- 1Department of Atmospheric and Oceanic Sciences, Institute of Atmospheric Sciences, Fudan University, Shanghai, China
- 2Shanghai Key Laboratory of Atmospheric Particle Pollution and Prevention (LAP3), Department of Environmental Science and Engineering, Fudan University, Shanghai, China
- 3Shanghai Environmental Monitoring Center, State Ecology and Environment Scientific Observation and Research Station for the Yangtze River Delta at Dianshan Lake (SEED), Shanghai, China
- 4Institute of Eco-Chongming (SIEC), Shanghai, China
- 5Shanghai Qi Zhi Institute, Shanghai, China
China experienced severe haze pollution in the past decades. However, systematic characterization of atmospheric fine particles with advanced measurement techniques has been very scarce in Shanghai, which is the largest megacity in China. Herein, we present the characterization of non-refractory submicron aerosol (NR-PM1) in urban Shanghai during winter 2017 by applying an Aerosol Chemical Speciation Monitor (ACSM, Aerodyne Research Inc.). The NR-PM1 is predominated by organics (43%), followed by nitrate (29%), sulfate (18%), ammonium (7%), and chloride (3%). Regarding the organic aerosol (OA) factors, three organic aerosol factors were identified and classified as hydrocarbon-like organic aerosol (HOA), less-oxygenated organic aerosol (LO-OOA), and more-oxygenated organic aerosol (MO-OOA), which contributed 32%, 22%, and 46% to total organic aerosol respectively. The NR-PM1 composition exhibited an increase in secondary inorganic aerosol (SIA) (sulfate, nitrate and ammonium) contribution from clean episodes (49%) to pollution episodes (59%), while organic aerosol contribution decreased accordingly from 48% to 39%. The strong increase of sulfate in high-relative humidity (RH) pollution episodes indicated that aqueous-phase oxidation of SO2 could be an important formation process for sulfate during particulate air pollution period. The contribution of nitrate was elevated from 25% during clean episodes to 32% during polluted episodes, likely owing to the increase of relative humidity which facilitates the hydrolysis of N2O5 and the gas-to-particle partitioning of hydrophilic NH4NO3 and. Further analysis of atmospheric formation relevance suggested that less-oxygenated organic aerosol formation was mainly driven by aqueous-phase chemistry reactions, whereas photochemical oxidation became an important process for more-oxygenated organic aerosol formation. Meanwhile, less-oxygenated organic aerosol formation may also be influenced by atmospheric oxidative tracer (i.e., Ox), as less-oxygenated organic aerosol exhibited a distinct peak at noon under high-relative humidity condition.
1 Introduction
Particulate pollution is a critical environmental issue in China, which has significant impacts on regional and global climate, air quality, and human health. In recent winters, China has experienced severe and largescale haze pollution, which has garnered global attention and prompted extensive scientific research on air pollution. Measurements conducted in major Chinese cities have revealed that the daily average levels of PM2.5 during winter are approximately 1–2 orders of magnitude larger than those detected in the urban areas of Europe and United States (Huang et al., 2014). This severe particulate pollution is often accompanied by poor air quality and extremely low visibility, resulting in a sharp increase in respiratory illnesses. China had the highest number of deaths attributed to air pollution, with an estimated 1.1 million fatalities resulting from long-term exposure to high concentrations of particulate pollution in 2015 (Cohen et al., 2017). Hence, identifying the main sources and evolution processes of atmospheric particulates is necessary to implement optimized and targeted emission control strategies.
Although there have been attempts to understand haze pollution, our knowledge of its evolution processes and sources is currently not fully clear. Haze pollution often evolves dynamically and rapidly in the atmosphere by involving complicated processes such as aging and mixing, fog processing, direct emissions, and secondary formation. Nevertheless, previous research utilizing filter measurements has been restricted either by a lack of high temporal resolution or by sampling artifacts (e.g., evaporative loss) (Cao et al., 2012; Huang et al., 2014). At present, aerosol chemical speciation monitor (ACSM) and aerosol mass spectrometry (AMS) instruments with high time resolution and capability in characterization of non-refractory submicron aerosols (NR-PM1) composition, have been widely used in the measurements of aerosol chemical composition to investigate their sources, chemical composition, and secondary processes (Sun et al., 2013; Sun et al., 2014; Duan et al., 2020). Sun et al. (2010) first characterized the composition, sources, and variations of NR-PM1 species in Beijing during the summer of 2006, using a Quadrupole AMS (Q-AMS). Subsequently, by using a High-Resolution Time-of-Flight AMS and an ACSM, Huang et al. (2010) and Sun et al. (2012) conducted further investigations in the summer of 2008 and 2011 respectively. Throughout these studies, the composition of NR-PM1 species exhibited significant variability, but overall organic aerosol (OA) predominated NR-PM1 species, accounting for ∼35%–40% on average in summer (Huang et al., 2010; Sun et al., 2010; Sun et al., 2012). In general, applying positive matrix factorization (PMF) based on the characteristic mass spectral signal of OA, OA can be classified into primary organic aerosol (POA) [e.g., hydrocarbon-like OA (HOA), biomass burning OA (BBOA), coal combustion OA (CCOA), and cooking OA (COA)] and secondary organic aerosol (SOA), which can be further identified as less-oxygenated OA (LO-OOA) and more-oxygenated OA (MO-OOA) (Canonaco et al., 2013; Elser et al., 2016; Wang et al., 2017; Duan et al., 2020), although early nomenclatures such as semi-volatile oxygenated OA (SV-OOA) and low-volatility oxygenated OA (LV-OOA) are still widely used (Zhang et al., 2014; Budisulistiorini et al., 2016). Previous ACSM studies in China were conducted primarily in northern heating regions (e.g., Beijing, Xi’an, and Handan) (Sun et al., 2014; Sun et al., 2016; Li et al., 2017; Duan et al., 2020; Duan et al., 2022), while these are rare in southern urban cities. Since the aerosol particle composition are highly related to meteorological conditions and source emissions, OA composition and aerosol processing in northern and southern cities present different characteristics, especially in winter. In view of the frequent incidents of haze pollution in winter, it is essential to comprehensively understand the chemical composition, sources, and formation mechanisms of winter haze.
In this study, we carried out a routine measurement of NR-PM1 species in a megacity of China, Shanghai from 10 to 21 January 2018, presenting real-time measurements of ambient aerosol using ACSM instrument. The aim is to demonstrate the capability of ACSM for real-time quantification of OA mass concentration and composition in highly polluted environment, and analysis the sources and processes of OA components via positive matrix factorization (PMF) method. This study provides insights into the sources, properties and evolution processes of winter aerosols in Shanghai.
2 Materials and methods
2.1 Sampling site and instrumentation
NR-PM1 species were measured using an ACSM (Aerodyne Research, Billerica MA) from 10 to 21 January 2018. The site is situated on the rooftop of a commercial building (15 m) in Xuhui district, Shanghai, China (31°10′N, 121°25′E). This district is a typical urban area surrounded by commercial buildings, residential areas, and a traffic artery, and there are no significant pollution sources nearby, as shown on the map in Supplementary Figure S1.
Basically, the ACSM instrument consists of an aerodynamic lens that focuses a particle beam (with a vacuum aerodynamic diameter below 1 µm) and directs it through three vacuum chambers, the last one being a detection chamber where submicrometer aerosol are flash vaporized on a resistively heated surface (600°C) and ionized via 70 eV electron impact. The generated positive ions were finally analyzed with a commercial quadrupole mass spectrometer.
The air quality (e.g., PM2.5, NO, NOx, SO2, CO, and O3 concentrations) was simultaneously measured by several on-line monitors (Thermo Scientific, United States). Meteorological parameters [e.g., relative humidity (RH), temperature (T), wind direction (WD), wind speed (WS), and visibility] were obtained from the Shanghai Meteorological Data Sharing Service System.
2.2 Data analysis
2.2.1 ACSM data analysis
The final mass concentrations and mass spectra were processed with the “ACSM Local” toolkit (Aerodyne Research, Inc.) in Igor Pro V6.37 (WaveMetrics, Inc., Lake Oswego, Oregon United States), which is a widely applied procedure described in previous studies (Ng et al., 2011b; Middlebrook et al., 2012). The chemical composition and mass concentration of NR-PM1 species were obtained based on the default relative ionization efficiency (RIE), at 1.4, 1.1, and 1.3 for organics (Org), nitrate (NO3−), and chloride (Cl−), respectively (Canagaratna et al., 2007), and RIE values of 6.0 for ammonium (NH4+) and 1.2 for sulfate (SO42−) based on on-site calibrations. A particle collection efficiency (CE) of 0.5 was attained throughout the entire campaign with data uncertainties generally within ±30%, which is similar with most ambient studies (Middlebrook et al., 2012; Crenn et al., 2015).
2.2.2 Source apportionment
The source apportionment of organics was achieved through PMF analysis, and the results were evaluated with the multilinear engine (ME-2) (Paatero, 1999) via the interface SoFi (Source Finder) coded in Igor Wavemetrics (Canonaco et al., 2013). We determined a three-factor solution (hydrocarbon-like OA, “HOA”; less-oxidized oxygenated OA, “LO-OOA”; more-oxidized oxygenated OA, “MO-OOA”). PMF is a bilinear unmixing model that represents the input data as a linear combination of static factor profiles and their corresponding time series:
where
Normally, monitoring the total Q is not meaningful because the expected value for a suitable solution (Qexp) depends on the size of the data matrix and on the number of chosen factors. The Q/Qexp values can be used to choose the reasonableness of the model results, and a value of ∼1 generally indicates the relatively reasonable solution. The calculation of Qexp is shown in following Equation:
PMF requires no
where f corresponds to a row of the matrix F, j denotes the mass to charge ratio (m/z) of the ions, and
In this analysis, PMF model runs ranging from 1 to 7 factor numbers were carried out to ascertain the most reasonable solution. Each simulation was randomly conducted 10 times. The most appropriate number of factors were selected by some mathematical indicators calculated following the PMF model, including Q values, a possible explanation of the sources, and the residual distribution. Almost 100% of the scaled residuals were within ±3σ and were normally distributed for all species. The Q/Qexp value for three-factor was closer to 1.0 (Supplementary Figure S2), suggesting that the three-factor configuration was supposed. More details regarding the PMF key diagnostic process of the factors are presented in the Supplementary Material.
2.3 Aerosol liquid water content
The aerosol liquid water content (ALWC) was calculated by the ISORROPIA-II thermodynamic model using aerosol inorganic species and meteorological parameters (e.g., T and RH) as the model inputs (Fountoukis & Nenes, 2007). ISORROPIA-II was operated in forward mode, assuming the particles are “metastable” (Guo et al., 2015; Song et al., 2018). The input parameters for the forward-mode calculations included ambient RH, T, and the measured total (gas plus aerosol) concentrations of NH3, H2SO4, HCl, HNO3, K+, Na+, Ca2+, and Mg2+. Gaseous NH3 [i.e., NH3(g)], measured by a NH3 analyzer (G2103, Picarro, California, United States) (Huo et al., 2015), was taken into consideration, while the HNO3(g) and HCl(g) data was absent in the model during the wintertime campaign. Only total ammonium [TNH4+ = NH3(g) + NH4+] was inputted for the forward-mode calculations. More details and validation of the thermodynamic calculations could be found in previous studies (Song et al., 2018; Wang et al., 2020).
3 Results and discussion
3.1 Mass concentrations and chemical composition of NR-PM1 in Shanghai
The temporal variations of NR-PM1 species, meteorological conditions, and trace gases during the entire campaign is depicted in Figure 1. The mass concentrations of NR-PM1 (= Org + SO42− + NO3− + NH4+ + Cl−) for the entire campaign period ranged from 14.3 to 151.2 μg·m−3, with an average of 56.8 ± 30.0 μg·m−3 (see Figure 1; Supplementary Table S1). The average level here was found to be larger than that observed in Shanghai in the summer of 2010 (27.3 μg·m−3) and 2018 (15.6 μg·m−3) (Huang et al., 2012; Gao et al., 2019), which is attributed to higher source emissions in winter than in summer. This seasonal variation has also been observed in other urban cities (Li et al., 2015; Sun et al., 2015; Duan et al., 2020). Meanwhile, the average level of NR-PM1 here was generally comparable to that observed in the wintertime of Lanzhou (57.3 μg·m−3) (Xu et al., 2016), and lower than the wintertime concentrations measured in northern domestic heating regions (Supplementary Table S2), such as Beijing (76.8 μg·m−3), Handan (178 μg·m−3), and Shijiazhuang (178 μg·m−3) (Wang et al., 2015; Li et al., 2017; Huang et al., 2019). Additionally, the time series of NR-PM1 exhibited a strong correlation with that of PM2.5 (R2 = 0.81) with a regression slope of 0.66 (Supplementary Figure S3), suggesting that NR-PM1 is a dominant component of PM2.5 and thereby plays an important role in PM2.5 pollution in urban Shanghai. That agrees with previous studies in ambient aerosols from Baoji (Wang et al., 2017), Beijing (Sun et al., 2012), and Shijiazhuang (Huang et al., 2019).
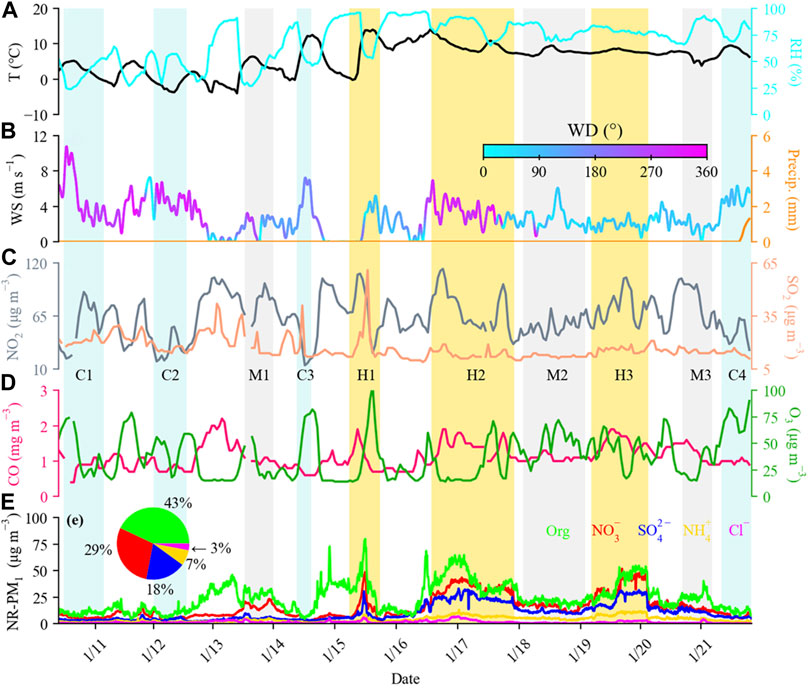
FIGURE 1. Time series of temperature (T) and relative humidity (RH) (A), wind speed (WS) colored by wind direction (WD) and precipitation (B), NO2 and SO2 (C), CO and O3 (D), and NR-PM1 species (E) during the observation period. Four clean episodes (C1–C4), three moderate-pollution episodes (M1–M3), and three high-pollution episodes (H1–H3) are marked for further discussion.
Similar to measurements at other urban sites (Zhang et al., 2015b; Xu et al., 2016; Duan et al., 2019; Duan et al., 2020), OA was the most abundant fraction among NR-PM1 species, with an average of 43% (25 %–80%), followed by 29% of nitrate (9 %–40%), 18% of sulfate (4 %–30%), 7% of ammonium (2 %–17%), and 3% of chloride (0.2 %–11%). The dominant contribution of OA indicated that OA played an important role in PM1 pollution, which is in agreement with measurements from other urban areas, e.g., Beijing (Elser et al., 2016; Sun et al., 2016) and Lanzhou (Xu et al., 2016) (see Supplementary Table S2). The larger contribution of nitrate than sulfate in winter was in contrast with the situation detected in summer, where there was a larger contribution from sulfate (27%) than nitrate (12%) (Gao et al., 2019). This suggests that the enhanced formation and increased contribution of nitrate in highly polluted days, probably because of the much lower temperature in winter which would be more favorable for the partitioning of nitrate from gas phase into particle phase. Meanwhile, the contribution of nitrate in our study was also larger than that detected in Shanghai during the winter of 2016 (23%) (Zhu et al., 2021b), indicating the increasing importance of nitrate pollution in comparation to sulfate pollution in recent years. This is in agreement with the interannual evolution trend of nitrate in Beijing (Xu et al., 2019).
The diurnal variations of aerosols are highly susceptible to the diurnal cycle of atmospheric processes and emissions, as well as the evolution of planetary boundary layer (PBL) height governing the vertical dispersion of pollutants. Figure 2A showed the diurnal variations of NR-PM1 species. The concentrations of pollutants increased at night, largely owing to a progressively shallower PBL and enhanced emissions from the regional transport of residential heating, particularly for organics and Cl−. Furthermore, the PBL height was developed by solar radiation during daytime, and therefore the pollutants became more dispersed and diluted in the afternoon, leading to the decline of organics, SO42−, NO3−, NH4+, and Cl−. Hence, the concentrations of pollutants were normalized by ΔCO (=CO-COmin) to minimize the influence of PBL height. CO is commonly regarded as an emission tracer to illustrate dilution on timescales of hours to days due to its relatively long lifetime against the oxidation of OH radicals (DeCarlo et al., 2010). In other words, using ΔCO as a normalization factor helps to account for dilution effects that can occur over relatively short timescales due to changes in PBL height. After offsetting the effect of PBL dilution, SO42−, NO3−, and NH4+ increased apparently from 09:00 to 14:30 (local time; Figure 2B), suggesting the efficient photochemical production of these secondary inorganic species. Meanwhile, the rise in NO3− levels (about 1.9 times, from 21 to 39 μg·m−3·ppb−1) is slightly higher than that of SO42− (about 1.5 times, from 17 to 26 μg·m−3·ppb−1). This result indicated more efficient photochemical production of nitrate than sulfate, in consideration of greater loss rate of nitrate than sulfate due to the semi-volatility of nitric acid and further evaporation loses. Moreover, the overall rise of organics from 09:00 to 14:30 LT implied the efficient photochemical production of SOA, which would be further explored in more detail in the Section 3.3.
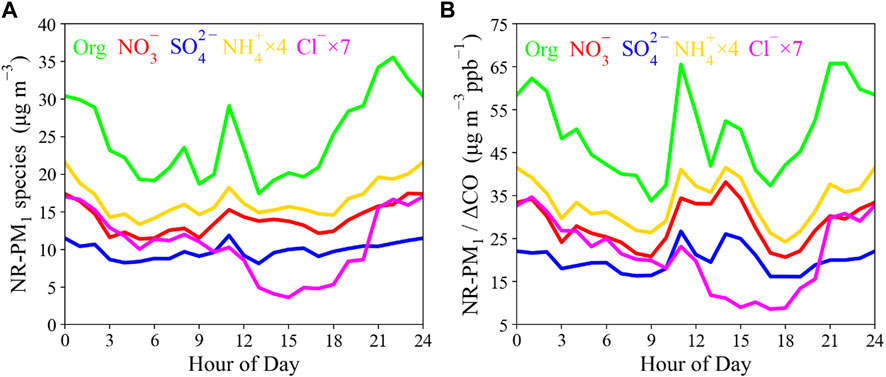
FIGURE 2. Diurnal variation of NR-PM1 composition (A) and NR-PM1/ΔCO (B). Data of ammonium and chloride are multiplied by factors of 4 and 7, respectively.
3.2 Sources of organic aerosol
Three OA sources (i.e., HOA, LO-OOA, and MO-OOA) were resolved during winter observation by applying PMF to analyze high-resolution OA mass spectra. Details about characteristics of OA sources were discussed below.
The HOA mass spectrum in this study was dominated by typical hydrocarbon ion series of [CnH2n+1]+ and [CnH2n−1]+, particularly m/z 27, 29, 41, 43, 55, and 57, which is similar to the source spectra of diesel fuels (Canagaratna et al., 2004; Mohr et al., 2009) and the previously reported HOA spectra (Ng et al., 2011a). HOA was tightly correlated with CO (R2 = 0.71 Figure 3), a tracer for vehicle emissions, yet presented much weaker correlations with secondary inorganic species (SO42−, NO3−; R2 ≤ 0.17, Supplementary Table S3). Previous studies have reported that HOA in urban areas is mostly linked to vehicle-related emissions (Xu et al., 2016; Hu et al., 2017). However, the HOA mass spectrum exhibited obvious hydrocarbon-like signals at m/z 67 and m/z 69, with ion fragments of [C5H7]+ and [C5H9]+, respectively, which could be associated with emissions from cooking PMF POA (Zhu et al., 2021a). The HOA from cooking and traffic emissions in this study averagely accounted for 32% of total OA (Figure 4B), which is comparable to the contribution of POA (= HOA + COA) in 2006 and 2008 Beijing (41%–42%) (Jimenez et al., 2009; Huang et al., 2010). Furthermore, the contribution of HOA to OA in Shanghai was higher than those observed in the wintertime of Beijing (7%–11%) (Zhang et al., 2014; Gu et al., 2020) and Xi’an (15%) (Elser et al., 2016), largely owing to high contributions from other OA factors (e.g., BBOA and CCOA) during the heating season. As shown in Figure 4A, the diurnal pattern of HOA in winter of Shanghai showed three predominant peaks in the morning (08:00–9:00), midday (11:00–12:00), and evening (22:00–23:00), likely attributed to traffic rush hour and cooking activities, as well as enhanced truck emissions (only allowed to drive on the road at midnight) and a shallow PBL during the night. Similar diurnal cycles were found in the wintertime of Xi’an and Beijing (Elser et al., 2016; Sun et al., 2016).
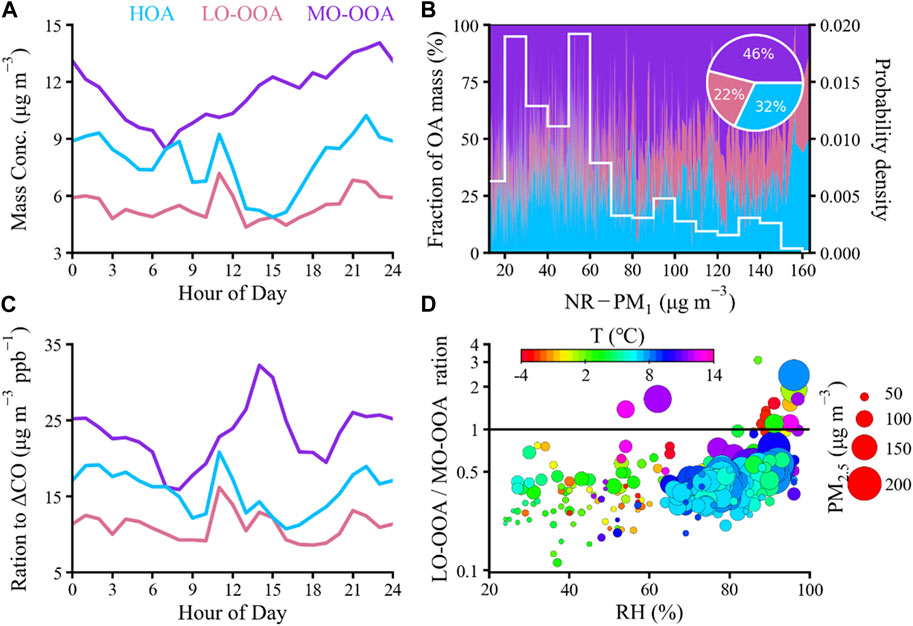
FIGURE 4. (A) Diurnal variations of OA sources (HOA, LO-OOA, and MO-OOA). (B) Variation of OA compositions as a function of NR-PM1 mass concentrations. The probabilities of PM1 mass in each study are also shown on the right axes, and the average composition fractional pie chart of OA factors is inserted. (C) Diurnal variations of OA sources normalized by △CO. (D) Variations of LO-OOA/MO-OOA ratios as a function of RH.
Two OOA factors were resolved by PMF in urban areas, i.e., LO-OOA and MO-OOA, corresponding to fresh SOA and aged SOA (Jimenez et al., 2009; Duan et al., 2020), and OOA was characterized by high signals at m/z 43 (C3H7+ or C2H3O+) and m/z 44 (CO2+) (Xu et al., 2016; Wang et al., 2017). LO-OOA accounts for 40% of m/z 43% and 32% of m/z 44 signals, whereas MO-OOA contributes 27% of m/z 43% and 68% of m/z 44 signals. LO-OOA and MO-OOA constitute 22% and 46% of the total OA mass, respectively, which is consistent with early studies (Huang et al., 2010; Sun et al., 2010). The diurnal total contribution of OOA (LO-OOA + MO-OOA) to OA varied between 29% and 78% in the wintertime of Shanghai (Figure 4B), indicating the significant role of SOA in air pollution throughout the day. The diurnal cycle of MO-OOA reaches the trough in the morning (08:00 LT) and peaks in the midnight (23:00 LT), probably owing to the effect of regional transport and the decrease of PBL height in the evening. Moreover, MO-OOA increased stably during daytime, suggesting that photochemistry is an important process of atmospheric aging. The time series of LO-OOA correlated weakly with MO-OOA (R2 = 0.37, Supplementary Table S3), but the resulting linear combination indicates the evolution of SOA. Meanwhile, LO-OOA showed a good correlation with certain primary species, such as, HOA (R2 = 0.72) and chloride (R2 = 0.58), implying that LO-OOA is more likely to be produced from local sources or combustion in winter. LO-OOA correlated strongly with nitrate (R2 = 0.65) which is associated with photochemical oxidation, and MO-OOA presented a strong correlation with sulfate (R2 = 0.76) which is related to aqueous-phase oxidation processing. This result is consistent with previous studies in urban areas (Zhang et al., 2011; Sun et al., 2014; Zhang et al., 2015a; Xu et al., 2017). However, Zhan et al. (2021) in rural region illustrated a contrary result that the temporal variation of sulfate correlated better with LO-OOA (R2 = 0.73) than with MO-OOA (R2 = 0.34), while nitrate presented tighter correlation with MO-OOA (R2 = 0.68) than with LO-OOA (R2 = 0.40), hinting the different formation processes of OOA in urban and rural environments. Furthermore, OA is predominantly derived from industrial and vehicle emissions in urban areas, while OA in rural region primarily comes from biomass burning and coal combustion providing sufficient precursors for the conversion processes of OOA (Kuang et al., 2020).
3.3 Evolution from clean days to polluted days
To better elucidate aerosol sources and atmospheric evolution processes during the winter campaign in Shanghai, we selected NR-PM1 composition and OA sources on clean episodes (mean NR-PM1 < 25 μg·m−3), moderate-pollution episodes (M-pollution; 40 μg·m−3 < mean e NR-PM1 < 70 μg·m−3), and high-pollution episodes (H-pollution; mean NR-PM1 > 70 μg·m−3) for further analysis. The mass concentrations of gaseous pollutants, NR-PM1 species, and OA components, as well as meteorological parameters for the clean and pollution periods are summarized in Supplementary Table S1. As shown in Figure 5 and Supplementary Table S1, the mean concentration of NR-PM1 in the H-pollution episodes (92.6 μg·m−3) was 2 and 4 times larger than those on M-pollution episodes (55.2 μg·m−3) and clean episodes (21.7 μg·m−3), respectively. Specifically, the NR-PM1 composition exhibited an increase in secondary inorganic aerosol (SIA: SO42−, NO3− and NH4+) contribution from clean episodes (49%) to pollution episodes (59%), while OA contribution decreased accordingly from 48% to 39%. This evolution trend emphasized the significant enhancements in the formation of SIA during haze pollution episodes (Huang et al., 2014; Zheng et al., 2015; Duan et al., 2019). Additionally, the mass concentrations of OA increased substantially from clean episodes (10.4 μg·m−3) to M-pollution episodes (23.8 μg·m−3) and further to H-pollution episodes (35.7 μg·m−3). Despite a decrease in their mass fraction from 75% during clean days to 71% during H-pollution days, the mass concentrations of SOA (LO-OOA and MO-OOA) increased by 3 times. The increasing rates in LO-OOA levels from clean days to pollution days were slightly larger than that of MO-OOA; thus, the contribution of LO-OOA to OA increased from 17% during clean days to 22% during M-pollution days and further to 25% during H-pollution days, whereas the contribution of MO-OOA to OA declined from 58% during clean days to 53% during M-pollution days and further to 46% during H-pollution days. This observation is consistent with the result reported by Duan et al. (2020) for wintertime in Beijing. In comparison with SOA, the fraction of POA (HOA) in OA increased from 25% during clean days to 29% during H-pollution days, suggesting that primary contribution is as important as secondary contribution for OA during extreme haze events.
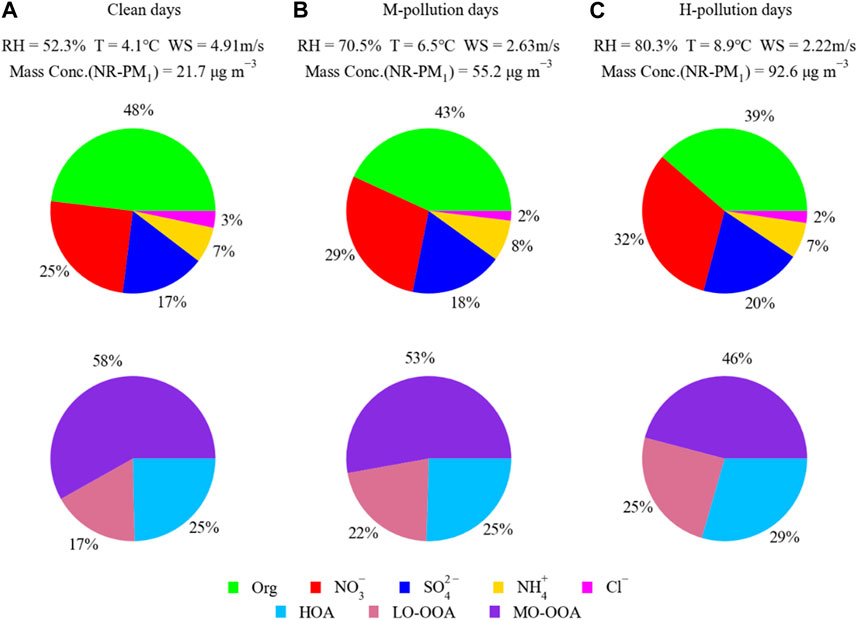
FIGURE 5. Relative contributions of NR-PM1 species and OA sources on clean days (A), M-pollution days (B) and H-pollution days (C). We denote the days with daily average NR-PM1 mass concentration below 25 μg·m−3 as the clean days, within the range from 40 to 70 μg·m−3 as the M-pollution days, and higher than 70 μg·m−3 as the H-pollution days in the wintertime of Shanghai.
The clean and pollution episodes occurred in “sawtooth cycles,” in which local emissions, regional transport, secondary formation, and meteorological conditions intertwine and play different roles in the evolution processes of PM pollution. It should be noted that the rise of SIA and SOA are accompanied with decreased wind speed and increased RH and T (Figure 5), suggesting that meteorological conditions played a very important role in the formation of secondary aerosol in highly polluted days in Shanghai. The mass fractions of NR-PM1 species and the variations of meteorological parameters (RH, T, and WS) as a function of NR-PM1 mass were shown in Figure 6. In general, the pollution episodes were related to higher RH and T, as well as lower wind speeds compared with clean episodes (Figures 5, 6). RH was usually higher than 65% during pollution episodes and lower than 55% during clean episodes in the winter of Shanghai (Figure 2). Additionally, the substantial surge of sulfate in high-RH pollution episodes (Figures 5, 6) indicated that the aqueous-phase oxidation of SO2 could be a significant mechanism for the formation of sulfate during particulate air pollution period, which is in good agreement with prior studies (Elser et al., 2016; Sun et al., 2016; Wang et al., 2017). The contribution of nitrate was elevated from 25% during clean episodes to 32% during polluted episodes, likely owing to increasing NO2 as well as the increase of RH which facilitates the partitioning of hydrophilic NH4NO3 from gas phase into particle phase and the hydrolysis of N2O5. In comparation with SIAs, OOA formation is still very uncertain and considerably more ambiguous, especially during particulate pollution period. To gain a better comprehension of the factors governing SOA formation, we further evaluate the correlation of SOA with meteorological conditions (e.g., RH) and atmospheric oxidative capacity (Ox = O3 + NO2) in next section.
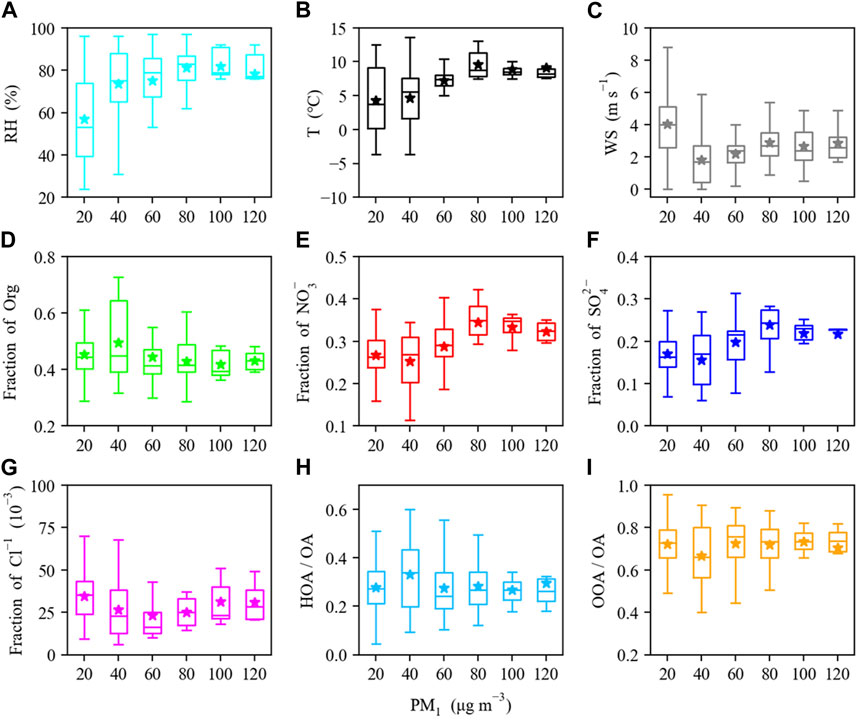
FIGURE 6. (A) Relative humidity, (B) temperature, (C) wind speed, and the mass fraction of (D) organics, (E) nitrate, (F) sulfate, and (G) chloride in NR-PM1, as well as the mass fraction of (H) HOA and (I) OOA in OA as a function of NR-PM1 mass loadings. The data are binned according to the total mass, and mean (cross), median (horizontal line), 25th and 75th percentiles (lower and upper box), and 10th and 90th percentiles (lower and upper whiskers) are shown for each bin.
3.4 Influence of aqueous-phase chemistry and photochemical oxidation on SOA formation
To further elucidate the formation mechanisms of secondary aerosol, the relationships between two SOA factors (MO-OOA and LO-OOA) and Ox or ALWC were analyzed. It should be noted that Ox and ALWC are widely employed to characterize the photochemical oxidation and aqueous-phase process of SOA, respectively (Xu et al., 2017; Duan et al., 2020). In comparison to O3, Ox has been proven to be a more conserved tracer for photochemical processing on account of the reaction of NO with O3 to form NO2 (Herndon et al., 2008). It is clear that ALWC showed a good correlation with RH (R2 = 0.63), suggesting the potential effects of aqueous-phase chemistry at high-RH levels.
The difference in the growth rates of LO-OOA and MO-OOA during daytime indicated a potential distinction in their formation (Figure 4C). To better understand the role of photochemical oxidation in SOA formation, we investigated the relationship between the fraction of LO-OOA or MO-OOA in OA (fLO-OOA or fMO-OOA) and Ox during the day. Figure 7 showed the daytime variations of fLO-OOA and fMO-OOA versus Ox during the entire measurement period. Although both LO-OOA and MO-OOA exhibited overall increasing trends with an increase in the Ox levels, the increasing rate of fMO-OOA was larger than that of fLO-OOA. For instance, fMO-OOA ranged from 41% to 59% and yet fLO-OOA remained largely unchanged, with Ox levels ranging from 75 to 135 μg·m−3. Meanwhile, Ox correlated tighter with MO-OOA (R2 = 0.51) than LO-OOA (R2 = 0.17) (see in Supplementary Table S3), indicating that photochemical oxidation could play a more significant role in the formation of MO-OOA, which is consistent with a recent study (Zhan et al., 2021). In comparison, the positive correlation of ALWC was stronger with LO-OOA (R2 = 0.54) than with MO-OOA (R2 = 0.26), indicating aqueous-phase processing was of vital importance in LO-OOA formation. Furthermore, LO-OOA/MO-OOA exhibited the largest value in the upper right corner with high RH in winter (Figure 4D), further suggesting that aqueous-phase processing likely played a more important role in LO-OOA formation. As shown in Figure 4D, the peak mass concentration of PM2.5 was concentrated under high-RH conditions (60%–100%). LO-OOA/MO-OOA mass ratios increased significantly as RH rose during high-PM2.5 periods, implying that aqueous-phase chemistry played a significant role in LO-OOA formation during haze episodes. Conversely, MO-OOA during low-RH periods was more important. Furthermore, LO-OOA/MO-OOA ratio presented a negligible relationship with T at the same level of RH. Meanwhile, both LO-OOA and MO-OOA revealed no clear correlation with T, and the no clear correlation of T with Ox or RH was also observed (Supplementary Table S3), indicating a more complicated impact of T on the formation of SOA in our study.
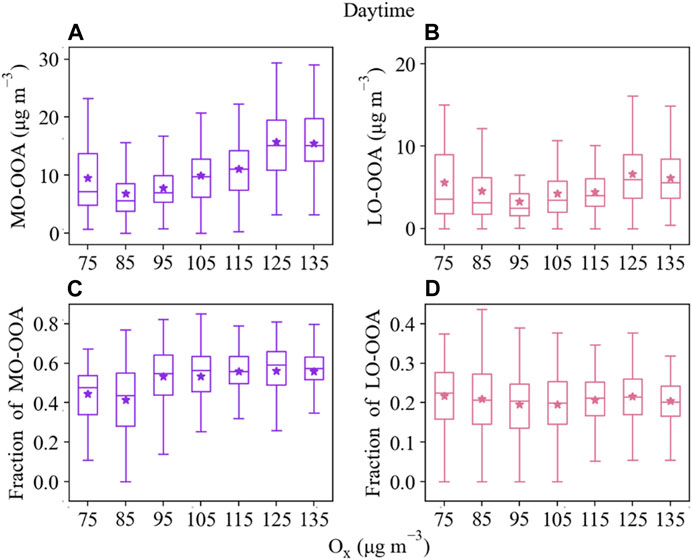
FIGURE 7. The mass concentration of MO-OOA (A) and LO-OOA (B), as well as fMO-OOA (C) and fMO-OOA (D) as a function of Ox during the daytime. The data were binned according to the Ox concentration (10 μg·m−3 increment), and mean (star), median (horizontal line), and 5th and 95th percentiles (lower and upper whiskers) were displayed for data in each bin.
Photochemical and aqueous-phase processes exerted a combined influence on the formation of SOA (Xu et al., 2017; Duan et al., 2020). The combined influence of Ox and ALWC on the concentration and composition of OOA during the clean and H-pollution episodes are illustrated in Figure 8. The highest values of OOA mass concentrations mainly appeared under large ALWC levels (100–300 μg·m−3) accompanied by moderate Ox levels (70–110 μg·m−3). During H-pollution episodes (Figure 8D), the LO-OOA/MO-OOA ratios and OOA concentrations exhibited similar distributions, attaining high values at in the top left corner. This indicated that aqueous-phase chemistry processes dominated LO-OOA formation, which was also supported by the peak value of LO-OOA level during nighttime. Meanwhile, LO-OOA also exhibited a distinct peak at noon, suggesting that Ox may also affect LO-OOA formation. In comparison, LO-OOA/MO-OOA ratios during clean episodes were less than 0.6 under low ALWC levels (2–47 μg·m−3) and OOA concentrations attained high values at in the top right corner, which further indicated that aqueous-phase processes perhaps facilitate the formation of LO-OOA, whereas MO-OOA formation is promoted by gas-phase photochemical oxidation. In general, the difference in the diurnal patterns of LO-OOA or MO-OOA during clean and H-pollution episodes suggested a potential difference in their formation under different aerosol conditions (Figures 8A, C).
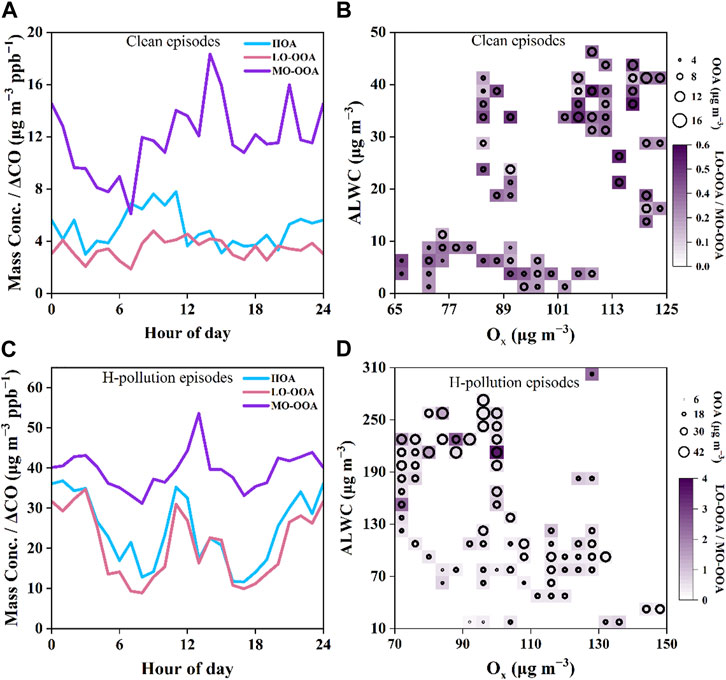
FIGURE 8. Diurnal patterns of the three types of OA (HOA, LO-OOA, and MO-OOA) during (A) the clean episodes and (C) H-pollution episodes; AWLC and Ox dependence of the OOA concentration and LO-OOA/MO-OOA ratio during (B) the clean episodes and (D) H-pollution episodes, respectively.
4 Conclusion
In this study, an Aerosol Chemical Speciation Monitor (ACSM, Aerodyne Research Inc.) was deployed in a megacity of China, Shanghai from 10 to 21 January 2018. The non-refractory submicron aerosol (NR-PM1) is predominated by organics (43%), followed by nitrate (29%), sulfate (18%), ammonium (7%) and chloride (3%). Regarding the OA factors, three OA factors were identified and classified as HOA, LO-OOA, and MO-OOA, which contributed 32%, 22%, and 46% to total OA, respectively. The NR-PM1 composition exhibited an increase in SIA (sulfate, nitrate and ammonium) contribution from clean episodes (49%) to pollution episodes (59%), while OA contribution decreased accordingly from 48% to 39%. The strong increase of sulfate in high-RH pollution episodes indicated that aqueous-phase oxidation of SO2 could be an important formation process for sulfate during particulate air pollution period. The contribution of nitrate was elevated from 25% during clean episodes to 32% during polluted episodes, likely owing to the increase of RH which facilitates the hydrolysis of N2O5 and the gas-to-particle partitioning of hydrophilic NH4NO3. We explored the formation mechanisms of SOA and found the good correlations of LO-OOA and MO-OOA with ALWC and Ox, respectively, indicating that aqueous-phase processes perhaps facilitates LO-OOA formation, whereas MO-OOA formation is promoted by gas-phase photochemical oxidation. Meanwhile, LO-OOA exhibited a distinct peak at noon under high-RH condition, suggesting that Ox may also affect the formation of LO-OOA.
Data availability statement
The datasets presented in this article are not readily available because this dataset is regulated by government agencies. Requests to access the datasets should be directed to DC, dongmei_cai@fudan.edu.cn.
Author contributions
Data curation, YQ, DC, MZ, XH, JH, and YD; Formal analysis, YQ; Supervision, TC; Writing—original draft, YQ.; Writing—review and editing, DC and TC. All authors have read and agreed to the published version of the manuscript.
Funding
This work was supported by the National Natural Science Foundation of China (No. 42175179) and China Postdoctoral Science Foundation (No. 2022M720031).
Conflict of interest
The authors declare that the research was conducted in the absence of any commercial or financial relationships that could be construed as a potential conflict of interest.
Publisher’s note
All claims expressed in this article are solely those of the authors and do not necessarily represent those of their affiliated organizations, or those of the publisher, the editors and the reviewers. Any product that may be evaluated in this article, or claim that may be made by its manufacturer, is not guaranteed or endorsed by the publisher.
Supplementary material
The Supplementary Material for this article can be found online at: https://www.frontiersin.org/articles/10.3389/fenvs.2023.1199652/full#supplementary-material
References
Budisulistiorini, S. H., Baumann, K., Edgerton, E. S., Bairai, S. T., Mueller, S., Shaw, S. L., et al. (2016). Seasonal characterization of submicron aerosol chemical composition and organic aerosol sources in the southeastern United States: Atlanta, Georgia,and Look Rock, Tennessee. Atmos. Chem. Phys. 16 (8), 5171–5189. doi:10.5194/acp-16-5171-2016
Canagaratna, M. R., Jayne, J. T., Ghertner, D. A., Herndon, S., Shi, Q., Jimenez, J. L., et al. (2004). Chase studies of particulate emissions from in-use New York City vehicles. Aerosol Sci. Technol. 38 (6), 555–573. doi:10.1080/02786820490465504
Canagaratna, M. R., Jayne, J. T., Jimenez, J. L., Allan, J. D., Alfarra, M. R., Zhang, Q., et al. (2007). Chemical and microphysical characterization of ambient aerosols with the aerodyne aerosol mass spectrometer. Mass Spectrom. Rev. 26 (2), 185–222. doi:10.1002/mas.20115
Canonaco, F., Crippa, M., Slowik, J. G., Baltensperger, U., and &Prevot, A. S. H. (2013). SoFi, an IGOR-based interface for the efficient use of the generalized multilinear engine (ME-2) for the source apportionment: ME-2 application to aerosol mass spectrometer data. Atmos. Meas. Tech. 6 (12), 3649–3661. doi:10.5194/amt-6-3649-2013
Cao, J.-J., Shen, Z.-X., Chow, J. C., Watson, J. G., Lee, S.-C., Tie, X.-X., et al. (2012). Winter and summer PM2.5 chemical compositions in fourteen Chinese cities. J. Air and Waste Manag. Assoc. 62 (10), 1214–1226. doi:10.1080/10962247.2012.701193
Cohen, A. J., Brauer, M., Burnett, R., Anderson, H. R., Frostad, J., Estep, K., et al. (2017). Estimates and 25-year trends of the global burden of disease attributable to ambient air pollution: An analysis of data from the global burden of diseases study 2015. Lancet 389 (10082), 1907–1918. doi:10.1016/s0140-6736(17)30505-6
Crenn, V., Sciare, J., Croteau, P. L., Verlhac, S., Froehlich, R., Belis, C. A., et al. (2015). ACTRIS ACSM intercomparison - Part 1: Reproducibility of concentration and fragment results from 13 individual Quadrupole Aerosol Chemical Speciation Monitors (Q-ACSM) and consistency with co-located instruments. Atmos. Meas. Tech. 8 (12), 5063–5087. doi:10.5194/amt-8-5063-2015
DeCarlo, P. F., Ulbrich, I. M., Crounse, J., de Foy, B., Dunlea, E. J., Aiken, A. C., et al. (2010). Investigation of the sources and processing of organic aerosol over the Central Mexican Plateau from aircraft measurements during MILAGRO. Atmos. Chem. Phys. 10 (12), 5257–5280. doi:10.5194/acp-10-5257-2010
Duan, J., Huang, R.-J., Li, Y., Chen, Q., Zheng, Y., Chen, Y., et al. (2020). Summertime and wintertime atmospheric processes of secondary aerosol in Beijing. Atmos. Chem. Phys. 20 (6), 3793–3807. doi:10.5194/acp-20-3793-2020
Duan, J., Huang, R.-J., Lin, C., Dai, W., Wang, M., Gu, Y., et al. (2019). Distinctions in source regions and formation mechanisms of secondary aerosol in Beijing from summer to winter. Atmos. Chem. Phys. 19 (15), 10319–10334. doi:10.5194/acp-19-10319-2019
Duan, J., Huang, R. J., Gu, Y., Lin, C., Zhong, H., Xu, W., et al. (2022). Measurement report: Large contribution of biomass burning and aqueous-phase processes to the wintertime secondary organic aerosol formation in Xi'an, Northwest China. Atmos. Chem. Phys. 22 (15), 10139–10153. doi:10.5194/acp-22-10139-2022
Elser, M., Huang, R.-J., Wolf, R., Slowik, J. G., Wang, Q., Canonaco, F., et al. (2016). New insights into PM2.5 chemical composition and sources in two major cities in China during extreme haze events using aerosol mass spectrometry. Atmos. Chem. Phys. 16 (5), 3207–3225. doi:10.5194/acp-16-3207-2016
Fountoukis, C., and Nenes, A. (2007). Isorropia II: A computationally efficient thermodynamic equilibrium model for K+-Ca2+-Mg2+-nh(4)(+)-Na+-SO42--NO3--Cl--H2O aerosols. Atmos. Chem. Phys. 7 (17), 4639–4659. doi:10.5194/acp-7-4639-2007
Gao, J., Qiao, L.-p., Lou, Y.-r., Yan, R.-s., Zhou, M., Liu, Y.-c., et al. (2019). Secondary aerosol formation in urban Shanghai: Insights into the roles of photochemical oxidation and aqueous-phase reaction. Huanjing Kexue 40 (6), 2510–2518. doi:10.13227/j.hjkx.201812067
Gu, Y., Huang, R.-J., Li, Y., Duan, J., Chen, Q., Hu, W., et al. (2020). Chemical nature and sources of fine particles in urban Beijing: Seasonality and formation mechanisms. Environ. Int. 140, 105732. doi:10.1016/j.envint.2020.105732
Guo, H., Xu, L., Bougiatioti, A., Cerully, K. M., Capps, S. L., Hite, J. R., et al. (2015). Fine-particle water and pH in the southeastern United States. Atmos. Chem. Phys. 15 (9), 5211–5228. doi:10.5194/acp-15-5211-2015
Herndon, S. C., Onasch, T. B., Wood, E. C., Kroll, J. H., Canagaratna, M. R., Jayne, J. T., et al. (2008). Correlation of secondary organic aerosol with odd oxygen in Mexico City. Geophys. Res. Lett. 35 (15), L15804. doi:10.1029/2008gl034058
Hu, W., Hu, M., Hu, W.-W., Zheng, J., Chen, C., Wu, Y., et al. (2017). Seasonal variations in high time-resolved chemical compositions, sources, and evolution of atmospheric submicron aerosols in the megacity Beijing. Atmos. Chem. Phys. 17 (16), 9979–10000. doi:10.5194/acp-17-9979-2017
Huang, R.-J., Wang, Y., Cao, J., Lin, C., Duan, J., Chen, Q., et al. (2019). Primary emissions versus secondary formation of fine particulate matter in the most polluted city (Shijiazhuang) in North China. Atmos. Chem. Phys. 19 (4), 2283–2298. doi:10.5194/acp-19-2283-2019
Huang, R.-J., Zhang, Y., Bozzetti, C., Ho, K.-F., Cao, J.-J., Han, Y., et al. (2014). High secondary aerosol contribution to particulate pollution during haze events in China. Nature 514 (7521), 218–222. doi:10.1038/nature13774
Huang, X. F., He, L. Y., Hu, M., Canagaratna, M. R., Sun, Y., Zhang, Q., et al. (2010). Highly time-resolved chemical characterization of atmospheric submicron particles during 2008 beijing olympic games using an aerodyne high-resolution aerosol mass spectrometer. Atmos. Chem. Phys. 10 (18), 8933–8945. doi:10.5194/acp-10-8933-2010
Huang, X. F., He, L. Y., Xue, L., Sun, T. L., Zeng, L. W., Gong, Z. H., et al. (2012). Highly time-resolved chemical characterization of atmospheric fine particles during 2010 Shanghai World Expo. Atmos. Chem. Phys. 12 (11), 4897–4907. doi:10.5194/acp-12-4897-2012
Huo, Q., Cai, X., Kang, L., Zhang, H., Song, Y., and &Zhu, T. (2015). Estimating ammonia emissions from a winter wheat cropland in North China Plain with field experiments and inverse dispersion modeling. Atmos. Environ. 104, 1–10. doi:10.1016/j.atmosenv.2015.01.003
Jimenez, J. L., Canagaratna, M. R., Donahue, N. M., Prevot, A. S. H., Zhang, Q., Kroll, J. H., et al. (2009). Evolution of organic aerosols in the atmosphere. Science 326 (5959), 1525–1529. doi:10.1126/science.1180353
Kuang, Y., He, Y., Xu, W., Yuan, B., Zhang, G., Ma, Z., et al. (2020). Photochemical aqueous-phase reactions induce rapid daytime formation of oxygenated organic aerosol on the north China plain. Environ. Sci. Technol. 54 (7), 3849–3860. doi:10.1021/acs.est.9b06836
Li, H., Zhang, Q., Zhang, Q., Chen, C., Wang, L., Wei, Z., et al. (2017). Wintertime aerosol chemistry and haze evolution in an extremely polluted city of the north China plain: Significant contribution from coal and biomass combustion. Atmos. Chem. Phys. 17 (7), 4751–4768. doi:10.5194/acp-17-4751-2017
Li, Y. J., Lee, B. P., Su, L., Fung, J. C. H., and &Chan, C. K. (2015). Seasonal characteristics of fine particulate matter (PM) based on high-resolution time-of-flight aerosol mass spectrometric (HR-ToF-AMS) measurements at the HKUST Supersite in Hong Kong. Atmos. Chem. Phys. 15 (1), 37–53. doi:10.5194/acp-15-37-2015
Middlebrook, A. M., Bahreini, R., Jimenez, J. L., and &Canagaratna, M. R. (2012). Evaluation of composition-dependent collection efficiencies for the aerodyne aerosol mass spectrometer using field data. Aerosol Sci. Technol. 46 (3), 258–271. doi:10.1080/02786826.2011.620041
Mohr, C., Huffman, J. A., Cubison, M. J., Aiken, A. C., Docherty, K. S., Kimmel, J. R., et al. (2009). Characterization of primary organic aerosol emissions from meat cooking, trash burning, and motor vehicles with high-resolution aerosol mass spectrometry and comparison with ambient and chamber observations. Environ. Sci. Technol. 43 (7), 2443–2449. doi:10.1021/es8011518
Ng, N. L., Canagaratna, M. R., Jimenez, J. L., Zhang, Q., Ulbrich, I. M., and &Worsnop, D. R. (2011a). Real-time methods for estimating organic component mass concentrations from aerosol mass spectrometer data. Environ. Sci. Technol. 45 (3), 910–916. doi:10.1021/es102951k
Ng, N. L., Herndon, S. C., Trimborn, A., Canagaratna, M. R., Croteau, P. L., Onasch, T. B., et al. (2011b). An aerosol chemical speciation monitor (ACSM) for routine monitoring of the composition and mass concentrations of ambient aerosol. Aerosol Sci. Technol. 45 (7), 780–794. doi:10.1080/02786826.2011.560211
Paatero, P. (1999). The multilinear engine - a table-driven, least squares program for solving multilinear problems, including the n-way parallel factor analysis model. J. Comput. Graph. Statistics 8 (4), 854–888. doi:10.2307/1390831
Song, S., Gao, M., Xu, W., Shao, J., Shi, G., Wang, S., et al. (2018). Fine-particle pH for Beijing winter haze as inferred from different thermodynamic equilibrium models. Atmos. Chem. Phys. 18 (10), 7423–7438. doi:10.5194/acp-18-7423-2018
Sun, J., Zhang, Q., Canagaratna, M. R., Zhang, Y., Ng, N. L., Sun, Y., et al. (2010). Highly time- and size-resolved characterization of submicron aerosol particles in Beijing using an Aerodyne Aerosol Mass Spectrometer. Atmos. Environ. 44 (1), 131–140. doi:10.1016/j.atmosenv.2009.03.020
Sun, Y., Du, W., Fu, P., Wang, Q., Li, J., Ge, X., et al. (2016). Primary and secondary aerosols in beijing in winter: Sources, variations and processes. Atmos. Chem. Phys. 16 (13), 8309–8329. doi:10.5194/acp-16-8309-2016
Sun, Y., Jiang, Q., Wang, Z., Fu, P., Li, J., Yang, T., et al. (2014). Investigation of the sources and evolution processes of severe haze pollution in Beijing in January 2013. J. Geophys. Research-Atmospheres 119 (7), 4380–4398. doi:10.1002/2014jd021641
Sun, Y. L., Wang, Z. F., Du, W., Zhang, Q., Wang, Q. Q., Fu, P. Q., et al. (2015). Long-term real-time measurements of aerosol particle composition in beijing, China: Seasonal variations, meteorological effects, and source analysis. Atmos. Chem. Phys. 15 (17), 10149–10165. doi:10.5194/acp-15-10149-2015
Sun, Y. L., Wang, Z. F., Fu, P. Q., Yang, T., Jiang, Q., Dong, H. B., et al. (2013). Aerosol composition, sources and processes during wintertime in Beijing, China. Atmos. Chem. Phys. 13 (9), 4577–4592. doi:10.5194/acp-13-4577-2013
Sun, Y., Wang, Z., Dong, H., Yang, T., Li, J., Pan, X., et al. (2012). Characterization of summer organic and inorganic aerosols in beijing, China with an aerosol chemical speciation monitor. Atmos. Environ. 51, 250–259. doi:10.1016/j.atmosenv.2012.01.013
Wang, Q., Sun, Y., Jiang, Q., Du, W., Sun, C., Fu, P., et al. (2015). Chemical composition of aerosol particles and light extinction apportionment before and during the heating season in Beijing, China. J. Geophys. Research-Atmospheres 120 (24), 12708–12722. doi:10.1002/2015jd023871
Wang, Y. C., Huang, R. J., Ni, H. Y., Chen, Y., Wang, Q. Y., Li, G. H., et al. (2017). Chemical composition, sources and secondary processes of aerosols in Baoji city of northwest China. Atmos. Environ. 158, 128–137. doi:10.1016/j.atmosenv.2017.03.026
Wang, Y., Hu, M., Wang, Y.-C., Li, X., Fang, X., Tang, R., et al. (2020). Comparative study of particulate organosulfates in contrasting atmospheric environments: Field evidence for the significant influence of anthropogenic sulfate and NOx. Environ. Sci. Technol. Lett. 7 (11), 787–794. doi:10.1021/acs.estlett.0c00550
Xu, J., Shi, J., Zhang, Q., Ge, X., Canonaco, F., Prevot, A. S. H., et al. (2016). Wintertime organic and inorganic aerosols in Lanzhou, China: Sources, processes, and comparison with the results during summer. Atmos. Chem. Phys. 16 (23), 14937–14957. doi:10.5194/acp-16-14937-2016
Xu, W., Han, T., Du, W., Wang, Q., Chen, C., Zhao, J., et al. (2017). Effects of aqueous-phase and photochemical processing on secondary organic aerosol formation and evolution in beijing, China. Environ. Sci. Technol. 51 (2), 762–770. doi:10.1021/acs.est.6b04498
Xu, W., Sun, Y., Wang, Q., Zhao, J., Wang, J., Ge, X., et al. (2019). Changes in aerosol chemistry from 2014 to 2016 in winter in beijing: Insights from high-resolution aerosol mass spectrometry. J. Geophys. Research-Atmospheres 124 (2), 1132–1147. doi:10.1029/2018jd029245
Zhan, B., Zhong, H., Chen, H., Chen, Y., Li, X., Wang, L., et al. (2021). The roles of aqueous-phase chemistry and photochemical oxidation in oxygenated organic aerosols formation. Atmos. Environ. 266, 118738. doi:10.1016/j.atmosenv.2021.118738
Zhang, J. K., Sun, Y., Liu, Z. R., Ji, D. S., Hu, B., Liu, Q., et al. (2014). Characterization of submicron aerosols during a month of serious pollution in Beijing. Atmos. Chem. Phys. 14 (6), 2887–2903. doi:10.5194/acp-14-2887-2014
Zhang, Q. J., Beekmann, M., Freney, E., Sellegri, K., Pichon, J. M., Schwarzenboeck, A., et al. (2015a). Formation of secondary organic aerosol in the Paris pollution plume and its impact on surrounding regions. Atmos. Chem. Phys. 15 (24), 13973–13992. doi:10.5194/acp-15-13973-2015
Zhang, Q., Jimenez, J. L., Canagaratna, M. R., Ulbrich, I. M., Ng, N. L., Worsnop, D. R., et al. (2011). Understanding atmospheric organic aerosols via factor analysis of aerosol mass spectrometry: A review. Anal. Bioanal. Chem. 401 (10), 3045–3067. doi:10.1007/s00216-011-5355-y
Zhang, Y. J., Tang, L. L., Wang, Z., Yu, H. X., Sun, Y. L., Liu, D., et al. (2015b). Insights into characteristics, sources, and evolution of submicron aerosols during harvest seasons in the Yangtze River delta region, China. Atmos. Chem. Phys. 15 (3), 1331–1349. doi:10.5194/acp-15-1331-2015
Zheng, G. J., Duan, F. K., Su, H., Ma, Y. L., Cheng, Y., Zheng, B., et al. (2015). Exploring the severe winter haze in beijing: The impact of synoptic weather, regional transport and heterogeneous reactions. Atmos. Chem. Phys. 15 (6), 2969–2983. doi:10.5194/acp-15-2969-2015
Zhu, W., Guo, S., Zhang, Z., Wang, H., Yu, Y., Chen, Z., et al. (2021a). Mass spectral characterization of secondary organic aerosol from urban cooking and vehicular sources. Atmos. Chem. Phys. 21 (19), 15065–15079. doi:10.5194/acp-21-15065-2021
Keywords: non-refractory submicron aerosols (NR-PM1), aerosol chemical speciation monitor (ACSM), secondary organic aerosol (SOA), aqueous-phase oxidation, Shanghai
Citation: Qian Y, Cai D, Zhang M, Huang X, Huo J, Duan Y and Cheng T (2023) Chemical composition, sources and evolution of wintertime inorganic and organic aerosols in urban Shanghai, China. Front. Environ. Sci. 11:1199652. doi: 10.3389/fenvs.2023.1199652
Received: 03 April 2023; Accepted: 27 April 2023;
Published: 09 May 2023.
Edited by:
Liubin Huang, Shandong University, ChinaReviewed by:
Hengqing Shen, Shandong University, ChinaYan Lyu, Zhejiang University of Technology, China
Copyright © 2023 Qian, Cai, Zhang, Huang, Huo, Duan and Cheng. This is an open-access article distributed under the terms of the Creative Commons Attribution License (CC BY). The use, distribution or reproduction in other forums is permitted, provided the original author(s) and the copyright owner(s) are credited and that the original publication in this journal is cited, in accordance with accepted academic practice. No use, distribution or reproduction is permitted which does not comply with these terms.
*Correspondence: Dongmei Cai, dongmei_cai@fudan.edu.cn; Tiantao Cheng, ttcheng@fudan.edu.cn