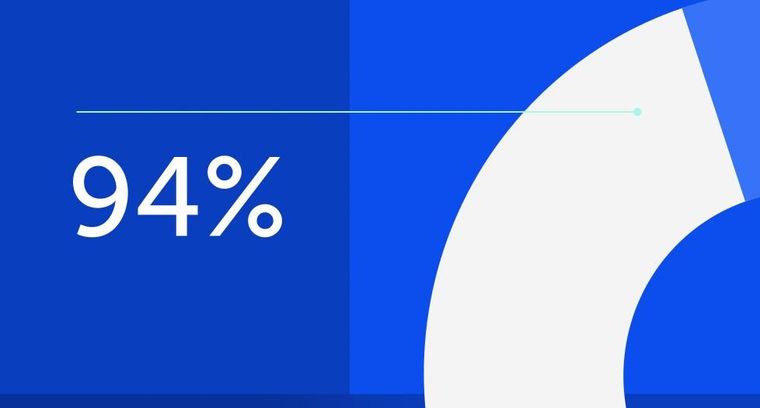
94% of researchers rate our articles as excellent or good
Learn more about the work of our research integrity team to safeguard the quality of each article we publish.
Find out more
ORIGINAL RESEARCH article
Front. Environ. Sci., 22 December 2023
Sec. Interdisciplinary Climate Studies
Volume 11 - 2023 | https://doi.org/10.3389/fenvs.2023.1205515
This article is part of the Research TopicInnovations in Climate ResilienceView all 6 articles
As the prospect of exceeding global temperature targets set forth in the Paris Agreement becomes more likely, methods of climate intervention are increasingly being explored. With this increased interest there is a need for an assessment process to understand the range of impacts across different scenarios against a set of performance goals in order to support policy decisions. The methodology and tools developed for Performance Assessment (PA) for nuclear waste repositories shares many similarities with the needs and requirements for a framework for climate intervention. Using PA, we outline and test an evaluation framework for climate intervention, called Performance Assessment for Climate Intervention (PACI) with a focus on Stratospheric Aerosol Injection (SAI). We define a set of key technical components for the example PACI framework which include identifying performance goals, the extent of the system, and identifying which features, events, and processes are relevant and impactful to calculating model output for the system given the performance goals. Having identified a set of performance goals, the performance of the system, including uncertainty, can then be evaluated against these goals. Using the Geoengineering Large Ensemble (GLENS) scenario, we develop a set of performance goals for monthly temperature, precipitation, drought index, soil water, solar flux, and surface runoff. The assessment assumes that targets may be framed in the context of risk-risk via a risk ratio, or the ratio of the risk of exceeding the performance goal for the SAI scenario against the risk of exceeding the performance goal for the emissions scenario. From regional responses, across multiple climate variables, it is then possible to assess which pathway carries lower risk relative to the goals. The assessment is not comprehensive but rather a demonstration of the evaluation of an SAI scenario. Future work is needed to develop a more complete assessment that would provide additional simulations to cover parametric and aleatory uncertainty and enable a deeper understanding of impacts, informed scenario selection, and allow further refinements to the approach.
Stratospheric Aerosol Injection (SAI), a proposed climate intervention strategy to mitigate anthropogenic warming, is not at present a widely accepted response to mitigating the impacts of climate change. However, the implementation of SAI at climatically relevant scales would pose security risks around the world (Nightingale and Cairns, 2014; Versen et al., 2021) warranting a deeper understanding of both the technology and its climate impacts. While there have been numerous advances in numerical modeling and laboratory studies of aerosols relevant to SAI, there are no widely accepted regulations or global treaties by which the international community might design or evaluate strategies for implementation (Grisé et al., 2021). A study by the National Academies of Sciences, Engineering, and Medicine (NASEM) identifies several research areas needed to understand SAI holistically, including assessment frameworks, before considering SAI as a viable strategy for intervening to mitigate the impacts of climate change (NASEM, 2021). The report highlights 13 specific areas of research that can be encompassed by three broad categories: context and goals for solar geoengineering research, which prioritizes areas that explore the “decision space” of research, development, and deployment options; impacts and technical dimensions, which aims to clarify the technical feasibility of various solar geoengineering strategies, their effectiveness, and their potential impacts; and social dimensions, which explores questions surrounding the social acceptability of solar geoengineering research.
If the deployment of SAI is ever seriously considered, policymakers will require tools to facilitate informed decision making in support of regional interests, geopolitical stability, and future regulations. A precedent for a decision making framework for such a multifaceted problem already exists for nuclear waste disposal, called Performance Assessment (PA) (Meacham et al., 2011). The similarities between radioactive waste disposal and climate change, and the opportunities for modifying and applying the tools developed in the radioactive waste disposal community to climate change has been suggested previously by McKinley et al. (2022). The PA for nuclear waste disposal is based on models that predict evolution of nuclear waste repository systems for thousands of years into the future and are very mature by comparison to those for climate change, however.
Projections of the long- and short-term climate impacts of a given emissions, mitigation, or intervention pathway are developed using either a Global Climate Model (GCM) (Raju and Kumar, 2020) or Earth System Model (ESM) (Kawamiya et al., 2020). There is a large body of research investigating SAI using GCM and ESM simulations and the subsequent quantification of their impacts across the earth system (e.g., see Tracy et al., 2022 for a recent review), but there is still need for the development of a quantitative decision making framework, as these impacts have been assessed across a wide range of SAI and emissions scenarios and generally, in a piecemeal way (Moore et al., 2021; NASEM, 2021; Smith and Henly, 2021; Tracy et al., 2022). For example, the Assessing Responses and Impacts of Solar climate intervention on the Earth system with stratospheric aerosol injection (ARISE-SAI; Richter et al., 2022) and the Geoengineering Large Ensemble (Tilmes et al., 2018; Geoengineering Large Ensemble, 2022) employ an annual feedback algorithm between the injection amount and the temperature response but each ensemble uses a different emissions scenario (SSP2-4.5 versus RCP8.5), and the initiation of the SAI begins at different points in the future, 2035 versus 2020. In addition to these large ensemble archives, there are numerous individual studies of different injection scenarios to understand the climate response to injection amount and location (e.g., MacMartin et al., 2017; Nalam et al., 2018), seasonal timing of the injection (e.g., Visioni et al., 2019; Lee et al., 2021), and regional injection scenarios (e.g., Caldiera and Wood, 2008; Robock et al., 2008; Kravitz et al., 2019; Lee et al., 2023), to name a few.
One of the logistical difficulties in the development of a comprehensive assessment of climate and climate intervention impacts is the computational expense to run fully coupled simulations of the earth system. GCMs and ESMs can produce terabytes of data for a single simulation and multiple simulations as part of an ensemble are required to develop a broad understanding of the impacts with uncertainty. This results in a volume of data that can be unwieldy to analyze and interpret. Furthermore, GCMs and ESMs output physically based diagnostics of the earth system, which may not be as interpretable to policymakers as the outputs from sector-specific models. Perhaps even a more substantial challenge to establishing a PA-like framework for climate intervention is the development of the goals of the intervention. In the case of nuclear waste repository systems, this was done in conjunction with scientists and United States Federal regulators over many years for a problem with a relatively small sphere of influence. Given that the climate change and climate intervention are global in nature and can impact many systems, the development of appropriate goals will be a substantially greater effort. Nonetheless, to facilitate decision making strategies for, and scenarios of, climate intervention there is a need to identify and provide decision makers with information on the impacts of greatest importance, as well as the potential impacts of each decision across multiple sectors (Jinnah and Nicholson, 2019).
Here, we develop a possible framework, leveraging PA, for evaluating climate intervention scenarios, focusing on SAI, against theoretical performance goals. Our goal is to outline the Performance Assessment for Climate Intervention (PACI) framework and demonstrate an assessment of regional climate responses, across multiple climate variables, using a set of performance goals, to a particular SAI strategy which enables a theoretical ranking (at regional scales) of those outcomes.
The United States Environmental Protection Agency (EPA) (United States Environmental Protection Agency, 1993) defines “performance assessment” in the context of radioactive waste disposal as:
“…an analysis that: (1) identifies the processes and events that might affect the disposal system; (2) examines the effects of these processes and events on the performance of the disposal system; and (3) estimates the cumulative releases of radionuclides, considering the associated uncertainties, caused by all significant processes and events. These estimates shall be incorporated into an overall probability distribution of cumulative release to the extent practicable.”
A PA is typically developed as one component of a repository license application. The regulations governing a PA, the features, events, and processes (FEPs) under consideration, and the final selection of models are iterated upon until both the applicant and regulator are confident that the technical basis is sound and defensible, and that the PA results illustrate the range of possible future outcomes (Galson et al., 2000).
Currently, the Waste Isolation Pilot Plant (WIPP) is the only deep geologic repository in the United States for long-lived radioactive transuranic waste. WIPP is owned by the United States Department of Energy (DOE) and is subject to waste containment requirements defined by Title 40 of the United States Code of Federal Regulations (CFR) §191.13. As required by 40 CFR 191.13, a PA framework was developed for the WIPP to demonstrate a reasonable expectation that the probabilities of cumulative radionuclide releases from the disposal system during the 10,000 years following closure will fall below specified limits (Meacham et al., 2011).
Though now closed, the Yucca Mountain Project (YMP) was a proposed deep geological repository storage facility for spent nuclear fuel and other high-level radioactive waste in the United States. Before the YMP was defunded in 2011, a Total System Performance Assessment (TSPA) was developed on behalf of the DOE to support the YMP site recommendation and license application process (Meacham et al., 2011). Like the WIPP, the YMP was subject to requirements defined by 10 CFR 63, which states (among other requirements) that there must be a reasonable expectation that for 10,000 years following disposal from all pathways, the “reasonably maximally exposed individual” would receive no more than the stated dose equivalent, and that releases of radionuclides to the accessible environment will not exceed specified limits.
The PA framework has previously been utilized for its ability to encompass the complexities and large timescales important to ensuring the integrity of the disposal facility and safety to the public and the environment. This framework has been applied beyond the nuclear waste disposal community, a generic nuclear waste disposal PA framework is summarized in Lowry (2021) (modified from Meacham et al., 2011). For instance, Lowry (2021) applied the PA framework to a geothermal energy project to identify pathways for reducing or eliminating underlying risks and though not a direct application of the PA, McKinley et al. (2022) outlines a risk assessment approach originating with the approaches and tools for radioactive waste disposal to the risk management associated with climate change, citing similarities between the two problems with respect to timescale, multidisciplinary nature, and need to enhance public understanding and political buy-in, among others. We follow by utilizing the historical development of the PA processes for both the WIPP and YMP to develop a preliminary framework supporting the application of a PA methodology to the problem of climate intervention. The original PA for nuclear waste disposal and modified PA for climate intervention as applied here are shown in Figure 1, and the development process is discussed in the following sections.
FIGURE 1. (A) PA Methodology for nuclear waste disposal (reproduced from Meacham et al. 2011). (B) PA methodology as outlined in (Meacham et al., 2011) modified for climate intervention. Areas where a PA framework focused on climate intervention may modify or differ from the PA framework applied to nuclear waste management are outlined in green.
The PA framework as applied in the WIPP case relies upon a range of input parameters and scenarios, including up to 3 million stochastically derived independent potential futures, to derive a response curve that meets minimum regulatory requirements to show compliance with regulatory limits on output releases (United States Department of Energy, 2019). These regulatory limits are de facto performance goals that have been codified in United States Federal regulations. EPA regulations require the DOE to provide sufficient confidence that the mean results are representative (United States Environmental Protection Agency, 1993).
The case of WIPP PA accounts for uncertainty in current knowledge of key input parameters (e.g., rock salt properties) by introducing distributions of values for these uncertain parameters (United States Department of Energy, 2019). For the latest compliance PA calculation, 69 independent variables were sampled to create 300 independent sets of inputs for the WIPP PA models. Additionally, the uncertainty associated with future events in and around the repository (e.g., the potential for inadvertent human intrusion via surface drilling into the repository) is accounted for by introducing stochastically applied events into the repository timeline. For each of the 300 independent input sets, 10,000 independent futures are simulated which differ by being punctuated by the stochastically determined events to generate a single complementary cumulative distribution function (CCDF) of releases for each input set. In sum, three million potential repository futures are simulated to form 300 CCDFs, and the mean CCDF is compared to regulatory release limits at specified probabilities as a way of showing compliance to the regulations (United States Department of Energy, 2019).
Ideally, a PACI framework designed for decision making (i.e., compliance with some external performance goal or regulation) would also have as its basis sufficient independent calculations of future states to provide confidence that the average result is in some sense representative of an expected future state. This is a challenge for the more complicated, computationally expensive, and intricate GCMs and ESMs, which require more computing power than the WIPP PA calculations for a single realization. Two obvious solutions are to reduce the confidence level required to show compliance or to increase the number of independent realizations. However, reducing the confidence level may be objectionable to both the regulator and to the public. Increasing the number of independent realizations would come at the cost of time and computing power but may ultimately be the more defensible approach.
The evaluation and assessment of the different climate pathways or climate intervention strategies is determined by the performance goal. Although the WIPP PA assesses a specific approach to modeling the potential radionuclide releases from a nuclear waste repository against release limits defined by federal regulation, we can consider here a PACI framework that, in its early stages, can be flexible enough to be used to assess the impacts of different pathways (e.g., different emissions or intervention scenarios) in an ESM against a performance goal (or multiple performance goals). A PACI framework may also be developed to focus on a specific aspect of the different pathways, such as SAI, and compare multiple approaches for the relative impacts on specified output measures, for example, surface temperature targets.
For the purposes of this discussion, we focus only on intervention scenarios relevant to SAI. Climate intervention and SAI have no such limits, yet, and there are several considerations for the development of the performance goal(s). The establishment of performance goals is non-trivial and for a meaningful PACI framework would require a dedicated effort to integrate the varied social and political factors (which will vary globally) that must be considered. Ultimately, any assessment under consideration must be both defensible and complete. Any potential future regulation is likely to be adjusted as it is informed by both technical modeling and socio-political concerns; therefore, a useful PACI framework will anticipate and plan for iterative improvements.
Once performance goals have been identified, implementing them in the PACI framework requires additional considerations that are based on the limitations of existing models and tools. These considerations include, but are not limited to, the ability of the model to resolve the output at the resolution needed to address the performance goal, the reassessment needs due to anticipated monitoring or anticipated realities of implementation, incorporation of new uncertainties, and overall model improvements.
First and foremost, performance goals must be designed within the scope of current model capabilities, such as model resolution. If a model does not sufficiently resolve processes at the relevant spatial and temporal scale for a performance goal, it will be difficult to provide an assessment with sufficient confidence. For example, if a performance goal is defined as some minimum fraction of land area that experiences a specified threshold of regional temperature differences, then model output must be available in the form of temperature differences on a regional scale. If performance goals are designed in such a way that they extend beyond the capabilities of current models, then this highlights a research and development priority.
Performance goals must also be designed to balance the needs for stability but also reassessment and reevaluation. Changes to the performance goal after its initial codification risks loss in confidence from the regulator and the public. However, this should be considered alongside the benefits of periodically reassessing the performance goal. Reassessment may become necessary as the time from the initial assessment and the actual implementation of the SAI scenario increases. An initial assessment of performance goals could benefit from a range of goals to use for a range of implementation dates. The longer lapse in time before implementation may require more lax performance goals due to progressively worsening climate conditions. Reassessment of the performance goals may also be necessary as part of a continued monitoring plan once an SAI scenario has been implemented. Observations made as part of such a monitoring program would be used to assess the performance of the climate system after implementation and, in part, demonstrate whether the initial performance goals are reasonable. This may seem contradictory to the previous statement regarding reassessing the goals over time; however, a possible strategy would be to implement multiple goal stages: preliminary goals prior to implementation and revised goals to be implemented sometime after implementation and some observation period.
Another key factor in the development of the performance goals and the selection of the models for assessing against that goal, is that the models should be selected and constructed with their ultimate use in mind. Using a model that was built for a different application could introduce additional epistemic risk to the assessment as the features and processes included in the model may not be inclusive of those necessary to make an informed, actionable decision. When a performance goal is decided, significant effort must then be undertaken to determine whether any existing models (and their associated uncertainties) are adequate for assessing the performance goal. A new model that focuses on those features and processes relevant to the performance metric may need to be constructed or adequacy studies on existing models may be required.
Using a PACI framework that incorporates uncertainty into the model inputs and key outputs, model and parameter uncertainties can be calculated which identify the relative contributions of individual models or parameters to the observed range of outputs. This information can be valuable for identifying directions for further model development or areas of future research with a focus on reducing uncertainty where possible. Uncertainty reduction could increase the confidence in the models used, as well as the relative confidence in the range of observed outputs.
The WIPP PA framework is based in part on a series of computational models used to represent process models that were identified to be impactful and relevant to the repository system and, ultimately, the radionuclide releases calculated as the key output measure. The process for identifying key FEPs used to represent the system is called a “FEPs screening” (United States Department of Energy, 2019). The FEPs screening process is used to determine which features, events, and processes should be represented in the PA. A comprehensive FEPs list is first derived which contains any feature, event, or process which could potentially impact the system, regardless of potential significance. The initial list of FEPs is then assessed in the context of both regulation and performance goals. This comprehensiveness is used to demonstrate that all possible impacts have been investigated (Galson et al., 2000).
A FEP can be “screened-in” (included in the assessment) or “screened-out” (excluded from the assessment) based on potential consequence to the system and the probability of occurrence during the timeframe of interest. For example, a process that would not have an appreciable impact on the output measure of interest would be screened-out, as would an event that had a very low probability of occurrence and low consequence. FEPs may also be screened-in or out based on a regulation. The FEPs screening process also includes an evaluation of the level of detail required for the implementation of each listed FEP. This level of detail should be informed by uncertainty and sensitivity analysis, and the importance of each FEP is judged relative to its impact on defined performance goals. By screening in more FEPs, the modeling and simulation components of the PA framework become more complicated and computationally expensive. Proceeding with the minimum number of FEPs necessary to provide a robust computational framework that includes all key aspects of the system is prudent.
For nuclear waste repositories, a master list of FEPs has been developed by the Nuclear Energy Agency (NEA) that can serve as an initial list for a PA framework applied to climate intervention (Nuclear Energy Agency, 2019). From this master list, the FEPs screening process can be used to derive a smaller list of FEPs relevant to the new system. The resulting FEPs list can then be used as a basis for determining which process models are important for inclusion in the PA. Initially, the scope of potential FEPs must also be broadened to account for the differences between nuclear waste repositories and climate intervention strategies, and to account for the specific performance goal/metric under consideration.
Although a formal FEP screening process may not have been followed, in the case of a PACI framework in which the ESM outputs already exist, the FEPs have essentially already been screened; whichever FEPs are included in the ESMs were screened-in during the model development process and all other FEPs have been screened-out. Although the screened-in FEPs are included in the ESM documentation, formalizing the FEPs documentation and their screening status is beneficial in identifying and clarifying the scope of a the PACI framework. For example, the FEPs documentation could provide a baseline to update for cases where there is a change to the definition of the system or timeframe to be modeled. Or, if the scope of the calculation has changed from utilizing all components of the ESM to only a subset of components of the ESM (e.g., just the atmosphere), the FEPs list could be pared down.
Given the complexity of ESMs and the range of possible outputs, a simple performance goal or metric is not likely to encompass the important contributions of the entire system, nor the range of outputs generated from a range of inputs based on uncertainty. For the relatively complex WIPP repository system, the performance metric takes the form of two specified radionuclide release levels, each at a specified probability of occurrence (United States Environmental Protection Agency, 1996). Similarly, given the complexity and uncertainty associated with climate modeling, a new PACI framework might also consider a probabilistic approach, where the likelihood of achieving a specific performance goal is stated in terms of percentages. Careful choice of performance goals that are achievable and relevant is important and will require a global effort in order to encompass socio-political concerns which, of course, vary at global to local scales. While a regulatory-specified goal may not be currently available, the probability-based performance goals defined may illustrate a subset of the type of goals that could be used as a tool for making go, no-go decisions for implementing a specific SAI scenario.
The definition of the system of interest is another key component of the PA framework. In the case of WIPP PA, the system is defined as a specific area surrounding the repository in southeast New Mexico for 10,000 years following closure. For a PACI framework, the system would be defined as global in scale as SAI has global impacts, even when implemented regionally (e.g., Caldiera and Wood, 2008; Robock et al., 2008; Kravitz et al., 2019; Lee et al., 2023), and could span any specified time period. The system definition draws a clear distinction, at a broad level, for what is included in the PA framework and what is left out. This is a key step that should occur prior to FEPs screening process.
Uncertainty quantification is an important part of the PA framework that focuses on quantitatively characterizing uncertainties in the model results. Uncertainty arises from aleatoric (i.e., stochastic) and epistemic sources, as well as from the model itself. Uncertainties associated with model parameters can be investigated when distributions of potential model parameters are implemented in model realizations, such as in the WIPP case described briefly above.
Parameter sensitivity can be used to identify the uncertain input variables that have the greatest impact on the observed range of output and similarly, the variables that have low impact can also be identified. The process of identifying impactful model parameters allows for the opportunity to focus research and development (e.g., additional laboratory or field experiments) on reducing the uncertainty for the most impactful parameters to reduce the overall uncertainty in modeling results. In the PACI case described here, the GLENS results are not based on ranges of model parameters. Across the GLENS ensemble members only the initial temperature conditions and injection amounts vary (Tilmes et al., 2018). As such, a parameter sensitivity analysis is not possible for the present work. However, there is existing work on assessing uncertainty in stratospheric aerosol injection across models, scenarios, and model parameterizations (Kravitz and MacMartin, 2020; Visioni et al., 2021; Laakso et al., 2022).
The FEPs development process for the WIPP PA was used as a template to guide the development process for a preliminary FEP list for PACI. The initial PACI evaluation is performed using model outputs from the GLENS archive (Tilmes et al., 2018) which were simulated using the Whole Atmosphere Community Climate Model (WACCM) (Mills et al., 2017). However, ESMs generally contain similar processes and parameterizations across their atmospheric models, and so the Energy Exascale Earth System Model (E3SM) (Golaz et al., 2019) was selected as a starting point for an initial investigation into applying the principles underlying the FEP development process for WIPP PA to climate intervention, with a focus on the atmosphere component called the E3SM Atmospheric Model (EAM). E3SM is the DOE’s state-of-the-art ESM which simulates and couples processes within the different components of the earth system (e.g., atmosphere, land, ocean, and sea ice) (Golaz et al., 2019). Using EAM as an analog of WIPP FEPs, the EAM consists of a pre-defined set of process models and potential scenarios. The process models are those processes that are included in the EAM model, and the scenarios take the form of user inputs into the system.
The initial FEPs list was developed from existing E3SM and Community Earth System Model (CESM) documentation (Golaz et al., 2019; Rasch et al., 2019; Wang et al., 2020). Since E3SM was branched from CESM (Golaz et al., 2019), some of the details of the implementation of process models are not directly documented for E3SM but are included in implementation documentation for CESM (Garcia et al., 2007; Neale et al., 2010; Hurrell et al., 2013). Any feature, event, or process that is indicated as being implemented in the EAM was included in the initial FEPs list (Table 1). This list includes only those FEPs described in the relevant literature; it does not include a discussion of potential FEPs that are not modeled in E3SM, and which would be considered screened-out. An investigation into screened-out FEPs is planned future work.
The process models included in E3SM were classified as either a Feature or a Process. Events such as volcanic eruptions or anthropogenic emissions, take the form of user inputs and have not yet been itemized. Classification of FEPs assists in assessing and developing comprehensiveness and allows organization according to different keywords or criteria (Nuclear Energy Agency, 2000). Figure 2 is an example classification for a sample of FEPs screened-in to E3SM. The FEP Category column of Table 1 contains a basic level of classification for the FEPs derived from the EAM documentation.
After classification, FEPs are typically examined individually to determine whether they should be screened-in or screened-out. For the ESM evaluated here, the FEPs were screened during the development of the model. The features, events, and processes that were determined to be important to an Earth-scale environmental model were essentially screened-in during the development of the model, and those determined not to be important were screened-out.
A scenario is a combination of FEPs (Galson et al., 2000). The scenario development exercise ensures a comprehensive consideration of the possible future states of the system and identifies scenarios that could potentially impact the system (Swift et al., 1999). Numerous SAI scenarios have been modeled, and there are several major modeling efforts associated with SAI. Most notable are the Geoengineering Model Intercomparison Project (GeoMIP) (Kravitz et al., 2011; Kravitz et al., 2013; Kravitz et al., 2015), GLENS (Tilmes et al., 2018), and the more recent ARISE-SAI (Richter et al., 2022). Each of these archives provides simulation output from idealized, annual injection scenarios of SO2 into the stratosphere. As previously mentioned, in addition to these large model archives, there are also simulations aimed at addressing different aspects of SAI deployment scenarios. For instance, there has been research into the climate response to varying injection rates of SO2 seasonally (Visioni et al., 2019) and targeted SAI in polar regions (Lee et al., 2023). However, these deployment scenarios and the ultimate process of developing and assessing them must also consider unplanned events to capture the aleatory uncertainty. For any given scenario that may be explored, such as the politically relevant scenarios designed and outlined in Lockley et al. (2022), it will be important to include the impact of disruptions or unplanned events on the scenario.
There has been some research simulating disruptions to the deployment scenario to date, such as the simulations of the climatic response of interruptions of a deployment scenario due to potential “technological failures” and intermittent volcanic eruptions in Jackson et al. (2015), and simulations of the “termination shock,” which are often simulated with a sudden and dramatic end to the deployment as in some of the GeoMIP scenarios (e.g., Kravitz et al., 2011), but the addition of the uncertainty from interruption to deployment has not often been incorporated into the simulation plan. For instance, the GLENS archive does not include a subset of simulations that simulate a series of high probability interruptions to the scenario as is done in Jackson et al. (2015). However, capturing uncertainty relevant to deployment operations has not been the intended goal of many of these simulation studies and therefore it makes sense that it was not included in the design.
A list of disruptive scenarios is outlined in Table 2. These scenarios may have downstream impacts and increase uncertainty and thus require further assessment. While there are an essentially infinite number of possible future states of the system, the scenario development process must generate a finite number of scenarios that are representative of the range of potentially relevant futures (Swift et al., 1999). Under a WIPP PA framework, the developed scenarios would then be screened using the same criteria defined for the general FEPs list (Galson et al., 2000).
In this section we describe the methods for defining performance goals for an SAI scenario from a technical point-of-view (independent of socio-political influence), as well as those for evaluating the scenario against the performance goals. Since SAI is proposed as a climate intervention method, we aim to identify evaluation metrics which assess a particular SAI scenario against the alternative, an emissions pathway without SAI. This frames the impact evaluation as a risk-risk assessment, as has been suggested by others such as Keith (2017) and Felgenhauer et al. (2022). While this is the approach taken in this implementation of PACI, there are other principles and approaches which could be used to define the assessment (e.g., do no harm) and should be explored in future implementations. In an actual implementation of PACI in which decision makers are using this tool, multiple risks and influences should be addressed and appropriate levels of precaution considered (Wiener, 2002).
To demonstrate an assessment against a set of defined performance goals, the GLENS scenario was selected. Other SAI simulation archives were considered, such as ARISE-SAI and GeoMIP, but ultimately not included to simplify the demonstration. The GLENS injection scenario was designed for achieving specified temperature targets, where increasing temperatures were reduced using a feedback algorithm which tailored the aerosol injection into the stratosphere based on the temperature response relative to the target temperatures. Aerosols were injected into the stratosphere at four latitudes around the globe (30°N, 30°S, 15°N, and 15°S) at approximately 5 km above the tropopause. To use the same terminology as the PA framework, the GLENS simulations were targeting three performance goals which the aerosol injection was designed to achieve a constant; 1) surface temperature (T0), 2) interhemispheric temperature gradient (T1), and 3) the equator-to-pole temperature gradient (T2), all relative to 2020 conditions. The 2020 conditions were derived from the RCP8.5 control simulation ensemble averages from 2015 to 2025. Tilmes et al. (2018) describes the ensemble performance relative to these performance goals, as such, we do not evaluate against the original GLENS performance goals here. Knowing that any scenario and simulation archive may be designed with different performance goals in mind, this methodology is demonstrative of how assessments could be developed.
Publicly available datasets were analyzed in this study from the GLENS archive and the data can be found in the Climate Data Gateway at NCAR at https://www.earthsystemgrid.org/dataset/ucar.cgd.ccsm4.GLENS.html. From the GLENS archive, we selected the following monthly model outputs: mean surface temperature, minimum surface temperature, maximum surface temperature, mean precipitation, maximum precipitation rate, soil moisture (10 cm), surface runoff, and solar radiation flux. In addition to this directly available output from the GLENS archive, we calculated the Standard Precipitation Index (SPI) independently using the climate-indices Python package (Adams, 2017). SPI is a precipitation-based meteorological drought index (McKee et al., 1993) which can be used to characterize different types of droughts, depending on the time scale. The input dataset can be aggregated at 2–3 months to indicate agricultural drought, 2–6 months to represent streamflow, and 5–24 months to signify meteorological drought that impacts groundwater levels (Lloyd-Hughes and Saunders, 2002). SPI values are classified as: extremely dry (≤−2.00), severely dry (−1.99 to −1.50), moderately dry (−1.49 to −1.00), mildly dry (−0.99 to 0.00), mildly wet (0.00–0.99), moderately wet (1.00–1.49), severely wet (1.50–1.99), and extremely wet (≥2.00) (McKee et al., 1993). There have been several studies of hydrological impacts of SAI but few on drought and these have been regionally focused (Abiodun et al., 2021; Alamou et al., 2022). Using 2010 to 2020 as the drought calibration years, SPI is calculated globally from a gamma distribution of total monthly precipitation and aggregated at the 24-month time scale to understand the risk of meteorological drought impacts on the groundwater system.
As previously mentioned, performance goals across the earth system could vary widely, making defining performance goals a difficult task, particularly when incorporating socio-political influences. Some of the global regulations and definitions for performance goals may come from existing treaties, such as those governing ozone depleting substances like the Montreal Protocol (Bhasin et al., 2022). However, the impacts of climate intervention will also be felt on local and regional scales and performance goals at these higher-resolution spatial scales may vary due to regulations from their respective country, geopolitical interests, and local community desires. Additionally, performance goals will be prioritized differently by different regions. For instance, a land-locked nation may not prioritize the impacts and risks associated with sea level rise and small island nations may not prioritize the impacts and risks of alpine snowpack loss. If the impacts of SAI are demonstrated to influence tropospheric PM2.5 (e.g., Eastham et al., 2018), the EPA in the United States may apply existing regulatory limits. In addition to existing regulations, performance goals could be driven by geopolitical and societal factors, such as lending more weight towards the performance goals of either low emission countries or nations who may experience the worst impacts of climate change.
Ferraro et al. (2014) developed a probabilistic method for assessing whether the risk of SAI increases or decreases relative to the risk of climate change, which they called a risk ratio. However, they exclusively looked at annual mean temperature and precipitation. We expand on the assessment and methodology in Ferraro et al. (2014) by assessing model output metrics beyond mean temperature and mean precipitation, calculating the risk ratio at monthly timescales, developing performance goals that include both upper and lower thresholds, and including uncertainty across multiple ensemble members.
We define the performance goals for each variable (mean surface temperature, minimum surface temperature, maximum surface temperature, mean precipitation, maximum precipitation rate, soil moisture (10 cm), surface runoff, SPI and solar radiation flux) in Table 3. The performance goals defined in this demonstration do not reflect any specific socio-political influences, which would be required for a real-world implementation of PACI. We consider the performance goal for a given environmental variable to be a combination of the monthly mean plus or minus 1σ, as in Ferraro et al. (2014), or some cases a fraction of 1σ. The mean and σ for the reference period are calculated for each month from the years 2010 through 2019, this reference period is the same across all performance goals and is calculated for each model grid point. As an example, for the monthly mean surface temperature, the performance goal is defined as an upper threshold, meaning that for any grid cell, a value of Tmean that is greater than μ + 1σ would be out of compliance with the performance goal.
TABLE 3. Performance goals for each climate metric from the GLENS model output and SPI which was calculated independently from the model run.
From the difference in the simulation and the performance goal limit, we calculate empirical cumulative distribution functions of the exceedance magnitudes at each grid point over the duration of interest (2020–2029 and 2020–2099) for both the control and SAI simulations. The probability at a difference of zero represents the probability of exceedance of the performance goal. In the case of a performance goal which is an upper threshold, p is the probability of exceedance >0, and for a lower threshold, p is the probability of exceedance <0.
As in Ferrero et al. (2014) we define the risk ratio as:
Where, pSAI and pRCP8.5 are the respective probabilities of exceedance of the performance goal limit (ratio of points exceeding the limit within a given region/timeframe among ensemble members) for the SAI simulations (referred to as the feedback simulation in GLENS literature) and control simulations (the RCP8.5 emissions scenario). A risk ratio value greater than 1.0 therefore means that the RCP8.5 scenario has a lower risk of exceeding the performance goal and a risk ratio less than 1.0 means that the SAI scenario has a lower risk of exceeding the performance goal.
The performance goal(s) for each variable are defined in Table 3. Across these variables, we explore a few alternative methods in the definitions as we believe that this reflects the reality of a potential assessment. While most performance goals are either an upper or lower threshold defined by μ + 1σ, the addition on ±1σ is meant to reflect the natural climate variability within the system. Some variables, such as mean precipitation and maximum precipitation rate, however have lower thresholds that are some fraction of σ. This is to represent the possibility that for precipitation, there may be a lower tolerance to a decrease in precipitation and thus a more restrictive limit on the performance goal is desired. SPI is unique, in that instead of a variation of σ, the performance goal is targeted to be within one index level (±0.5) of the mean. Lastly, the performance goal for surface runoff is defined as the mean.
To understand the short-term and long-term impacts we evaluated the risk ratio for over two separate time periods, for the first 10 years of deployment (2020–2029) and for the full duration of deployment through the end of the century (2020–2099). We focus on ensemble members 001–003 and 021 since these are the four members that were run through 2099 and enable a comparison of the long-term impacts through the end of the century. As noted in Tilmes et al. (2018), due to some instabilities in simulating RCP8.5, not all the simulations reach the year 2099. Simulations 001, 002, and 021 from the RCP8.5 control set only extend through 2097. Years where there are no data for a single simulation are therefore excluded from the assessment. Finally, risk ratios for each performance goal are aggregated by region. Regions are defined by the IPCC WG1 Atlas from the AR6 Report (Iturbide et al., 2020). The regional risk ratios are presented alongside the cross-ensemble standard deviation for each region.
Having outlined the PACI framework and defined a set of performance goals, the GLENS SAI strategy was evaluated against those performance goals with the aim of demonstrating an assessment of regional climate responses which enables a theoretical ranking of the outcomes and, ultimately, decision making.
There are several trends visible in the geographic distributions of the risk ratios for the GLENS SAI scenario (Figures 3, 4). Across the temperature variables (mean, maximum, and minimum), in the first decade of deployment (2020–2029), SAI will have lower risk of exceeding the temperature goals relative to the RCP8.5 scenario. However, there are still many regions, in particular over the southern hemisphere oceans, the Arctic, and Antarctic, where the risk ratio is greater than 1.0 and the RCP8.5 scenario has lower risk of exceeding the temperature goals than the SAI scenario. Over the course of the deployment, and through the end of the century, the risk ratio for mean, maximum, and minimum temperature over most of the globe falls below 1.0, with some risk ratios over the oceans (near latitudinal bands 60°S and 60°N) remaining close to 1.0. GLENS was designed to reduce global surface temperatures, and though the temperature performance goals in Table 3 differ from the GLENS designed targets (2015 versus 2020 decadal averages), the SAI scenario still has a lower risk of exceeding the performance goals relative to the RCP8.5 scenario.
FIGURE 3. Ensemble average risk ratios for 2020–2029 (A–K) and 2020–2099 (B–L) for mean surface temperature (A, B), maximum surface temperature (C, D), minimum surface temperature (E, F), mean precipitation (G, H), maximum precipitation (I, J), and meteorological drought (SPI) (K, L) against the performance goals listed in Table 1. The color gradients are centered around a risk ratio of 1.0. Blues are risk ratios below 1.0 and thus the SAI scenario has performed better against the performance goal. Reds are risk ratios above 1.0 and thus the RCP8.5 scenario has performed better against the performance goal.
FIGURE 4. Ensemble average risk ratios for 2020–2029 (A, C, E) and 2020–2099 (B, D, F) for soil moisture (10 cm) (A, B), surface runoff (C, D), and solar flux at the surface (E, F) against the performance goals listed in Table 1. The color gradients are centered around a risk ratio of 1. Blues are risk ratios below 1.0 and thus the SAI scenario has performed better against the performance goal. Reds are risk ratios above 1.0 and thus the RCP8.5 scenario has performed better against the performance goal.
Unsurprisingly, the risk ratios for precipitation have more spatial variability and uncertainty than the risk ratios for the different temperature performance goals and the risk of exceeding the performance goals is not clearly reduced for either scenario. Risk ratios for both mean and maximum precipitation for the first decade of deployment are very close to 1.0. Through the end of the century, risk ratios for the mean and maximum precipitation do trend towards <1.0. But this is likely because, under an RCP8.5 scenario, the atmosphere is warmer and capable of holding more water vapor, resulting in more precipitation. The SAI scenario would reduce temperatures, reducing the capacity for the atmosphere to hold water, and thus reduce precipitation or maintain values close to the climate variability of 2015.
In the first decade of deployment, the performance goals for meteorological drought are best achieved through the RCP8.5 scenario. However, for much of the land surfaces through the end of deployment, the risk ratio for meteorological drought is generally below 1.0. For surface runoff, during the first decade of deployment risk ratios are generally close to 1.0. Through the end of deployment though, the risk ratios are generally above 1.0, meaning that the RCP8.5 scenario is at a lower risk of exceeding the 2015 mean relative to the SAI scenario.
Lastly, the risk ratio for the total solar flux at the surface is much closer to 1.0 during the first decade of deployment and, for most regions, increases to above 1.0 through the end of the century. This is not surprising as the purpose of injecting aerosols into the stratosphere is to reduce incoming solar radiation to reduce temperatures but is worth evaluating as it may impact agricultural and renewable energy sectors. Based on the performance goal and subsequent risk ratios, the RCP8.5 scenario would have lower risk in achieving the performance goal.
While the spatial distributions are valuable as they demonstrate the higher resolution variability across each risk ratio, it is still difficult to interpret and make informed decisions based on the spatial distributions and projections of risk across multiple variables. In order to distill the information, the risk ratios and cross-ensemble means over the land surface for a selection of IPCC regions (N.W. North America, South-American-Monsoon, N. Europe, W. Southern-Africa, Russian-Arctic, S. Asia, and S. Australia) are shown in Table 4. The results for all 46 regions are available in the Supplementary Table S1. From the regional means and uncertainty, it is possible to begin weighing tradeoffs for a region without being overwhelmed by the spatial and temporal variability.
TABLE 4. Ensemble mean risk ratios and cross-ensemble uncertainty (in brackets) over regional land surfaces for 7 IPCC regions.
For instance, for N.W. North America within the first decade of deployment, the risk of exceeding the performance goals across all the metrics is higher for the SAI scenario. However, out of nine climate metrics evaluated across N.W. North America, six suggest a lower risk of exceedance of the performance goals under the SAI scenario relative to RCP8.5 scenario through the end of the century. The remaining regions shown in Table 4 share similar patterns across the temperature performance goals and their risk ratios to that of N.W. North America. In general, the risk ratios across the temperature-based performance goals are >1.0 and therefore, the risk to exceeding the performance goals is lower under the RCP8.5 scenario during the first decade of the SAI deployment but through the end of the century, the risk to exceeding the performance goals is lower under the SAI scenario. For mean and maximum precipitation, based on these performance goals, none of the regions listed in Table 4 have risk ratios that would suggest that the SAI scenario is the more favorable of the two pathways during the first decade of deployment, and few that would suggest SAI through the end of the decade, and only for maximum precipitation, never for mean precipitation.
Dividing the data in a way that demonstrates both the short- and long-term risks will also highlight the potential risk for perceived failure (Keys et al., 2022). If deployment is pursued and the risk ratios for a region are very close to 1.0 during the first decade of deployment, this would suggest that the climate impacts of the SAI scenario versus the RCP8.5 pathway are likely so similar that they may be indistinguishable in achieving the performance goal. Regions with very low risk ratios, however, are at lower risk of exceeding their performance goal limits and thus may highlight regions which may be more interested in pursuing the SAI scenario over the RCP8.5 pathway.
Assessment tools from the nuclear waste disposal community have been previously proposed for application to address risk management for climate change (McKinley et al., 2022). The needs for the PA for nuclear waste disposal share several commonalities with the needs for the assessment framework called for climate intervention. Key components of a PA framework include identifying performance goals, identifying the extent of the system under study, and then identifying which FEPs are relevant and impactful to calculating model output for the system given the performance goals identified. At that point, the performance of the system (including various forms of uncertainty) can be evaluated against the performance goal (Meacham et al., 2011). The regulations governing a PA, the FEPs under consideration, and the final included models are iterated to build confidence that the technical basis is sound and defensible, and that the PA results illustrate the range of possible future outcomes (Galson et al., 2000). Any potential future regulation governing SAI is likely to be adjusted as it is informed by both technical modeling and political concerns; therefore, a useful PACI framework will anticipate and plan for iterative improvements. The introduction and results of this paper outline a possible path forward for developing such a framework for climate intervention and show a preliminary implementation.
With a set of performance goals (Table 3) and results from the evaluation of those performance goals (Figures 3, 4; Table 4), we build on previous research to develop an assessment strategy. Since an existing simulation archive was utilized, key components of the PA framework were not included in this instance (e.g., model uncertainty, parameter sensitivity, and aleatory uncertainty). What this research does allow, however, is an initial discussion of what evaluation metrics should be considered and what uncertainty may be the most impactful. This preliminary framework shows a mechanism to initially address whether the outcomes of an SAI strategy are within the stated goals or regulations and how those outcomes compare to the alternative of an emissions pathway in a risk-risk framing. Based on a set of value criteria, it is then possible to begin to make informed decisions. The risk-risk framing of the impacts of SAI has been called for in several reports (Keith, 2017; Aldy et al., 2021; NASEM, 2021). Some suggest that the risk-risk framing of impacts leads to a more comprehensive analysis, improved understanding for policymakers, and a more effective method for maximizing the benefits in decision making (NASEM, 2021; Felgenhauer et al., 2022). The work here however, is only a first step as there is still more information that needs to be considered and included as part of a comprehensive assessment strategy. A more comprehensive PACI framework will enable a deeper understanding of impacts and scenario selection and allow further refinements to the approach. Several avenues for future work and expanding the assessment are discussed below.
The selection of an existing model archive and SAI scenario limits the uncertainty that can be addressed. The GLENS scenario is simulated from a single climate model, WACCM. As such, the selection of GLENS only allows us to evaluate the uncertainty in the initial conditions. Cross-model comparisons for instance, would enable discovery of the extent of uncertainty in the climate response to SAI due to model selection in the representation of aerosol microphysics, a key uncertainty in simulations of SAI (Kravitz and MacMartin, 2020). Future work and implementations of scenario evaluation should address the highest importance FEPs and key areas of uncertainty associated with those FEPs (model uncertainty, parameter sensitivity, and aleatory uncertainty). However, ESMs are computationally expensive to run, and they generate large volumes of data. Rather than run many simulations through the end of the century, simulation plans could first assess near-term impacts, in the first 5–10 years of deployment, and run only a subset of simulations through the end of the century as is done in the GLENS archive. An alternative approach to running many shorter simulations to acquire more data for decision making may be to develop computationally efficient surrogate models to generate estimates of the model output. Machine learning models have the potential to reduce the time to solution for expensive simulations (Weber et al., 2020) or to highlight areas of uncertainty in existing simulation realizations (Gal and Ghahramani, 2016; Watson-Paris, 2021). Regardless of the mechanism for simulating the climate output, future work within such a framework should focus on policy-relevant scenarios. However, few simulations of policy-relevant scenarios exist, though several have been recently proposed by Lockley et al. (2022) and MacMartin et al. (2022).
The IPCC regions used here cross geopolitical boundaries. While the IPCC regions themselves are not ideal for consideration of the most likely pathways for deployment and decision making, they were selected to discourage any interpretation of the scenario for geopolitical purposes as GLENS is an idealized SAI scenario. This methodology was intended as a test case for assessment and, in doing so, we have chosen an SAI scenario that was initiated in the past (2020) and is unlikely to be applicable to today. Moving forward, the boundaries for the assessment should focus on those that impact decision making, such as at the sub-national scale (e.g., drainage basins, county-level, recreational border areas, etc). In addition to moving towards more relevant boundaries, future assessments should incorporate population projections to understand the impacts against population density with time. Furthermore, the risk ratios for the environmental variables that are directly output by the model are useful but do not yet enable a quantification of the impacts on a specific sector. Developing composite and weighted risk metrics where the environmental variables are aligned and grouped by sector could be a first step towards applying this approach in a sector-based analysis.
Additionally, the choice of performance goals may ultimately be decided by some combination of community engagement and consensus, intergovernmental panels focused on the socio-political impacts of climate change and climate intervention and scientific research. We look to the history of the EPA’s establishment of acceptable release criteria for nuclear waste disposal, in which input was taken from public meetings, as well as from the scientific community, as an example (Rechard, 1999). The scientific community can provide key information on the relevancy of a performance goal toward addressing scientifically significant measures of climate change. As a first step, the models used in an assessment should be thoroughly tested against a range of performance goals for various types of model output. The results of those tests could then be used to support informed selection of the performance goals(s) with broader governmental and public input.
Demonstrating the capability and credibility of ESMs and GCMs provides confidence in the computer simulation results if they are to be used for decision making (Kaizer et al., 2015). Key components of the PA process include verification, validation, and uncertainty quantification. Verification assures that the underlying equations are being solved correctly, as well as assessing the extent of numerical error. Validation is a process that determines the accuracy of the computational simulations with respect to representing the “real world” as approximated by experimental data. Uncertainty quantification addresses the question of uncertainty in the final calculated results based on, among other things, the uncertainty in the input parameters.
Several approaches to assessing model credibility have been established (Athe and Dinh, 2019; Riedmaier et al., 2021), including the Predictive Capability Maturity Model (PCMM) (Beghini and Hough, 2016). The PCMM is a framework that has been formalized to include six primary elements that are evaluated for a given simulation: geometric fidelity (spatial and temporal features and resolution), physics fidelity (range of applicability of chosen physics modeling capabilities), code verification (testing of the code against known solutions), solution verification (addressing numerical error), model validation (comparing model results against test data), and uncertainty quantification (consideration of all sources of uncertainty). In the end, evaluation of these credibility elements is used as a multi-dimensional qualitative metric to facilitate discussion and communication of the overall evidence for credibility of the model. PCMM is one example of a planning tool that can be used at the early stages of an analysis for informing risk in the use of a chosen modeling and simulation approach. While it has not been applied to the nuclear waste or climate model applications, it could be a fruitful exercise later when the decision is made to use climate model output as part of decision making for climate intervention applications.
Finally, clustering together metrics of interest with a range of performance goals demonstrates a possible path forward for both regional decision making and regulation, as well as identifying where additional research and higher-resolution modeling may be required to better quantify the risks and tradeoffs of a scenario. For example, running additional simulations using higher temporal resolution output to capture hydrologic extremes at basin scales as is done in Camilloni et al. (2013).
The PA Framework developed for nuclear waste disposal has been considered here by outlining a possible path forward for developing such a framework for climate intervention and showing a preliminary implementation. An assessment strategy has been developed by first defining a set of performance goals and using an existing simulation archive to assess the performance of the system against those goals. Key components of the PA framework were not included in this instance (e.g., model uncertainty, parameter sensitivity, and aleatory uncertainty). However, we provide an initial discussion of what evaluation metrics should be considered and what uncertainty is potentially the most impactful. This preliminary framework allows us to begin to address whether the outcomes of this scenario are within the desired goals or regulations across a range of environmental variables and how they compare to the alternative pathway of a specific emissions scenario. Based on a set of value criteria, it is possible to begin to make informed decisions. This is only a first step, however, as a complete assessment strategy would require additional extensive simulations to cover parametric uncertainty in the models. A more comprehensive framework will enable a deeper understanding of impacts and scenario selection and allow further refinements to the approach.
The original contributions presented in the study are included in the article/Supplementary Material, further inquiries can be directed to the corresponding author.
LW conceptualized the study and all authors contributed to development and design. TZ and SB adapted the FEPs approach and wrote the PA sections of the manuscript. JL and LW contributed to data processing. LW performed the statistical analysis and wrote sections of the manuscript. All authors contributed to the article and approved the submitted version.
This work was funded by the Laboratory Directed Research and Development (LDRD) program at Sandia National Laboratories. Sandia National Laboratories is a multimission laboratory managed and operated by the National Technology and Engineering Solutions of Sandia, LLC., a wholly owned subsidiary of Honeywell International, Inc., for the United States Department of Energy’s National Nuclear Security Administration under contract DE-NA0003525. This paper describes objective technical results and analysis.
The authors would like to acknowledge Erik Webb for his guidance which influenced the selection of Performance Assessment in this project, Cliff Hansen for his review of the manuscript and Peter Swift and Peter Davies for their expertise and discussion which influenced contents in the manuscript. The authors would also like to acknowledge the LDRD Office and Earth Science Research Foundation for their support and Ben Cook, Andrew Glen, and Lori Parrott, for their support and guidance.
The authors declare that the research was conducted in the absence of any commercial or financial relationships that could be construed as a potential conflict of interest.
All claims expressed in this article are solely those of the authors and do not necessarily represent those of their affiliated organizations, or those of the publisher, the editors and the reviewers. Any product that may be evaluated in this article, or claim that may be made by its manufacturer, is not guaranteed or endorsed by the publisher.
This article has been authored by an employee of National Technology & Engineering Solutions of Sandia, LLC under Contract No. DE-NA0003525 with the U.S. Department of Energy (DOE). The employee owns all right, title and interest in and to the article and is solely responsible for its contents. The United States Government retains and the publisher, by accepting the article for publication, acknowledges that the United States Government retains a non-exclusive, paid-up, irrevocable, world-wide license to publish or reproduce the published form of this article or allow others to do so, for United States Government purposes. The DOE will provide public access to these results of federally sponsored research in accordance with the DOE Public Access Plan https://www.energy.gov/downloads/doe-public-access-plan.
The Supplementary Material for this article can be found online at: https://www.frontiersin.org/articles/10.3389/fenvs.2023.1205515/full#supplementary-material
Abiodun, B. J., Odoulami, R. C., Sawadogo, W., Oloniyo, O. A., Abatan, A. A., New, M., et al. (2021). Potential impacts of stratospheric aerosol injection on drought risk managements over major river basins in Africa. Clim. Change 169, 31. doi:10.1007/s10584-021-03268-w
Adams, J. (2017). climate_indices, an open source Python library providing reference implementations of commonly used climate indices. Available at: https://github.com/monocongo/climate_indices.
Alamou, A. E., Obada, E., Biao, E. I., Zandagba, E. B. J., Da-Allada, C. Y., Bonou, F. K., et al. (2022). Impact of stratospheric aerosol geoengineering on meteorological droughts in west africa. Atmosphere 13, 234. doi:10.3390/atmos13020234
Aldy, J. E., Felgenhauer, T., Pizer, W. A., Tavoni, M., Belaia, M., Borsuk, M. E., et al. (2021). Social science research to inform solar geoengineering. Science 374, 815–818. doi:10.1126/science.abj6517
Athe, P., and Dinh, N. (2019). A framework for assessment of predictive capability maturity and its application in nuclear thermal hydraulics. Nucl. Eng. Des. 354, 110201. doi:10.1016/j.nucengdes.2019.110201
Beghini, L. L., and Hough, P. D. (2016). Sandia verification and validation challenge problem: a PCMM-based approach to assessing prediction credibility. J. Verification, Validation Uncertain. Quantification 1, 011002. doi:10.1115/1.4032369
Bhasin, S., Ravindran, B., and Moro, E. (2022). Solar geoengineering and the Montreal Protocol: a case for global governance. New Delhi: Council on Energy, Environment and Water. Available at: https://www.ceew.in/publications/solar-geoengineering-and-montreal-protocol.
Caldeira, K., and Wood, L. (2008). Global and Arctic climate engineering: numerical model studies. Philosophical Trans. R. Soc. A Math. Phys. Eng. Sci. 366, 4039–4056. doi:10.1098/rsta.2008.0132
Camilloni, I. A., Saurral, R. I., and Montroull, N. B. (2013). Hydrological projections of fluvial floods in the Uruguay and Paraná basins under different climate change scenarios. Int. J. River Basin Manag. 11, 389–399. doi:10.1080/15715124.2013.819006
Eastham, S. D., Weisenstein, D. K., Keith, D. W., and Barrett, S. R. H. (2018). Quantifying the impact of sulfate geoengineering on mortality from air quality and UV-B exposure. Atmos. Environ. 187, 424–434. doi:10.1016/j.atmosenv.2018.05.047
Felgenhauer, T., Govindasamy, B., Borsuk, M. E., Brune, M., Camilloni, I., Wiener, J. B., et al. (2022). Solar radiation modification: a risk-risk analysis. New York, NY: Carnegie Climate Governance Initiative C2G. Available at: www.c2g2.net.
Ferraro, A. J., Charlton-Perez, A. J., and Highwood, E. J. (2014). A risk-based framework for assessing the effectiveness of stratospheric aerosol geoengineering. PLOS ONE 9, e88849. doi:10.1371/journal.pone.0088849
Gal, Y., and Ghahramani, Z. (2016). “Dropout as a bayesian approximation: representing model uncertainty in deep learning,” in International conference on machine learning (PMLR) (New York, NY, United States: ACM), 1050–1059.
Galson, D. A., Swift, P. N., Anderson, D. R., Bennett, D. G., Crawford, M. B., Hicks, T. W., et al. (2000). Scenario development for the waste isolation Pilot plant compliance certification application. Reliab. Eng. Syst. Saf. 69, 129–149. doi:10.1016/S0951-8320(00)00029-6
Garcia, R. R., Marsh, D. R., Kinnison, D. E., Boville, B. A., and Sassi, F. (2007). Simulation of secular trends in the middle atmosphere, 1950–2003. J. Geophys. Res. Atmos. 112, 7485. doi:10.1029/2006JD007485
Geoengineering Large Ensemble (GLENS) (2022). Earthsystemgrid. Avaliable at: https://www.earthsystemgrid.org/dataset/ucar.cgd.ccsm4.GLENS.html (Accessed November 1, 2022).
Golaz, J.-C., Caldwell, P. M., Roekel, L. P. V., Petersen, M. R., Tang, Q., Wolfe, J. D., et al. (2019). The DOE E3SM coupled model version 1: overview and evaluation at standard resolution. J. Adv. Model. Earth Syst. 11, 2089–2129. doi:10.1029/2018MS001603
Goldstein, B., Kobos, P. H., and Brady, P. V. (2010). Unintended consequences of atmospheric injection of sulphate aerosols. United States: OSTI. doi:10.2172/1000289
Grisé, M., Yonekura, E., Blake, J. S., DeSmet, D., Garg, A., and Preston, B. L. (2021). Climate control: international legal mechanisms for managing the geopolitical risks of geoengineering RAND corporation. Available at: https://www.rand.org/pubs/perspectives/PEA1133-1.html (Accessed June 6, 2023).
Heyen, D., Horton, J., and Moreno-Cruz, J. (2019). Strategic implications of counter-geoengineering: clash or cooperation? J. Environ. Econ. Manag. 95, 153–177. doi:10.1016/j.jeem.2019.03.005
Hurrell, J. W., Holland, M. M., Gent, P. R., Ghan, S., Kay, J. E., Kushner, P. J., et al. (2013). The community earth system model: a framework for collaborative research. Bull. Am. Meteorological Soc. 94, 1339–1360. doi:10.1175/BAMS-D-12-00121.1
Iturbide, M., Gutiérrez, J. M., Alves, L. M., Bedia, J., Cerezo-Mota, R., Cimadevilla, E., et al. (2020). An update of IPCC climate reference regions for subcontinental analysis of climate model data: definition and aggregated datasets. Earth Syst. Sci. Data 12, 2959–2970. doi:10.5194/essd-12-2959-2020
Jackson, L. S., Crook, J. A., Jarvis, A., Leedal, D., Ridgwell, A., Vaughan, N., et al. (2015). Assessing the controllability of Arctic sea ice extent by sulfate aerosol geoengineering. Geophys. Res. Lett. 42, 1223–1231. doi:10.1002/2014GL062240
Jinnah, S., and Nicholson, S. (2019). The hidden politics of climate engineering. Nat. Geosci. 12, 876–879. doi:10.1038/s41561-019-0483-7
Kaizer, J. S., Heller, A. K., and Oberkampf, W. L. (2015). Scientific computer simulation review. Reliab. Eng. Syst. Saf. 138, 210–218. doi:10.1016/j.ress.2015.01.020
Kawamiya, M., Hajima, T., Tachiiri, K., Watanabe, S., and Yokohata, T. (2020). Two decades of earth system modeling with an emphasis on model for interdisciplinary research on climate (MIROC). Prog. Earth Planet. Sci. 7, 64. doi:10.1186/s40645-020-00369-5
Keith, D. (2017). Toward a responsible solar geoengineering research program issues in science and technology. Available at: https://issues.org/toward-a-responsible-solar-geoengineering-research-program/.
Keys, P. W., Barnes, E. A., Diffenbaugh, N. S., Hurrell, J. W., and Bell, C. M. (2022). Potential for perceived failure of stratospheric aerosol injection deployment. Proc. Natl. Acad. Sci. 119, e2210036119. doi:10.1073/pnas.2210036119
Kravitz, B., Caldeira, K., Boucher, O., Robock, A., Rasch, P. J., Alterskjær, K., et al. (2013). Climate model response from the geoengineering model Intercomparison project (GeoMIP). J. Geophys. Res. Atmos. 118, 8320–8332. doi:10.1002/jgrd.50646
Kravitz, B., and MacMartin, D. G. (2020). Uncertainty and the basis for confidence in solar geoengineering research. Nat. Rev. Earth Environ. 1, 64–75. doi:10.1038/s43017-019-0004-7
Kravitz, B., MacMartin, D. G., Tilmes, S., Richter, J. H., Mills, M. J., Cheng, W., et al. (2019). Comparing surface and stratospheric impacts of geoengineering with different SO2 injection strategies. J. Geophys. Res. Atmos. 124, 7900–7918. doi:10.1029/2019JD030329
Kravitz, B., Robock, A., Boucher, O., Schmidt, H., Taylor, K. E., Stenchikov, G., et al. (2011). The geoengineering model Intercomparison project (GeoMIP). Atmosph. Sci. Lett. 12, 162–167. doi:10.1002/asl.316
Kravitz, B., Robock, A., Tilmes, S., Boucher, O., English, J. M., Irvine, P. J., et al. (2015). The geoengineering model Intercomparison project phase 6 (GeoMIP6): simulation design and preliminary results. Geosci. Model. Dev. 8, 3379–3392. doi:10.5194/gmd-8-3379-2015
Laakso, A., Kokkola, H., Partanen, A.-I., Niemeier, U., Timmreck, C., Lehtinen, K. E. J., et al. (2016). Radiative and climate impacts of a large volcanic eruption during stratospheric sulfur geoengineering. Atmos. Chem. Phys. 16, 305–323. doi:10.5194/acp-16-305-2016
Laakso, A., Niemeier, U., Visioni, D., Tilmes, S., and Kokkola, H. (2022). Dependency of the impacts of geoengineering on the stratospheric sulfur injection strategy – Part 1: Intercomparison of modal and sectional aerosol modules. Atmos. Chem. Phys. 22, 93–118. doi:10.5194/acp-22-93-2022
Lee, W. R., MacMartin, D. G., Visioni, D., and Kravitz, B. (2021). High-latitude stratospheric aerosol geoengineering can Be more effective if injection is limited to spring. Geophys. Res. Lett. 48, e2021GL092696. doi:10.1029/2021GL092696
Lee, W. R., MacMartin, D. G., Visioni, D., Kravitz, B., Chen, Y., Moore, J. C., et al. (2023). High-latitude stratospheric aerosol injection to preserve the arctic. Earth’s Future 11, e2022EF003052. doi:10.1029/2022EF003052
Lloyd-Hughes, B., and Saunders, M. A. (2002). A drought climatology for Europe. Int. J. Climatol. 22, 1571–1592. doi:10.1002/joc.846
Lockley, A., Xu, Y., Tilmes, S., Sugiyama, M., Rothman, D., and Hindes, A. (2022). 18 Politically relevant solar geoengineering scenarios. Socio-Environmental Syst. Model. 4, 18127. doi:10.18174/sesmo.18127
Lowry, T. S. (2021). Understanding uncertainty in geothermal energy development using a formalized performance assessment approach. Geotherm. Resour. Counc. Trans. 45, 5.
MacMartin, D. G., Kravitz, B., Tilmes, S., Richter, J. H., Mills, M. J., Lamarque, J.-F., et al. (2017). The climate response to stratospheric aerosol geoengineering can Be tailored using multiple injection locations. J. Geophys. Res. Atmos. 122 (12), 26868. doi:10.1002/2017JD026868
MacMartin, D. G., Visioni, D., Kravitz, B., Richter, J. H., Felgenhauer, T., Lee, W. R., et al. (2022). Scenarios for modeling solar radiation modification. Proc. Natl. Acad. Sci. 119, e2202230119. doi:10.1073/pnas.2202230119
McKee, T. B., Doesken, N. J., and Kleist, J. (1993). The relationship of drought frequency and duration to time scales. in.
McKinley, I. G., West, J. M., and Hardie, S. M. L. (2022). A risk management perspective on climate change: lessons learned from the nuclear industry. Environ. Manag. 69, 835–846. doi:10.1007/s00267-022-01594-y
Meacham, P. G., Anderson, D. R., Bonano, E. J., and Marietta, M. G. (2011). Sandia National Laboratories performance assessment methodology for long-term environmental programs: the history of nuclear waste management. Albuquerque, NM, and Livermore, CA (United States: Sandia National Laboratories SNL. doi:10.2172/1031300
Mills, M. J., Richter, J. H., Tilmes, S., Kravitz, B., MacMartin, D. G., Glanville, A. A., et al. (2017). Radiative and chemical response to interactive stratospheric sulfate aerosols in fully coupled CESM1(WACCM). J. Geophys. Res. Atmos. 122, 27006. doi:10.1002/2017JD027006
Moore, J. C., Mettiäinen, I., Wolovick, M., Zhao, L., Gladstone, R., Chen, Y., et al. (2021). Targeted geoengineering: local interventions with global implications. Glob. Policy 12, 108–118. doi:10.1111/1758-5899.12867
Nalam, A., Bala, G., and Modak, A. (2018). Effects of Arctic geoengineering on precipitation in the tropical monsoon regions. Clim. Dyn. 50, 3375–3395. doi:10.1007/s00382-017-3810-y
National Academies of Sciences Engineering, and Medicine (2021). Reflecting sunlight: recommendations for solar geoengineering research and research governance. Washington, D.C.: National Academies Press. doi:10.17226/25762
Neale, R. B., Gettelman, A., Park, S., Chen, C.-C., Lauritzen, P. H., Williamson, D. L., et al. (2010). Description of the NCAR community atmosphere model. Boulder, CO, United States: Community Earth System Model, 283.
Nightingale, P., and Cairns, R. (2014). The security implications of geoengineering:blame,imposed agreement and the security of critical infrastructure. Available at: http://geoengineering-governance-research.org/perch/resources/workingpaper18nightingalecairnssecurityimplications.pdf (Accessed June 6, 2023).
Nuclear Energy Agency (2000). Features, events, and processes (FEPs) for geologic disposal of radioactive waste. Paris: OECD Publishing. Available at: https://www.oecd-nea.org/rwm/reports/2000/nea2549-features-events-processes-for-disposal.pdf.
Nuclear Energy Agency (2019). International features, events and processes (IFEP) list for the deep geological disposal of radioactive waste version 3.0. Paris, France: NEA.
Parker, A., and Irvine, P. J. (2018). The risk of termination shock from solar geoengineering. Earth’s Future 6, 456–467. doi:10.1002/2017EF000735
Raju, K. S., and Kumar, D. N. (2020). Review of approaches for selection and ensembling of GCMs. J. Water Clim. Change 11, 577–599. doi:10.2166/wcc.2020.128
Rasch, P. J., Xie, S., Ma, P.-L., Lin, W., Wang, H., Tang, Q., et al. (2019). An overview of the atmospheric component of the energy Exascale earth system model. J. Adv. Model. Earth Syst. 11, 2377–2411. doi:10.1029/2019MS001629
Rechard, R. P. (1999). Historical relationship between performance assessment for radioactive waste disposal and other types of risk assessment. Risk Anal. 19, 763–807. doi:10.1023/a:1007058325258
Richter, J. H., Visioni, D., MacMartin, D. G., Bailey, D. A., Rosenbloom, N., Dobbins, B., et al. (2022). Assessing Responses and Impacts of Solar climate intervention on the Earth system with stratospheric aerosol injection (ARISE-SAI): protocol and initial results from the first simulations. Geosci. Model. Dev. 15, 8221–8243. doi:10.5194/gmd-15-8221-2022
Riedmaier, S., Danquah, B., Schick, B., and Diermeyer, F. (2021). Unified framework and survey for model verification, validation and uncertainty quantification. Archives Comput. Methods Eng. 28, 2655–2688. doi:10.1007/s11831-020-09473-7
Robock, A., Oman, L., and Stenchikov, G. L. (2008). Regional climate responses to geoengineering with tropical and Arctic SO2 injections. J. Geophys. Res. Atmos. 113. doi:10.1029/2008JD010050
Smith, W. (2020). The cost of stratospheric aerosol injection through 2100. Environ. Res. Lett. 15, 114004. doi:10.1088/1748-9326/aba7e7
Smith, W., and Henly, C. (2021). Updated and outdated reservations about research into stratospheric aerosol injection. Clim. Change 164, 39. doi:10.1007/s10584-021-03017-z
Swift, P., Barr, G., Barnard, R., Rechard, R., Schenker, A., Freeze, G., et al. (1999). Feature, event, and process screening and scenario development for the Yucca Mountain total system performance assessment. Albuquerque, NM (United States): Sandia National Lab. SNL-NM. Available at: https://www.osti.gov/biblio/5678.
Tilmes, S., Richter, J. H., Kravitz, B., MacMartin, D. G., Mills, M. J., Simpson, I. R., et al. (2018). CESM1(WACCM) stratospheric aerosol geoengineering large ensemble project. Bull. Am. Meteorological Soc. 99, 2361–2371. doi:10.1175/BAMS-D-17-0267.1
Tracy, S. M., Moch, J. M., Eastham, S. D., and Buonocore, J. J. (2022). Stratospheric aerosol injection may impact global systems and human health outcomes. Elem. Sci. Anthropocene 10, 00047. doi:10.1525/elementa.2022.00047
United States Department of Energy (2019). Title 40 CFR Part 191 compliance recertification application for the waste isolation Pilot plant. Carlsbad, NM: U.S. DOE Carlsbad Field Office.
United States Environmental Protection Agency (1993). 40 CFR Part 191: environmental radiation protection standards for the management and disposal of spent nuclear fuel, high-level and transuranic radioactive wastes: final rule. Washington, DC: Waste Isolation Pilot Plant Land Withdrawal Act, Pub.
United States Environmental Protection Agency (1996). 40 CFR Part 194: criteria for the certification and recertification of the waste isolation Pilot plant’s compliance with the 40 CFR Part 191 disposal regulations. Washington, DC: EPA.
Versen, J., Mnatsakanyan, Z., and Urpelainen, J. (2021). Preparing the United States for security and governance in a geoengineering future. Brookings. Available at: https://www.brookings.edu/research/preparing-the-united-states-for-security-and-governance-in-a-geoengineering-future/ (Accessed May 15, 2023).
Visioni, D., MacMartin, D. G., Kravitz, B., Boucher, O., Jones, A., Lurton, T., et al. (2021). Identifying the sources of uncertainty in climate model simulations of solar radiation modification with the G6sulfur and G6solar Geoengineering Model Intercomparison Project (GeoMIP) simulations. Atmos. Chem. Phys. 21, 10039–10063. doi:10.5194/acp-21-10039-2021
Visioni, D., MacMartin, D. G., Kravitz, B., Tilmes, S., Mills, M. J., Richter, J. H., et al. (2019). Seasonal injection strategies for stratospheric aerosol geoengineering. Geophys. Res. Lett. 46, 7790–7799. doi:10.1029/2019GL083680
Wang, H., Easter, R. C., Zhang, R., Ma, P.-L., Singh, B., Zhang, K., et al. (2020). Aerosols in the E3SM version 1: new developments and their impacts on radiative forcing. J. Adv. Model. Earth Syst. 12, e2019MS001851. doi:10.1029/2019MS001851
Watson-Parris, D. (2021). Machine learning for weather and climate are worlds apart. Philosophical Trans. R. Soc. A Math. Phys. Eng. Sci. 379, 20200098. doi:10.1098/rsta.2020.0098
Weber, T., Corotan, A., Hutchinson, B., Kravitz, B., and Link, R. (2020). Technical note: deep learning for creating surrogate models of precipitation in Earth system models. Atmos. Chem. Phys. 20, 2303–2317. doi:10.5194/acp-20-2303-2020
Keywords: performance assessment, risk-risk, climate change, stratospheric aerosol injection, climate intervention
Citation: Wheeler L, Zeitler T, Brunell S, Lien J, Shand L, Wagman B, Martinez C and Potter K (2023) Performance assessment for climate intervention (PACI): preliminary application to a stratospheric aerosol injection scenario. Front. Environ. Sci. 11:1205515. doi: 10.3389/fenvs.2023.1205515
Received: 13 April 2023; Accepted: 21 November 2023;
Published: 22 December 2023.
Edited by:
Amy Heintz, Battelle, United StatesReviewed by:
Eduardo Landulfo, Instituto de Pesquisas Energéticas e Nucleares (IPEN), BrazilCopyright © 2023 Wheeler, Zeitler, Brunell, Lien, Shand, Wagman, Martinez and Potter. This is an open-access article distributed under the terms of the Creative Commons Attribution License (CC BY). The use, distribution or reproduction in other forums is permitted, provided the original author(s) and the copyright owner(s) are credited and that the original publication in this journal is cited, in accordance with accepted academic practice. No use, distribution or reproduction is permitted which does not comply with these terms.
*Correspondence: Lauren Wheeler, bHdoZWVsZUBzYW5kaWEuZ292
Disclaimer: All claims expressed in this article are solely those of the authors and do not necessarily represent those of their affiliated organizations, or those of the publisher, the editors and the reviewers. Any product that may be evaluated in this article or claim that may be made by its manufacturer is not guaranteed or endorsed by the publisher.
Research integrity at Frontiers
Learn more about the work of our research integrity team to safeguard the quality of each article we publish.