- Accounting School, Harbin University of Commerce, Harbin, China
With the increasing concern of all sectors of society about climate change, enterprises have become an important subject to undertake environmental protection. At the same time, enterprises have to seek survival and development under the background of economic policy uncertainty. Therefore, companies need to not only fulfill their environmental responsibilities but also find ways to respond to changes in economic policies through innovation. In the study, a three-variable time-varying vector autoregressive (TVP-VAR) model with stochastic volatility was used to investigate the nonlinear dynamic effects of economic policy uncertainty and low-carbon economic transition on enterprise innovation in China. It is found that the influence of economic policy uncertainty on enterprise innovation is time-varying and asymmetrical, especially with the 2008 financial crisis as the turning point. After the financial crisis, the positive impact of economic policy uncertainty on enterprises has been weakening, and in 2015, the positive impact turned into a negative impact. In general, economic policy uncertainty is not conducive to the transition to low-carbon economic transition, and with the outbreak of the financial crisis in 2008, the negative impact reached a negative peak. The low-carbon economic transition has a significant crowding-out effect on enterprise innovation, while enterprise innovation has a significant spillover effect on low-carbon economic transition. The conclusion can provide the scientific basis for enterprises to adapt to the change in the economic environment to complete low-carbon economic transition and formulate innovation strategies, which is conducive to the sustainable development of the ecological environment and economic society.
1 Introduction
Climate change is a common challenge facing the whole world and has a profound impact on human development. With the development of science and technology and the improvement of human cognition of natural environment systems, human beings are more concerned about climate change than ever before. In particular, under the premise of sustainable economic development, the response to climate change has been great attention. Economic activity and industrialization deteriorate environmental quality (Khan et al., 2021). In this context, a large number of concepts related to carbon emission reduction have emerged in the academic world, such as the low-carbon economy (The first government document to appear was the 2003 UK Energy White paper, Our Energy Future: Building a Low Carbon Economy.), carbon peak, carbon neutrality, etc. Governments have also come up with their own carbon reduction strategies or targets. To actively address climate change, China has assumed the responsibility of building a community with a shared future for mankind. In September 2020, at the General Debate of the 75th session of the UN General Assembly, China explicitly proposed the goal of “striving to reach the peak of carbon dioxide emissions by 2030 and striving to achieve carbon neutrality by 2060”. At the same time, the target was included in the 2021 Chinese Government Work Report for the first time. Carbon peaking means carbon dioxide emissions stop growing and gradually decrease after reaching a peak. Carbon neutrality means offsetting carbon dioxide emissions through planting trees, energy conservation, and emission reduction, and achieving “zero emissions” of carbon dioxide. The carbon peaking goal and carbon reduction goal are called the “two-carbon” goal (Zhang, 2021).
The major strategic deployment of “accelerating the construction of a new development pattern with domestic and international cycles as the main body” provides strategic guidance for the diffusion of low-carbon technology knowledge. By promoting the circular flow of low-carbon technology knowledge, we can help achieve the “two-carbon” goal in China. To achieve this goal, the development of low-carbon technologies is extremely significant (Chao, 2021). Indeed, it is necessary to consider the relationship between economic and social development and carbon emission control in the process of realizing the “two-carbon” goal. We also need to promote the invention and creation of low-carbon technologies and promote the diffusion and large-scale adoption of low-carbon technology knowledge. In the process, we will accelerate energy transformation, optimize the energy mix, strengthen technological innovation, develop renewable energy, and vigorously promote energy conservation and emission reduction (Han et al., 2023; Huang and Cheng, 2023).
Since the outbreak of the financial crisis in 2008, global economic growth has slowed down and the world economy has entered a period of profound adjustment. In particular, in recent years, the international community has been hit by the COVID-19 pandemic and the Russia-Ukraine conflict. Governments frequently adjust their economic policies to smooth out economic fluctuations. For example, the Chinese government has introduced a series of macroeconomic policies, such as “mass entrepreneurship and innovation”, and “three cuts in the exchange rate requirement”. These measures are helping to smooth China’s economic growth, but they are also adding to uncertainty about economic policy (Liu et al., 2020). In this context, scholars pay increasing attention to the economic consequences of economic policy uncertainty.
According to the research results of Baker et al. (2013), the calculated monthly data of China’s economic policy uncertainty index from January 1995 to December 2021 are shown in Figure 1. The index can effectively capture the dynamics of economic policy uncertainty in China. It can be seen that in the long run, the overall trend of China’s economic policy uncertainty index is rising. In the short term, major international events will lead to increased uncertainty about China’s economic policy. In particular, the global financial crisis, the European debt crisis and the outbreak of COVID-19 have led to local spikes in economic policy uncertainty. It also reflects China’s policy adjustment to cope with an increasingly complex internal and external environment.
In addition, scholars have found that technological innovation has a significant contribution to achieving the goals of Industry 4.0 and sustainable development (Jayashree et al., 2021). In the current international environment, innovation has become the core and key to the sustainable development of the national economy. Innovation is closely correlated with enterprise sustainability (Li et al., 2021). Technological innovation can significantly improve corporate productivity (Kahn et al., 2022) and promote corporate financial performance (Khan et al., 2021). At the same time, innovation promotes GDP growth and has an important impact on promoting long-term sustainable economic development (Mohamed et al., 2022). Furthermore, scholars deeply discussed the influence mechanism of innovative organizational culture on enterprise performance from the perspective of enterprise resource management (Ashraf and Ali, 2022). As the main body of innovation, enterprises must have sufficient motivation to carry out innovative activities to maintain competitive advantages.
Therefore, the study takes the uncertainty of economic policy as the starting point, the low-carbon economic transition as the bridge, and the innovation of enterprises as the landing point. There are three innovations in the study.
Firstly, this study adopts the time-varying parameter vector autoregressive model (TVP-VAR) with stochastic volatility, which can better capture the dynamic change of the relationship between economic policy uncertainty and enterprise innovation. It makes up for the shortcomings of previous scholars who only studied their relationships from the perspective of simply single-line relationships. This study finds that the relationship between economic policy uncertainty and enterprise innovation has significant time-varying characteristics. In particular, the outbreak of the financial crisis in 2008 was an important turning point in the transformation of the relationship between economic policy uncertainty and enterprise innovation. After the financial crisis, the positive impact of economic policy uncertainty on enterprise innovation has been declining and has turned from positive to negative. It shows that the impact effect of economic policy uncertainty on enterprise innovation varies greatly under different economic development backgrounds, and may even be the opposite.
Secondly, with the goal of “double carbon”, the low-carbon economic transition is introduced into the research, and the indirect channels of the relationship between economic policy uncertainty and enterprise innovation are deeply explored. The low-carbon economic transition is not only closely related to economic development but also strongly advocated by ecological environmental protection. Therefore, the inclusion of low-carbon economic transition in this study raises the perspective of this study.
Thirdly, combined with theoretical analysis and model tests, this study deeply analyzes the differences in interaction between low-carbon economic transition and enterprise innovation. In the past, scholars often explored the relationship between them based on single-direction research. In this study, the time-varying impact effect between them is concerned. It is more scientific and persuasive to study the relationship between them in the same research context. It is found that low-carbon economic transition has a negative impact effect on enterprise innovation (crowding out effect). Enterprise innovation has a positive impact effect (spillover effect) on the low-carbon economic transition.
To sum up, the contribution of this study lies in the following aspects: 1) In the process of China’s vigorous economic development to build an ecological civilization country, the coordination of economic development, enterprise innovation, and environmental protection is bound to be involved. This study creatively changes the research perspective on the relationship between economic policy uncertainty, low-carbon economy transition, and firm innovation from linear to time-varying nonlinear. It broadens the scholars’ research ideas. 2) The low-carbon economic transition is the focus of the academic circle in the study of ecological environmental protection and economic development. A systematic analysis of the relationship between enterprise innovation and low-carbon economic transition is helpful to clarify the relationship between enterprise innovation and ecological environmental protection and provides an important basis for enterprises to make scientific decisions.
The organizational structure of this paper is as follows: Section 2 is the summary and comment of the relevant literature. Section 3 introduces a systematic method used in the TVP-VAR model and describes the related variables. Section 4 introduces data collection and data analysis. Section 5 deeply analyzes the impact of economic uncertainty and low-carbon economic transition on enterprise innovation. Section 6 summarizes the basic findings and insights of this study.
2 Literature review
In recent years, scholars have paid much attention to the research on the economic consequences of economic policy uncertainty. Economic policy uncertainty generally contains two meanings: on the one hand, economic entities cannot predict whether the policymakers will enact new economic policies or change the existing economic policies in the future. On the other hand, the potential impact caused by economic policy changes is unpredictable (Gulen and Ion, 2012). Myers believes that enterprise investment can be regarded as an option to buy assets for sale in the future (Myers, 1977). Options are valuable only if the expected future investment return exceeds the current investment cost. Gulen confirmed that about two-thirds of the reason for the decline in investment by American enterprises from 2007 to 2009 could be attributed to the uncertainty of economic policies (Gulen and Ion, 2012). Enterprise innovation is an important basis to support strategy implementation. The act of strategic investment itself can be seen as a commitment to a more positive future strategy. Therefore, the relationship between economic policy uncertainty and enterprise innovation has been widely concerned by scholars.
2.1 Economic policy uncertainty and enterprise innovation
In the research on the relationship between economic policy uncertainty and enterprise innovation, scholars have not reached a unanimous conclusion. According to Growth Option Theory, under uncertain conditions, if the expected future income increases and the current investment cost may be low, the enterprise will increase investment under the increased uncertainty (Hartman, 1972; Abel, 1983). In particular, strategic investments enable enterprises to obtain new profit growth opportunities and win future competitive advantages (Kulatilaka and Perotti, 1998). The increasing uncertainty of economic policies will encourage enterprises to make innovation investments as soon as possible to obtain future growth options (Liang and Xie, 2019). At the same time, due to the legal protection of innovation patents, enterprises can obtain the income generated by patents and also create entry barriers with the technological monopoly generated by patents. In this way, the irreversibility of innovation investment can be offset and enterprises can accelerate innovation investment in uncertain environments (Bloom, 2013). According to the principle of risk preference, in the face of uncertainty, the negative impact on an enterprise will at most lead to the loss of initial investment. However, the increase in uncertainty will increase the possible profits of the enterprise, and there is no upper limit. Compared with the cost with a lower limit, the income without an upper limit is more likely to encourage enterprises to increase investment. Therefore, when the uncertainty increases, the investment will not change and may even increase (de la Horra et al., 2022). In addition, the uncertainty of economic policies will produce the “back-forcing effect”, promoting enterprise R&D (Chen and Wang, 2016). Under certain conditions, enterprises are more likely to stabilize existing profits, accelerate cumulative market share, consolidate the market position, and resist external risks and market competition pressure by increasing innovative activities (Kulatilaka and Perotti, 1998). When there is no cooperative relationship between market players, each participant is willing to invest in innovation as soon as possible for fear that competitors will achieve innovation results earlier and monopolize the benefits. Further, some scholars find that the impact of uncertainty is related to the type of investment (Weeds, 2002).
However, Knight’s theory of uncertainty holds that only unexpected changes are sufficient conditions for profit or loss. If future changes can be accurately predicted, profits will disappear (Autor et al., 2003). Economic policy plays a vital role in economic development. Although the policy itself will not affect the innovation of enterprises, the uncertainty of economic policy increases the pessimistic expectation of enterprises on the future environment (Jin et al., 2016; Rao et al., 2017). Therefore, economic policy uncertainty will hinder enterprise innovation (Bhattacharya et al., 2017), which is not conducive to export and performance improvement (Istiak and Serletis, 2018), inhibit the economic development process (He et al., 2020), and even lead to a macroeconomic recession (Bloom, 2009). Further, the study found that this effect is transmitted through the cost of funds channel and marginal rate of return of capital channel (Chen and Wang, 2016). According to real options theory, when enterprises face uncertainty, their future returns are uncertain. Once the investment is made, it is not completely reversible. Although delaying investment will lose the opportunity to make profits in the future, the enterprise can avoid the possibility of large-scale losses by waiting for the strategy to obtain more information about the uncertainty. It means that increased uncertainty will actually increase the waiting value of investment opportunities. Therefore, enterprises will choose to wait patiently for future investment opportunities and reduce current investment (Li and Yang, 2015). Financial friction theory holds that macroeconomic fluctuations will lead to inefficient allocation of resources in financial markets. Economic policy uncertainty may inhibit the efficiency of capital allocation by increasing the degree of financial friction. When the uncertainty of economic policies increases, banks and other financial sectors will face higher default risk, and the motivation for self-protection will be enhanced. They will be more reluctant to lend money out of consideration of the safety of their assets (Valencia, 2017). The decline in enterprise investment is caused by the increase in enterprise financing cost (Song et al., 2019), so the uncertainty of economic policy will inhibit enterprise investment. In the face of uncertainty, if adjustment costs are not taken into account, corporate investment can only reflect the opportunities and profits behind investment projects. However, innovative activities themselves have high uncertainty and high adjustment costs (Bloom, 2007). Economic policy uncertainty intensifies corporate financial constraints (Ma and Hao, 2022) and inhibits intellectual capital investment (Hoang and Tran, 2021). Further, the risk of enterprise innovation is intensified, which may inhibit enterprise innovation activities (Ya et al., 2018).
2.2 Economic policy uncertainty and low-carbon economic transition
The increased uncertainty of economic policies will lead to the relaxation of environmental regulation by local governments, resulting in increased carbon emissions (Amin and Dogan, 2021) and industrial pollution (Wen and Zhang, 2022). There is a causal relationship between renewable energy and carbon dioxide emissions. Governments need to invest in renewable energy to control environmental degradation (Batool et al., 2022). In the context of international turbulence and increasing uncertainty of economic policies, once the implementation effect of economic policies is different from the expected, the uncertainty of economic policies will bring about a very adverse impact on economic development (Wang et al., 2021). The destructive impact will affect the development and policy planning of energy, manufacturing, transportation, and so on, which is not conducive to the realization of the “two-carbon” goal. Both fiscal decentralization and renewable energy intensity have unidirectional negative Granger causality on carbon footprint (Shahzad and Fareed, 2023). Although rents from natural resource pools are an important contributor to economic growth, it is necessary to take into account the finite nature of natural resources (Huang et al., 2020). When economic policies change frequently, banks will require companies to pay higher interest rates in order to avoid risks. This behavior increases the financing cost of enterprises and inhibits the production activities of enterprises (Tan and Zhang, 2017). The financial development of low-cost renewable energy as a remedy for achieving environmental sustainability (Chunyu et al., 2021). This also suggests that financial conditions should be taken into account when implementing energy policies (Pata and Fareed, 2023). At the same time, economic policy uncertainty leads to a slowdown in economic development, which further affects carbon emissions (Dietz and Rosa, 1994; Adedoyin and Zakari, 2020) and ultimately has a negative impact on the low-carbon economic transition. In addition, the adjustment of economic policies affects the degree of emphasis the government attaches to the implementation of environmental protection policies, leads to changes in the intensity of environmental regulation and supervision, and ultimately affects the low-carbon economic transition. When the uncertainty of economic policies increases, the government will pay more attention to stabilizing economic development and overcoming the adverse impact of interest rate and exchange rate fluctuations and will relax the intensity of environmental regulation (Cai et al., 2020). Because punishment is not strong enough, companies tend to pay environmental fines and reduce investment in environmental protection. On the contrary, when the government attaches great importance to the development of environmental protection, enterprises will gradually phase out and replace high-emission and high-pollution products in order to build a good image of actively fulfilling their social responsibilities (Chen et al., 2021).
2.3 Low-carbon economic transition and enterprise innovation
From the existing research results, scholars have studied relatively little on the relationship between low-carbon economic transition and enterprise innovation, but there are abundant researches on the relationship between ecological environmental protection, environmental regulation, and enterprise innovation. In these related studies, scholars mainly have three opinions. The first is the promotion theory. According to Porter’s hypothesis, scholars have found that the increase in air pollution significantly hinders technological innovation, and air pollution has a significant crowding-out effect on innovation funds, thus reducing innovation output (Lin et al., 2021). There was a strong negative correlation between CO2 and the global energy innovation index (Shah et al., 2023). The availability of low-cost renewable energy should reduce domestic demand for fossil fuels and achieve environmental sustainability (Raza et al., 2022). Reducing energy consumption and increasing investment in pollution control have a significant driving effect on the improvement of technological innovation efficiency of industrial enterprises (Li et al., 2019). The second is the inhibition theory. Wuegerz found that neither the strong nor weak Porter hypothesis was valid (Wu and You, 2019). Environmental regulations inhibit technological innovation, and the implementation of carbon emission trading policies will significantly reduce enterprise innovation (Feng et al., 2017). Further, Wang concluded through empirical tests that the Xin’an River Ecological Compensation Bet Agreement (ECGA) inhibited the technological progress of upstream industrial enterprises, especially the heavily polluting enterprises (Wang et al., 2021). The third is the uncertainty theory. Wang believes that there is a complex nonlinear relationship between technological innovation capability and environmental pollution (Wang and Luo, 2020). There is a U-shaped relationship between environmental regulation and technological innovation, and environmental regulation has an “offsetting effect” on the research and innovation capacity of industrial sectors in the short term (Li and Ouyang, 2020). Huang found that environmental regulatory policies would have different impacts on the number of patent applications accepted and industrial sulfur dioxide emissions in different time periods (Huang et al., 2020). Jiang found that there was a U-shaped dynamic characteristic between environmental regulation and enterprise technological innovation (Jiang et al., 2013).
In the long run, technological innovation is an important means to solve environmental problems (Hossain et al., 2022). Technological innovation optimizes industrial structure and reduces environmental pollution (Xin and Lu, 2021). Technological innovation is the key factor to achieve the emission reduction breakthrough (Erdogan, 2021; Xie et al., 2021). According to the legitimacy theory, the development of the low-carbon economy by supply chain enterprises is conducive to the establishment of legitimacy and the acquisition of key resources, such as financial subsidies, tax incentives, and brand value (Zhu et al., 2021). In this way, business risk is reduced and enterprise value is enhanced. According to the signal theory, the development of the low-carbon economy by enterprises can convey a “safe and healthy” signal to consumers, which is helpful to increase consumers’ willingness to buy enterprise products (Zhao et al., 2021). Innovation theory holds that enterprises’ active research and development of low-carbon technologies can effectively improve product production efficiency (Li et al., 2021). According to the economic growth theory, the diffusion of technological knowledge is a by-product of other economic activities, such as investment and trade, with positive externality and spillover (Yan, 2020). A high degree of technological innovation brings a stronger carbon emission reduction effect (Li et al., 2021). The improvement of urban innovation level has a significant inhibitory effect on environmental pollution (Yang et al., 2020). Energy innovation mitigated the harmful impact of air transport on carbon dioxide emissions (Balsalobre-Lorente et al., 2021). Meanwhile, independent innovation has a significant inhibitory effect on haze pollution (Yuan et al., 2021).
The discussion of the above scholars provides important ideas for this study.
No consensus has been reached on the impact of economic policy uncertainty and low-carbon economic transition on enterprise innovation. To a large extent, the reason is that scholars only analyze the relationship between various economic variables from a linear or static perspective. At different times, the economic environment is different. In particular, the impact of major financial events such as the financial crisis cannot be underestimated. After the impact of the external environment, the relationship between different variables will change, and the conclusions will be obviously different. Of course, it also provided the direction for this study. This study focuses on the time-varying characteristics of each variable, and analyzes the dynamic relationship between economic variables in detail, so as to provide strong support for scientific description and explanation of the influence mechanism between variables.
At present, few scholars directly study the relationship between low-carbon economic transition and enterprise innovation and only study the relationship between environmental regulation or environmental protection and enterprise innovation. The previous research perspectives are relatively simple, and the research on low-carbon economic transition and enterprise innovation is not deep, which also provides the possibility of innovation for this study. In addition, the low-carbon economic transition includes ecological environment protection and economic development. Therefore, the study of the interaction mechanism between low-carbon economic transition and enterprise innovation can systematically reflect the interaction between economic and social changes, ecological environmental protection, and sustainable development of enterprises. The theoretical analysis framework of this study is shown in Figure 2.
3 Research methods and data description
3.1 Research methods
In “Macroeconomics and Reality”, Sims introduced vector autoregression (VAR) to study macroeconomic fluctuations. It is of pioneering significance to quantify and analyze macroeconomic fluctuation. The VAR models enable the parameters to act according to a first-order random walk process (Sims, 1980). Therefore, the VAR models constitute not only temporary changes but also permanent changes to the parameters. In different stages of the economic cycle, the economic situation and other factors constantly change. As a result, the traditional vector autoregressive model cannot accurately capture the changes in model parameters. If the random fluctuation time-varying parameter model (TVP-VAR) is adopted, the time-varying of VAR coefficient and error variance can be included in the study, which is conducive to describing the dynamic characteristics of parameters, so as to obtain more accurate research results.
Due to the many advantages of the TVP-VAR model, many scholars often apply the TVP-VAR method to test connectivity and overflow (Diebold and Yılmaz, 2014; Chatziantoniou et al., 2021). With the continuous enrichment of research objects, scholars began to use the TVP-VAR model as an important method to study the impact of macroeconomic variables on specific markets, microeconomic variables, and the behavior of micro-enterprises (Toparlı et al., 2019; Zhang and Yang, 2020; Akyildirim et al., 2021). This provides an important idea and reference for this study.
Therefore, the study uses the TVP-VAR model proposed by Primiceri (2003) that includes stochastic volatility and the parameter estimation method by Nakajima (2011). The TVP-VAR model with stochastic volatility provides for possible changes while examining the structure of the economy because it is both flexible and robust. In the paper, Matlab R2021a software is used to simulate and test the TVP-VAR model. Since the existence of random fluctuations is valuable in the methodology of this study, the Markov chain Monte Carlo (MCMC) method was used in this study to prevent the misspecification of the results. The estimation of a time-varying parameter VAR model with stochastic volatility of the form can be expressed, after Nakajima (2011), as follows:
for t = s+1,….,T, where yt is a (k × 1) vector of observed variables, β1t,…., βst are (k×k) matrices of time-varying coefficients and Ωt is a (k×k) matrices of time-varying covariance matrix. Through the decomposition of Ωt = At−1ΣtΣt′At′−1, At is a lower triangle matrix with diagonal elements equal to one and Σt = diag (σ1t,….,σkt). βt is the stacked row vector of β1t,…., βst; ɑt is the stacked row vector of the free lower-triangular elements of At. In addition, ht is defined as ht=(h1t,…., hkt)′and hjt = logσ2jt.
In this study, the time-varying parameters follow a random walk process:
for t = s+1,…., T with et = At−1 Σt εt, where Σβ, Σɑ and Σh are diagonal, βs+1∼N (µβ0, Σβ0), ɑs+1∼N (µɑ0, Σɑ0), hs+1∼N (µh0, Σh0).
For the identification of the shocks, let var (et) = Ht. Then, we factor the variance-covariance matrix as:
These time-varying matrices are defined as:
The parameters of the TVP-VAR model in Eqs 1–4 are estimated using the Bayesian approach of Primiceri (2003) and Nakajima (2011). The existence of a Bayesian inference enables the estimation of the TVP-VAR models using MCMC methods; the objective of the MCMC methods is to evaluate the joint posterior distributions of the parameters of interest. The parameters of the TVP-VAR model are estimated using Bayesian inference via MCMC. In the application of the MCMC method, the goal is to assess the joint posterior distributions of the parameters of the model defined in Eqs 1–4. For the evaluation of joint posterior distributions, one needs to set certain priors for all the unknown parameters in the model, except for the time-varying state variables, which are estimated recursively given the initial states. Following Nakajima [79], we use the following priors: Σβ∼IW(25, 0.01I), (Σɑ)i−1∼G (4, 0.02), (Σh)i−1∼G (4, 0.02), where (Σɑ)i and (Σh)i are the ith diagonal elements of (Σɑ)i and (Σh)i, respectively. IW and G represent the inverse Wishart and gamma distributions, respectively. For the initialization of the TVPs, we use flat priors by setting µβ0 = µɑ0 = µh0 = 0 and Σβ0 = Σɑ0 = Σ = 10 × I.
3.2 Variable description
In this study, three dimensions of economic policy uncertainty, low-carbon economic transition, and enterprise innovation are selected for modeling analysis. The sample data cover the period between Q1 1995 and Q4 2021. The data mainly come from the website of the National Bureau of Statistics of China (www.stats.gov.cn), the WIND database, and the CSMAR database. In order to make the time-frequency of different variables selected to match each other, the three types of variables are converted into quarterly data by referring to the practice of previous scholars. In order to avoid sequence heteroscedasticity, it is necessary to take the logarithms of all variables before substituting correlation sequences into the TVP-VAR model. The selection and description of each indicator are as follows.
3.2.1 Economic policy uncertainty (EPU)
The economic policy uncertainty is the unpredictable risk accompanying the adjustment of economic policy. It is an unknown state in which it is difficult for economic agents to predict the process of policy-making, the timing of its release, and its potential impact. As a result, it is difficult to measure economic policy uncertainty effectively. Baker [7] used text filtering technology to construct China’s economic policy Uncertainty Index (EPU) by taking Hong Kong’s South China Morning Post as a text information source and using the proportion of relevant reports containing four keywords of “China”, “economy”, “uncertainty” and “policy” in the total number of articles in the month. Since this index can effectively reflect the uncertainty level of economic policies and has good traceability, continuity, and time-varying characteristics, a large number of studies based on the EPU index have appeared. Therefore, this study draws on Baker’s method and uses this index for analysis.
3.2.2 The low-carbon economic transition (CE)
According to the research of Wang et al. (2019), considering that carbon productivity directly reflects the comprehensive utilization efficiency of carbon resources in the social economy, carbon productivity, namely, the gross national income created by each unit of CO2 emission, is adopted to measure the degree of the low-carbon economic transition.
3.2.3 Enterprise innovation (RD)
According to the method proposed by Yang (2021), the full-value equivalent of R&D personnel in large and medium-sized industrial enterprises is used to measure enterprise innovation. It is a recognized index of enterprise innovation input. In China, the proportion of large and medium-sized enterprises in R&D is relatively high, and its trend can basically measure the overall trend of R&D.
4 Data collection and data analysis
Data stability is an important basis of macroeconomic variable modeling. If a time series is not stationary, the randomness of the sequence population cannot be revealed through the sequence samples. Therefore, a unit root test is carried out for each sequence before the time-varying parameter estimation to test whether each sequence is stationary. In this study, the Phillips-Perron method (PP) (Phillips and Perron, 1988) was used for the unit root test. Except for EPU, which passed the stationarity test in Level with Intercept and Trend test, other variables did not pass the stationarity test. Therefore, these variables are treated with a first-order difference. Table 1.
Since all variables are non-stationary, their co-integration relationships should be examined to determine the correct model specification. We used a trace test of multivariate VAR and a maximum eigenvalue test to conduct an order test of co-integration (Johansen, 1991; Johansen and Juselius, 2009). Based on the response surface critical values provided by Kripfganz and Schneider, the robustness of Johansen’s test is further tested by using the autoregressive distributed auto regression (ARDL) boundary test method (Kripfganz and Schneider, 2020). As shown in Table 2, in the ordinal test of co-integration, the lag order determined by LR test statistics, FPE criterion, and AIC information criterion is 2. The subsequent order determined by the SC criterion and HQ criterion is 1. The sample size of this study is relatively small, and the AIC information criterion performs better in small samples. Therefore, after comprehensive consideration, the lag order of VAR is determined to be 2 in the co-integration test and TVP-VAR model estimation.
The results of the Johansen co-integration test are shown in Table 3. The trace test and maximum eigenvalue test fail to reject the null hypothesis of “no co-integration” at the 5% level. Both trace test and maximum eigenvalue test statistics show that at the 5% significance level, there is no co-integration relationship between economic policy uncertainty, low-carbon economic transition, and enterprise innovation sequence.
We use boundary tests to test the robustness of the Johansen co-integration test results. The boundary test results are shown in Table 4. F-test and t-test type boundary checks are performed using two different deterministic component specifications, one with only constants and the other with constants and trends. The table also reports the upper bound of the critical value when the mandatory variable is purely an integral of order 1, i.e., I(1), and the lower bound of the critical value when the mandatory variable is purely an integral of order 0, i.e., I(0). When boundary checks are performed using deterministic component specifications with only constants, the F-test estimate value is 1.434 and the t-test estimate value is −0.319, so both F-test and t-test do not reject the null hypothesis of no co-integration at the 5% level. At the same time, when boundary checks are performed using deterministic component specifications with a constant and trend, the F-test estimate value is 1.155 and the t-test estimate value is −0.932, so both F-test and t-test do not reject the null hypothesis of no co-integration at the 5% level. Neither the F-test nor the t-test rejects the null hypothesis that there is no co-integration at the 5% level. Therefore, the boundary test confirms the results of the multivariable Johansen co-integration test, indicating that there is no co-integration relationship between the three variables.
The study covers a long period, especially the financial crisis of 2008. Such a major crisis may cause the parameters of the estimated VAR model or ARDL model to be unstable, making inferences based on these constant parameter models invalid. In order to test the instability of parameters, we conducted CUSUM and CUSUM squared tests for each equation of the VAR model.
Figure 3 shows the CUSUM and CUSUMSQ tests, which are based on recursively estimated parameters. It is known that the CUSUM test has better testing power when the structural fracture is within the intercept of the regression equation. When the structural fracture affects the slope of the regression equation or the variance of the error term, the CUSUMSQ test has better testing power. Panel A, B, and C in Figure 2 show the CUSUM and CUSUMSQ tests of economic policy uncertainty, low-carbon economic transition, and enterprise innovation, respectively. In the CUSUM test, the parameters of the enterprise innovation equation show instability at the significance level of 5% in different periods. In the CUSUMSQ test, the parameters of the low-carbon economic transition equation and enterprise innovation equation both show instability at the significance level of 5% in different periods. Therefore, the inference based on the constant parameter model is inappropriate, and this result supports the preference for the TVP-VAR model.
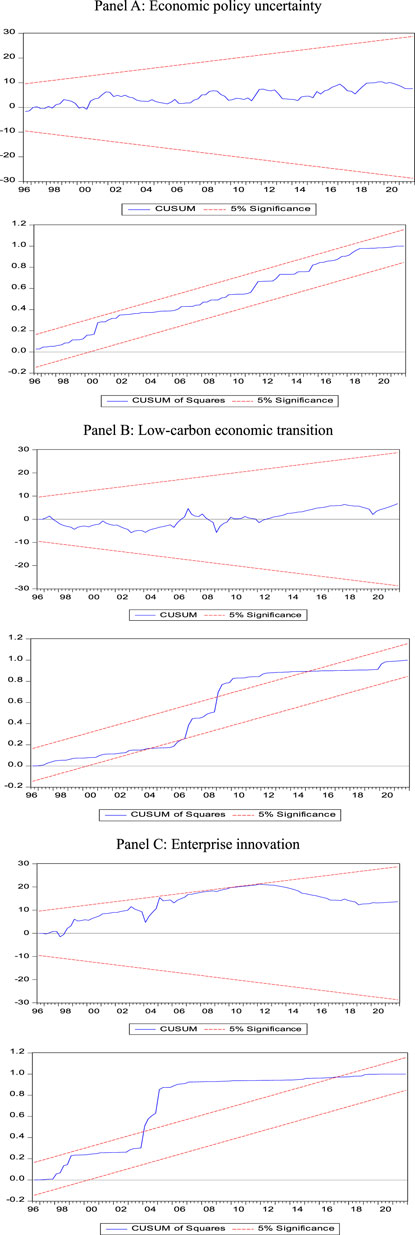
FIGURE 3. CUSUM tests and CUSUM of squares test for parameter stability of economic policy uncertainty (A), lowcarbon economic transition (B), and enterprise innovation (C).
5 Results
Figure 4 successively shows the graphs of the sample autocorrelation function, value path, and posterior distribution density function of the MCMC method. It can be seen that the autocorrelation degree of parameter estimation results is low and stable. The extreme value of the value path is small, indicating that the value path is stable. The posterior density function basically conforms to the normal distribution. Therefore, it can be judged that there is no correlation between samples generated by the MCMC method, and it is effective. The model fits well.
As shown in Table 5, irrelevant samples contain more sample information, which can ensure the effectiveness of parameter estimation. The MCMC method was used for model estimation, and 11,000 simulation samples were selected to obtain effective samples, among which the first 1,000 simulation results were discarded as the “burn-in” phase. The convergence of Markov chains can be measured by the Geweke value. The invalid impact factor is used to measure the number of unrelated samples produced by the model simulation. These two indexes are the important basis for judging the simulation and estimation effect of MCMC. The Geweke values of all parameters are less than 1, far less than the critical value of 1.96, so the model can accept the null hypothesis that converges to a posterior distribution. In other words, 11,000 simulations can produce a valid sample. The smaller the invalid factor value, the more valid the sample. The invalid influencing factors of the estimation results of each parameter in this model are small, among which the largest invalid influencing factor is 70.24 (μh), and the invalid factor values are all less than 100, indicating that the samples generated for each parameter are valid enough. That is, the null hypothesis that the statistics tend to a posterior distribution cannot be rejected. In summary, the sampling results are valid and robust.
TVP-VAR model provides two kinds of impulse response functions: impulse response at equal intervals and impulse response at specific time points. The function of impulse response with equal intervals is used to observe the change of the dependent variable when the independent variable is given positive shock at equal intervals at each time point. Impulse response function at a specific time point refers to giving a positive influence to the independent variable at a specific time point, and the dynamic change of the dependent variable decreases with time. In this study, 4 steps ahead, 8 steps ahead, and 12 steps ahead were selected to measure short-term, medium-term, and long-term time constraints respectively.
5.1 The impulse response function at equal intervals
Figure 5 shows the pulse response function graph with equal intervals. By observing the effect of economic policy uncertainty on enterprise innovation (ε EPU↑→RD), it can be found that the trend of the impulse response function of different lead periods is similar, but there are some differences. In general, the positive response of economic policy uncertainty to enterprise innovation reached its maximum around 2008. After the outbreak of the financial crisis in 2008, the positive impact of economic policy uncertainty on enterprise innovation has been weakening. Around 2015, the response of economic policy uncertainty to enterprise innovation changed from positive to negative. It reached its lowest point around 2018, and then the negative impact effect tended to converge to 0. The results show that the influence of economic policy uncertainty on enterprise innovation has asymmetric characteristics. After the financial crisis, the social expectation of the economic environment presents a pessimistic attitude, and the economic policy uncertainty of enterprise investment gradually shows the inhibitory effect on enterprise innovation. Specifically, the intensity of impulse response decreases with the increase in lead time, indicating that enterprise innovation is sensitive to the impact of economic policy changes.
By observing the effect of economic policy uncertainty on the low-carbon economic transition (ε EPU↑→CE), it can be found that economic policy uncertainty has a negative impact on the low-carbon economic transition. In general, before 2003, economic policy uncertainty had a weak negative impact on the low-carbon economic transition. After 2003, the negative impact effect of economic policy uncertainty on the low-carbon economic transition continued to strengthen and gradually weakened after reaching a negative peak around 2008, especially the impulse response function of 4 steps ahead. It indicates that the frequent introduction of economic policies during the financial crisis has a great impact on the low-carbon economic transition and can continue to bring about adverse impacts quickly. Around 2015, the negative impact tends to converge to 0, which means that the negative impact of economic policy uncertainty on the low-carbon economic transition presents a “V” shaped trend. After 2015, the negative impact gradually increased.
By observing the effect of enterprise innovation on economic policy uncertainty (ε RD↑→EPU), it can be found that three impulse response curves are all above the zero line, indicating that enterprise innovation has a positive impact on economic policy uncertainty. The impulse response trend of the 4 steps ahead is quite different from the 8 stages and 12 stages ahead impulse responses. The pulse response diagram of 4 steps ahead presented an inverted “V” shape and reached the maximum around 2008. However, the impulse response of 8 steps ahead and 12 steps ahead were similar, showing a positive “V” shape, and both reached the lowest value around 2008, and the amplitude of decrease was obvious. In the medium and long term, corporation innovation has an absorbing effect on the fluctuation of economic policy uncertainty during the outbreak of the financial crisis. In the short term, corporation innovation does not have an obvious absorbing effect on economic policy uncertainty, which indicates that corporation innovation has a certain cumulative effect on macroeconomic needs.
By observing the effect of enterprise innovation on the low-carbon economic transition (ε RD↑→CE), it can be found that the impact of enterprise innovation on the low-carbon economic transition presents a positive “V” shape. The three impulse response trends all reached the lowest values around 2008, indicating that the financial crisis weakened the positive impact effect of enterprise innovation on the low-carbon economic transition. Specifically, the positive impact of enterprise innovation on the low-carbon economic transition gradually weakens with the increase in lead time, indicating that the low-carbon economic transition is sensitive to enterprise innovation and responds quickly.
By observing the effect of low-carbon economic transition on economic policy uncertainty (ε CE↑→EPU), it can be found that all three impulse response curves are above the zero line, indicating that the low-carbon economic transition will have a positive impact on economic policy uncertainty. Relatively speaking, the impulse response curve of the 4 steps ahead has little fluctuation, indicating that in the short term, the impact of the low-carbon economic transition on economic policy uncertainty does not have an obvious abrupt effect. The impulse response curves of 8 steps ahead and 12 steps ahead reached the lowest value around 2008, indicating that in the medium and long term, the financial crisis broke the inherent response rule of the low-carbon economic transition to economic policy uncertainty, and had a great destructive effect on economic stability.
By observing the effect of the low-carbon economic transition on enterprise innovation (ε CE↑→RD), it can be found that the three impulse response trends are all below the zero line, indicating that the low-carbon economic transition will bring a negative impact on enterprise innovation. The impact effect of the low-carbon economic transition on enterprise innovation in the 4 steps and 8 steps ahead is significantly stronger than the impulse response of the 12 steps ahead, indicating that the crowding out effect of the low-carbon economic transition on enterprise innovation is more obvious in the short term.
5.2 Impulse response analysis at a specific point in time
Figure 6 shows the graph of the impulse response function at specific time points. In terms of the response of enterprise innovation to economic policy uncertainty (ε EPU↑→RD), there are significant differences at three specific time points. In general, in 2003 and 2008, enterprise innovation showed a positive response to economic policy uncertainty. In the first quarter of 2020, the response of enterprise innovation to economic policy uncertainty was negative and shrinking, gradually approaching 0. It also shows that in the rising stage of economic development, the response of enterprise innovation to the impact of economic policy uncertainty is generally positive. In the down phase of the business cycle, the impulse response of economic policy uncertainty is always negative and gradually approaches 0. The time-varying impulse response function of economic policy uncertainty on enterprise innovation at specific time points shows again that the influence of economic policy uncertainty on enterprise innovation has asymmetric characteristics.
In terms of the response of the low-carbon economic transition to economic policy uncertainty (ε EPU↑→CE), the economic policy uncertainty at all three-time points has a negative impact on the low-carbon economic transition, reaching the peak in the 2–3 period, and eventually converging. In the peak area, the peak value was the highest in 2008, indicating that the outbreak of the financial crisis intensified the negative impact of economic policy uncertainty on the low-carbon economic transition. The result is consistent with the performance of the impulse response function with equal intervals mentioned above.
In terms of the response of economic policy uncertainty to enterprise innovation (ε RD↑→EPU), the three specific time points have roughly the same performance. In general, the response of economic policy uncertainty to enterprise innovation is positive and reaches a peak rapidly in the first period, after which the positive feedback effect gradually weakens and tends to converge. It suggests that innovation has a significant and sustainable impact on macroeconomic policy.
In terms of the response of low-carbon economic transition to enterprise innovation (ε RD↑→CE), the trend of impulse response function at different time points is slightly different, but the overall response is positive. The positive impact can be maintained for a long time, which once again verifies the positive externality of enterprise innovation to the low-carbon economic transition.
In terms of the response of economic policy uncertainty to the low-carbon economic transition (ε CE↑→EPU), the three specific time points show roughly the same positive response. The peak value is reached in the second period, then decreases continuously, and tends to 0. It indicates that when economic policy uncertainty is positively impacted by the low-carbon economic transition, it will lead to an increase in economic policy uncertainty. However, with the accumulation of time, the response of low-carbon economic transition to economic policy uncertainty is weakening.
In terms of the response of enterprise innovation to the low-carbon economic transition (ε CE↑→RD), the trend of impulse response function at different time points is roughly the same, which is a negative response. The negative response increased over time and reached a peak at stage 5, then gradually decreased. It also indicates that the low-carbon economic transition has an obvious crowding-out effect on enterprise innovation, and the inhibition effect is gradually weakened with the accumulation of time.
6 Discussions and conclusion
6.1 Discussions
6.1.1 Economic policy uncertainty and enterprise innovation
Referring to the division of economic development stages by scholars, 2010 is regarded as the turning point of the economic development stage in China (Kripfganz and Schneider, 2020). This study finds that before 2010, the economy was on the upswing in China, at which time the uncertainty of economic policies had a significant positive impact on enterprise innovation. However, after 2010, the economy was in a downtrend, and the positive impact of economic policy uncertainty on enterprise innovation was weakened or even negative inhibition. In different stages of the economic cycle, the influence of economic policy uncertainty on enterprise innovation has an asymmetric effect, namely, the “Matthew effect”.
Su discussed the path to breaking through the “Matthew effect” from the perspective of resource allocation (Su, Tao, and Xiao). Similarly, is there a path to breaking through the Matthew effect of economic policy uncertainty on enterprise innovation? In order to make the uncertainty of economic policy play a positive role, scholars need to think about this issue further. Further, the “Matthew effect” magnifies the effect of the economic environment on micro subjects and plays an obvious magnifying glass role. It is a very important revelation for the government. During the economic upturn, the moderate increase in economic policy uncertainty is conducive to enterprise innovation. However, during the economic downturn, the uncertainty of economic policy should be reduced as far as possible to reduce the destructive effect of economic policy uncertainty on enterprise innovation.
6.1.2 Economic policy uncertainty and low-carbon economic transition
Gao and Wang (2021) has found that the possibility of enterprises investing in low-carbon technology is influenced by policy stability. Furthermore, scholars found that increased uncertainty of economic policies would inhibit enterprises’ investment in environmental protection (Yao and Lin, 2023). This study clearly confirms that economic policy uncertainty has a negative impact on the low-carbon economic transition. This is consistent with the research conclusion that economic policy uncertainty has a significant negative effect on carbon-neutral development (Li et al., 2022). It is worth noting that when the financial crisis occurred, the negative impact of economic policy uncertainty on the low-carbon economic transition reached its peak, which shows that the financial crisis has a strong destructive effect on the low-carbon economic transition. The financial crisis has disrupted the relatively stable dynamic between economic policy uncertainty and the low-carbon economic transition.
Financial development can effectively regulate the negative impact of economic policy uncertainty on regional haze pollution, exacerbate pollution in the short term, and restrain haze pollution in the long term (Han and Qi, 2019). From another perspective, whether the time-varying characteristics of the negative impact of economic policy uncertainty on the low-carbon economic transition can predict the financial crisis to some extent? From 2008 to 2015, the negative impact of economic policy uncertainty on the low-carbon economic transition decreased continuously and tended to 0, and then gradually expanded. Will the next peak also mean the arrival of the next financial crisis? It is worth scholars to continue to carry out in-depth research on this issue.
6.1.3 Enterprise innovation and low-carbon economic transition
From the perspective of mechanism analysis, it is not contradictory that there are differences in the interaction results between enterprise innovation and low-carbon economic transition. Enterprise innovation has a significant positive impact on the low-carbon economic transition, indicating that technological progress brought by innovation provides an important driving force for the development of the low-carbon economic transition. This is consistent with the scholars’ conclusion that without the support of research and development (R&D) and government subsidies, the attempt to implement low-carbon technologies will fail (Świadek et al., 2021). It is worth noting that the low-carbon economic transition has a significant negative impact on enterprise innovation. The development of the low-carbon economic transition has crowded out the inflow of enterprise resources into the field of enterprise research and development. Scholars should consider the reasons why eco-environmental protection does not help enterprises to innovate. It is possible to strengthen the protection of intellectual property rights and encourage the full use of innovation resources to improve the crowding effect of low-carbon economic transition on enterprise innovation. Of course, it requires scholars to continue to explore from these perspectives.
6.1.4 Limitations of the study
Although this study creatively reveals the time-varying characteristics of the relationship between economic policy uncertainty and firm innovation and systematically analyzes the interaction between low-carbon economic transition and firm innovation. However, there are still some limitations. On the one hand, the research background of this study is the Chinese economy and society. Due to China’s economic size, population size, geographical environment, and other factors, the conclusions of this study may not be directly applicable to other countries and regions. On the other hand, the uncertainty of economic policy is complicated and difficult to be measured. Although this study refers to the usual practices of scholars for measurement, the selection of indicators still requires scholars’ continuous research and exploration to ensure that each economic variable can be measured better.
6.2 Conclusion, implications, and future recommendations
The results show that, firstly, the impact of economic policy uncertainty on enterprise innovation has time-varying characteristics and “asymmetry”. Specifically, “there is a positive impact in the economic uptrend period and a negative impact in the economic downtrend period”, and it is greatly influenced by the financial crisis. After the financial crisis, the positive impact of economic policy uncertainty on enterpris innovation has been weakening, and in 2015, the positive impact turned into a negative impact. Secondly, economic policy uncertainty has a negative impact on the low-carbon economic transition, and the occurrence of a financial crisis worsens the negative impact of economic policy uncertainty on the low-carbon economic transition. Thirdly, the low-carbon economic transition has an obvious inhibitory effect on enterprise innovation, namely, the crowding out effect. However, enterprise innovation has an obvious positive externality on the low-carbon economic transition, namely, the spillover effect.
Therefore, we provide two contributions to the theory. Firstly, this study considers the time-varying characteristic relationship between macroeconomic policy changes and firm behaviors through the TVP-VAR model. This paper creatively proposes and tests the asymmetric influence of economic policy uncertainty on firm innovation. This conclusion enriches the relevant research on firm innovation and contributes to the understanding of the impact of economic policy changes on firm behavior. Secondly, we deeply study the impact relationship between enterprise low-carbon economic transition and enterprise innovation and enrich the relevant theories of environmental protection and enterprise innovation.
At the same time, this study also has important practical significance. On the one hand, it identifies the negative impact of economic policy uncertainty on the low-carbon economic transition in enterprises. This research result provides valuable information for economic policymakers, and the influence of policymakers on enterprises’ environmental protection behavior when changing economic policies is an important factor worth considering. On the other hand, this study found the interaction between enterprises’ low-carbon economic transformation and enterprises’ innovation behavior, which provides important ideas for the resource allocation between enterprises’ managers’ fulfillment of environmentally sustainable development responsibilities and enterprises’ innovation strategy layout. In general, this study rethinks the strategic layout of enterprise environmental protection and innovation from the perspective of China’s economic development and makes an important contribution to the theoretical and practical fields.
Further research can try to measure the level of economic policy uncertainty through social media messages. Some scholars have found that polarity, subjectivity, economic uncertainty, or market uncertainty can be manifested on social media platforms such as Twitter (Ali et al., 2022). Therefore, using information on social media platforms such as Twitter and Sino Weibo to measure economic policy uncertainty can be interesting extensions of this study.
Data availability statement
The original contributions presented in the study are included in the article/Supplementary Material, further inquiries can be directed to the corresponding author.
Author contributions
CS was mainly responsible for data collation, empirical analysis, model building, and wrote manuscript. LZ provided research route, theoretical analysis, JW provided software operation guidance, helped with manuscript modification, and wrote the manuscript. All authors contributed to the article and approved the submitted version.
Funding
Harbin University of Commerce Faculty “Innovation” Project (22JYC335).
Conflict of interest
The authors declare that the research was conducted in the absence of any commercial or financial relationships that could be construed as a potential conflict of interest.
Publisher’s note
All claims expressed in this article are solely those of the authors and do not necessarily represent those of their affiliated organizations, or those of the publisher, the editors and the reviewers. Any product that may be evaluated in this article, or claim that may be made by its manufacturer, is not guaranteed or endorsed by the publisher.
References
Adedoyin, F. F., and Zakari, A. (2020). Energy consumption, economic expansion, and CO2 emission in the UK: the role of economic policy uncertainty. Sci. Total Environ. 738, 140014. doi:10.1016/j.scitotenv.2020.140014
Akyildirim, E., Aysan, A. F., Cepni, O., and Darendeli, S. P. C. (2021). Do investor sentiments drive cryptocurrency prices? Econ. Lett. 206, 109980. doi:10.1016/j.econlet.2021.109980
Ali, F., Bouri, E., Naifar, N., Shahzad, S. J. H., and AlAhmad, M. (2022). An examination of whether gold-backed islamic cryptocurrencies are safe havens for international islamic equity markets. Res. Int. Bus. Finance 63, 101768. doi:10.1016/j.ribaf.2022.101768
Amin, A., and Dogan, E. (2021). The role of economic policy uncertainty in the energy-environment nexus for China: evidence from the novel dynamic simulations method. J. Environ. Manage 292, 112865. doi:10.1016/j.jenvman.2021.112865
Ashraf, Y., and Ali, F. (2022). How innovative organization culture affects firm performance in the wake of enterprise resource planning? Evidence from energy- and non-energy-sector firms in Pakistan. Front. Environ. Sci. 10. doi:10.3389/fenvs.2022.991319
Autor, D. H., Levy, F., and Murnane, R. J. (2003). The skill content of recent technological change: an empirical exploration. Q. J. Econ. 118, 1279–1333. doi:10.1162/003355303322552801
Baker, S. R., Bloom, N., and Davis, S. J. (2013). Measuring economic policy uncertainty. SSRN Electron. J. 131. doi:10.2139/ssrn.2198490
Balsalobre-Lorente, D., Driha, O. M., Leitao, N. C., and Murshed, M. (2021). The carbon dioxide neutralizing effect of energy innovation on international tourism in Eu-5 countries under the prism of the ekc hypothesis. J. Environ. Manage 298, 113513. doi:10.1016/j.jenvman.2021.113513
Batool, Z., Raza, S. M. F., Ali, S., and Abidin, S. Z. U. (2022). Ict, renewable energy, financial development, and Co(2) emissions in developing countries of east and South asia. Environ. Sci. Pollut. Res. Int. 29, 35025–35035. doi:10.1007/s11356-022-18664-7
Bhattacharya, U., Hsu, P.-H., Tian, X., and Xu, Y. (2017). What affects innovation more: policy or policy uncertainty? J. Financial Quantitative Analysis 52, 1869–1901. doi:10.1017/s0022109017000540
Bloom, N. (2007). Uncertainty and the dynamics of R&D. Am. Econ. Rev. 97, 250–255. doi:10.1257/aer.97.2.250
Chao, Q. (2021). The scientific connotation of "Carbon peak and carbon neutrality" and China's policy measures. Environ. Sustain. Dev. 46, 14–19. doi:10.19758/j.cnki.issn1673-288x.202102014
Chatziantoniou, I., Gabauer, D., and Marfatia, H. A. (2021). Dynamic connectedness and spillovers across sectors: evidence from the Indian stock market. Scott. J. Political Econ. 69, 283–300. doi:10.1111/sjpe.12291
Chen, G., and Wang, S. (2016). How does economic policy uncertainty affect firm investment behavior. Finance trade Econ. 2016, 5–21. doi:10.19795/j.cnki.cn11-1166/f.2016.05.001
Chen, L., Huang, J., Cao, F., and Zhou, Z. (2021). Executive ownership, economic policy uncertainty and corporate green investment. Financial theory Pract. 42, 58–64. doi:10.16339/j.cnkihdxbcjb.2021.03.008
Chunyu, L., Zain-Ul-Abidin, S., Majeed, W., Raza, S. M. F., and Ahmad, I. (2021). The non-linear relationship between carbon dioxide emissions, financial development and energy consumption in developing European and central asian economies. Environ. Sci. Pollut. Res. Int. 28, 63330–63345. doi:10.1007/s11356-021-15225-2
de la Horra, L. P., Perote, J., and de la Fuente, G. (2022). The impact of economic policy uncertainty and monetary policy on R&D investment: an option pricing approach. Econ. Lett. 214, 110413. doi:10.1016/j.econlet.2022.110413
Diebold, F. X., and Yılmaz, K. (2014). On the network topology of variance decompositions: measuring the connectedness of financial firms. J. Econ. 182, 119–134. doi:10.1016/j.jeconom.2014.04.012
Dietz, T., and Rosa, E. A. (1994). Rethinking the environmental impacts of population, affluence and technology. Hum. Ecol. Rev. 1, 277–300. doi:10.2307/24706840
Erdogan, S. (2021). Dynamic nexus between technological innovation and building sector carbon emissions in the brics countries. J. Environ. Manage 293, 112780. doi:10.1016/j.jenvman.2021.112780
Feng, C., Shi, B., and Kang, R. (2017). Does environmental policy reduce enterprise innovation? evidence from China. Sustainability 9, 872. doi:10.3390/su9060872
Gao, S., and Wang, C. (2021). How to design emission trading scheme to promote corporate low-carbon technological innovation: evidence from China. J. Clean. Prod. 298, 126712. doi:10.1016/j.jclepro.2021.126712
Gulen, H., and Ion, M. (2012). Political uncertainty and corporate investment. SSRN Electron. J. 29. doi:10.2139/ssrn.2188090
Haijing, C., Zhang, H., and Wu, Y. (2020). The impact of economic policy uncertainty on environmental investment. Account. Friends 2020, 112–117. Available at: https://kns.cnki.net/kcms/detail/14.1063.F.20201203.1342.032.html.
Han, W., Yang, N., and Xiong, Y. (2023). Study on the evolution of energy consumption structure and its influencing factors in scientific and technological countries. Sci. Technol. Manag. Res. 43, 199–205. Available at: https://kns.cnki.net/kcms2/article/abstract?v=O7UxWaLGDVwH7PscKIYvhPjnkZHwNoO3IZkHMS8ohedqV5G2CuovfD1bpxkEmYNqyJwJis2uMxeRBOtSwrgjNpnH76SmEDnEht8IZMc447jVW4LfqrqOL2M5A6O5xFjk4ESTORKJAFw=&uniplatform=NZKPT&language=CHS.
Han, Y., and Qi, X. (2019). Economic policy Uncertainty, financial development and smog pollution: based on the study of coordinated emission reduction in Western China. Ind. Tech. Econ. 38, 8. Available at: https://kns.cnki.net/kcms2/article/abstract?v=Zx5UZ-cNHTgUBG5LwJ7IZknqMZwUofuuJ0azPrd6HtS17lbz6SEOmpueeYc0RH14ZItv7z-8nsr0_Hv-oP9p3pSF8jFXOlz2QkVUf1ZWfxBdrh3JMEVkK3Xp_LhM-f2hgeNnKZ8PhIs=&uniplatform=NZKPT&language=CHS.
Hartman, R. (1972). The effects of price and cost uncertainty on investment. J. Econ. Theory 5, 258–266. doi:10.1016/0022-0531(72)90105-6
He, F., Wang, Z., and Yin, L. (2020). Asymmetric volatility spillovers between international economic policy uncertainty and the U.S. Stock market. North Am. J. Econ. Finance 51, 101084. doi:10.1016/j.najef.2019.101084
Hoang, K., and Tran, T. T. (2021). Policy uncertainty and intellectual capital investment. Appl. Econ. Lett. 29, 1369–1377. doi:10.1080/13504851.2021.1934383
Hossain, M. E., Islam, M. S., Bandyopadhyay, A., Awan, A., Hossain, M. R., and Rej, S. (2022). Mexico at the crossroads of natural resource dependence and Cop26 pledge: does technological innovation help? Resour. Policy 77, 102710. doi:10.1016/j.resourpol.2022.102710
Huang, Q., and Cheng, C. (2023). Study on regional carbon emission and industrial structure transformation and upgrading under the dual carbon target: a case study of anhui province. Sci. Technol. Manag. Res. 43, 221–228. Available at: https://kns.cnki.net/kcms2/article/abstract?v=Zx5UZ-cNHThAJ7_I2yle_-na0FqXZWNJRI0IcCeL8MC4CqWfqDK4t8q024odw63uHxIl4Q_Lf1S5EjpGUZT_hNurhXDWLZoPabPyXOO2u78d1CCvnrEcylwIL8TXGV6VhNkJ9HcRou0=&uniplatform=NZKPT&language=CHS.
Huang, T., Zhao, X., and Chen, K. (2020). Technological innovation, environmental pollution, and regulatory policy: the perspective of Transformational innovation policy. Sci. Sci. Technol. Manag. 41, 49–65. Available at: https://kns.cnki.net/kcms2/article/abstract?v=Zx5UZ-cNHTgp8bSoB-4kEhRUMEtuBKxfPUR6ogElWeb2BhRW0vfsFy5XmFSjPBr4GeMM0VA31ObJFd41CCKes0_L0jQWiudG6t8DoxaX3HEF42dVzHdXnqb6mwRvstyFLdYuBSJZego=&uniplatform=NZKPT&language=CHS.
Huang, Y., Raza, S. M. F., Hanif, I., Alharthi, M., Abbas, Q., and Zain-ul-Abidin, S. (2020). The role of forest resources, mineral resources, and oil extraction in economic progress of developing asian economies. Resour. Policy 69, 101878. doi:10.1016/j.resourpol.2020.101878
Istiak, K., and Serletis, A. (2018). Economic policy uncertainty and real output: evidence from the G7 countries. Appl. Econ. 50, 4222–4233. doi:10.1080/00036846.2018.1441520
Jayashree, S., Hassan Reza, M. N., Malarvizhi, C. A. N., Maheswari, H., Hosseini, Z., and Kasim, A. (2021). The impact of technological innovation on industry 4.0 implementation and sustainability: an empirical study on Malaysian small and medium sized enterprises. Sustainability 13, 10115. doi:10.3390/su131810115
Jiang, F., Wang, Z., and Bai, J. (2013). The dual effect of environmental regulation on technological innovation: an empirical study based on dynamic panel data of Jiangsu Manufacturing industry. Chin. Ind. Econ. 2013, 44–55. doi:10.19581/j.cnki.ciejournal.2013.07.004
Jin, G., Liu, Z., and Guiru, H. (2016). Policy uncertainty and firm Investment: an empirical study of strategic emerging industries. Manag. Rev. 28, 3–16. doi:10.14120/j.cnki.cn11-5057/f.2016.09.001
Johansen, S. (1991). Estimation and hypothesis testing of cointegration vectors in Gaussian vector autoregressive models. Econometrica 59, 1551. doi:10.2307/2938278
Johansen, S., and Juselius, K. (2009). Maximum likelihood estimation and inference on cointegration - with applications to the demand for money. Oxf. Bull. Econ. Statistics 52, 169–210. doi:10.1111/j.1468-0084.1990.mp52002003.x
Kahn, A., Sithole, M., and Buchana, Y. (2022). An analysis of the impact of technological innovation on productivity in South African manufacturing firms using direct measures of innovation. South Afr. J. Econ. 90, 37–56. doi:10.1111/saje.12310
Khan, S. A. R., Ponce, P., Tanveer, M., Aguirre-Padilla, N., Mahmood, H., and Shah, S. A. A. (2021). Technological innovation and circular economy practices: business strategies to mitigate the effects of Covid-19. Sustainability 13, 8479. doi:10.3390/su13158479
Khan, S. A. R., Ponce, P., and Yu, Z. (2021). Technological innovation and environmental taxes toward a carbon-free economy: an empirical study in the context of cop-21. J. Environ. Manage 298, 113418. doi:10.1016/j.jenvman.2021.113418
Kripfganz, S., and Schneider, D. C. (2020). Response surface regressions for critical value bounds and approximate P-values in equilibrium correction Models1. Oxf. Bull. Econ. Statistics 82, 1456–1481. doi:10.1111/obes.12377
Kulatilaka, N., and Perotti, E. C. (1998). Strategic growth options. Manag. Sci. 44, 1021–1031. doi:10.1287/mnsc.44.8.1021
Kun, Y., Luo, F., and Li, Q. (2018). Economic Policy Uncertainty, Financial asset allocation and innovation investment. Finance trade Econ. 39, 95–110. doi:10.3969/j.issn.1002-8102.2018.12.008
Li, F., Yang, M., Wong, M. H., and Ballester, P. J. (2015). Improving AutoDock vina using random forest: the growing accuracy of binding affinity prediction by the effective exploitation of larger data sets. Financial Res. 34, 115–126. doi:10.1002/minf.201400132
Li, F., Xu, X., Li, Z., Du, P., and Ye, J. (2021). Can low-carbon technological innovation truly improve enterprise performance? The case of Chinese manufacturing companies. J. Clean. Prod. 293, 125949. doi:10.1016/j.jclepro.2021.125949
Li, P., and Ouyang, Y. (2020). Quantifying the role of technical progress towards China’s 2030 carbon intensity target. J. Environ. Plan. Manag. 64, 379–398. doi:10.1080/09640568.2020.1764343
Li, Q., Liu, J., and Liu, T. (2022). The impact of economic policy uncertainty on carbon-neutral development. Circulation Econ. China 36, 11. doi:10.14089/j.cnki.cn11-3664/f.2022.06.007
Li, R., Cui, Y., and Zheng, Y. (2021). The impact of corporate strategy on enterprise innovation based on the mediating effect of corporate risk-taking. Sustainability 13, 1023. doi:10.3390/su13031023
Li, Z., Liu, Y., and Yin, X. (2019). Analysis of amino acids in blood by combining zeolitic imidazolate framework-8-based solid phase extraction and capillary electrophoresis. Statistics Decis. 35, 30–37. doi:10.1016/j.jpba.2019.02.015
Liang, K., and Xie, H. (2019). Has policy uncertainty hurt the Chinese economy's long-term growth potential? - evidence from firms' innovative behavior. J. Central Univ. Finance Econ. 2019, 79–92. doi:10.19681/j.cnki.jcufe.2019.07.007
Lin, S., Xiao, L., and Wang, X. (2021). Does air pollution hinder technological innovation in China? A perspective of innovation value chain. J. Clean. Prod. 278, 123326. doi:10.1016/j.jclepro.2020.123326
Liu, G., Liu, Y., and Zhang, J. (2020). Economic policy uncertainty and portfolio allocation of Chinese listed companies: a discussion on the "financialization" trend of real enterprises. Econ. Q. 20, 65–86. doi:10.13821/j.cnki.ceq.2020.04.05
Ma, H., and Hao, D. (2022). Economic policy uncertainty, financial development, and financial constraints: evidence from China. Int. Rev. Econ. Finance 79, 368–386. doi:10.1016/j.iref.2022.02.027
Mohamed, M. M. A., Liu, P., and Nie, G. (2022). Causality between technological innovation and economic growth: evidence from the economies of developing countries. Sustainability 14, 3586. doi:10.3390/su14063586
Myers, S. C. (1977). Determinants of corporate borrowing. J. Financial Econ. 5, 147–175. doi:10.1016/0304-405x(77)90015-0
Nakajima, J. (2011). Time-varying parameter var model with stochastic volatility: an overview of methodology and empirical applications. Jouchi Nakajima, Available at: https://www.imes.boj.or.jp/research/papers/english/11-E-09.pdf.
Pata, U. K., and Fareed, Z. (2023). Quantifying the asymmetric and dependence structure between financial inclusion and energy efficiency: evidence from quantile methods. Energy Effic. 16, 16. doi:10.1007/s12053-023-10087-8
Phillips, P. C. B., and Perron, P. (1988). Testing for a unit root in time series regression. Biometrika 75, 335–346. doi:10.1093/biomet/75.2.335
Primiceri, G. E. (2003). Time varying structural vector autoregressions and monetary policy. SSRN Electron. J. 72. doi:10.2139/ssrn.352960
Rao, P., Yue, H., and Jiang, G. (2017). Economic policy uncertainty and firm investment behavior. World Econ. 40, 27–51. doi:10.19985/j.cnki.cassjwe.2017.02.003
Raza, S. M. F., Ali, I., Malik, M. Y., Ahmad, M., Abidin, S. Z. U., and Masood, S. (2022). Renewable energy consumption towards environmental sustainability: evidence of ekc hypothesis in the framework of economic growth and Co2 emissions. PalArch’s J. Archaeol. Egypt/Egyptol. 19, 1238–1253. Available at: https://archives.palarch.nl/index.php/jae/article/view/11177.
Shah, M. I., Foglia, M., Shahzad, U., and Fareed, Z. (2023). Corrigendum to ‘green innovation, resource price and carbon emission during the Covid-19 times: new findings from wavelet local multiple correlation analysis’ [technol. Forecast. Soc. Change, 184 (2022) 121957]. Technol. Forecast. Soc. Change 191, 122394. doi:10.1016/j.techfore.2023.122394
Shahzad, F., and Fareed, Z. (2023). Examining the relationship between fiscal decentralization, renewable energy intensity, and carbon footprints in Canada by using the newly constructed bootstrap fourier granger causality test in quantile. Environ. Sci. Pollut. Res. Int. 30, 4617–4626. doi:10.1007/s11356-022-22513-y
Song, Q., Xiao, L., and Qian, L. (2019). Economic policy uncertainty and corporate loan costs. Financial Res. 2019, 57–75. Available at: https://kns.cnki.net/kcms2/article/abstract?v=Zx5UZ-cNHTjyljNiTMuNbRIui-f12Llb_B3JArksQxTBXINk4syUqttZMIDR9kYDdJcqskIEVVd89eGFk0XOs87NGAyAibZoC1cY51GWPhhn7i502gIpgQ0Vrlt5K5Aq48W1iZ-y4Oo=&uniplatform=NZKPT&language=CHS.
Su, Ai, Tao, X., and Xiao, Y. (2015). Study on the path to break through the "Matthew Effect": based on the optimization perspective of resource allocation. J. Syst. Sci. 1-7, 1806. Available at: https://kns.cnki.net/kcms/detail/14.1333.N.20221117.1806.002.html.
Świadek, A., Gorączkowska, J., and Godzisz, K. (2021). Conditions driving low-carbon innovation in a medium-sized European country that is catching up–case study of Poland. Energies 14, 1997. doi:10.3390/en14071997
Tan, X., and Zhang, J. (2017). Channel analysis of economic policy uncertainty affecting corporate investment. World Econ. 40, 3–26. doi:10.19985/j.cnki.cassjwe.2017.12.002
Toparlı, E. A., Çatık, A. N., and Balcılar, M. (2019). The impact of oil prices on the stock returns in Turkey: a tvp-var approach. Phys. A Stat. Mech. its Appl. 535, 122392. doi:10.1016/j.physa.2019.122392
Valencia, F. (2017). Aggregate uncertainty and the supply of credit. J. Bank. Finance 81, 150–165. doi:10.1016/j.jbankfin.2017.05.001
Wang, H., Chen, Z., Wu, X., and Nie, X. (2019). Can a carbon trading system promote the transformation of a low-carbon economy under the framework of the porter hypothesis? —empirical analysis based on the psm-did method. Energy Policy 129, 930–938. doi:10.1016/j.enpol.2019.03.007
Wang, H., Yang, G., Ouyang, X., Tand, Z., Long, X., and Yue, Z. (2021). Horizontal ecological compensation mechanism and technological progress: theory and empirical study of Xin’an River Ecological compensation gambling agreement. J. Environ. Plan. Manag. 66, 501–523. doi:10.1080/09640568.2021.1990030
Wang, X., and Luo, Y. (2020). Has technological innovation capability addressed environmental pollution from the dual perspective of fdi quantity and quality? Evidence from China. J. Clean. Prod. 258, 120941. doi:10.1016/j.jclepro.2020.120941
Weeds, H. (2002). Strategic delay in a real options model of R&D competition. Rev. Econ. Stud. 69, 729–747. doi:10.1111/1467-937X.t01-1-00029
Wen, Q., and Zhang, T. (2022). Economic policy uncertainty and industrial pollution: the role of environmental supervision by local governments. China Econ. Rev. 71, 101723. doi:10.1016/j.chieco.2021.101723
Wu, G., You, D., Xiong, Y., Liu, X., and Li, X. (2019). The impact of environmental regulation on technological innovation and green total factor productivity: a moderating effect based on fiscal decentralization. J. Manag. Eng. 33, 37–50. doi:10.1186/s12968-019-0552-5
Xie, Z., Wu, R., and Wang, S. (2021). How technological progress affects the carbon emission efficiency? Evidence from national panel quantile regression. J. Clean. Prod. 307, 127133. doi:10.1016/j.jclepro.2021.127133
Xin, X., and Lv, L. (2021). Spatial differentiation and mechanism of technological innovation affecting environmental pollution in major cities in China. Geogr. Sci. 41, 129–139. doi:10.13249/j.cnki.sgs.2021.01.014
Yan, C. (2020). The development context and Future prospects of Modern economic growth Theory - and the defects of modern economic growth theory from the perspective of China's economic growth. Econ. Res. 55, 191–208. Available at: https://kns.cnki.net/kcms2/article/abstract?v=Zx5UZ-cNHThC2954c32bcHMO0DuonSsKOGG9NRJXC7QLGZe0dXSyT3PeEr1rHFDBw97ucJTWB_NFyepaIYGgYUHgAPsdJMvdjZoXStxZhM_j9KcIs7rQtVatzHwspJjRdRV-UmnIVdA=&uniplatform=NZKPT&language=CHS.
Yang, L., Han, Y., Jaffré, F., Nilsson-Payant, B. E., Bram, Y., Wang, P., et al. (2021). An immuno-cardiac model for macrophage-mediated inflammation in COVID-19 hearts. Tech. Econ. Manag. Res. 2021, 33–46. doi:10.1161/CIRCRESAHA.121.319060
Yang, X., Ran, Q., and Zhang, J. (2020). Urban innovation behavior, fiscal decentralization and environmental pollution. Industrial Econ. Res. 2020, 1–16. doi:10.13269/j.cnki.ier.2020.03.001
Yao, S., and Lin, Y. (2023). Economic policy uncertainty and corporate environmental investment. J. China Univ. Min. Technol. Soc. Sci. Ed. 25, 18. doi:10.20089/j.cnki.issn.1009-105x.2023.01.012
Yuan, Z., Tian, Z., Ting, W., Shujing, X., and Shuwang, Y. (2021). Effects of technological progress from different sources on haze pollution in China. Sustainability 13, 2730. doi:10.3390/su13052730
Zhang, H., and Yang, Z. (2020). The temporal impact of external shocks on inventory investment. Statistics Decis. 36, 139–144. doi:10.13546/j.cnki.tjyjc.2020.21.028
Zhang, Z. (2021). China and the World under the goal of carbon Peak and carbon neutrality: green low-carbon transition, green finance, carbon market and carbon border regulation Mechanism. People's Forum Acad. Front. 2021, 69–79. doi:10.16619/j.cnki.rmltxsqy.2021.14.008
Zhao, Y., Qiao, Y., Zhou, L., and Zhao, J. (2021). How emission reduction measures improve the financial performance of energy-intensive firms: the mediating role of carbon performance. Syst. Eng. 39, 14–24. Available at: https://kns.cnki.net/kcms/detail/43.1115.N.20210617.0845.002.html.
Zhu, B., Xu, C., Wang, P., Zhao, C., Wu, Z., and Song, L. (2021). Whether internal carbon pricing mechanism achieves both emission reduction and income increase. Account. Res. 2021, 178–192. Available at: https://kns.cnki.net/kcms2/article/abstract?v=Zx5UZ-cNHTg0WoXxSlkotfze5FMXienMHFuRFRrJcdIirCO13hKwFAhxkjBJd_hxV20CyxzJE1bRoE-k7Pox_Y2yKqID7Gp7W93LcUesdt-hDnRhhvFwbvFwhK9nPHBYAXLqOPRoLvo=&uniplatform=NZKPT&language=CHS.
Keywords: economic policy uncertainty, low-carbon economic transition, enterprise perspective of transformational innovation policy, enterprise innovation, financial crisis
Citation: Zhang L, Shao C and Wang J (2023) The time-varying effects of economic policy uncertainty and low-carbon economic transition on enterprise innovation in China. Front. Environ. Sci. 11:1208632. doi: 10.3389/fenvs.2023.1208632
Received: 19 April 2023; Accepted: 04 August 2023;
Published: 15 August 2023.
Edited by:
Mobeen Ur Rehman, Shaheed Zulfikar Ali Bhutto Institute of Science and Technology (SZABIST), United Arab EmiratesReviewed by:
Fahad Ali, Zhejiang University of Finance and Economics, ChinaQiying Ran, Xinjiang University, China
Fengshu Li, Ghent University, Belgium
Copyright © 2023 Zhang, Shao and Wang. This is an open-access article distributed under the terms of the Creative Commons Attribution License (CC BY). The use, distribution or reproduction in other forums is permitted, provided the original author(s) and the copyright owner(s) are credited and that the original publication in this journal is cited, in accordance with accepted academic practice. No use, distribution or reproduction is permitted which does not comply with these terms.
*Correspondence: Jia Wang, wangjia5651work@163.com