria, Firmicutes, Bacteroidetes, and two seasonal-specific phyla, Thermotogae and Synergistetes, at each urban site. Previous studies demonstrated that Proteobacteria, Firmicutes and Bacteroidetes were prevalent in the airborne microbial communities sampled in cold periods of Italian Peninsula, except for Thermotogae and Synergistetes (Franzetti et al., 2011; Innocente et al., 2017; Romano et al., 2019). In particular, most of the Thermotogae species have optimal growth temperatures in the range of 45°C–80°C and great tolerance to NaCl (Lanzilli et al., 2020). Synergistetes have been described as member of dust-borne bacterial communities, extremely stress-resistant, isolated during a severe Saharan dust intrusion in the Iberian Peninsula (González-Toril et al., 2020). Two most abundant genera, Petrotoga and Acetomicrobium, belonging to Thermotogae and Synergistetes respectively, are capable to colonize a plenty of environmental niches due to its thermotolerance and osmotolerance. As already suggested by Pollegioni et al. (2022), such characteristics, typical of extremotolerant microbes, can contribute to microbial survival under conditions of dryness due to lack of precipitation in the typical harsh-polluted urban environments of Rome in winter. In this season, the persistence of three fungal genera, Aspergillum, and Penicillium and Trametes, which showed remarkable differences between heavy-haze and non-haze PM10 samples in Beijing (Yan et al., 2016), reinforced this view. Similar to Rome, the Chinese capital has been in fact suffering from frequent and heavy smog events caused mainly by exhaust emission in the cold periods. However, the back-trajectory pathway analysis also indicated that the Central Mediterranean basin was affected by an African dust outbreak from 24th to 27th February 2021. Federici et al. (2018) reported that airborne Saharan dust loaded a highly abundant and diverse bacterial community as well as persistent organic pollutants in Central Italy. In agreement with our findings in winter, samples collected during dust events had a significantly more diverse microbiome than samples collected in dust-free days, with a predominance of Proteobacteria, Firmicutes, and Bacteroidetes. In addition, a progressive increment of sulfates driven by seasonal evolution from winter to spring were often associated to mineral dust transport events in Rome (Massimi et al., 2021). Considering the highest relative abundance of S-cycling and dust-carried bacteria in the winter communities, as well as the presence of bacterial species typical of deserts such as Synergistetes, we cannot rule out that dust from African deserts represented an important long-distance particle emission source for Rome in late winter. Overall, we can hypothesize the coexistence of a highly diverse microbial community resistant to high concentrations of organic pollutants in winter, with aerosols from Sahara acting as a vector for introducing soil-associated microbes into local seasonal-specific communities. Finally, it's worth noticing that, coupled with the most prevalence of soil-inhabiting bacteria and fungi, a marked increment of insoluble fractions of allumium (Al_Ins), rubidium (Rb_Ins), lithium (Li_Ins), cesium (Cs_Ins), tallium (Tl_Ins) and titanium (Ti_Ins) has been also recorded in two different seasons, winter and summer. These elements are considered reliable tracers for soil resuspension, which can be favored, as in this specific case, by the passage of vehicles, particularly during warm and/or dry periods (Soleimanian et al., 2019). As proved by Pollegioni et al. (2022), road dust can be a primary local source of out-door air bacteria and fungi in urban environments of Rome. These results suggest that the lack of precipitation and the subsequent resuspension of dust produced by vehicular traffic might have further contributed to the maximum abundance of soil-associated microbes in winter and summer and induced changes to the microbial community structure.
Pollutant factors influencing airborne bacterial communities
In the current study, we observed a consistent decline of airborne microbial richness passing from less (fall, summer and spring) to most polluted season (winter). On the contrary, an overall correlation between three species diversity indices and air pollution levels of urban sites hasn’t been inferred. A significant positive correlation between PM10 and the concentration of total microbes has been verified in the Eurasian continent (Ruiz-Gil et al., 2020). The coarse particles were reported to act as carriers or refuges and provide energy sources for the microbes attached to the particles (Smets et al., 2016). As observed in Pollegioni et al. (2022), the resuspension or deposition of road dust favored by heavy traffic loads accelerates the movement of convective air and further increased the airborne microbial diversity in Residential and Traffic Road areas of Rome. Therefore, the variation in microbial concentration across urban macro areas was determined by the influence of two contrasting factors. We can postulate that the resuspension of the most wind-dispersible soil particles rich in microorganisms might have served as important sources of airborne bacteria and fungi, and balanced the adverse impacts of high level of chemical pollutants on microbial growth at the most polluted urban site of Rome at each season. However, despite the lack of a correlation between seasonal alpha diversity and PM10 levels, a trend of gradual increase in terms of relative abundance of few bacterial taxa has been observed, passing from green to polluted or residential areas. In particular, functional annotation revealed that the relative abundance of Paracoccus sp. and bacteria involved in N-cycles and dark hydrogen oxidation gradually increased from Urban Park to Traffic Road passing through Residential zone during each season, with the highest fraction occurring in winter. Notably, Paracoccus (e.g., P. contaminans), which has been often identified as a key taxon in airborne bacterial communities from winter samples (Yan et al., 2018), is one of the most represented genera of hydrogen-oxidizing bacteria and strongly associated with denitrification processes (Lin et al., 2022). Nitrogen in various forms is one of the primary contributors to the formation of particulate matter (e.g., anions such as NO3−) that is transported by particles and can cause significant cardiovascular effects and oxidative stress in humans (Ghio et al., 2012; Alessandria et al., 2014; Rezaei et al., 2014). Our data indicated that members of Paracoccus may benefit from nitrogen compounds present in airborne particulate matter and use nitrate as a terminal electron acceptor in anaerobic respiration to drive the oxidation of organic compounds under extremotolerant conditions (Gong et al., 2020). Irrespective of seasonality, we can assume that PM10 levels associated with dust road resuspension and traffic activities promotes an increase of the extremotolerant and pollutant removal-hydrogen oxidation bacteria in the most polluted urban site of Rome.
Potential health threats
Daily, a human individual inhales approximately 11,000 L of the air. Since the species richness of airborne microbial community is high, comparable to those measured in the soil (Gao et al., 2018), our immune system is constantly exposed to this great biological diversity. Pathogenic and opportunistic microorganisms make part of airborne community and airborne transmission is highlighted as a possible infection root (Casadevall and Pirofski, 2007; Jones and Brosseau, 2015; Wang et al., 2021). In order to be able to control the spread of airbone-trasmitted diseases, it is crucial to get into the factors which affect the distribution and persistence in the air of related pathogens.
In this study, relative abundance of pathogenic bacteria in Rome air varied between 1.0% to 6.3% which is in the range reported for Hangzhou (China) for the air of good and moderate quality (range approx. 2%–6% Liu et al., 2018) and for different functional zones of Xiamen (China) (range 3.03%–6.77%, Li et al., 2021). Both studies report an increase in the abundance of bacterial pathogens with worsening of the air quality in time (Liu et al., 2018) and space (Li et al., 2021). For Rome, such observation cannot be confirmed. Indeed, abundance of bacteria pathogens in Madrid (Spain) over 2 years of periodic measurements accounted on average to 12% - almost 5 times higher than the observed average abundance in Rome (2.5%) (Núñez et al., 2021). While average annual temperatures between Madrid and Rome are similar, Madrid is characterized by much drier climate. So, although the air quality in the period of sampling in Madrid was good and never reached values measured for winter in Rome, harsh environmental conditions might favor extremotolerant features. Similarly, pathogenic and opportunistic traits allow such microorganisms to cope better with the adverse environment than normal microflora explaining the Madrid pattern (Gostinčar et al., 2018; Pollegioni et al., 2022). Vicinity and density of such local sources of pathogenic bacteria as farming sector and waste treatment plants should also be considered alongside climatic and environmental variables (Lai et al., 2009; Gao et al., 2018; Song et al., 2021).
Finally, a total of 95 opportunistic fungi were found in Rome with combined relative abundance of 9.8%. This value is higher than what has been identified in similar studies in Chinese cities where pathogens accounted to 12–17 species (Li et al., 2021; Jiang et al., 2022; Nie et al., 2023) with relative abundance varying in the range of 0.99–1.31 (Li et al., 2021). Yet, methodological differences in accounting related for example to a pool size (most abundant pool vs. all identified species) or databases used should be considered. In Lecce (Italy), 7 potentially pathogenic genera were detected among the most representative taxa (Fragola et al., 2021). In this study, the inferred human-pathogenic fungi showed in part a seasonal trend and tended to progressively increased in terms of fraction and relative abundance passing from the Urban Park to the Traffic Road zone in fall, spring and summer. In spring and summer, Alternaria infectoria (Ascomycota) dominated in all urban sampling sites. This species is a saprotroph capable of causing skin infections in immunocompromised patients (Schuermans et al., 2017). The dominance of Alternaria genus is also inferred in the air of Tianjin (China) from April to July (Nageen et al., 2023), as well as in summer in France (Tignat-Perrier et al., 2020). In autumn, Ceriporia lacerate, causing bronchopulmonary infection in patients with concomitant chronic lung diseases, dominated in all functional areas (Singh et al., 2013). During this season, the highest relative abundance of opportunistic species in relation to other groups (14%–16%) was noted, which is also reported by other authors (Núñez et al., 2021; Jiang et al., 2022). However, as observed in Beijing (Yan et al., 2016), the highest percentage of fungal pathogens were found in winter with peak value at the Traffic Road site. Bjerkandera adusta dominated among all opportunistic fungi in our urban sites. B. adusta has been implicated as an agent responsible for chronic cough as well as bronchial asthma (Chowdhary et al., 2013). In addition, consistent with a previous study (Pollegioni et al., 2022), our data suggested that the opportunistic-pathogenic fungi increased with PM10 levels of urban sites at each season in Rome. To infect the human body, opportunistic and pathogenic fungi should be capable to overcome multiple barriers such as elevated body temperature, unfavorable pH and humidity, epithelial and mucus obstacles. The opportunist fungi are not specialized for infection of mammals but their acquired adaptability and stress tolerance allow the establishment in the host (Pollegioni et al., 2022). Our data confirmed that such characteristics can contribute to microbial survival in harsh-polluted urban environments of Rome, ensuring the possibility of opportunism.
Conclusion
In the present study, we identified large changes in airborne microbial community compositions across four seasons and their close relationship with specific atmospheric factors, such as temperature and precipitation, local sources, long-distance air mass and pollution degree of three urban sites in Rome. Our data revealed that the lack of precipitation and the subsequent resuspension of dust produced by vehicular traffic might contribute to the maximum abundance of soil-associated microbes in winter and summer. However, the increase of PM10 concentrations favoured also by climatic conditions, domestic heating and dust advection event from African desert further shaped the community structure of winter. In addition, our results highlight a close interrelationship between PM10 content and abundance of certain predicted functional bacterial and fungal groups also with potential implications for human health. Across three seasons, the pollutant removal-hydrogen oxidation bacteria and the opportunist-human pathogenic fungi progressively increased with pollution levels, in the sequence from green to residential and/or polluted area close to the traffic roads, with highest fraction during winter.
Data availability statement
The datasets presented in this study can be found in online repositories. The names of the repository/repositories and accession number(s) can be found below: www.ncbi.nlm.nih.gov/bioproject/, PRJNA962806.
Author contributions
Conceptualization, PP, SC (2nd author), CM, and OG; methodology, PP, SC (2nd author), CM, RP, MR, SC (7th author), DO, MK, AS, and OG; software, PP, SC (2nd author), and RP; data curation, PP, SC (2nd author), CM, RP, MK, AS, and OG; investigation, PP, SC (2nd author), CM, MR, DO, and OG; resources, CC and OG; writing-original draft preparation, PP, SC (2nd author), CM, RP, MK, and OG; writing-review and editing, PP, SC (2nd author), CM, RP, MR, SC (7th author), DO, MK, AS, CC, and OG; project administration, OG; funding acquisition, CC and OG. All authors contributed to the article and approved the submitted version.
Funding
This research was funded from 2020 to 2021 by Russian Foundation for Basic Research (RFBR), project number 19-05-50112. Chemical characterization of PM was supported by the project PRIN 20173RRN2S: EUFORICC. Analysis of fungal pathogens was supported by the RUDN University Scientific Projects Grant System.
Acknowledgments
The authors warmly thank Stefano Listrani and Manuela Riva of Agenzia Regionale Protezione Ambiente del Lazio, Rome, (Italy) for valuable support in collecting environmental data and Michele Mattioni for his help with instrumentation set-up.
Conflict of interest
The authors declare that the research was conducted in the absence of any commercial or financial relationships that could be construed as a potential conflict of interest.
Publisher’s note
All claims expressed in this article are solely those of the authors and do not necessarily represent those of their affiliated organizations, or those of the publisher, the editors and the reviewers. Any product that may be evaluated in this article, or claim that may be made by its manufacturer, is not guaranteed or endorsed by the publisher.
Supplementary material
The Supplementary Material for this article can be found online at: https://www.frontiersin.org/articles/10.3389/fenvs.2023.1213833/full#supplementary-material
References
Alessandria, L., Schilirò, T., Degan, R., Traversi, D., and Gilli, G. (2014). Cytotoxic response in human lung epithelial cells and ion characteristics of urban-air particles from Torino, a northern Italian city. Environ. Sci. Pollut. Res. 21, 5554–5564. doi:10.1007/s11356-013-2468-1
Bowers, R. M., Mcletchie, S., Knight, R., and Fierer, N. (2011). Spatial variability in airborne bacterial communities across land-use types and their relationship to the bacterial communities of potential source environments. ISME J. 5, 601–612. doi:10.1038/ismej.2010.167
Cáliz, J., Triadó-Margarit, X., Camarero, L., and Casamayor, E. O. (2018). A long-term survey unveils strong seasonal patterns in the airborne microbiome coupled to general and regional atmospheric circulations. Proc. Natl. Acad. Sci. U S A 115, 12229–12234. doi:10.1073/pnas.1812826115
Callahan, B. J., McMurdie, P. J., Rosen, M. J., Han, A. W., Johnson, A. J. A., and Holmes, S. P. (2016). DADA2: High-resolution sample inference from Illumina amplicon data. Nat. Methods 13, 581–583. doi:10.1038/nmeth.3869
Canepari, S., Cardarelli, E., Giuliano, A., and Pietrodangelo, A. (2006a). Determination of metals, metalloids and non-volatile ions in airborne particulate matter by a new two-step sequential leaching procedurePart A: Experimental design and optimisation. Talanta 69, 581–587. doi:10.1016/j.talanta.2005.10.023
Canepari, S., Cardarelli, E., Pietrodangelo, A., and Strincone, M. (2006b). Determination of metals.; metalloids and non-volatile ions in airborne particulate matter by a new two-step sequential leaching procedure: Part B: Validation on equivalent real samples. Talanta 69, 588–595. doi:10.1016/j.talanta.2005.10.024
Cao, C., Jiang, W., Wang, B., Fang, J., Lang, J., Tian, G., et al. (2014). Inhalable microorganisms in Beijing’s PM2.5and PM10 Pollutants during a severe smog event. Environ. Sci. Technol. 48, 1499–1507. doi:10.1021/es4048472
Casadevall, A., and Pirofski, L. A. (2007). Accidental virulence, cryptic pathogenesis, Martians, lost hosts, and the pathogenicity of envi-ronmental microbes. Eukaryot. Cell 6, 2169–2174. doi:10.1128/ec.00308-07
Chavent, M., Simonet, V. K., Liquet, B., and Kuentz-simonet, V. (2012). ClustOfVar: An R package for the clustering of variables. J. Stat. 50, 1–16. doi:10.18637/jss.v050.i13
Chen, X., Kumari, D., and Achal, V. (2020). A review on airborne microbes: The characteristics of sources, pathogenicity and geography. Atmosphere 11, 919. doi:10.3390/atmos11090919
Chowdhary, A., Agarwal, K., Kathuria, S., Singh, P. K., Roy, P., Gaur, S. N., et al. (2013). Clinical significance of filamentous basidiomycetes illustrated by isolates of the novel opportunist Ceriporia lacerata from the human respiratory tract. J. Clin. Microbiol. 51 (2), 585–590. doi:10.1128/JCM.02943-12
de Hoog, G. S., Guarro, J., Gené, J., Ahmed, S. A., Al-Hatmi, A. M. S., Figueras, M. J., et al. (2020). “Atlas of clinical fungi,” in Foundation Atlas of Clinical Fungi, Hilversum. 4th Edn.
de Mendiburu, F. (2021). Agricolae: Statistical procedures for agricultural research. rpackage version 1.3-5. 155. Available at: https://CRAN.R-project.org/package=agricolae.
Delgado-Baquerizo, M., Oliverio, A. M., Brewer, T. E., Benavent-Gonzalez, A., Eldridge, D. J., Bardgett, R. D., et al. (2018). A global atlas of the dominant bacteria found in soil. Sci 359, 320–325. doi:10.1126/science.aap9516
Dommergue, A., Amato, P., Tignat-Perrier, R., Magand, O., Thollot, A., Joly, M., et al. (2019). Methods to investigate the global atmospheric microbiome. Front. Microbio.l 10, 243. doi:10.3389/fmicb.2019.00243
Du, P., Du, R., Ren, W., Lu, Z., Zhang, Y., and Fu, P. (2018). Variations of bacteria and fungi in PM2.5 in Beijing, China. Atmos. Environ 172, 55–64. doi:10.1016/j.atmosenv.2017.10.048
Federici, E., Petroselli, C., Ceci, E., Franzetti, A., Casagrande, C., Selvaggi, R., et al. (2018). Airborne bacteria and persistent organic pollutants associated with an intense Saharan dust event in the Central Mediterranean. Sci. Total Environ. 645, 401–410. doi:10.1016/j.scitotenv.2018.07.128
Ferguson, R. M. W., Garcia-Alcega, S., Coulon, F., Dumbrell, A. J., Whitby, C., and Colbeck, I. (2019). Bioaerosol biomonitoring: Sampling optimization for molecular microbial ecology. Mol. Eco.l Resour. 19, 672–690. doi:10.1111/1755-0998.13002
Fragola, M., Perrone, M. R., Alifano, P., Talà, A., and Romano, S. (2021). Seasonal variability of the airborne eukaryotic community structure at a coastal site of the central mediterranean. Toxins 13 (8), 518. doi:10.3390/toxins13080518
Francis, R. M. (2017). pophelper: an R package and web app to analyse and visualize population structure. Mol. Ecol. Resour. 17 (1), 27–32. doi:10.1111/1755-0998.12509
Franzetti, A., Gandolfi, I., Gaspari, E., Ambrosini, R., and Bestetti, G. (2011). Seasonal variability of bacteria in fine and coarse urban air particulate matter. Appl. Microbiol. Biotechnol. 90, 745–753. doi:10.1007/s00253-010-3048-7
Fröhlich-Nowoisky, J., Kampf, C. J., Weber, B., Huffman, J. A., Pöhlker, C., Andreae, M. O., et al. (2016). Bioaerosols in the earth system: Climate, health, and ecosystem interactions. Atmos. Res. 182, 346–376. doi:10.1016/j.atmosres.2016.07.018
Gandolfi, I., Bertolini, V., Bestetti, G., Ambrosini, R., Innocente, E., Rampazzo, G., et al. (2015). Spatio-temporal variability of airborne bacterial communities and their correlation with particulate matter chemical composition across two urban areas. Appl. Microbiol. Biotechnol. 9911, 4867–4877. doi:10.1007/s00253-014-6348-5
Gao, M., Qiu, T., Sun, Y., and Wang, X. (2018). The abundance and diversity of antibiotic resistance genes in the atmospheric environment of composting plants. Environ. Int. 116, 229–238. doi:10.1016/j.envint.2018.04.028
Gat, D., Mazar, Y., Cytryn, E., and Rudich, Y. (2017). Origin-dependent variations in the atmospheric microbiome community in eastern mediterranean dust storms. Environ. Sci. Technol. 51, 6709–6718. doi:10.1021/acs.est.7b00362
Genitsaris, S., Stefanidou, N., Katsiapi, M., Kormas, K. A., Sommer, U., and Moustaka-Gouni, M. (2017). Variability of airborne bacteria in an urban Mediterranean area (Thessaloniki, Greece). Atmos. Environ. 157, 101–110. doi:10.1016/j.atmosenv.2017.03.018
Ghio, A. J., Carraway, M. S., and Madden, M. C. (2012). Composition of air pollution particles and oxidative stress in cells, tissues, and living systems. J. Toxicol. Environ. Health, Part B 15, 1e21–21. doi:10.1080/10937404.2012.632359
Gong, J., Qi, J., Beibei, . E., Yin, Y., and Gao, D. (2020). Concentration, viability and size distribution of bacteria in atmospheric bioaerosols under different types of pollution. Environ. Pollut. 257, 113485. doi:10.1016/j.envpol.2019.113485
González-Toril, E., Osuna, S., Viúdez-Moreiras, D., Navarro-Cid, I., Toro, S. D., Sor, S., et al. (2020). Impacts of saharan dust intrusions on bacterial communities of the low troposphere. Sci. Rep. 10, 6837. doi:10.1038/s41598-020-63797-9
Gostinčar, C., Zajc, J., Lenassi, M., Plemenitaš, A., De Hoog, S., Al-Hatmi, A. M., et al. (2018). Fungi between extremotolerance and opportunistic pathogenicity on humans. Fungal Divers. 93, 195–213. doi:10.1007/s13225-018-0414-8
Grinn-Gofron, A., and Bosiacka, B. (2015). Effects of meteorological factors on the composition of selected fungal spores in the air. Aerobiol. (Bologna) 31, 63–72. doi:10.1007/s10453-014-9347-1
Innocente, E., Squizzato, S., Visin, F., Facca, C., Rampazzo, G., Bertolini, V., et al. (2017). Influence of seasonality, air mass origin and particulate matter chemical composition on airborne bacterial community structure in the Po Valley, Italy. Sci. Total Environ. 593-594, 677–687. doi:10.1016/j.scitotenv.2017.03.199
Jiang, S., Sun, B., Zhu, R., Che, C., Ma, D., Wang, R., et al. (2022). Airborne microbial community structure and potential pathogen identification across the PM size fractions and seasons in the urban atmosphere. Sci. Total Environ. 831, 154665. doi:10.1016/j.scitotenv.2022.154665
Jones, R. M., and Brosseau, L. M. (2015). Aerosol transmission of infectious disease. JOEM 57 (5), 501–508. doi:10.1097/JOM.0000000000000448
Jones, A. M., and Harrison, R. M. (2004). The effects of meteorological factors on atmospheric bioaerosol concentrations—A review. Sci. Total Environ. 326, 151–180. doi:10.1016/j.scitotenv.2003.11.021
Kassambara, A. (2019). Ggcorrplot: Visualization of a correlation matrix using ‘ggplot2. R. Package Version 1, 3.
Klindworth, A., Pruesse, E., Schweer, T., Peplies, J., Quast, C., Horn, M., et al. (2013). Evaluation of general 16S ribosomal RNA gene PCR primers for classical and next generation sequencing-based diversity studies. Nucleic. Acids Res. 41, e1. doi:10.1093/nar/gks808
Kõljalg, U., Nilsson, R. H., Abarenkov, K., Tedersoo, L., Taylor, A. F. S., Bahram, M., et al. (2013). Towards a unified paradigm for sequence-based identification of fungi. Mol. Ecol. 22, 5271–5277. doi:10.1111/mec.12481
Lai, K. M., Emberlin, J., and Colbeck, I. (2009). Outdoor environments and human pathogens in air. Environ. Health 8 (1), S15. doi:10.1186/1476-069X-8-S1-S15
Lanzilli, M., Esercizio, N., Vastano, M., Xu, Z., Nuzzo, G., Gallo, C., et al. (2020). Effect of cultivation parameters on fermentation and hydrogen production in the phylum Thermotogae. Int. J. Mol. Sci. 22 (1), 341. doi:10.3390/ijms22010341
Li, H., Wu, Z. F., Yang, X. R., An, X. L., Ren, Y., and Su, J. Q. (2021). Urban greenness and plant species are key factors in shaping air microbiomes and reducing airborne pathogens. Environ. Int. 153, 106539. doi:10.1016/j.envint.2021.106539
Lin, L., Huang, H., Zhang, X., Dong, L., and Chen, Y. (2022). Hydrogen-oxidizing bacteria and their applications in resource recovery and pollutant removal. Sci. Total Environ. 835, 155559. doi:10.1016/j.scitotenv.2022.155559
Liu, H., Zhang, X., Zhang, H., Yao, X., Zhou, M., Wang, J., et al. (2018). Effect of air pollution on the total bacteria and pathogenic bacteria in different sizes of particulate matter. Environ. Pollut. 233, 483–493. doi:10.1016/j.envpol.2017.10.070
Liu, H., Hu, Z., Zhou, M., Hu, J., Yao, X., Zhang, H., et al. (2019). The distribution variance of airborne microorganisms in urban and rural environments. Environ. Pollut. 247, 898–906. doi:10.1016/j.envpol.2019.01.090
Liu, C., Cui, Y., Li, X., and Yao, M. (2021). Microeco: An R package for data mining in microbial community ecology. FEMS Microbiol. Ecol. 97, fiaa255. doi:10.1093/femsec/fiaa255
Maki, T., Lee, K. C., Kawai, K., Onishi, K., Hong, C. S., Kurosaki, Y., et al. (2019). Aeolian dispersal of bacteria associated with desert dust and anthropogenic particles over continental and oceanic surfaces. J. Geophys. Res. Atmos. 124, 5579–5588. doi:10.1029/2018JD029597
Massimi, L., Ristorini, M., Astolfi, M. L., Perrino, C., and Canepari, S. (2020). High resolution spatial mapping of element concentrations in PM10: A powerful tool for localization of emission sources. Atmos. Res. 244, 105060. doi:10.1016/j.atmosres.2020.105060
Massimi, L., Pietrodangelo, A., Frezzini, M. A., Ristorini, M., De Francesco, N., Sargolini, T., et al. (2021). Effects of COVID-19 lockdown on PM10 composition and sources in the Rome Area (Italy) by elements' chemical fractionation-based source apportionment. Atmos. Res. 266, 105970. doi:10.1016/j.atmosres.2021.105970
McMurdie, P. J., and Holmes, S. (2013). phyloseq: an R package for reproducible interactive analysis and graphics of microbiome census data. PLoS ONE 8, e61217. doi:10.1371/journal.pone.0061217
Moelling, K., and Broecker, F. (2020). Air microbiome and pollution: Composition and potential effects on human health, including SARS coronavirus infection. J. Environ. Public. Health 2020, 1646943. doi:10.1155/2020/1646943
Morris, C. E., Conen, F., Alex Huffman, J., Phillips, V., P¨oschl, U., and Sands, D. C. (2014). Bioprecipitation: A feedback cycle linking earth history, ecosystem dynamics and land use through biological ice nucleators in the atmosphere. Glob. Change Biol. 20, 341–351. doi:10.1111/gcb.12447
Nageen, Y., Wang, X., and Pecoraro, L. (2023). Seasonal variation of airborne fungal diversity and community structure in urban outdoor environments in Tianjin, China. Front. Microbiol. 13, 1043224. doi:10.3389/fmicb.2022.1043224
Namgung, H. G., Kim, J. B., Woo, S. H., Park, S., Kim, M., Kim, M. S., et al. (2016). Generation of nanoparticles from friction between railway brake disks and pads. Environ. Sci. Technol. 50, 3453–3461. doi:10.1021/acs.est.5b06252
Nguyen, N. H., Song, Z., Bates, S. T., Branco, S., Tedersoo, L., Menke, J., et al. (2016). FUNGuild: An open annotation tool for parsing fungal community datasets by ecological guild. Fungal Ecol. 20 (1), 241–248. doi:10.1016/j.funeco.2015.06.006
Nie, C., Geng, X., Ouyang, H., Wang, L., Li, Z., Wang, M., et al. (2023). Abundant bacteria and fungi attached to airborne particulates in vegetable plastic greenhouses. Sci. Total Environ. 857, 159507. doi:10.1016/j.scitotenv.2022.159507
Núñez, A., García, A. M., Moreno, D. A., and Guantes, R. (2021). Seasonal changes dominate long-term variability of the urban air microbiome across space and time. Environ. Int. 150, 106423. doi:10.1016/j.envint.2021.106423
Oksanen, J., Blanchet, F. G., Kindt, R., Legendre, P., Minchin, P. R., O’Hara, R. B., et al. (2015) Vegan community ecology package: Ordination methods, diversity analysis and other functions for community and vegetation ecologists. R package ver, 2–3.
Padoan, E., Malandrino, M., Giacomino, A., Grosa, M. M., Lollobrigida, F., Martini, S., et al. (2016). Spatial distribution and potential sources of trace elements in PM10 monitored in urban and rural sites of Piedmont Region. Chemosphere 145, 495–507. doi:10.1016/j.chemosphere.2015.11.094
Pollegioni, P., Mattioni, C., Ristorini, M., Occhiuto, D., Canepari, S., Korneykova, M. V., et al. (2022). Diversity and source of airborne microbial communities at differential polluted sites of rome. Atm 13 (2), 224. doi:10.3390/atmos13020224
Põlme, S., Abarenkov, K., Nilsson, R. H., Lindahl, B. D., Clemmensen, K. E., Kauserud, H., et al. (2020). FungalTraits: A user-friendly traits database of fungi and fungus-like stramenopiles. Fungal Divers. 105 (1), 1–16. doi:10.1007/s13225-020-00466-2
Rezaei, M., Salimi, A., Taghidust, M., Naserzadeh, P., Goudarzi, G., Seydi, E., et al. (2014). A comparison of toxicity mechanisms of dust storm particles collected in the southwest of Iran on lung and skin using isolated mitochondria. Toxicol. Environ. Chem. 96, 814–830. doi:10.1080/02772248.2014.959317
Romano, S., Di Salvo, M., Rispoli, G., Alifano, P., Perrone, M. R., and Talà, A. (2019). Airborne bacteria in the Central Mediterranean: Structure and role of meteorology and air mass transport. Sci. Total Environ. 697, 134020. doi:10.1016/j.scitotenv.2019.134020
Romano, S., Becagli, S., Lucarelli, F., Rispoli, G., and Perrone, M. R. (2020). Airborne bacteria structure and chemical composition relationships in winter and spring PM10 samples over southeastern Italy. Sci. Total Environ. 15, 138899. doi:10.1016/j.scitotenv.2020.138899
Ruiz-Gil, T., Acuña, J. J., Fujiyoshi, S., Tanaka, D., Noda, J., Maruyama, F., et al. (2020). Airborne bacterial communities of outdoor environments and their associated influencing factors. Environ. Int., 106156. doi:10.1016/j.envint.2020.106156
Sakiyan, N., and Inceoglu, Ö. (2003). Atmospheric concentrations of cladosporium link and Alternaria nees spores in ankara and the effects of meteorological factors. Turk J. Bot. 27, 77–81.
Schuermans, W., Hoet, K., Stessens, L., Meeuwissen, J., Vandepitte, A., Van Mieghem, A., et al. (2017). Molecular identification of cutaneous alternariosis in a renal transplant patient. Mycopathologia 182, 873–877. doi:10.1007/s11046-017-0166-0
Shi, Y., Zhang, K., Li, Q., Liu, X., He, J.-S., and Chu, H. (2020). Interannual climate variability and altered precipitation influence the soil microbial community structure in a Tibetan Plateau grassland. Sci. Total Environ. 714, 136794. doi:10.1016/j.scitotenv.2020.136794
Singh, P. K., Kathuria, S., Agarwal, K., Gaur, S. N., Meis, J. F., and Chowdhary, A. (2013). Clinical significance and molecular characterization of nonsporulating molds isolated from the respiratory tracts of bronchopulmonary mycosis patients with special reference to basidiomycetes. J. Clin. Microbiol. 51 (10), 3331–3337. doi:10.1128/JCM.01486-13
Smets, W., Moretti, S., Denys, S., and Lebeer, S. (2016). Airborne bacteria in the atmosphere: Presence, purpose, and potential. Atmos. Environ. 139, 214–221. doi:10.1016/j.atmosenv.2016.05.038
Soleimanian, E., Taghvaee, S., Mousavi, A., Sowlat, M. H., Hassanvand, M. S., Yunesian, M., et al. (2019). Sources and temporal variations of coarse particulate matter (PM) in central tehran, Iran. Atmosphere 10, 291. doi:10.3390/atmos10050291
Song, L., Wang, C., Jiang, G., Ma, J., Li, Y., Chen, H., et al. (2021). Bioaerosol is an important transmission route of antibiotic resistance genes in pig farms. Environ. Int. 154, 106559. doi:10.1016/j.envint.2021.106559
Stewart, J. D., Shakya, K. M., Bilinski, T., Wilson, J. W., Ravi, S., and Choi, C. S. (2020). Variation of near surface atmosphere microbial communities at an urban and a suburban site in Philadelphia, PA, USA. Sci. Total Environ. 724, 138353. doi:10.1016/j.scitotenv.2020.138353
Tignat-Perrier, R., Dommergue, A., Thollot, A., Magand, O., Amato, P., Joly, M., et al. (2020). Seasonal shift in airborne microbial communities. Sci. Total Environ. 716, 137129. doi:10.1016/j.scitotenv.2020.137129
Uetake, J., Tobo, Y., Uji, Y., Hill, T. C. J., DeMott, P. J., Kreidenweis, S. M., et al. (2019). Seasonal changes of airborne bacterial communities over Tokyo and influence of local meteorology. Front. Microbiol. 10, 1572. doi:10.3389/fmicb.2019.01572
Vu, V. Q. (2011). ggbiplot: A ggplot2 based biplot. R package version 0.55. 2011. Available at: https://github.com/vqv/ggbiplot.
Wang, Q., Garrity, G. M., Tiedje, J. M., and Cole, J. R. (2007). Naive Bayesian classifier for rapid assignment of rRNA sequences into the new bacterial taxonomy. Appl. Environ. Microbiol. 73, 5261–5267. doi:10.1128/AEM.00062-07
Wang, C. C., Prather, K. A., Sznitman, J., Jimenez, J. L., Lakdawala, S. S., Tufekci, Z., et al. (2021). Airborne transmission of respiratory viruses. Science 373 (6558), eabd9149. doi:10.1126/science.abd9149
Werner, M., Kryza, M., and Dore, A. J. (2014). Differences in the spatial distribution and chemical composition of PM10 between the UK and Poland. Model. Assess. 19, 179–192. doi:10.1007/s10666-013-9384-0
White, J. R., Maddox, C., White, O., Angiuoli, S. V., and Fricke, W. F. (2013). CloVR-ITS: Automated internal transcribed spacer amplicon sequence analysis pipeline for the characterization of fungal microbiota. Microbiome 5, 6–11. doi:10.1186/2049-2618-1-6
Yan, D., Zhang, T., Su, J., Zhao, L. L., Wang, H., Fang, X. M., et al. (2016). Diversity and composition of airborne fungal community associated with particulate matters in Beijing during haze and non-haze days. Front. Microbiol. 7, 487. doi:10.3389/fmicb.2016.00487
Yan, D., Zhang, T., Su, J., Zhao, L.-L., Wang, H., Fang, X.-M., et al. (2018). Structural variation in the bacterial community associated with airborne particulate matter in Beijing, China, during hazy and nonhazy days. Appl. Environ. Microbiol. 84, e00004–e18. doi:10.1128/AEM.00004-18
Keywords: particulate matter, seasonality, airborne, pollution, metabarcoding, urban area, pathogenicity
Citation: Pollegioni P, Cardoni S, Mattioni C, Piredda R, Ristorini M, Occhiuto D, Canepari S, Korneykova MV, Soshina AS, Calfapietra C and Gavrichkova O (2023) Variability of airborne microbiome at different urban sites across seasons: a case study in Rome. Front. Environ. Sci. 11:1213833. doi: 10.3389/fenvs.2023.1213833
Received: 28 April 2023; Accepted: 31 August 2023;
Published: 13 September 2023.
Edited by:
Ioar Rivas, Instituto Salud Global Barcelona (ISGlobal), SpainReviewed by:
Linnea Katherine Honeker, University of Arizona, United StatesXianhua Liu, Tianjin University, China
Copyright © 2023 Pollegioni, Cardoni, Mattioni, Piredda, Ristorini, Occhiuto, Canepari, Korneykova, Soshina, Calfapietra and Gavrichkova. This is an open-access article distributed under the terms of the Creative Commons Attribution License (CC BY). The use, distribution or reproduction in other forums is permitted, provided the original author(s) and the copyright owner(s) are credited and that the original publication in this journal is cited, in accordance with accepted academic practice. No use, distribution or reproduction is permitted which does not comply with these terms.
*Correspondence: Paola Pollegioni, paola.pollegioni@cnr.it; Claudia Mattioni, claudia.mattioni@cnr.it; Olga Gavrichkova, olga.gavrichkova@cnr.it
†These authors have contributed equally to this work and share first authorship