- 1Business School and MBA Center, Henan University of Science and Technology, Luoyang, China
- 2College of Economics, Tianjin Normal University, Tianjin, China
- 3Institute of Resources, Environment and Ecology, Tianjin Academy of Social Sciences, Tianjin, China
Improving agricultural eco-efficiency (AEE) is a promising way to achieve the sustainability of agricultural development. Although AEE evaluation and driving forces were widely explored, few studies have systematically examined how urbanization, the core driving force, affected AEE. To supplement the existing literature, the GB-US-SBM model was used to assess AEE in China during 2004–2020. Furtherly, it used the mediation effect model to investigate how urbanization influenced the AEE in different agriculture development regions by reducing agricultural labor and changing rural residents’ income. The results showed that: 1) During 2004–2020, China’s AEE revealed a stable improvement, with the mean score increasing from 0.138 to 0.744. Regarding spatial distribution, AEE exhibited a gradient decrease: optimized development region > moderate development region > protected development region. 2) Urbanization had a significantly positive effect on AEE, with the magnitude of the effect greatest in the protected development region, followed by the moderate development region and the optimized development region. 3) The mediator variables, agricultural labor and rural residents’ income, positively mediated the relationship between urbanization and AEE, and the former had a larger mediating effect. Notably, rural residents’ income did not mediate the effect of urbanization on AEE in the protected development region. According to the findings, to improve sustainable agriculture development and urbanization development in China, some policy suggestions were put forward from the aspects of transforming agricultural development mode, accelerating the urbanization process, enhancing vocational education for the middle-aged and young rural labor force, and expanding channels for increasing rural residents’ income.
1 Introduction
China has witnessed an unprecedented urbanization process (Wang et al., 2021b; Cai et al., 2021). China’s population urbanization rate increased from 17.9% in 1978 to 64.72% in 2021, with a rapid increase of more than one percentage point per year (Cao et al., 2021). Just as the Nobel laureate in economics Joseph E. Stiglitz once said: “high-tech development in the United States and China’s urbanization would be two key factors affecting the process of human society development in the 21st century” (Guan et al., 2018). All countries depend on agriculture to provide food (Karimi Alavijeh et al., 2022), especially China, one of the world’s most populous developing countries and the world’s largest food consumer (Cao et al., 2021). Notably, urbanization demonstrated an increasing impact on agricultural development (She, 2015; Hou et al., 2019; Jayadevan, 2020). Thus, the relationship between urbanization and agricultural development has emerged as hotspot of academic attention (Chen et al., 2022; Yang et al., 2022).
Although urbanization absorbed the rural surplus labor and increased the rural residents’ income (Cai, 2017; Li and Li, 2019), it brought tremendous pressure on cultivated land utilization and led to agricultural water resource shortage and fertilizer overuse (Bren d’Amour et al., 2017; Jiang and Li, 2016; Peng, 2011; Zhou et al., 2021), resulting in the deterioration of the agricultural ecological environment (Guo et al., 2021). Ministry of Agriculture (2015) China’s Agricultural Sustainable Development Plan (2015–2030) revealed that China’s agri-environmental pollution was prominent, with a utilization rate of fertilizers and pesticides of less than one-third and a recovery rate of agricultural film of less than two-thirds (Liu et al., 2020b). Moreover, according to the Asian Development Bank, the direct economic loss due to the deterioration of resources and environment in China’s agriculture accounted for 0.1%–1% of the Gross Domestic Product (GDP) each year (Nie and Yu, 2017). Meanwhile, the tightening of agricultural resources and environmental constraints has restricted China’s agricultural development (Huang et al., 2022). It was estimated that China’s agricultural production efficiency was only 2% of the average level of developed countries and 64% of the global average level (Han et al., 2020; Zhu and Wang, 2021) Given this, achieving sustainable agriculture development is a necessary choice in the process of urbanization (Du et al., 2022).
Eco-efficiency (Schaltegger and Sturm, 1990), which incorporates the economic value and environmental impact of economic activities, can be used as an effective instrument to assess the sustainability of agricultural development (Schmidheiny and Stigson, 2000; Camarero et al., 2013; Rybaczewska-Błażejowska and Gierulski, 2018; Magarey et al., 2019; Gava et al., 2020; Wang and Lin, 2021). In other words, AEE is the process that produces more agricultural output while reducing resource consumption and environmental pollution.
It was observed that factors, including the agricultural economic development level, agricultural labor, agricultural industrial structure, agricultural machinery level and fiscal expenditure on environmental protection, found to be influencing AEE were explored in previous studies (Ma and Feng, 2013; Pang et al., 2016; Fei and Lin, 2017; Hou and Yao, 2018b; Gao and Wang, 2018; Gras and Cáceres, 2020; Xu and Tan, 2020; Zhu et al., 2022b; Jiang et al., 2022). In recent years, there has been an increasing interest in the impact of urbanization on AEE. Zhang (2018) analyzed 11 provinces in Western China and found that population urbanization negatively affected AEE (Zhang, 2018). Besides, Cai et al. (2021) examined the coupling and coordinated development of China’s urbanization and the agricultural ecological environment. It revealed that the two systems were in an antagonistic stage. Previous research on East China showed that urbanization lagged behind AEE, and there was still significant room for improvement in the urbanization development level (Liu et al., 2021).
Contrary to the established conclusions, (Shang et al., 2020) found that urbanization significantly and positively impacted AEE in China’s major grain-producing areas by employing a panel Tobit model. More recently, literature has emerged that offers contradictory findings about the effect of urbanization on AEE. Moreover, to our knowledge, no single study existed that focused on the influencing mechanism of urbanization on AEE in different agricultural development regions.
Given this, the purpose of this study was to extend the existing studies in two areas. 1) According to the National Agricultural Sustainable Development Plan 2015–2030, the 31 provinces of China were divided into three agriculture development regions: optimized development region, moderate development region and protected development region. Due to the different strategy missions of the three regions in sustainable agriculture development, it is urgent to reveal the spatial-temporal characteristics of AEE in different regions. 2) Using the mediation effect model, this study explored the influence of urbanization on AEE through mediator variables, agricultural labor and rural residents’ income, in different agriculture development regions, which provided a reference for formulating policies tailored to local conditions.
2 Literature review
By combing the existing literature, this paper constructed an analytical framework illustrating the impact of urbanization on AEE through agricultural labor and rural residents’ income, as shown in Figure 1.
With the advancement of urbanization, substantial surplus labor left rural and transferred to urban areas (Harris and Todaro, 1970; Antrop, 2004; Zhang et al., 2019). Since 2005, the outward migration of rural labor in China has increased explosively. However, compared with mature economies, rural labor’s surplus and unreasonable allocation in China were much more severe (Kong, 2019; Wu et al., 2019). A comparative analysis of the agricultural labor input of 68 countries found that China’s agricultural labor input redundancy reached 34.5%, higher than average level (Zhang and Chen, 2019). Using a utility man-days conversion model (Zhao et al., 2018) found that the surplus proportion of agricultural labor was 45.25% in 2015. Furthermore, it was predicted that by the end of the 14th Five-Year Plan (2020–2025), the total rural labor in China would be 259 million, among which 116–148 million needed to migrate away from rural (Xie, 2021).
Excessive agricultural labor input is the primary source of AEE loss (Zhang et al., 2021). Urbanization was a promising way to solve excessive agricultural labor input. The outward migration of rural surplus labor alleviated the agricultural involution and improved agricultural production efficiency (Wang et al., 2013; Qi, 2014). Using the panel threshold model, It was found that migration was conducive to promoting AEE when rural labor’s proportion of nonagricultural employment exceeded the threshold value of 44.93% (Hou and Yao, 2018a). Besides, urbanization promoted large-scale farming and environmental protection due to the release of rural labor and rural land resources. In this way, urbanization can effectively improve AEE (Li et al., 2022). In other words, large-scale agriculture operations mediated agricultural labor’s effect on AEE (Zhu et al., 2022b). The sowing area per agricultural labor positively impacted AEE in Henan province (Yan et al., 2022). It is noteworthy that, by 2050, agricultural labor migration will release 5.8 million hectares of rural land, equivalent to 4.1% of China’s total cropland area in 2015 (China Yearbook, 2015; Wang et al., 2021b; China Yearbook, 2022). A related study discovered, in the Yangtze River economic belt, agricultural labor transfer had a positive impact on AEE, and large-scale farming operations played a positive mediating role between agricultural labor transfer and AEE (Zhu et al., 2022a). Existing research recognized the critical role played by agricultural labor transfer in the relationship between urbanization an AEE. However, the mechanism by which urbanization affected AEE through absorbing agricultural labor has not yet been closely examined.
In addition, urbanization affected AEE by changing rural residents’ income. In theory, urbanization increased rural residents’ income through the following channels. First, migration from rural to urban areas was expected to increase rural residents’ income through remittances (McKenzie and Sasin, 2007). Second, job opportunities provided by nonagricultural industries clustered in urban areas increases rural residents’ income (Deichmann et al., 2009). Third, urbanization increased rural residents’ income by enhancing the demand for agricultural products (Cali and Menon, 2013). Finally, the spillover effect of urbanization on rural areas is conducive to improving agricultural labor productivity, thereby increasing the rural residents’ income (Allen, 2009; Satterthwaite et al., 2010; Cuong et al., 2014). However, there was a growing concern that urbanization led to the shrinking of the agricultural sector by taking away the production factors (Lin and Ho, 2003; Gao et al., 2019; Huang et al., 2021), consequently decreasing agricultural income (Arouri et al., 2017). Therefore, urbanization does not necessarily lead to higher rural residents’ income.
The rural residents’ income generates income and substitution effect on AEE (Liu et al., 2020b; Guo et al., 2021). More specifically, higher income enhances rural households’ risk tolerance by alleviating their constraints. Thus, it encouraged farmers enlarge the input of pesticides and fertilizers to gain a more yield (Taylor and Lopez-Feldman, 2010; Wu and Liu, 2017), resulting in more agricultural pollutant discharges (Wang and Zhang, 2018). However, an increase in rural residents’ income promoted the application of agricultural production technology, which benefited agri-environmental protection and resource conservation (Guo et al., 2020; Lu et al., 2021). Adopting the geographical detector model (Wang et al., 2021c) detected that rural residents’ income was an important driving factor of AEE spatial differentiation. Based on the panel Tobit model, Zhang et al. (2022) found that rural residents’ income positively impacted AEE in Hunan, which was consistent with the conclusion obtained in a research on Henan province (Yan et al., 2022). With the increase in rural residents’ disposable income by 100 CNY (China yuan), AEE increased by 9.5% (Liu et al., 2020b). However, Wang and Huang (2022) examined the relationship between rural residents’ income and AEE in China’s major grain-producing areas. They argued that rural residents’ income negatively affected AEE because less of the increased income was invested in improving agricultural production conditions. In summary, the impact of rural residents’ income on AEE was unclear yet. Therefore, investigating the mediating role of rural residents’ income between urbanization and AEE is essential.
3 Methods and data
3.1 Methods
3.1.1 GB-US-SBM model
There were two basic methods widely being adopted to measure AEE, namely, Stochastic Frontier Analysis (SFA) and Data Envelopment Analysis (DEA) (Alene and Zeller, 2005; Wang and Zhang, 2018). The former, a parametric approach that needed to set a production function, was only suitable for solving problems of multi-output and single-output (Deng and Gibson, 2019; Jin et al., 2019). By contrast, the latter was a non-parametric approach, which did not require a preset function of production boundaries (Kuosmanen and Kortelainen, 2005; Suzigan et al., 2020). Moreover, it had the advantage of solving problems of multi-input and multi-output (Vlontzos et al., 2014; Toma et al., 2017; Liu et al., 2020b).
To ensure the accuracy of AEE assessed by DEA, at least the following issues, i.e., the biased efficiency caused by the radial and oriented model, the undesired output and the efficiency ranking of Frontier decision-making units, should be noted (Huang et al., 2014). However, the generally used DEA models, such as the traditional DEA model, SBM (slack-based measure)-Undesirable model, network DEA model and super efficiency SBM model (Liu et al., 2015; Hong et al., 2016; Pang et al., 2016; Jiang et al., 2022), did not ultimately settle the three problems mentioned above.
To adress the literature gap on those issues, Huang et al. (2014) proposed a comprehensive eco-efficiency measure, namely, the GB-US-SBM model, which combined US-SBM (undesirable output, super efficiency and SBM) model and global benchmark technology (GBT). In addition, the GB-US-SBM model effectively solved the infeasible linear solutions due to too many input and output variables. In recent years, the GB-US-SBM model has been applied extensively in assessing agricultural carbon emission efficiency and planting eco-efficiency and other research fields (Caiado et al., 2017; Sueyoshi et al., 2017; Liu et al., 2020a; Liu and Shi, 2020; Wu et al., 2021). Assume that there are
The GB-US-SBM model is represented as follows:
Where
3.1.2 Mediation effect model
The mediation effect depicts an indirect impact of X on Y through M (Judd and Kenny, 1981; Baron and Kenny, 1986). M is the mediator or mediating variable (Tang et al., 2020; Dong et al., 2021; Jiang, 2022b). To better understand the mediating role of agricultural labor and rural residents’ income and their effects, the paper constructed the mediation effect model to examined the influence mechanisms of urbanization on AEE through the two mediator variables. The mediation effect model is shown in Figure 2.
Where
The stepwise regression method was proposed (Baron and Kenny, 1986) to test the mediating effect, and the following conditions were needed: 1) Parameter
Wald test and Wooldridge test were employed to perform heteroscedasticity and autocorrelation tests. F test, LM test, and Hausman test were applied in choosing mixed regression, random effect, and fixed-effect models. According to the previous research (Fuinhas et al., 2021), a conceptual framework related to the methodological approach was shown in Figure 3.
This study mainly used the econometric software MaxDEA to measure AEE and Stata 16.0 to realize the mediation effect model. Indeed, the Stata commands used in this study included sum, xtcsd, xttest0, vif, xttest3, xtserial, hausman, and xtreg. These commands were used to realize the preliminary tests and the model estimations.
3.2 Variable selection and data sources
3.2.1 Variable selection
(1) Dependent variable: agricultural eco-efficiency (AEE). AEE refers to a process that seeks to maximize agricultural economic output while minimizing resource consumption and negative environmental impact (Coluccia et al., 2020; Wang and Huang, 2022; Zhou et al., 2022). Therefore, this study constructed an AEE evaluation indicator system, as shown in Table 1.
As shown in Table 1, this study selected agriculture in the broad sense (including planting, forestry, animal husbandry, and fishery) as the research object, which would yield more realistic and accurate results than choosing agriculture in a narrow sense (Liu et al., 2020b; Yan et al., 2022). Referring to relevant literature (Pan and Ying, 2013; Wang and Zhang, 2018; Lu and Xiong, 2020), the evaluation system of AEE was divided into an input dimension, a desirable output dimension, and an undesirable output dimension. Eight indicators were considered as the inputs, including labor, land, chemical fertilizer, pesticides, agricultural plastic film, diesel oil, agricultural machinery, and irrigation. The agricultural output was selected as the desirable output. The undesirable outputs incorporated agricultural carbon emissions and agricultural pollution. The agricultural carbon emissions included cultivated land utilization, rice production, livestock and poultry breeding. Here is the equation
(2) Core explanatory variable: urbanization rate. The most straightforward expression of urbanization is the agglomeration of the population in urban areas (Zhou et al., 2019; Luo et al., 2021). Therefore, this study selected population urbanization rate as the core explanatory variable. To avoid potential endogeneity problems, this study used the urbanization rate lagged one period after logarithm transformation in the regression (Mao et al., 2015; Gao et al., 2020).
(3) Mediator variables: agricultural labor and rural residents’ income. Agricultural employees and rural residents’ per capita net income were selected as the proxy variables of agricultural labor and rural residents’ income, respectively.
(4) Control variables: Control variables were chosen from the perspective of natural disasters, agricultural production and socioeconomic development (Pan and Ying, 2013; Zhang et al., 2022). Natural disasters can damage the agricultural environment while reducing agricultural output (Wang and Zhang, 2018), which is closely related to AEE (Yang et al., 2023). Therefore, the proportion of the covered area in the sown area of crops (z1) was selected to control the impact of natural disasters on AEE.
Crops have different demands for production factors, especially chemical inputs, resulting in significant differences in agricultural pollutants and carbon emissions (Yan et al., 2022). Therefore, crop planting structure (z2, the logarithm form of the variable) was selected as a control variable. Adequate irrigation can enhance agricultural output and indirectly reduce the use of chemical fertilizers (Zhu et al., 2021). The effective irrigation rate (z3, the logarithm form of the variable) was used as a control variable. Agricultural economic status (z4, the logarithm form of the variable) was the contribution of agriculture to regional economic development, which was closely related to the agricultural ecological environment (Zhang, 2018; Ali et al., 2019).
Some scholars argued that China’s fiscal expenditure on agriculture primarily focused on improving agricultural production infrastructures and subsidizing the input of agricultural production resources, such as fertilizers, diesel oil, seeds and agricultural machinery, which eventually led to AEE loss (Hong et al., 2016; Wang and Zhang, 2018; Wang et al., 2021a; Guo et al., 2021; Jiang, 2022a). However, some scholars argued that financial support for agriculture stimulated rural residents’ enthusiasm for agriculture production and promoted the application of green production technology, which was helpful in improving AEE (Chen et al., 2020; Zhu et al., 2022a). Thus, this paper selected financial support for agriculture (z5, the logarithm form of the variable) as a control variable. In general, if the rural residents had a higher education, they would have a more robust initiative to use new technologies or accept pollution reduction policies (Bayyurt and Yılmaz, 2012; Chen and Zhang, 2019; Liu et al., 2020b). Thus, the education level of rural residents (z6, the logarithm form of the variable) was selected as a control variable. In addition, the urban-rural income gap (z7, the logarithm form of the variable) was taken as a control variable.
3.2.2 Data sources
China’s 31 provinces, autonomous regions and municipalities in the mainland were included in this study (Hong Kong, Taiwan and Macao were not involved due to the data availability). Then, this study divided the 31 provinces into optimized, moderateand protected development regions.
Since 2004, China has continuously released Central Document No.1 with agriculture, rural areas, and farmers as its themes. In 2004, China’s agricultural policies began to change, and the government introduced a series of policies to support agricultural development. Meanwhile, China’s urbanization also entered a rapid stage. Therefore, selecting data after 2004 to examine the relationship between urbanization and AEE was meaningful. Given the availability of data, the panel data from 2004 to 2020 of China’s 31 provinces were obtained from China Statistical Yearbook and China Rural Statistical Yearbook. All the price-related variables were deflated.
4 Results
4.1 Spatial distribution pattern of AEE in China
Considering the panel data of 31 provinces in China, the GB-US-SBM was used to measure the AEE. To better discover the spatial evolution of AEE, the scores of AEE were subdivided into four levels referring to the previous research (Liu and Shi, 2020). The four levels were as follows: the score of AEE between 0 and 0.3 was called low level, the score of AEE between 0.3 and 0.6 was called medium level, the score of AEE between 0.6 and 0.9 was called medium-high level, and the score of AEE higher than 0.9 was called high level. The AEE maps in 2004, 2010, 2015, and 2020 were reported in Figure 4.
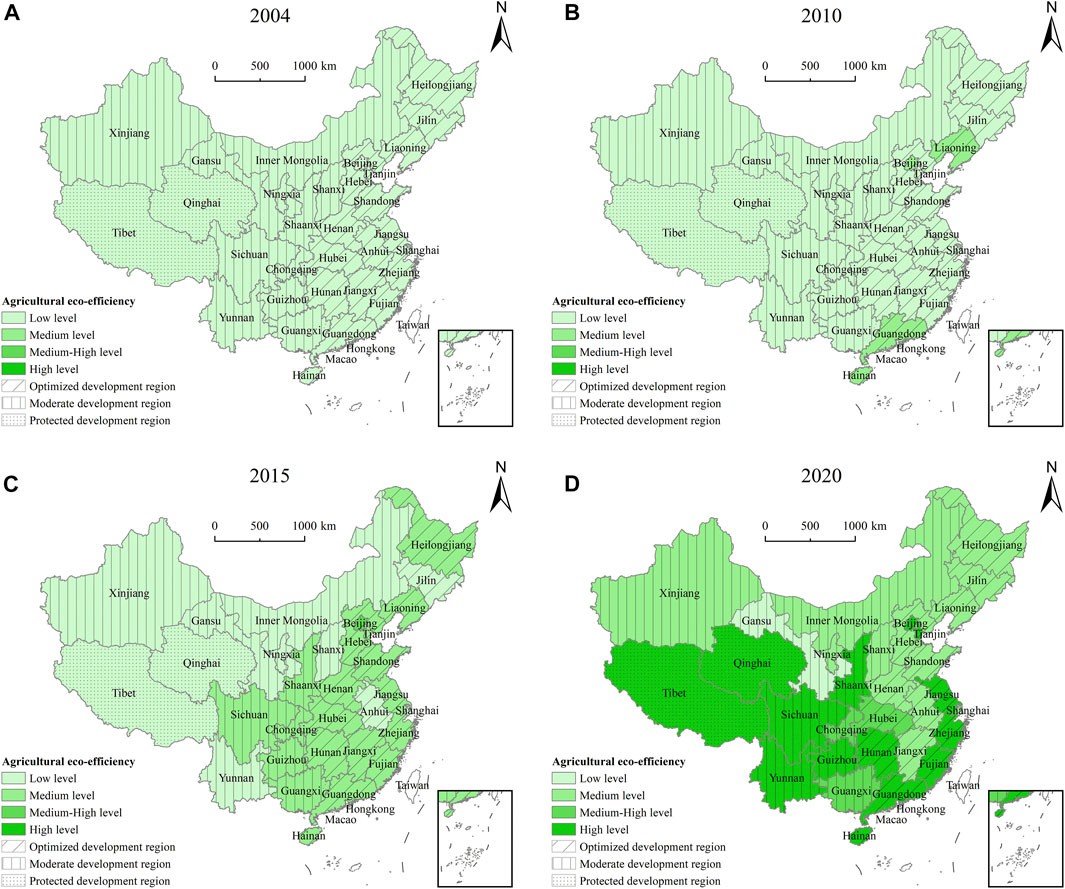
FIGURE 4. Spatial distribution pattern of AEE in 2004, 2010, 2015, and 2020. (A) represents the AEE in 2004, (B) represents the AEE in 2010, (C) represents the AEE in 2015, and (D) represents the AEE in 2020.
Figure 4 illustrated that the 31 provinces’ AEE was at a low level in 2004. In 2010, the provinces with a medium level of AEE were Liaoning, Guangdong, Beijing and Hainan, and the others were at a low level. Between 2004 and 2010, the AEE increased slowly and had no noticeable regional differences.
Then, China listed the transformation of agriculture development mode as the priority of agricultural and rural work in 2010. What followed was the ecological development of agriculture and the control of agricultural non-point source pollution, which was mentioned in Central Document No.1 of 2012. In 2014, China focused on agricultural ecological protection and proposed to develop Two-oriented Agriculture. Under the implementation of the agricultural pollution control policies, the AEE in more than two-thirds of the provinces was above the medium level at the end of the 12th Five-Year Plan (2015).
Then, a series of action plans, such as the Zero Growth Action Plan in applying chemical fertilizers and pesticides, and the Agricultural and Rural Pollution Control Action Plan, were implemented in 2015 and 2018. Consequently, the AEE experienced remarkable improvement in the 13th Five-Year Plan period, consistent with a previous study’ conclusion (Liu et al., 2020b). In 2020, the AEE in 14 provinces was higher than 1. Moreover, the spatial differences of AEE were more prominent.
As illustrated in Figure 4, the AEE exhibited a descending order in different agriculture development regions: optimized development region > moderate development region > protected development region. The optimized development region was the major producing area of bulk agricultural commodities. In the optimized development region, the AEE in Southern China and the Middle-Lower Yangtze were higher than that in Northeast China. The moderate development region, where resources and environment-carrying capacity were limited, had an AEE lower than the national average. Notably, the AEE in the Southwest was highest in the moderate development region, consistent with a previous study using the agriculture sustainable development index (Wang and Yu, 2021). The protected development region included Qinghai and Tibet. During 2015–2020, the AEE in this region increased rapidly. By 2020, the AEE of Qinghai and Tibet hit 1.0869 and 1.0256, respectively.
4.2 The scatterplot between urbanization and AEE
Figure 5 demonstrated the scatterplot between urbanization and AEE. The abbreviations of 31 provinces were included in Table A1. From 2004 to 2020, the average value of the urbanization rate increased from 43.4968% to 63.7245%. Meanwhile, the average AEE rose from 0.1386 to 0.7443. In 2020, 17 provinces failed to reach the production Frontier, implying ample room for improvement of the AEE. This finding was consistent with the existing literature (Liu et al., 2020b; Akbar et al., 2021; Wang et al., 2021c). As depicted in Figure 5, an increasing number of provinces were moving towards the first quadrant, where the urbanization rate and AEE were above average. However, it was uneasy to determine the role agricultural labor and rural residents’ income played in the relationship between urbanization and AEE. Consequently, this paper analyzed the impact of urbanization on AEE and the mediated role of agricultural labor and rural residents’ income in different agriculture development regions.
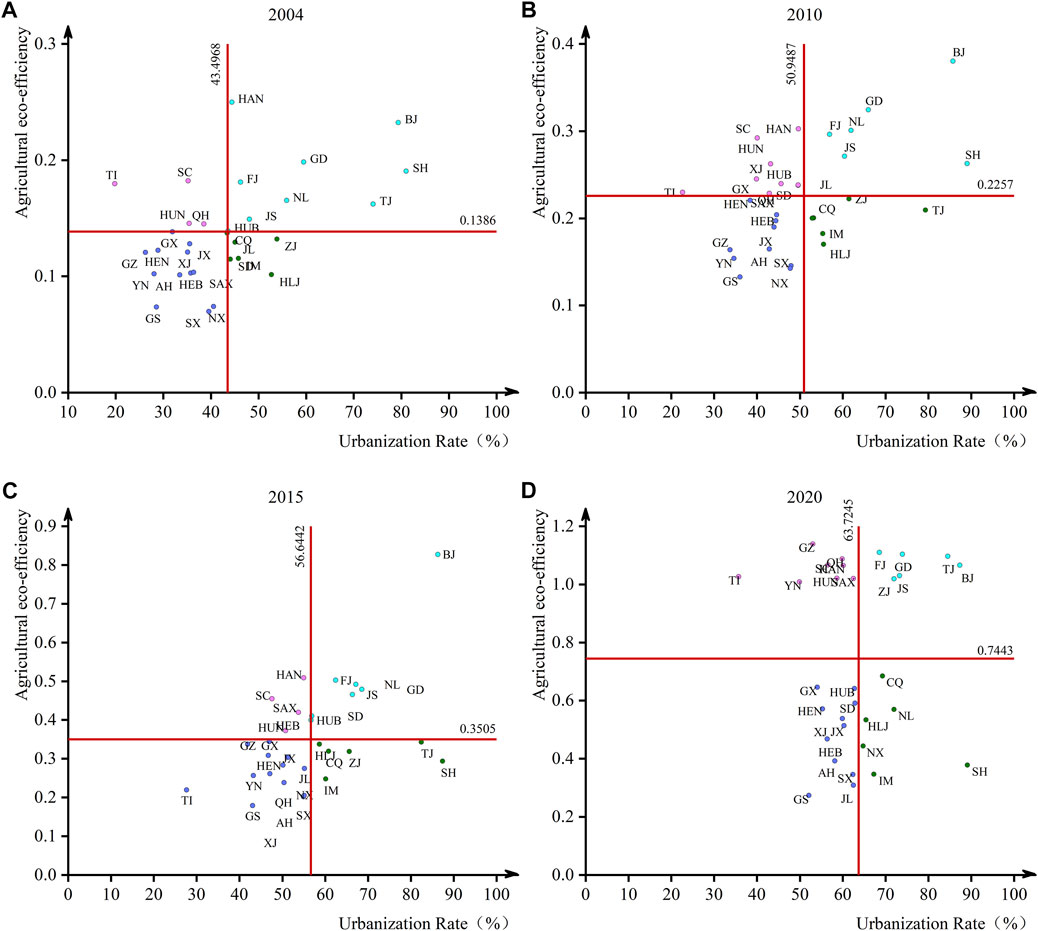
FIGURE 5. Scatterplot between urbanization and AEE. (A) denotes the scatterplot between urbanization and AEE in 2004, (B) denotes the scatterplot between urbanization and AEE in 2010, (C) denotes the scatterplot between urbanization and AEE in 2015, (D) denotes the scatterplot between urbanization and AEE in 2020.
4.3 The influence of urbanization on AEE and the mediation effect
The results of the mediation effect model in three agriculture development regions were reported in Table 2, Table 3 and Table 4, respectively.
Table 2 indicated that urbanization had a positive and significant impact on AEE in the optimized development region, with a total effect of 0.4478. The coefficients of urbanization on agricultural labor and rural residents’ income were −0.3212 and 0.3123, respectively. In the direct effect model, urbanization, agricultural labor and rural residents’ income coefficients were 0.2228, −0.3273, and 0.3138, respectively. And 0.2228 was smaller than 0.4478, which satisfied the principle of the mediation effect model. Consequently, in the optimized development region, the agricultural labor and rural residents’ income both mediated the impact of urbanization on AEE, and the mediating effects were (−0.3212) × (−0.3273) = 0.1051 and 0.3123 × 0.3138 = 0.0980, respectively.
As shown in Table 3, the total effect of urbanization on AEE in the moderate development region was 1.0450. The coefficients of urbanization on the two mediator variables were −0.7295 and 1.9669, respectively. In the direct effect model, the coefficients of urbanization, agricultural labor and rural residents’ income were 0.6895, −0.4269, and 0.1001, respectively. And 0.6895 was smaller than 1.0450, which satisfied the principle of the mediation effect model. Consequently, in the moderate development region, the agricultural labor and rural residents’ income both mediated the influence of urbanization on AEE, and the mediating effects were (−0.7295) × (−0.4269) = 0.3114 and 1.9669 × 0.1001 = 0.1969, respectively.
As illustrated in Table 4, the total effect of urbanization on AEE was 5.3110 in the protected development region. The mediating effect of agricultural labor was as follows: (−0.8466) × (−2.1068) = 1.7836. The coefficient of urbanization on the rural residents’ income was negative but failed the significance test. Also, the impact of a rural residents’ income on AEE was negative but failed the significance test. Thus, rural residents’ income didnot mediate the impact of urbanization on AEE.
Overall, urbanization positively affected AEE in China, consistent with previous research (Zhang et al., 2022). Expressly, the total effect of urbanization on AEE declined in the following order: protected development region > moderate development region > optimized development region.
The agricultural labor and rural residents’ income mediated the effect of urbanization on AEE. The decrease in agricultural labor caused by urbanization promoted the improvement of AEE. The possible reason was that the agricultural production inputs and the large-scale farming operations were improved due to the transfer of agricultural labor induced by rapid urbanization. The mediating effect of agricultural labor showed a spatial pattern of decreasing gradually from the protected development region to the moderate development region and then the optimized development region.
Rural residents’ income significantly and positively mediated the relationship between urbanization and AEE in both optimized and moderate development regions. However, the rural residents’ income did not mediate the impact of urbanization on AEE in the protected development region. The urbanization process in Qinghai and Tibet lagged behind other regions (Qi, 2019). A previous study found that urbanization decreased the rural residents’ income in Qinghai and Tibet. They argued that the deterioration of agricultural production conditions and few opportunities to increase nonagricultural income hindered rural residents’ income growth (Jiu, 2013). Furthermore, it was found that the impact of rural residents’ income on AEE was negative in Qinghai and Tibet (Wang and Lin, 2021).
4.4 Analysis of other influencing factors
The ratio of the covered area to the sown area of crops negatively impacted AEE. Still, it did not pass the significance test, which was consistent with the conclusion of previous studies (Zhang, 2018; Zhang et al., 2022). Crop planting structure significantly improved AEE in the moderate development region but failed to pass the significance test in the other two regions. The effective irrigation rate positively affected AEE in the optimized development region but failed the significance test in the other two regions. Agricultural economic status was positively related to AEE in the optimized and moderate development region. Previous studies found regional differences in the effect of financial support for agriculture on AEE (Wang and Lin, 2021; Liu et al., 2022). This paper further confirmed the regional differences: financial support for agriculture significantly inhibited the improvement of AEE in the optimized development region. By contrast, it promoted the improvement of AEE in the protected development region. However, it did not significantly affect AEE in the moderate development region. Rural residents’ education level positively influenced the AEE in the moderate development region but failed the significance test in the other two regions. The urban-rural income gap negatively affected the AEE in the moderate development region, and positively impacted the AEE in the optimized development region, but had no significant impact on AEE in the protected development region.
5 Discussion
5.1 Relationship between urbanization and AEE
This paper mainly explored how urbanization, the core driving force, affected AEE through the mediator variables of agricultural labor and rural residents’ income in three agriculture development regions. Extensive research was carried out to analyze the relationship between urbanization and AEE (Zhang, 2018; Shang et al., 2020; Liu et al., 2021; Chen et al., 2022). They used the panel-Tobit model to examine the effect of urbanization on AEE, because the AEE obtained by the SBM model was truncated. In contrast, there were some innovations made in this study.
Firstly, the AEE evaluation index combined carbon emissions and environmental pollution, by which the results would be more reliable and realistic than previous literature from a low-carbon and pollution perspective (Zhang, 2018; Liu et al., 2020b), respectively. Secondly, the AEE obtained using the GB-US-SBM model was not truncated. Therefore, it was applicable to more regression models. Thirdly, the mediation effect model was used to explore the mediation effect of the agricultural labor and rural residents’ income in three agriculture development regions. Through these innovative explorations, it would gain more insights into the relationship between urbanization and AEE.
5.2 Limitations and future recommendations
This study proposed some limitations and corresponding recommendations for future research directions. In this paper, AEE in 2021 and 2022 was not included due to the availability of data, which resulted in the inability of this study to capture the recent developments and changes in AEE.
This study meticulously combed relevant literature and then strictly screened AEE evaluation indicators, there may still be some indicators that have not been incorporated. Hence, in future, indicators should be further screened according to the actual situation of the study area.
Although a full discussion of the relationship between China’ urbanization and AEE was made in this study. But we must acknowledge that considering the differences in economic development stages and agricultural production conditions in different countries and regions, the findings may not directly apply to other countries or regions with different contexts and characteristics.
Possible areas of future research would be to investigate the long-term sustainability of AEE, the inequality, evolutionary trends, and driving forces of inequality in China’s AEE. By this way, it can be helpful for formulating differentiated strategies for improving AEE in China.
6 Robustness tests
6.1 Sub-samples regression
To verify the reliability of the mediation effects of agricultural labor and rural residents’ income, in the relationship between urbanization and AEE, the samples were divided into two sub-samples according to the median of urbanization rate of 31 provinces during 2004–2020. The two sub-samples were areas with high urbanization rate and areas with low urbanization rate. The estimation results were presented in Table 5 and Table 6.
As shown in Table 5, the total effect of urbanization on AEE in areas with a high urbanization rate was 0.8389. The coefficients of urbanization on the two mediator variables were −0.0315 and 0.6164, respectively. In the direct effect model, urbanization, agricultural labor and rural residents’ income coefficientswere 0.2812, −0.6449 and 1.0030, respectively. And 0.2812 was smaller than 0.8389, which satisfied the principle of the mediation effect model. Consequently, in the areas with a high urbanization rate, the agricultural labor and rural residents’ income both mediated the influence of urbanization on AEE, and the mediating effects were
As illustrated in Table 6, the total effect of urbanization on AEE in areas with a low urbanization rate was 1.3620. The coefficients of urbanization on the two mediator variables were −0.8869 and 1.4875, respectively. In the direct effect model, the coefficients of urbanization, agricultural labor and rural residents’ income were 0.8622, −0.4312, and 0.1096, respectively. And 0.8622 was smaller than 1.3620, which satisfied the principle of the mediation effect model. Consequently, in the areas with low urbanization rate, the agricultural labor and rural residents’ income both mediated the influence of urbanization on AEE, and the mediating effects were
6.2 Replace the core explanatory variable
Further, this paper replaced the core explanatory variable to check the robustness. In-situ urbanization is an essential mode of urbanization in China, which is helpful to enrich the connotation of urbanization (Chen and Tan, 2015; Pang and Ran, 2017; Zhao and Xu, 2021). Thus, the in-situ urbanization level (lnurban1), namely, the proportion of employment in rural private enterprises and individual rural employment in rural populations, was used as the alternative variable of the urbanization rate. Due to the lack of data, Beijing and Shanghai were excluded from the analysis. The remaining 29 provinces were divided into areas with high in-situ urbanization level and areas with low in-situ urbanization level. The results were shown in Table 7 and Table 8.
The estimated results of urbanization’ effects on AEE in areas with low in-situ urbanization level were shown in Table 8.
As shown in Table 7 and Table 8, in-situ urbanization contributed to the decrease of agricultural labor, and then the reduction of agricultural labor had a positive effect on AEE. At the same time, in-situ urbanization also promoted the increase of rural residents’ income, and the increase had a significant positive impact on AEE. After replacing the core explanatory variables, the roles of the two mediator variables (agricultural labor force and rural residents’ income) in the influence mechanism of urbanization on AEE remained the same as above, which further verified the robustness of the results.
7 Conclusion and policy implications
7.1 Conclusion
To explore and promote sustainable agriculture development through high-quality urbanization, accurately analyzing the relationship between urbanization and AEE is a fundamental work. Based on the provincial panel data during 2004–2020, this study extended the existing literature by adopting the GB-US-SBM model to accurately measure the AEE of 31 provinces in mainland China. Compared with the previous researches, a mediation effect model was constructed for the first time to investigate the influence mechanism of urbanization on AEE through the two mediator variables, agricultural labor and rural residents’ income. The main conclusions were as follows:
1) China’s AEE rose steadily, with the mean value increasing from 0.138 to 0.744. However, there was still ample room for improvement, especially in some provinces with a lower AEE. The spatial pattern of AEE showed a cascade decline in the following order:optimized development region > moderate development region > protected development region. 2) Urbanization positively improved the AEE, and its total effect decreased as follows: protected development region > moderate development region > optimized development region. 3) Agricultural labor and rural residents’ income played dual mediating effects, the former played a stronger mediating role. Through the absorption of agricultural labor, urbanization promoted the improvement of AEE. Moreover, agricultural labor played a decreasing mediating role from the protected development region to the moderate development region and the optimized development region. Rural residents’ income positively mediated the impact of urbanization on AEE in the optimized and moderate development region. However, rural residents’ income did not mediate the effect of urbanization on AEE in the protected development region.
7.2 Policy implications
In promoting urbanization, China has recognized the importance of improving AEE, and has introduced some relevant policies. To further enhance the effectiveness of the policies, precision and refinement can be regarded as essential directions, avoiding the “one size fits all” simplification approach as much as possible. Therefore, based on the conclusions, this study proposed the following policy suggestions.
1) To improve AEE, it is urgent to formulate policies tailored to regional conditions. In the optimized development region, 10 provinces did not reach the optimal production Frontier. Among them, Hubei, Henan, Jiangxi and Anhui had higher agricultural carbon emissions. Thus, reducing agricultural resource consumption should be centred in the four provinces. In addition, Shandong faced the most severe agricultural pollution in the region. Hence, reducing the agricultural pollutant discharges should be further reinforced in Shandong.
In the moderate development region, Gansu had the lowest AEE. Agricultural carbon emissions and pollution caused by pesticides and agricultural plastic film were the primary sources of the AEE loss in Gansu. Thus, realizing the recycling of agricultural plastic film should be accelerated.
The protected development region has a crucial strategic position in ecological protection and construction. Comfortingly, both Qinghai and Tibet reached the optimal production Frontier. Ecological agriculture and plateau-characterized agriculture should be moderately developed.
2) Urbanization should be accelerated to provide more nonagricultural job opportunities. Specifically, in the process of urbanization development, it is necessary to ensure public services and infrastructure supply in urban areas, attracting more rural labor transferred from rural areas to urban areas. And amounts of agricultural land resources will be released when rural labor is continuously transferred to urban areas.
In response, it is necessary to guide the orderly transfer of agricultural land resources (by coordinating the income distribution between contractors and operators, accelerating the transfer of rural land contractual rights), which can promote large-scale farming operations and thus improve the AEE. The comprehensive quality of agricultural labor will be more important with decreased agricultural labor. Therefore, to improve the overall quality of agricultural labor, the local government should set up a special fund and establish a modern vocational education system to encourage young and middle-aged returnees to receive agricultural education and training.
In addition, tax incentives and subsidies for production factors should be increased for agricultural labor, who use new low-energy agricultural machinery, organic fertilizers, degradable agricultural films, water-saving irrigation equipment and other low-carbon agricultural production factors to reduce agricultural production carbon emissions and other non-point source pollutants.
3) Based on inter-regional differences, targeted measures to improve AEE should be implemented. Expanding the channels by which urbanization increases rural residents’ income should be urgent in optimized and moderate development regions. Furthermore, it is necessary to give full play to new industries and business forms (leisure agriculture, rural tourism, rural e-commerce) to increase rural residents’ income. According to the conditions in the protected development region, it is urgent to increase the nonagricultural income by enhancing rural residents’ labor and vocational skills. Meanwhile, it is urgent to increase agricultural income by improving the agricultural production infrastructures and fully developing plateau-characterized agriculture.
Data availability statement
The original contributions presented in the study are included in the article/Supplementary Material, further inquiries can be directed to the corresponding author.
Author contributions
XjZ: conceptualization, methodology, writing—review and editing. JY: conceptualization, data curation. HC: software, formal analysis, visualization. XyZ: investigation, supervision, formal analysis. YX: resources, validation. All authors contributed to the article and approved the submitted version.
Funding
This research was funded by the Project of Humanities and Social Science of Henan Province (grant number: 2022-ZZJH-037); Soft Science Research Planning Project of Henan Province (grant number: 232400410114).
Conflict of interest
The authors declare that the research was conducted in the absence of any commercial or financial relationships that could be construed as a potential conflict of interest.
Publisher’s note
All claims expressed in this article are solely those of the authors and do not necessarily represent those of their affiliated organizations, or those of the publisher, the editors and the reviewers. Any product that may be evaluated in this article, or claim that may be made by its manufacturer, is not guaranteed or endorsed by the publisher.
References
Akbar, U., Li, Q.-L., Akmal, M. A., Shakib, M., and Iqbal, W. (2021). Nexus between agro-ecological efficiency and carbon emission transfer: Evidence from China. Environ. Sci. Pollut. Res. 28 (15), 18995–19007. doi:10.1007/s11356-020-09614-2
Alene, A. D., and Zeller, M. (2005). Technology adoption and farmer efficiency in multiple crops production in eastern Ethiopia: A comparison of parametric and non-parametric distance functions. Agric. Econ. Rev. 6 (389-2016-23429), 5–17. doi:10.22004/ag.econ.44089
Ali, S., Gucheng, L., Ying, L., Ishaq, M., and Shah, T. (2019). The relationship between carbon dioxide emissions, economic growth and agricultural production in Pakistan: An autoregressive distributed lag analysis. Energies 12 (24), 4644. doi:10.3390/en12244644
Allen, R. (2009). The British industrial revolution in global perspective. United States: Cambridge University Press.
Antrop, M. (2004). Landscape change and the urbanization process in Europe. Landsc. Urban Plan. 67 (1), 9–26. doi:10.1016/s0169-2046(03)00026-4
Arouri, M., Youssef, A. B., and Nguyen, C. (2017). Does urbanization reduce rural poverty? Evidence from vietnam. Econ. Model. 60, 253–270. doi:10.1016/j.econmod.2016.09.022
Baron, R. M., and Kenny, D. A. (1986). The moderator–mediator variable distinction in social psychological research: Conceptual, strategic, and statistical considerations. J. personality Soc. Psychol. 51 (6), 1173–1182. doi:10.1037/0022-3514.51.6.1173
Bayyurt, N., and Yılmaz, S. (2012). The impacts of governance and education on agricultural efficiency: An international analysis. Procedia-Social Behav. Sci. 58, 1158–1165. doi:10.1016/j.sbspro.2012.09.1097
Bren d’Amour, C., Reitsma, F., Baiocchi, G., Barthel, S., Güneralp, B., Erb, K.-H., et al. (2017). Future urban land expansion and implications for global croplands. Proc. Natl. Acad. Sci. 114 (34), 8939–8944. doi:10.1073/pnas.1606036114
Cai, J., Li, X., Liu, L., Chen, Y., Wang, X., and Lu, S. (2021). Coupling and coordinated development of new urbanization and agro-ecological environment in China. Sci. Total Environ. 776, 145837. doi:10.1016/j.scitotenv.2021.145837
Caiado, R. G. G., de Freitas Dias, R., Mattos, L. V., Quelhas, O. L. G., and Leal Filho, W. (2017). Towards sustainable development through the perspective of eco-efficiency-A systematic literature review. J. Clean. Prod. 165, 890–904. doi:10.1016/j.jclepro.2017.07.166
Cali, M., and Menon, C. (2013). Does urbanization affect rural poverty? Evidence from Indian districts. World Bank Econ. Rev. 27 (2), 171–201. doi:10.1093/wber/lhs019
Camarero, M., Castillo, J., Picazo-Tadeo, A. J., and Tamarit, C. (2013). Eco-efficiency and convergence in OECD countries. Environ. Resour. Econ. 55 (1), 87–106. doi:10.1007/s10640-012-9616-9
Cao, B., Tang, L., Hu, B., and Zhao, X. (2021). Global grain crisis and China's grain security. Int. Econ. Rev. 2, 9–21.
Chen, F., Qiao, G., Wang, N., and Zhang, D. (2022). Study on the influence of population urbanization on agricultural eco-efficiency and on agricultural eco-efficiency remeasuring in China. Sustainability 14 (20), 12996. doi:10.3390/su142012996
Chen, H., and Zhang, X. (2019). Study on the path of agricultural ecological construction improving agricultural production efficiency. Ekoloji Derg. 107, 1–10.
Chen, J., Xin, M., Ma, X., Chang, B., and Zhang, Z. (2020). Chinese agricultural eco-efficiency measurement and driving factors. China Environ. Sci. 40 (7), 3216–3227. doi:10.19674/j.cnki.issn1000-6923.2020.0360
Chen, X., and Tan, Y. (2015). Empirical research on the influence of situ urbanization on regional consumption market. Econ. Geogr. 35 (3), 80–86. doi:10.15957/j.cnki.jjdl.2015.03.012
China Yearbook (2015). National agricultural sustainable development plan 2015-2030. United States: Food and Agriculture.
Coluccia, B., Valente, D., Fusco, G., De Leo, F., and Porrini, D. (2020). Assessing agricultural eco-efficiency in Italian Regions. Ecol. Indic. 116, 106483. doi:10.1016/j.ecolind.2020.106483
Cuong, N. V. (2014). Does urbanization help poverty reduction in rural areas? Evidence from vietnam. Work. Pap. 2014, 1.
Deichmann, U., Shilpi, F., and Vakis, R. (2009). Urban proximity, agricultural potential and rural non-farm employment: Evidence from Bangladesh. World Dev. 37 (3), 645–660. doi:10.1016/j.worlddev.2008.08.008
Deng, X., and Gibson, J. (2019). Improving eco-efficiency for the sustainable agricultural production: A case study in Shandong, China. Technol. Forecast. Soc. Change 144, 394–400. doi:10.1016/j.techfore.2018.01.027
Dong, H., Xue, M., Xiao, Y., and Liu, Y. (2021). Do carbon emissions impact the health of residents? Considering China's industrialization and urbanization. Sci. Total Environ. 758, 143688. doi:10.1016/j.scitotenv.2020.143688
Du, Z., Chen, W., Lu, F., Liao, H., and Wang, W. (2022). Comprehensively promoting rural revitalization: Interpretation of No 1 central document. J. Soc. Sci. Hunan Normal Univ. 51 (3), 10–26.
Fei, R., and Lin, B. (2017). The integrated efficiency of inputs–outputs and energy–CO2 emissions performance of China's agricultural sector. Renew. Sustain. Energy Rev. 75, 668–676. doi:10.1016/j.rser.2016.11.040
Fuinhas, J. A., Koengkan, M., Leitão, N. C., Nwani, C., Uzuner, G., Dehdar, F., et al. (2021). Effect of battery electric vehicles on greenhouse gas emissions in 29 European union countries. Sustainability 13 (24), 13611. doi:10.3390/su132413611
Gao, P., and Wang, X. (2018). Fiscal input, environmental regulation and efficiency of green technological innovation: Based on the data of large industrial enterprises from 2008 to 2015. Ecol. Econ. 34, 93–99.
Gao, Y., Wang, Z., Guo, C., Hou, Z. Q., and Huang, K. J. (2019). Cycling profile of layered MgAl2O4/reduced graphene oxide composite for asymmetrical supercapacitor. Rural. Econ. 10, 38–44. doi:10.1016/j.jcis.2018.12.045
Gao, Y., Zhang, Z., and Wang, Z. (2020). Does urbanization improve agricultural mechanization: Panel evidence from 31 provinces in China. Econ. Surv. 37 (3), 37–44. doi:10.15931/j.cnki.1006-1096.20200324.001
Gava, O., Bartolini, F., Venturi, F., Brunori, G., and Pardossi, A. (2020). Improving policy evidence base for agricultural sustainability and food security: A content analysis of life cycle assessment research. Sustainability 12 (3), 1033. doi:10.3390/su12031033
Gras, C., and Cáceres, D. M. (2020). Technology, nature’s appropriation and capital accumulation in modern agriculture. Curr. Opin. Environ. Sustain. 45, 1–9. doi:10.1016/j.cosust.2020.04.001
Guan, X., Wei, H., Lu, S., Dai, Q., and Su, H. (2018). Assessment on the urbanization strategy in China: Achievements, challenges and reflections. Habitat Int. 71, 97–109. doi:10.1016/j.habitatint.2017.11.009
Guo, Q., Li, S., and Nan, L. (2020). Farming households’ pro-environmental behaviors from the perspective of environmental literacy. Resour. Sci. 42, 856–869. doi:10.18402/resci.2020.05.05
Guo, Y., Tong, L., Mei, L., and Pan, Z. (2021). Evaluation and influencing factors of agricultural green efficiency in jianghuai ecological economic zone. Sustainability 14, 30. doi:10.3390/su14010030
Han, H., Ding, T., Nie, L., and Hao, Z. (2020). Agricultural eco-efficiency loss under technology heterogeneity given regional differences in China. J. Clean. Prod. 250, 119511. doi:10.1016/j.jclepro.2019.119511
Harris, J. R., and Todaro, M. P. (1970). Migration, unemployment and development: A two-sector analysis. Am. Econ. Rev. 60 (1), 126–142.
Hong, K., Chen, C., Chao, F., and Huang, J. (2016). The spatial temporal differences of agricultural eco-efficiency and its influential factors. J. South China Agric. Univ. Soc. Sci. Ed. 15 (2), 31–41. doi:10.7671/j.issn.1672-0202.2016.02.004
Hong, M., and Zheng, L. (2020). Regional differences and spatiotemporal and characteristics of agricultural eco-efficiency in China. Statistics Decis. 548, 56–61. doi:10.13546/j.cnki.tjyjc.2020.08.011
Hou, M., and Yao, S. (2018b). Spatial spillover effects and threshold characteristics of rural labor transfer on agricultural eco-efficiency in China. Resour. Sci. 40 (12), 2475–2486. doi:10.18402/resci.2018.12.14
Hou, M., and Yao, S. (2018a). Spatial-temporal evolution and trend prediction of agricultural eco-efficiency in China: 1978-2016. Acta Geogr. Sin. 73 (11), 2168–2183. doi:10.11821/dlxb201811009
Hou, X., Liu, J., Zhang, D., Zhao, M., and Xia, C. (2019). Impact of urbanization on the eco-efficiency of cultivated land utilization: A case study on the Yangtze River economic belt, China. J. Clean. Prod. 238, 117916. doi:10.1016/j.jclepro.2019.117916
Huang, J., Hu, R., Yi, H., Sheng, Y., Wang, J., Bao, M., et al. (2022). Development visions and policies of China's agriculture by 2050. Strategic Study CAE 24 (1), 011–019. doi:10.15302/j-sscae-2022.01.002
Huang, J., Yang, X., Cheng, G., and Wang, S. (2014). A comprehensive eco-efficiency model and dynamics of regional eco-efficiency in China. J. Clean. Prod. 67, 228–238. doi:10.1016/j.jclepro.2013.12.003
Huang, X., Wang, Z., and Ren, Z. (2021). Research on the influence of urbanization structure on farmers’ income increase—based on the perspective of income structure. Open J. Soc. Sci. 9 (5), 540–552. doi:10.4236/jss.2021.95029
Jayadevan, C. (2020). Impact of urbanization and industrialization on agriculture. Eur. J. Agric. Food Sci. 2 (4), 79. doi:10.24018/ejfood.2020.2.4.79
Jiang, G. (2022a). How does agro-tourism integration influence the rebound effect of China’s agricultural eco-efficiency? An economic development perspective. Front. Environ. Sci. 689, 921103. doi:10.3389/fenvs.2022.921103
Jiang, G. (2022b). Mediating effects and moderating effects in causal inference. China Ind. Econ. 5, 100–120. doi:10.19581/j.cnki.ciejournal.2022.05.005
Jiang, L., and Li, Z. (2016). Urbanization and the change of fertilizer use intensity for agricultural production in Henan Province. Sustainability 8 (2), 186. doi:10.3390/su8020186
Jiang, Z., Liu, Y., Zeng, Z., and Qin, G. (2022). The impact of public environmental expenditure on agricultural eco-efficiency: Empirical evidence from the Yangtze River economic belt. Econ. Problems 6, 113–122. doi:10.16011/j.cnki.jjwt.2022.06.001
Jin, G., Li, Z., Deng, X., Yang, J., Chen, D., and Li, W. (2019). An analysis of spatiotemporal patterns in Chinese agricultural productivity between 2004 and 2014. Ecol. Indic. 105, 591–600. doi:10.1016/j.ecolind.2018.05.073
Jiu, M. (2013). Survey analysis on the satisfactory level and changes in family income and expenditure of farmers and nomads in both Tibet and Qinghai autonomous regions under the process of urbanization. J. Tibet Univ. 28 (4), 26–32. doi:10.16249/j.cnki.1005-5738.2013.04.027
Judd, C. M., and Kenny, D. (1981). Process analysis estimating mediation in treatment evaluations. Eval. Rev. 5 (5), 602–619. doi:10.1177/0193841x8100500502
Karimi Alavijeh, N., Salehnia, N., Salehnia, N., and Koengkan, M. (2022). The effects of agricultural development on CO2 emissions: Empirical evidence from the most populous developing countries. Environ. Dev. Sustain. 2022, 1–21. doi:10.1007/s10668-022-02567-1
Kong, X. (2019). Estimate the agricultural labor quantity and agricultural productivity in China. Reform 5, 38–47.
Kuosmanen, T., and Kortelainen, M. (2005). Measuring eco-efficiency of production with data envelopment analysis. J. Industrial Ecol. 9 (4), 59–72. doi:10.1162/108819805775247846
Li, B., Zhang, J., and Li, H. (2011). Research on spatial-temporal characteristics and affecting factors decomposition of agricultural carbon emission in China. China Popul. Resour. Environ. 21 (8), 80–86. doi:10.3969/j.issn.1002-2104.2011.08.013
Li, J., and Li, Y. (2019). Influence measurement of rapid urbanization on agricultural production factors based on provincial panel data. Socio-Economic Plan. Sci. 67, 69–77. doi:10.1016/j.seps.2018.09.004
Li, M., Zhou, X., Zhou, Y., and Wang, J. (2022). Research on coupling and coordination of agricultural eco-efficiency and new urbanization in main grain production areas. Ecol. Econ. 5, 1–10.
Lin, G. C., and Ho, S. P. (2003). China's land resources and land-use change: Insights from the 1996 land survey. Land Use Policy 20 (2), 87–107. doi:10.1016/s0264-8377(03)00007-3
Liu, F., Zhong, P., and Zhang, W. (2015). Comprehensive evaluation on ecoligical efficiency of mountainous counties in China. J. Northwest A&f Univ. Sci. Ed. 15 (3), 94–99. doi:10.13968/j.cnki.1009-9107.2015.03.014
Liu, H., and Shi, Y. (2020). Spatial differentiation and promotion potential of agricultural eco-efficiency in China. J. Guangdong Univ. Finance 6, 51–64.
Liu, L., Yuchi, X., and Wang, G. (2020a). “Analysis of research progress on ecological efficiency based on histcite and web of science,” in Paper presented at The 3rd International Conference on Economy, Management and Entrepreneurship (ICOEME 2020), 08 September 2020 (Netherlands: Atlantis Press).
Liu, L., Zou, L., and Wang, Y. (2020b). Spatial-temporal characteristics and influencing factors of agricultural eco-efficiency in China in recent 40 years. Land Use Policy 97, 104794. doi:10.1016/j.landusepol.2020.104794
Liu, P., Huang, J., Li, X., Wang, C., Yan, S., Cain, A. K., et al. (2022). Length-based separation of Bacillus subtilis bacterial populations by viscoelastic microfluidics. J. Hebei Agric. Univ. Soc. Sci. 24 (1), 7–17. doi:10.1038/s41378-021-00333-3
Liu, P., Zhang, W., Zhou, Y., and Li, X. (2021). Couplig coordination between urbanization and agricultural ecological efficiency in East China. Jounal Hebei Agric. Univ. Soc. Sci. 23 (6), 25–31. doi:10.13320/j.cnki.jauhe.2021.0085
Lu, Q., Zhang, X., and Meng, X. (2021). Empirical analysis of the influence spatial correlation and influencing factors of ecological efficiency of grain planting in China. Econ. Problems 8, 82–94. doi:10.16011/j.cnki.jjwt.2021.08.010
Lu, S., and Xiong, J. (2020). Can the establishment of ecological civilization demonstration zones improve agricultural green efficiency? Based on the empirical data of hunan province. J. Central South Univ. Sci. 26 (3), 90–101. doi:10.11817/j.issn.1672-3104.2020.03.011
Luo, X., Sun, K., Li, L., Wu, S., Yan, D., Fu, X., et al. (2021). Impacts of urbanization process on PM2. 5 pollution in" 2+ 26" cities. J. Clean. Prod. 284, 124761. doi:10.1016/j.jclepro.2020.124761
Ma, S., and Feng, H. (2013). Will the decline of efficiency in China's agriculture come to an end? An analysis based on opening and convergence. China Econ. Rev. 27, 179–190. doi:10.1016/j.chieco.2013.04.003
Magarey, R. D., Klammer, S. S., Chappell, T. M., Trexler, C. M., Pallipparambil, G. R., and Hain, E. F. (2019). Eco-efficiency as a strategy for optimizing the sustainability of pest management. Pest Manag. Sci. 75 (12), 3129–3134. doi:10.1002/ps.5560
Mao, J., Lv, B., and Ma, G. (2015). The transfer payment and the goverment expansion: A study based on "the price effect. Manag. World 7, 29–41. doi:10.19744/j.cnki.11-1235/f.2015.07.005
McKenzie, D. J., and Sasin, M. J. (2007). Migration, remittances, poverty, and human capital: Conceptual and empirical challenges. United States: World Bank.
Min, J., and Hu, H. (2012). Calculation of greenhouse gases emission from agricultural production in China. China Popul. Resour. Environ. 22 (7), 21–27. doi:10.3969/j.issn.1002-2104.2012.07.004
Ministry of Agriculture (2015). “National agricultural sustainable development plan (2015–2030),” in National agricultural sustainable development plan (2015–2030) (China: Ministry of Agriculture Beijing).
Nie, W., and Yu, F. (2017). Review of methodology and application of agricultural eco-efficiency. Chin. J. Eco-Agriculture 25 (9), 1371–1380. doi:10.13930/j.cnki.cjea.170163
Pan, D., and Ying, R. (2013). Agricultural eco-efficiency evaluation in China based on SBM model. Acta Ecol. Sin. 33 (12), 3837–3845. doi:10.5846/stxb201207080953
Pang, J., Chen, X., Zhang, Z., and Li, H. (2016). Measuring eco-efficiency of agriculture in China. Sustainability 8 (4), 398. doi:10.3390/su8040398
Pang, X., and Ran, G. (2017). Study on the effect of traditional urbanization and local urbanization on farmers' income: The perspective of time varying analysis. China Soft Sci. 9, 91–98.
Peng, X. (2011). China’s demographic history and future challenges. Science 333 (6042), 581–587. doi:10.1126/science.1209396
Qi, M. (2014). Economic impact of rural to urban labor migration in China from 1991 to 2011. China Popul. Resour. Environ. 24 (4), 127–135.
Qi, W. (2019). Spatio-temporal pattern and drivers of the population urbanization in the Tibetan plateau. J. Geo-information Sci. 21 (8), 1196–1206.
Rybaczewska-Błażejowska, M., and Gierulski, W. (2018). Eco-efficiency evaluation of agricultural production in the EU-28. Sustainability 10 (12), 4544. doi:10.3390/su10124544
Satterthwaite, D., McGranahan, G., and Tacoli, C. (2010). Urbanization and its implications for food and farming. Philosophical Trans. R. Soc. B Biol. Sci. 365 (1554), 2809–2820. doi:10.1098/rstb.2010.0136
Schaltegger, S., and Sturm, A. (1990). Öologische rationalität (German/in English: Environmental rationality). Unternehm. 4, 117–131.
Schmidheiny, S., and Stigson, B. (2000). Eco-efficiency: Creating more value with less impact. United States: World Business Council for Sustainable Development.
Shang, J., Ji, X., and Chen, X. (2020). Study on the impact of China’s urbanization on agricultural ecological efficiency: Based on panel data of 13 major grain-producing regions in China from 2009 to 2018. Chin. J. Eco-Agriculture 28 (8), 1265–1276. doi:10.13930/j.cnki.cjea.200135
She, Z. (2015). The rural areas experiences conflicts under the urbanization process and its development issue. Chin. J. Environ. Manag. 7 (3), 1–7. doi:10.16868/j.cnki.1674-6252.2015.03.011
Sueyoshi, T., Yuan, Y., and Goto, M. (2017). A literature study for DEA applied to energy and environment. Energy Econ. 62, 104–124. doi:10.1016/j.eneco.2016.11.006
Suzigan, L. H., Peña, C. R., and Guarnieri, P. (2020). Eco-efficiency assessment in agriculture: A literature review focused on methods and indicators. J. Agric. Sci. 12, 118. doi:10.5539/jas.v12n7p118
Tang, M., Li, Z., Hu, F., and Wu, B. (2020). How does land urbanization promote urban eco-efficiency? The mediating effect of industrial structure advancement. J. Clean. Prod. 272, 122798. doi:10.1016/j.jclepro.2020.122798
Taylor, J. E., and Lopez-Feldman, A. (2010). Does migration make rural households more productive? Evidence from Mexico. J. Dev. Stud. 46 (1), 68–90. doi:10.1080/00220380903198463
Toma, P., Miglietta, P. P., Zurlini, G., Valente, D., and Petrosillo, I. (2017). A non-parametric bootstrap-data envelopment analysis approach for environmental policy planning and management of agricultural efficiency in EU countries. Ecol. Indic. 83, 132–143. doi:10.1016/j.ecolind.2017.07.049
Vlontzos, G., Niavis, S., and Manos, B. (2014). A DEA approach for estimating the agricultural energy and environmental efficiency of EU countries. Renew. Sustain. Energy Rev. 40, 91–96. doi:10.1016/j.rser.2014.07.153
Wang, B., and Zhang, W. (2018). Cross-provincial differences in determinants of agricultural eco-efficiency in China: An analysis based on panel data from 31 provinces in 1996-2015. Chin. Rural. Econ. 1, 47–62.
Wang, L., Bai, X., Zhang, X., Reis, S., Chen, D., Xu, J., et al. (2021b). Urbanization can benefit agricultural production with large-scale farming in China. Nat. Food 2 (3), 183–191. doi:10.1038/s43016-021-00228-6
Wang, L., Qi, Z., Pang, Q., Xiang, Y., and Sun, Y. (2021a). Analysis on the agricultural green production efficiency and driving factors of urban agglomerations in the middle reaches of the Yangtze River. Sustainability 13 (1), 97. doi:10.3390/su13010097
Wang, L., Yao, S., Hou, M., Jia, L., Li, Y., Deng, Y., et al. (2021c). Spatial-temporal differentiation and its influencing factors of agricultural eco-efficiency in China based on geographic detector. Chin. J. Appl. Ecol. 32 (11), 4039–4049. doi:10.13287/j.1001-9332.202111.026
Wang, S., and Lin, Y. (2021). Spatial evolution and its drivers of regional agro-ecological efficiency in China's from the perspective of water footprint and gray water footprint. Sci. Geogr. Sin. 41 (2), 290–301. doi:10.13249/j.cnki.sgs.2021.02.012
Wang, Y., and Huang, Y. (2022). Impact of agricultural resource misallocation on agricultural eco-efficiency: Evidence from 13 major grain producing areas in China. Ecol. Econ. 38 (5), 129–137.
Wang, Y., Yao, X., and Zhou, M. (2013). Rural labor outflow, regional difference and grain production. Manag. World 11, 67–76. doi:10.19744/j.cnki.11-1235/f.2013.11.007
Wang, Y., and Yu, Y. (2021). Evolution in the spatio-temporal pattern of agricultural sustainable development level in China based on multi-scales. J. Agric. Sci. Technol. 23 (3), 8–17. doi:10.13304/j.nykjdb.2020.0357
Wen, Z., Liu, h., and Hou, J. (2012). Analyses of moderating and mediating effects. United States: Educational Science Publishing House.
Wu, H., Huang, H., He, Y., and Chen, W. (2021). Asymmetric organocatalytic vinylogous michael addition triggered triple-cascade reactions of 2-hydroxycinnamaldehydes and vinylogous nucleophiles: Construction of benzofused oxabicyclo[3.3.1]nonane scaffolds. Chin. J. Eco-Agriculture 29 (10), 1762–1765. doi:10.1039/d0cc07761e
Wu, W., and Liu, Y. (2017). The impact of non-agircultural income on input structure of agricultural factors under the background of rural labour migration. Chin. J. Popul. Sci. 2, 70–79.
Wu, X., Ge, P., and Xu, Z. (2019). Urbanization and the promotion of agricultural total factor productivity: Heterogeneity and spatial effect. China Popul. Resour. Environ. 29 (5), 149–156. doi:10.12062/cpre.20181119
Xie, L. (2021). Employment of rural labor force in the 14th five-year Plan period: Situation outlook, structure forecast and thought contermeasure. Issues Agric. Econ. 3, 28–39. doi:10.13246/j.cnki.iae.2021.03.003
Xu, L., and Tan, J. (2020). Financial development, industrial structure and natural resource utilization efficiency in China. Resour. Policy 66, 101642. doi:10.1016/j.resourpol.2020.101642
Yan, M., Qiao, J., Qu, M., and Han, D. (2022). Agricultural eco-efficiency measurements, spatial spillover and influencing factors in Henan province. Jounal Ecol. Rural Environ. 3, 28. doi:10.19741/j.issn.1673-4831.2021.0772
Yang, B., Zhang, Z., and Wu, H. (2022). Detection and attribution of changes in agricultural eco-efficiency within rapid urbanized areas: A case study in the urban agglomeration in the middle reaches of Yangtze River, China. Ecol. Indic. 144, 109533. doi:10.1016/j.ecolind.2022.109533
Yang, G., Duan, Y., and Cui, L. (2023). Analysis of county differences and influence factors of agricultural ecological efficiency in Shanxi province. Chin. J. Agric. Resour. Regional Plan. 44 (3), 70–78. doi:10.7621/cjarrp.1005-9121.20230308
Zhang, R., Jiang, G., and Zhang, Q. (2019). Does urbanization always lead to rural hollowing? Assessing the spatio-temporal variations in this relationship at the county level in China 2000–2015. J. Clean. Prod. 220, 9–22. doi:10.1016/j.jclepro.2019.02.148
Zhang, Y., and Chen, J. (2019). International comparison of agroecological efficiency and chian's positioning study. China Soft Sci. 10, 165–172.
Zhang, Y. (2018). The threshold effect of Urbanization and Agro-ecological efficiency from a low-carbon perspective. Jounal Financial Econ. Theory 6, 78–88. doi:10.13894/j.cnki.jfet.2018.06.008
Zhang, Y., Zhang, L., and Han, L. (2021). Study on the evaluation and promotion path of agricultural ecological efficiency: An empirical analysis of 17 prefecture level cities in Shandong province. Ecol. Econ. 37 (4), 118–131.
Zhang, Z., Liao, X., Li, C., Yang, C., Yang, S., Li, Y., et al. (2022). Platelet membrane-encapsulated MSNs loaded with SS31 peptide alleviate myocardial ischemia-reperfusion injury. Econ. Geogr. 42 (2), 181–189. doi:10.3390/jfb13040181
Zhao, D., and Xu, J. (2021). The Spatio-temporal patterns evolution and influence mechanism of situ urbanization in Henan Province. Geogr. Res. 40 (7), 1978–1992. doi:10.11821/dlyj020200698
Zhao, W., Jiao, B., and Han, Y. (2018). Estimation and prediction of China's agricultural surplus labor stock in 1984-2050. Popul. Res. 42 (2), 54–69.
Zhou, B., Aggarwal, R., Wu, J., and Lv, L. (2021). Urbanization-associated farmland loss: A macro-micro comparative study in China. Land Use Policy 101, 105228. doi:10.1016/j.landusepol.2020.105228
Zhou, C., Wang, S., and Wang, J. (2019). Examining the influences of urbanization on carbon dioxide emissions in the Yangtze River Delta, China: Kuznets curve relationship. Sci. Total Environ. 675, 472–482. doi:10.1016/j.scitotenv.2019.04.269
Zhou, K., Zheng, X., Long, Y., Wu, J., and Li, J. (2022). Environmental regulation, rural residents’ health investment, and agricultural eco-efficiency: An empirical analysis based on 31 Chinese provinces. Int. J. Environ. Res. public health 19 (5), 3125. doi:10.3390/ijerph19053125
Zhu, W., Qi, L., and Wang, R. (2021). Impact of urbanization on pesticide use intensity and fertilizer use intensity: Analysis based on the mediation effect. Sustainability 8, 1–9.
Zhu, W., and Wang, R. (2021). Effect of urbanization on agricultural land-use intensity and mediation analysis. J. China Agric. Univ. 26 (4), 213–224. doi:10.11841/j.issn.1007-4333.2021.04.20
Zhu, Y., Sun, C., and Huang, D. (2022a). Labor transfer, agriculture large-scale operation and agricultural ecological efficiency. J. Fujian Agric. For. Univ. (Philosophy Soc. Sci. 25 (4), 32–42. doi:10.13322/j.cnki.fjsk.2022.04.006
Appendix A
Keywords: urbanization, agricultural eco-efficiency, GB-US-SBM model, mediation effect model, agricultural labor, rural residents’income
Citation: Zhao X, Yang J, Chen H, Zhang X and Xi Y (2023) The effect of urbanization on agricultural eco-efficiency and mediation analysis. Front. Environ. Sci. 11:1199446. doi: 10.3389/fenvs.2023.1199446
Received: 05 April 2023; Accepted: 24 May 2023;
Published: 01 June 2023.
Edited by:
Salvador García-Ayllón Veintimilla, Technical University of Cartagena, SpainReviewed by:
Sufyan Ullah Khan, University of Stavanger, NorwayMatheus Koengkan, University of Aveiro, Portugal
Copyright © 2023 Zhao, Yang, Chen, Zhang and Xi. This is an open-access article distributed under the terms of the Creative Commons Attribution License (CC BY). The use, distribution or reproduction in other forums is permitted, provided the original author(s) and the copyright owner(s) are credited and that the original publication in this journal is cited, in accordance with accepted academic practice. No use, distribution or reproduction is permitted which does not comply with these terms.
*Correspondence: Xiaojing Zhao, emhhb3hqMTk4NjAzQDE2My5jb20=