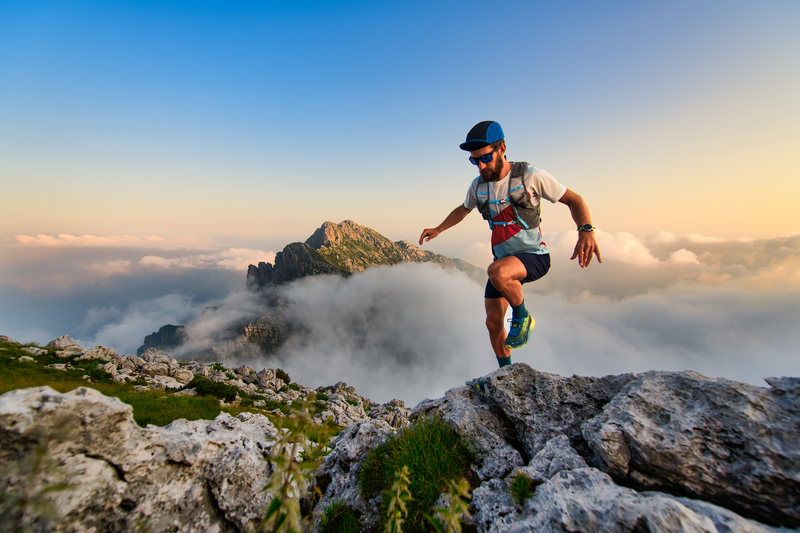
94% of researchers rate our articles as excellent or good
Learn more about the work of our research integrity team to safeguard the quality of each article we publish.
Find out more
ORIGINAL RESEARCH article
Front. Environ. Sci. , 11 October 2023
Sec. Environmental Citizen Science
Volume 11 - 2023 | https://doi.org/10.3389/fenvs.2023.1186238
This article is part of the Research Topic Environmental Citizen Studies in Freshwater Science View all 8 articles
Citizen science is often promoted as having the capacity to enable change–from increasing data provision and knowledge product development, via behavioral change of citizens, to problem-solving. Likewise, researchers increasingly emphasize the role of project design in initiating these changes through citizen science. However, respective claims are mostly based on single case studies and reviews, calling for a systematic comparative approach to understanding the effects of project design on change. Based on a survey of 85 water-related citizen science projects from 27 countries, we analyze the comparative effects of literature-based design principles on project impacts. Factor analysis first reveals three key impact factors which are ‘Data output’, ‘Citizen outcome’, and ‘Impact chain’. Regression analysis then shows that these impact factors are significantly influenced by several design factors, amongst which motivational factors are most prominent. The analysis also shows that design factors are most important for ‘Impact chain’, followed by ‘Citizen outcome’, and ‘Data output’. While design factors only partly explain the overall project effects, the regression results are rather stable and significant when including other potential influencing factors like project responsibility and funding. In sum, the results provide an empirically substantiated and differentiated understanding of citizen science impacts and how these are influenced by project design.
Human behavior and environmental change are inevitably interlinked in complex social-ecological systems: Humans influence the quantitative and qualitative status of environmental resources such as water, soil, and biodiversity. Likewise, environmental resources provide the boundaries for human action and wellbeing (Berkes et al., 2000; Steffen et al., 2015; Colding and Barthel, 2019). In the Anthropocene, these relationships between humanity and the environment are uniquely imbalanced, with detrimental effects of human actions on environmental resources, resulting in relatively poor quality of air, soils, and waters, as well as rapidly advancing biodiversity loss (Steffen et al., 2011; UN Environment, 2019). Hence, the international community calls upon modern societies to change their behavior towards more sustainable human-environmental interactions within its 2030 Agenda for Sustainable Development (A/RES/70/1, 2015; E/CN.3/2017/2, 2017).
While there are many ways to induce change, scholars and practitioners increasingly encourage citizens to provoke change through citizen science (Fritz et al., 2019; Fraisl et al., 2020; Shulla et al., 2020). There are various ways to define citizen science depending on the specific field of research. In the field of environmental sciences, citizen science is often defined as ‘(t)he collection and analysis of data relating to the natural world by members of the general public’ (Oxford Dictionary, 2020). Following this definition, citizen science may provoke change through environmental monitoring, in particular. Such monitoring processes likely increase the availability of environmental data and related products but may also trigger outcomes such as raising citizen’s awareness of environmental problems and, therefore, may also induce better management of the environment in support of the Sustainable Development Goals in their social, economic, and ecologically dimensions (Storey et al., 2016; Schröter et al., 2017; Church, et al., 2019; MfN, 2020; Turbé et al., 2020).
While visions aim high, scholars also emphasize that design affects success, and thus argue for analysing design factors of citizen science projects and how they influence projects’ aims to create change (e.g., Conrad and Hilchey, 2011; Buytaert et al., 2014; McKinley et al., 2017; Peter et al., 2021; Fraisl et al., 2022). Examples of such design factors are the actual knowledge of citizens, the training of citizens, or feedback loops, which may all improve socio-temporal data or increase citizen engagement (Kosmala et al., 2016; Jollymore et al., 2017; Zhou et al., 2020). But while individual design factors have been associated with specific types of effects in case studies and reviews, it is widely unclear how the different design factors influence project results from a comparative perspective. Are data outputs, societal outcomes, and political impacts influenced by the same design factors? Or are these different types of results also influenced by different design factors? Answers to these questions are crucial when discussing the role of citizen science in global environmental change since they show how change can be triggered through project design.
Taking freshwater-related citizen science projects as an example, this paper analyzes the comparative effects of various design principles on diverse project results. Based on a global survey amongst 85 citizen science projects in the field of water quality monitoring, we ask i) which impacts these projects have along the results chain including outputs, outcomes, and further impacts, ii) how these impacts can be traced back to specific design factors, and iii) how robust these relationships are under specific context conditions. To this end, this paper builds on a survey instrument on citizen-based water quality monitoring that was first introduced by Kirschke et al. (2022). This article presents as yet unpublished data on the impact of citizen science and links these to published data on project design to understand how these impacts may be influenced by design. Freshwater quality is a particularly interesting field for this analysis, given the increasing number of studies in the field which, however, lack a systematic comparative analysis of the effects of various design principles on different types of results (San Llorente Capdevila, 2020; Kirschke et al., 2022; Nath and Kirschke, 2023; Ramírez, 2023).
Section 2 introduces the conceptual framework for analyzing the effects of design factors on project results and provides literature-based guiding assumptions on the potential role of various design factors of citizen science projects for short-, medium-, and long-term impacts. Section 3 introduces the study field and the methodology of this research, including data collection through a global survey of water-related citizen science projects and the quantitative methods of descriptive and advanced statistics for analyzing the data. Section 4 presents the results of the descriptive analysis for 11 design variables, identifies three key impact factors based on factor analysis, and reveals the role of design factors for these impact factors based on regression analysis. Section 5 discusses the results in light of our guiding assumptions and Section 6 concludes on the role of citizen science design for inducing global environmental change.
Citizen science research from different fields of practice has revealed various understandings of citizen science, numerous design principles of citizen science projects, and various results of these projects along a chain of effects from data outputs to real word problem-solving (Gharesifard et al., 2019a; Hecker et al., 2019; Hicks et al., 2019; Haklay et al., 2021; Skarlatidou and Haklay, 2021; Wehn et al., 2021). For this research, we follow the narrow monitoring-related definition of citizen science introduced above. Thus, the focus is on citizen science projects that involve citizens at least in the monitoring process, which have also been denoted as ‘distributed intelligence’ (Haklay, 2013) or ‘contributory citizen science’ (Ngo et al., 2023). In this study, this narrow definition of citizen science does, however, not exclude further involvements of citizens such as in the joint design of research with citizens as it would be the case in ‘extreme citizen science’ (Haklay, 2013) and ‘transdisciplinary research’ (Lang et al., 2012; Lawrence et al., 2022).
We further use a comprehensive categorization of citizen science design principles including citizen characteristics (e.g., gender, age), institutional characteristics (e.g., organizational responsibility), and process mechanisms (e.g., training) (San Llorente Capdevila et al., 2020; Kirschke et al., 2022). This framework is combined with three impact categories, representing a chain of effects, from ‘Outputs’ (e.g., data quantity) via ‘Outcomes’ (e.g., awareness raising) to further ‘Impacts’ (e.g., on problem-solving) (UNDG, 2011; Church et al., 2019; Turbé et al., 2020). Below, we have outlined these well-recognized categories of design principles (‘Citizens’, ‘Processes’, and ‘Institutions’) and impact types (‘Outputs’, ‘Outcomes’, and further ‘Impacts’) (see Figure 1).
In terms of design principles, research in various fields of practice has come up with a multitude of design variables or principles that potentially influence project results (Chase and Levine, 2016; Wald et al., 2016; San Llorente Capdevila, 2020). Also, citizen science associations have formulated several principles for successful citizen science projects (ECSA, 2015; Haklay et al., 2020). Design variables that potentially influence results in the field of water resource monitoring have been condensed to key design factors related to citizen characteristics, institutional characteristics, and interaction forms (Kirschke et al., 2022).
In terms of ‘Citizens’, researchers emphasize that the citizen background is relevant, including age, education, and prior knowledge (Alender, 2016; Chase and Levin, 2016; Mac Domhnaill et al., 2020). Furthermore, citizen’s motivation has been discussed intensely, resulting in the identification of manifold motivational factors which have also been operationalized in various ways (Geoghegan et al., 2016; Wehn and Almomani, 2019; Larson et al., 2020; Lotfian et al., 2020; Land-Zandstraet al., 2021; West et al., 2021). Prominent examples of citizen’s motivations refer to i) intrinsic motivational factors such as environmental awareness, addressing specific local problems, or citizen’s interests in science, as well as ii) citizen’s extrinsic motivation such as financial compensation or the organization of social events (e.g., Alender, 2016; Larson et al., 2020; Mac Domhnaill et al., 2020; Land-Zandstra et al., 2021). These and additional motivational factors have further been related to different belief domains, including behavioral beliefs (and associated tangible and intangible outcomes as well as social outcomes), normative beliefs (perceived social pressure of referents), and control beliefs (including internal and external factors) of citizens (Wehn and Almomani, 2019).
With regard to ‘Institutions’, this analysis refers to academic entities, civil society organizations, or public bodies that are often involved in citizen science activities (Haklay et al., 2020; Kelly et al., 2020). Research has emphasized institution’s motivation to create both research outputs (e.g., high-quality data and knowledge products) and outcomes (e.g., awareness raising or network building), among others (e.g., Geoghegan et al., 2016; Chase and Levine, 2018; Wehn and Almomani, 2019; Haklay et al., 2020; MacPhail and Colla, 2020). Again, these and additional motivational factors have further been related to the three belief domains, including behavioral beliefs (different types of outcomes, including outcomes for the organization’s resources, activities, and strategic position, just as well as further societal outcomes), normative beliefs (perceived social pressure of referents), and control beliefs (including internal and external factors) of scientists and decision-makers as two example groups of involved institutions (Wehn and Almomani, 2019).
In terms of ‘Processes’, different exchange mechanisms for communication and feedback have been identified, amongst them direct exchange through emails and face-to-face, postal mail exchange, as well as digital exchange through websites, social media, and mobile apps (Le Coz et al., 2016; Ratniecks et al., 2016; Wald et al., 2016; Kelly et al., 2020; Aristeidou et al., 2021; Oliveira et al., 2021). Also, researchers emphasize training and support to collect and transfer data (Kosmala et al., 2016; Le Coz et al., 2016; Kelly et al., 2020; Zhou et al., 2020).
In terms of impacts, recent research in the field has argued for broad conceptualizations of impacts including a diversity of analytical categories including the five impact domains of ‘society, economy, environment, science and technology, and governance’ and specific guiding principles for consolidated impact assessment. This research also understands impact in a broad sense, covering all potential effects along the results chain from ‘outputs’ and ‘outcomes’ to further ‘impacts’. This understanding of impacts along the results chain allows for analyzing and interpreting specific results of projects rather than referencing back to broad impact categories. The following specific outputs, outcomes, and impacts have been detected in the water, environmental, and science of citizen science literature and thus also guided our empirical analysis in 2020. The identified outputs, outcomes, and impacts cover four out of five potential impact domains:
‘Outputs’ mostly relate to the domain of science and technology. Citizen science projects mostly emphasize data outputs, such as an increase in spatial and temporal data (Lottig et al., 2014; Breuer et al., 2015; Fraisl et al., 2022), good quality data (Crall et al., 2011; Kosmala et al., 2016; Quinlivan et al., 2020), and cost-effectiveness in data collection (Breuer et al., 2015). Furthermore, researchers highlight the generation of knowledge products based on the collected data. Research has shown, for instance, a relatively strong increase in citizen science-related research after 2010 in the field of biology and conservation (Kullenberg and Kasperowski, 2016).
Research further highlights ‘Outcomes’, which are here defined as the effects of citizen science projects on participating citizens. This category is thus closely related to the societal domain. Outcomes are, for instance, awareness raising (Chase and Levine, 2016; Brouwer et al., 2018; Peter et al., 2019), increased knowledge about science and nature (Church et al., 2019; Peter et al., 2019; Peter et al., 2021), engaging citizens in research or politics (McKinley et al., 2017; Peter et al., 2019), or building up a network (Johnson et al., 2014; Chase and Levine, 2016).
Finally, research also highlights further (potential) ‘Impacts’ on problem-solving, including the advancement of political decisions, and hence also actual solutions to sustainability problems (Muenich et al., 2016; McKinley et al., 2017; Carlson and Cohen, 2018; Pocock et al., 2019; Turbé et al., 2020; Schade et al., 2021; van Noordwijk et al., 2021). This category thus relates to the impact categories of ‘governance’ and ‘environment’ as highlighted by Wehn et al. (2021).
How are the design factors of citizen science projects and the potential impacts of citizen science projects connected? Given the vast literature in the field, we assume that the mentioned design factors affect impacts along the results chain, but in various ways. Two main assumptions guide our analysis:
The first guiding assumption regards the type of relationship. We assume that different (groups of) design factors influence different (groups of) results. Put differently, results at the output, outcome, and further impact level are likely influenced by different types of design factors. There are several reasons for this: Research first indicates that outputs, particularly the quality and quantity of the data collected, are mostly influenced by specific citizen characteristics and process mechanisms. To give some examples, the educational background (Chase and Levine, 2016; Mac Domhnaill et al., 2020) and knowledge of citizens (Jollymore et al., 2017), together with training and technical supporting structures (Kosmala et al., 2016; Ratnieks et al., 2016) likely influences the quality of data. Also, simple tools for data transfer such as apps and text messaging are supposed to increase spatial and temporal data (Lowry and Fienen, 2013; LeCoz et al., 2016). Outcomes, such as awareness raising, increase in knowledge, and the engagement of citizens, may mostly go back to citizen characteristics, e.g., the actual knowledge and awareness when starting the activity (Buytaert et al., 2014; Jollymore et al., 2017), or citizen’s educational and professional background (Chase and Levine, 2016; Mac Domhnaill et al., 2020). Further impacts, such as advancements in political decisions or improving environmental conditions may go back to the institutions involved in projects and their motivation to advance political decisions (Schade et al., 2021; van Noordwijk et al., 2021).
The second guiding assumption regards the strength of relationships. We assume here that design factors influence outputs more rigorously than outcomes and further impacts. In fact, the results-based management literature dealing with global environmental change suggests that outputs can be influenced more directly than outcomes and further impacts (UNDG, 2011; Bester, 2016). The citizen science literature has substantiated this argument, highlighting challenges in measuring middle to long-term outcomes and impacts from citizen science projects (Skarlatidou and Haklay, 2021; Wehn et al., 2021; Somerwill and When, 2022). Consequently, the strengths of the relationship between influencing factors and results may be much stronger at the output than at the outcome and the further impact level. Project managers, for instance, may therefore be able to design projects in a way, that the quantity and quality of products can be increased, e.g., through providing respective training material. Affecting citizens themselves and political decisions, however, will require more engagement and is probably less controllable by project managers.
This research builds on data collected through a global online survey amongst citizen science projects in the field of water quality monitoring (see Annex 1) which was first introduced by Kirschke et al. (2022). The survey aimed at understanding the comparative effects of citizen science projects in water quality monitoring on a global scale. To this end, the survey was designed iteratively based on systematic literature reviews related to citizen-based water monitoring and interviews with eight experts in the field of citizen science, water monitoring, and survey design including distinct regional perspectives. The final survey included five parts on basic project data (part one), the design of citizen science projects (part two to four), and their results (part 5). Data on parts one to four of the survey have been published while data on the effects of projects have remained unpublished and are first presented in this study. Thus, this paper presents new data on the impacts of citizen science which have not been reported elsewhere. These data have also been linked to published data on project design to understand how these impacts may be influenced by design. As such, only a small part of the data has been used again to answer a new scientific question that has not been addressed elsewhere.
The survey was distributed to the coordinators of 237 mature citizen science projects, representing 495 activities in 97 countries. There were 85 coordinators in 27 countries who completed the survey between 10 August and 8 September 2020, resulting in a response rate of 35.86%. Of the survey respondents, the average length of the project was 4 years and 4 months at the time of the survey. The majority of projects were from North America (54% of the answers), but there were also responses from Europe (17%), Latin America and the Caribbean (12%), Asia (9%), Africa (6%), and Oceania (2%). The project coordinators also judged both the actual project design and the impact chain including data outputs, societal outcomes, and impacts on problem-solving to the best of their knowledge as critical nodes between project members, citizens, funding agencies, and further stakeholders such as political actors.
Regarding this article’s key research interest on the impact of citizen science projects, this research analyses the unpublished data on impact variables collected through the global survey described above. The survey asked the respondents to rank the factual success of project results on a one to five scale, with 1 indicating no success and 5 indicating high success. The success was to be assessed along 11 impact variables. These include data-specific outputs (‘Increase in spatial data’, ‘Increase in temporal data’, ‘High-quality data provision’, ‘Cost-effectiveness’, and ‘Knowledge production’), citizen-related outcomes (‘Awareness raising’, ‘Citizen’s engagement in research’, ‘Citizen’s engagement in politics’, and ‘Network building’), and middle-term project impacts (‘Political decisions advancement’, and ‘Freshwater quality improvement’). In addition to the one to five scale, the survey offered a ‘do not know’ option to increase the likelihood of valid answers to the survey questions.
Regarding design variables and design factors, this research builds on previously published data by Kirschke et al., 2022 (see Annex 2). Based on the analysis of 45 design variables in the 85 citizen science projects, this research identified 10 key design factors using factor analysis. These factors comprise institutional characteristics (‘Output motivation of institutions’, and ‘Outcome motivation of institutions’), citizen characteristics (‘Citizen background’, ‘Problem-orientation of citizens’, and ‘Extrinsic motivation of citizens’), and interaction forms (‘Digital exchange’, ‘Training and technical support’, ‘Digital exchange 2.0’, ‘Direct exchange’, and ‘Mail exchange’). Respective factor scores are fully accessible to the team of authors and free to use for this analysis.
Further, and in terms of potential third influencing factors, this research builds on data collected through the same global survey. These data are also available in Annex 2 Tables 3 and 4. The dataset includes data on both the projects’ responsibility and respective funding agencies. With regards to responsible institutions, the data set differentiates single responsibilities (‘Academic institution’, ‘Non-governmental organization (NGO)’, or ‘Other responsible institution’), or multiple responsibilities including at least two out of the three categories mentioned before. In terms of the funding agency, the data set includes individual funding (‘Public agency’, ‘Non-profit organization’, and ‘Other funding institution’), as well as multiple funding responsibilities including at least two out of the three categories mentioned before. The data is available as dummy (0/1) variables for our analysis.
The data set is particularly well-suited for this analysis since it provides information on impact variables, key design factors identified by factor analysis, as well as on further potential influencing factors originating from the same survey and respondents. The survey paid attention to crucial challenges in defining the impacts of citizen science projects. First, the survey addressed citizen science projects that have been well-documented online. Second, the survey asked for 11 specific impacts along the results chain, narrowing down the vast field of potential impact interpretations in various project contexts (see also conceptual framework section). Third, the survey design allowed for ‘do not know’ answers to increase the likelihood of valid answers. Fourth, the survey generally asked for actual design and effects rather than potential impacts, which increases the likelihood of answers based on facts rather than assumptions. The authors do acknowledge though that any measurements of impacts are subject to important uncertainties which we further discuss in the discussion section.
The data analysis of impact variables started with descriptive statistics on the 11 defined variables. We first calculated median values, minimum and maximum values, as well as lower and upper quartiles and visualized respective results by boxplots. For this purpose, we used all valid answers without ‘do not know’ answers (response rate = 89.6% over 11 variables). Data analysis then continued with the identification of impact factors within this data set. For this purpose, we conducted a principal component-based factor analysis, using SPSS (version 27) (see also Backhaus et al., 2018; Brosius, 2018). To this end, we used all valid cases (84 out of 85), and all 11 variables, replacing missing values with variable means. Results showed that this data set is highly suitable for factor analysis, given a Kaiser-Meyer-Olkin measure of .716 and a significant Bartlett test at the 1 percent level (.000). This analysis revealed three impact factors, showing an eigenvalue above 1, and explaining 61.1% percent of the variance between the variables. The corresponding factor scores have been used in the regression analysis (see below).
As a next step of the analysis, we addressed this paper’s key question of how the three identified impact factors are influenced by the 10 design factors comprising citizen characteristics, institutional characteristics, and interaction forms. To this end, we applied linear multiple regression analysis, using SPSS (version 27) (see also Backhaus et al., 2018; Brosius, 2018), based on all 84 cases, and analyzing each impact factor individually. Hence, we estimated three models, with the estimations being based on standardized factor scores (z-factors with mean = 0 and standard deviation = 1), related to the 84 cases. The results show the impact of the (exogenous) design factors on the respective (endogenous) impact factor. The estimated coefficients can directly be compared as beta coefficients (using standardized factor scores for the estimation) and the significance levels show whether the estimates are acceptable. This is the basis for the interpretation of the estimation results. We further calculated the corrected R squared (including significance level) to assess the overall explanatory power of the respective estimation model. The calculated Durban-Watson statistics on error autocorrelation are acceptable.
We tested the stability of the estimation results and the relevance of the third influencing factor by integrating variables on project responsibility and funding agency into the regression model. We proceeded here step by step, to avoid multi-collinearity and to be able to assess the implications for the estimation. This approach yielded four additional estimations for each impact factor, summing up to 12 additional estimations. The analysis shows i) the potential implications on estimation results for the design factors, ii) the relevance of individual third influencing factors, and iii) the potential implications for the overall explanatory power of the estimation model.
The results of the survey indicate that the projects have been successful with regard to the impact variables, with a median of 4 or above for most variables. However, the results of the survey also reveal variations between the 11 variables. On the upper part of the spectrum, the variable ‘Cost-effectiveness’ has been rated very high (median = 5), which is closely followed by the variables ‘Increase in spatial data’ and ‘Awareness raising’ (both median = 4.5). Further, five variables have been rated as rather successful, including the variables ‘Increase in temporal data’, ‘High-quality data provision’, ‘Knowledge production’, ‘Citizen’s engagement in research’, and ‘Network building’ (all median = 4). On the lower part of the spectrum, three variables were rated moderately, including the variables ‘Citizen’s engagement in politics’, ‘Political decisions advancement’, and ‘Freshwater quality improvement’ (all median = 3). There is also considerable variation between the project answers, with minimum and maximum values ranging between 1 and 5 for all variables, and lower and upper quartiles ranging between 3 and 5 for most of the variables (see Figure 2 and Annex 3 Table 1).
FIGURE 2. Survey results for impact variables. Depicted are median, minimum, and maximum values, and lower and upper quartiles. Source: Own calculation based on survey data set and descriptive statistics analysis using Excel.
Impact factors were calculated using factor analysis and were based on the eleven impact variables. The analysis revealed three impact factors, with an eigenvalue above 1, in sum explaining 61.1% of the variance. Impact factor 1 has been denoted as ‘Citizen outcome’, as the factor specifies ‘Outcomes’ according to the conceptual framework presented in Section 2. The factor mainly refers to the variables ‘Citizen’s engagement in research’, ‘Awareness raising’, ‘Citizen‘s engagement in politics’, ‘Advancement of political decisions’, and ‘Network building’. Factor 2 can be best described as ‘Impact chain’ (from data to action), as the factor relates to both ‘Outputs’ and further ‘Impacts’ according to the conceptual framework. This factor mainly refers to the variables ‘Freshwater quality improvement’‚ ‘High-quality data provision’, and ‘Knowledge production’. Correspondingly, factor 3 relates to ‘Data output’ and mainly includes the variables ‘Increase in spatial data’, ‘Increase in temporal data’, and ‘Cost-effectiveness’ (see Table 1 and rotated component matrix in Annex 3 Table 2).
TABLE 1. Identification of impact factors based on factor analysis. Depicted are impact variables with a factor load >.45 in descending order. Source: Own calculation based on survey data set and factor analysis using SPSS.
Regression analysis reveals that the design factors affect project results, but that their effects are rather specific and that the overall contribution to project success is limited. Many of the estimated coefficients are close to zero and only eight out of 30 coefficients are significant at a 5% level (with one more coefficient at the limit). The corrected R squared varies between .104 and .281 which is rather low, but significant at a 5% level, clearly indicating that project success will also (and probably more) depend on other variables beyond the design factors. The calculated Durban-Watson statistics on error autocorrelation are acceptable. Hence, the regression analysis does not produce exceptional results, but the estimates are statistically convincing and they provide important insights on the role of design factors on project impacts.
Interestingly, the three impact factors are in part explained by different design factors, with some factors being more prominent than others, and other factors showing no significant effects. Results indicate that the factors ‘Output motivation of institution’ and ‘Outcome motivation of institution’ are the most important design factors, followed by ‘Training and technical support’, ‘Citizen background’, and ‘Extrinsic motivation of citizens’. In contrast, most interaction forms (‘Digital exchange’, ‘Digital exchange 2.0’, ‘Direct exchange’, and ‘Mail exchange’) as well as ‘Problem orientation of citizens’ could not be confirmed as significant design factors. The estimation results are presented in Table 2.
TABLE 2. Linear regression results on the role of design factors for project impacts. Depicted are regression coefficients, with significance levels in brackets (bold numbers indicate a 5% significance level). Source: Own calculations based on survey data set, factor analysis, and regression analysis using SPSS.
‘Data output’ is not influenced by the design factors, as most coefficients are close to zero and not significant. An exemption is the design factor ‘Output motivation of institutions’, showing a positive coefficient (.303) which is highly significant (.005). Another exemption is the factor ‘Problem orientation of citizens’, also showing a positive impact (.205) which is not significant at the 5% level (.052) but in a two-factor model (.207 (.049)). Taking all 10 factors together, the explained variance is rather low with about 10% (corrected R squared = .104), but also significant at the 5% level (.050).
‘Citizen outcome’ is also not influenced by the design factors, as again most coefficients are close to zero and not significant. There are, however, two exemptions. First, and most importantly, the design factor ‘Outcome motivation of institutions’ shows a considerable positive coefficient (.432) which is highly significant (.000). Second, ‘Citizen outcome’ is slightly negatively influenced by ‘Citizen background’ (−.224), which is also significant (.025). Taking all 10 factors together, the explained variance of the impact factor ‘Citizen outcome’ is slightly higher than for ‘Data output’, with an explained variance of about 20% (corrected R squared = .205), which is also significant (.002).
The third impact factor ‘Impact chain’ is slightly more influenced by the design factors, with five out of ten significant positive coefficients at the 5% level. The most important influencing factor is ‘Output motivation of institutions’ (.377 (.000)), followed by the design factors ‘Training and technical support’ (.266 (.006)), ‘Extrinsic motivation of citizens’ (.207 (.029)), ‘Citizen background’ (.204 (.032)), and ‘Outcome motivation of institution’ (.189 (.046)). Overall, the explained variance of the impact factor ‘Impact chain’ is higher than for ‘Data output’ and ‘Citizen outcome’, with an explained variance of about 28% (corrected R squared = .281), which is also significant at the 1% level (.000).
The regression analysis has shown that the impact factors are in part influenced by the exogenous design factors, but to a low degree only with an explained variance of 10%–28%. We, therefore, examine the relevance of third influencing factors, by analyzing i) the potential implications on estimation results for the design factors, ii) the relevance of individual third influencing factors, and iii) the potential implications for the overall explanatory power of the estimation model.
We focus on two potentially key third influencing factors: the responsible institutions and the funding agencies. Responsible institutions can include the individual actors ‘Academic institution’, ‘Non-governmental organization’, and ‘Other responsible institution’, or multiple actors including at least two out of the individual actors mentioned. Funding agencies can include individual funders such as ‘Public agency’, ‘Non-profit organization’, and ‘Other funding institution’, and again a combination of these, including at least two out of the individual institutions mentioned. The role of these third influencing factors has been analyzed following a stepwise approach, integrating responsible institutions and funding agencies separately into the regression model. Furthermore, to avoid multicollinearity, we formulated regression models for individual responsibilities and funding entities and for multiple responsibilities and funding entities, respectively. Hence, a total of 12 additional regression models have been estimated.
Regression analysis reveals that impact factors are only partly influenced by the potential third influencing factors, with only five out of 24 possible significant coefficients at a 5% level. Interestingly, four out of the five significant results refer to the role of funding agencies whereas only one refers to the responsible institutions, hinting at a more significant role of who funds rather than who leads a project. Surprisingly, the sign of several significant (and insignificant) coefficients is negative, suggesting that the impact of the corresponding responsible and/or funding institutions on project success is negative. However, the estimations show that the results concerning the 10 design factors are not strongly affected by the extended regression models. In most cases, the values of the estimated coefficients do not change a lot and the significant estimates are confirmed. This underlines that the estimation results concerning the design factors are robust and trustworthy. Overall, the extended regression models do not strongly contribute to the explanatory power of the model as compared to our analysis based on design factors only. Looking at the change in corrected R squared, an additional explanatory value due to extended modeling likely relates to funding agencies rather than to responsible institutions. These results are specified below as well as in Annex 3 Table 3a-c.
‘Data output’ is not influenced by third variables, as seven out of 8 estimated coefficients are not significant at a 5% level. An exemption is the third influencing factor ‘Public agency’ [.574 (.034)]. In consequence, the explanatory value of the third influencing factor is only minimal, increasing the explanatory power of a corrected R squared of .104 to a maximum of .169, for the regression model including individual funding agencies. Turning to the role of the potential third variables for the influence of design factors on ‘Data output’, we find that the identified coefficients are further substantiated. In fact, the design factor ‘Output motivation of institutions’ shows a slight increase for most calculations. The design factor ‘Problem-orientation of citizens’ shows a similar pattern, though to a lesser degree.
‘Citizen outcome’ is also not influenced by third variables, as seven out of eight coefficients are not significant at a 5% level. An exemption is the third influencing factor ‘Non-governmental organization’ as a responsible institution [−.592 (.029)]. Interestingly, the negative sign suggests a negative impact of this factor on ‘Citizen outcome’. Overall, the explanatory value of the third influencing factor is again minimal, with the analysis of individual responsible institutions increasing the explanatory value from a corrected R squared of .205 to a maximum of .235. Turning to the role of the potential third variables for the influence of design factors on ‘Citizen outcome’, we find that the identified coefficients are further substantiated.
‘Impact chain’ is also not influenced by third variables, although the results are here more diverse. The third influencing factor related to funding shows three coefficients that are significant at the 5% level. Funding through ‘Public agency’ and ‘Non-profit organization’ is negatively associated (−.535 (.029) and −.547 (.040) respectively), whereas the involvement of multiple funding agencies receives a positive coefficient (.445 (.026)). Overall, the explanatory value of the model increased from a corrected R squared of .281 to a maximum of .320 while the involvement of the responsible institutions does not show any of such effects. Turning to the role of the potential third variables for the influence of design factors on ‘Impact chain’, we find again that the identified coefficients are mainly substantiated, with coefficients for design factors ‘Output motivation of institution’, ‘Training and technical support’, ‘Extrinsic motivation of citizens’, ‘Citizen background’, and ‘Output motivation of institution’ changing slightly but staying mostly significant at a 5% level.
Citizen science can have manifold impacts, triggered by the design of citizen science projects and additional influencing factors. But while many studies have analysed the design effects of citizen science projects, a systematic comparison of impacts and how these are influenced by design was lacking. Our comparative analysis of 85 citizen science projects in the field of water quality monitoring addresses this research gap and reveals specific impact patterns of citizen science projects.
First, the results of the descriptive statistics suggest that citizen science projects in the field of water quality monitoring are successful in delivering impacts including data-related outputs, societal outcomes, and further impacts on problem-solving. However, the effects of citizen science projects differ as well, with some project results such as ‘Cost-effectiveness’ in data collection, the ‘Increase in spatial data’ and ‘Awareness raising’ being rated particularly positive, and other project results such as ‘Citizen’s engagement in politics’, ‘Advancement of political decisions’, and ‘Freshwater quality improvement’ being perceived less positively. These results substantiate the increasing body of literature which highlights that citizen science can increase spatial and temporal data and therefore is a valuable approach for addressing the existing lack of water quality and environmental data (Lottig et al., 2014; Breuer et al., 2015; Jollymore et al., 2017; Quinlivan et al., 2020). The results are also in line with citizen science evaluations highlighting the role of citizen science in raising awareness of environmental issues (Chase and Levine, 2016; Brouwer et al., 2018; Peter et al., 2019). The results, however, also question the probability of significant middle to long-term impacts of citizen science projects beyond ultimate data outputs (e.g., Fritz et al., 2019; Fraisl et al., 2020; Fritz et al., 2022; Sauermann et al., 2020).
In the next step, we analyzed how design influences the effects of these citizen science projects based on regression analysis using impact factors and design factors for the projects considered. To this end, we first identified impact factors underlying 11 impact variables for our data set in the field of water quality monitoring. As a result, factor analysis has revealed three impact factors denoted as ‘Data output’, ‘Citizen outcome’, and ‘Impact chain’. These three impact factors empirically substantiate our conceptual approach on impacts comprising ‘Outputs’, ‘Outcomes’, and further ‘Impacts’ as introduced in Section 2. The results are thus mostly in line with recent literature which differentiates impacts of citizen science projects along the chain of effects from more direct effects of data outputs to more indirect effects on citizens’ learning and network building up to political change (Skarlatidou and Haklay, 2021; Wehn et al., 2021). The results particularly substantiate the idea of change through knowledge product development based on citizen science data, since knowledge product development and political advancement are closely connected (Section 4.2). This, in turn, is in line with the environmental change literature, which highlights how citizen science can induce change at the policy level (Fritz et al., 2019; Fraisl et al., 2020; Fritz et al., 2022; Sauermann et al., 2020).
When it comes to the key question on the role of design effects for the impacts of water quality-related citizen science projects, we find that design has small but significant effects on impact factors. In terms of ‘Data output’, the identified positive effect of ‘Output motivation of institution’ is comprehensible, as high output motivation may encourage an institution to design the respective project in a way that increases data output. Also, a positive effect of ‘Problem orientation of citizens’ such as the awareness of potentially harmful environmental problems may incentivize citizens to collect data on the quality of water used for drinking or farming.
In terms of ‘Citizen outcome’, a positive effect of the ‘Outcome motivation of institution’ is again comprehensible, as high outcome motivation may encourage institutions to design the respective project in a way that also increases citizen change. The identified negative effect of ‘Citizen background’ (including the educational background) is not necessarily implausible as, e.g., citizens with higher educational levels may already have a certain level of knowledge, which may reduce learning in respective citizen science projects.
In terms of ‘Impact chain’, the identified positive effects of ‘Output motivation of institution’ and ‘Outcome motivation of institution’ fit the above-mentioned results and argumentation that the high motivation of institutions is a key factor for project design and success. The positive impacts of ‘Training and technical support’ as well as ‘Citizen background’ are also comprehensible, given that a high educational background paired with training may increase high-quality data that researchers can use for publications with a high impact on science and society (van der Wal, 2016). On the other hand, the positive effect of ‘Extrinsic motivation of citizens’ is surprising given the argument in the literature that intrinsic motivation is more relevant than extrinsic motivation for sustainable monitoring and public engagement (Lotfian et al., 2020).
Furthermore, the rather low overall role of exchange mechanisms for data transfer, communication, and feedback is surprising, as literature often argues for communication and feedback as well as for specific forms of interaction for inducing change (Le Coz et al., 2016; Ratniecks et al., 2016; Peter et al., 2021). One potential explanation is that different contexts, target groups, and research goals may require different forms of interaction, thus making the actual tools highly context-specific and less generalizable.
With regards to the question of the third influencing factor, we found that the institutions responsible for the water quality-related projects or their funding may indeed affect results. Still, these third influencing factors do not contribute significantly to the explanatory power of the estimated model. Also, the results on the role of design factors on project impacts are widely confirmed by the extended analysis.
In terms of ‘Data output’, a positive effect of a ‘Public agency’ may be in line with literature highlighting the role of financial means in monitoring processes, as means to fund technical tools for data collection, human resources for accompanying the monitoring processes scientifically, continuous feedback, etc. (Pateman et al., 2021). One may argue that public authorities can best address these needs for sustainable funding to secure data outputs.
In terms of ‘Citizen outcome’ and change, the negative effect of ‘Non-governmental organization’ as a responsible institution is very surprising, as one may rather expect that NGOs are particularly interested in citizen change and also have the best means to achieve this goal. One potential explanation is that NGOs often implement citizen science projects in regions with high problem pressure, which again may result in a stronger focus on data outputs than on the actual learnings of citizens.
Finally, and with regards to the ‘Impact chain’, the identified negative effects of individual funding by either a ‘Public agency’ or a ‘Non-profit organization’ and the rather strong positive effect of ‘Multiple funding institutions’ suggest that combined funding supports and induces change through a joint and integrated data-to-action approach. Interestingly, project responsibility alone does not significantly affect the ‘Impact chain’, suggesting that either individual (e.g., academic or NGO) or multiple responsibilities (e.g., academic and NGO) can induce change as long as the funding agencies team up in their funding of projects. This slightly questions the argument for joint project governance of science and practice (Sauermann et al., 2020) and may require further research.
The results have implications for the relevance of the two guiding assumptions of this article.
The first guiding assumption related to the type of relationship assumed that different (groups of) design factors influence different (groups of) results. Put differently, assumption one was that results at the output, outcome, and further impact level are influenced by different types of design factors. Based on the results described above, there is evidence to suggest that this assumption is confirmed: While we find relevant design factors (e.g., motivation of institutions) and others that are not relevant (e.g., specific forms of interactions), our analysis reveals significant variations on the role of specific design factors for the different impact factors. For instance, the design factor ‘Output motivation of institution’ influences ‘Data output’ whereas the design factor ‘Outcome motivation of institution’ influences ‘Citizen outcome’. These patterns are rather robust when additional influencing factors of responsible institutions and funding agencies are added to the analysis.
The second guiding assumption regarded the strength of relationships, in which we assumed that design factors influence outputs more rigorously than outcomes and further impacts. Based on our analysis, this guiding assumption has been rejected. While the descriptive analysis revealed higher impact values for outputs and outcomes than for further impact variables, regression analysis showed that design factors as such do not have a stronger effect on outputs than on outcomes and further impacts. In contrast, the explained variance of the impact factor ‘Impact chain’ is higher than for ‘Data output’ and ‘Citizen outcome’ and the regression yields the highest number of significant design factors. This is particularly interesting as it suggests that responsible institutions design projects according to their goals of creating outputs and outcomes, but may have to put additional effort into project design when it comes to creating impact along the impact chain from data to action. The identified role of multiple funding schemes including both non-profit organizations and public agencies as well as the significant design factors including training, citizen background, and diverse motivational aspects may be considered when designing citizen science for change in water monitoring.
Implications of findings considering potential caveats. There are important implications for further water quality-related research and practice. Above all, researchers and practitioners should consider that designing successful citizen science projects is complex and includes a diversity of potential impacts along the results chain and diverse design factors influencing these results. Those involved in project design should therefore allow for enough time to carefully design and discuss project design with those involved. Further, researchers and practitioners should carefully consider which impacts they aim for–from data outputs via societal outcomes to further impacts–as different design factors likely influence different types of impacts. Third, this research also suggests that water quality monitoring projects need to add additional effort to achieve further impacts beyond prominent data outputs and outcomes. Thus, researchers and practitioners who aim for long-term impacts of citizen science projects in the field of water quality monitoring should put special effort into the design phase of projects. To support this process, funding agencies should fund potentially time-intensive design phases in addition to the actual citizen science activity. Also, funding agencies may provide specific decision-support tools on how citizen science projects should be designed according to various goals and contexts to support projects in the environmental sciences. The funding of additional social science expertise in natural science-driven projects is key to designing and implementing impactful citizen science projects beyond the collection of data at a specific moment in time.
There are, however, a few caveats associated with our findings. First, the theoretical framework and the respective survey focused on the effects of specific design factors in the field of water quality monitoring. As such, the framework mainly builds on the respective literature of citizen science in the field of water quality monitoring, in particular, which was increasingly extended to the field of environmental sciences and the science of citizen science in the course of this research. As such, researchers having different foci or starting from different angles may involve alternative categories to define impact or design factors (e.g., Gharesifard et al., 2019a; Wehn and Almomani, 2019; Wehn et al., 2021). Consequently, any comparative analysis of the design effects of citizen science projects has to consider the respective purpose and angle the analysis stems from for drawing conclusions.
Second, the definition and selection of citizen science projects arguably affect the results as well as the transferability of results to other citizen science projects (Eitzel et al., 2017). Likewise, terminologies are constantly evolving and subject to ongoing discussion (Auerbach et al., 2019; Heigl et al., 2019; Haklay M. et al., 2021; Lin Hunter et al., 2023). This study focused on contributory citizen science projects in the field of water monitoring. As such, citizen science projects that are embedded in a more intensive transdisciplinary research process may have stronger effects on real-world problem-solving (Newig et al., 2019; Jahn et al., 2022). Likewise, an analysis of citizen science projects related to other environmental resources (e.g., soil or biodiversity), Sustainable Development Goals and targets (e.g., good ambient water quality vs. forest management), or including further impact domains (e.g., economy) may detect different effects due to alternative problem areas, citizen science practices, and analytical angles (Fraisl et al., 2020; Lee et al., 2020; Wehn et al., 2021).
Third, the background of respondents included in this survey obviously comes with potential biases in terms of the project settings (length of the projects) and regional perspectives (focus on Northern American focus). While these biases have to be considered with caution, we also find that this dataset provides a valuable snapshot of a certain moment in time. In addition, the levels of maturity of projects represented in this study as well as the existing patterns in regional distributions of citizen science projects are typical for water-related citizen science projects. Future studies will have to clarify if and how impact assessments change depending on the various moments in time and additional third influencing factors such as regional or cultural settings. Given frameworks for contextual analysis greatly support respective analyses (Gharesifard et al., 2019a; Gharesifard et al., 2019b). Closely related to this potential bias, the results build on project coordinators’ answers only and may be revised slightly by the involvement of additional perspectives such as the perspectives from other project members, the involved citizens, the funding agencies, or additional stakeholders such as political decision-makers. While including additional perspectives would have been desirable, we argue that project coordinators are best suited to answer design and impact questions based on their central position in complex networks of stakeholders, their role as principal investigators in citizen science projects, and their resulting knowledge about impacts and problems of impact assessments in their given project context (e.g., Sprinks et al., 2021). Also, our quantitative approach including 85 coordinators levels potential biases of individual coordinators.
Fourth, judging impact chains comes along with a substantial level of uncertainty, specifically when it comes to societal outcomes and further impacts. While data outputs in terms of quantity and quality can be judged based on clear criteria and test mechanisms, social outcomes cannot be measured directly but are usually based on surveys amongst participants, observations in projects, and feedback loops, and are thus subject to ongoing methodological discussions (Somerwill and Wehn, 2022). Further impacts are often delayed in time, crystallize far beyond short project durations, and are typically based on additional influencing factors such as interests of diverse actors, political majorities, or path dependencies, just to name a few (Lang et al., 2012; van Noordwijk et al., 2021; Lawrence, et al., 2022). Thus, any judgment of the political impacts of citizen science or other participatory research approaches has to be seen with caution and needs in-depth process tracing in individual cases. These potential uncertainties have always to be considered when judging impact-related research. Still, we argue that our quantitative approach provides robust complementary insights into the design effects of citizen science projects in a field that has been dominated by single case studies and study designs including different operationalizations of impacts and measurement approaches.
Research and practice often assume that carefully designed citizen science projects can enable change along the results chain–from actual data collection via behavioral change to actual problem-solving. We first substantiate this argument empirically, based on the designs and results of 85 water monitoring projects located in 27 countries around the globe. Our analysis first substantiates our basic assumption that the impact factors ‘Data output’, ‘Citizen outcome’, and ‘Impact chain’ are in part influenced by different types of design factors, amongst which motivational factors are most prominent. Our analysis, however, also questions our second guiding assumption by revealing that design mostly affects the ‘Impact chain’ while ‘Data output’ and ‘Citizen outcome’ are less affected. The inclusion of third variables on institutional responsibility and funding further substantiates these design effects, but also reveals that multiple funding agencies play a significant role in inducing change. In sum, results provide an empirically substantiated and differentiated understanding of citizen science’s impact on change and how this is influenced by design. We, therefore, suggest citizen science projects carefully consider the potential role of design and institutional funding when aiming for impactful citizen science projects as continuously claimed by the global community (A/RES/70/1; HLPF, 2018; UN Water, 2018). We suggest that researchers consider these findings in their water quality monitoring practice and also reflect how these findings apply in various contexts, including various regional settings and in a specific field of practice such as the monitoring of specific types of freshwater, marine water, soil, air, or biodiversity.
The raw data supporting the conclusions of this article will be made available by the authors, without undue reservation.
SK: Conceptualization, Methodology, Investigation, Formal analysis, Validation, Supervision, Writing–original draft; CB: Methodology, Investigation, Writing–review and editing; AG: Methodology, Investigation, Writing–review and editing; DK: Methodology, Formal analysis, Writing–review and editing; YL: Methodology, Investigation, Writing–review and editing; SL: Methodology, Investigation, Writing–review and editing; SN: Methodology, Investigation, Formal analysis, Visualization, Writing–review and editing. All authors contributed to the article and approved the submitted version.
The funding was provided by the United Nations University - Institute for Integrated Management of Material Fluxes and of Resources (UNU-FLORES), Dresden, Germany.
The authors thank interviewees and survey respondents for sharing their knowledge and experience related to the impacts of environmental citizen science projects. We also thank two reviewers and the editor for their constructive comments on earlier versions of this manuscript.
The authors declare that the research was conducted in the absence of any commercial or financial relationships that could be construed as a potential conflict of interest.
All claims expressed in this article are solely those of the authors and do not necessarily represent those of their affiliated organizations, or those of the publisher, the editors and the reviewers. Any product that may be evaluated in this article, or claim that may be made by its manufacturer, is not guaranteed or endorsed by the publisher.
The Supplementary Material for this article can be found online at: https://www.frontiersin.org/articles/10.3389/fenvs.2023.1186238/full#supplementary-material
A/RES/70/1 (2015). “Transforming our world: the 2030 Agenda for Sustainable Development,” in Resolution adopted by the general assembly on 25 september 2015 (A/RES/70/1).
Alender, B. (2016). Understanding volunteer motivations to participate in citizen science projects: a deeper look at water quality monitoring. J. Sci. Commun. 15 (3), A04. doi:10.22323/2.15030204
Aristeidou, M., Herodotou, C., Ballard, H. L., Young, A. N., Miller, A. E., Higgins, L., et al. (2021). Exploring the participation of young citizen scientists in scientific research: The case of iNaturalist. Plos one 16 (1), e0245682. doi:10.1371/journal.pone.0245682
Auerbach, J., Barthelmess, E. L., Cavalier, D., Cooper, C. B., Fenyk, H., Haklay, M., et al. (2019). The problem with delineating narrow criteria for citizen science. Proc. Natl. Acad. Sci. 116 (31), 15336–15337. doi:10.1073/pnas.1909278116
Backhaus, K., Erichson, B., Plinke, W., and Weiber, R. (2018). Multivariate analysemethoden. Eine anwendungsorientierte einführung 15, vollständig überarbeitete auflage. Berlin: Springer Gabler.
F. Berkes, C. Folke, and J. Colding (Editors) (2000). “Management Practices and Social Mechanisms for Building Resilience,” Linking social and ecological systems: Management practices and social mechanisms for building resilience (Cambridge: Cambridge University Press).
Bester, A. (2016). Results-based management in the united Nations development system: a report prepared for the united Nations department of economic and social affairs, for the quadrennial comprehensive policy review.
Breuer, L., Hiery, N., Kraft, P., Bach, M., Aubert, A. H., and Frede, H. G. (2015). HydroCrowd: a citizen science snapshot to assess the spatial control of nitrogen solutes in surface waters. Sci. Rep. 5, 16503. doi:10.1038/srep16503
Brosius, F. (2018). SPSS Umfassendes Handbuch zu Statistik und Datenanalyse 8. Frechen: Auflage. mitp.
Brouwer, S., van der Wielen, P. W., Schriks, M., Claassen, M., and Frijns, J. (2018). Public participation in science: the future and value of citizen science in the drinking water research. Water 10, 284. doi:10.3390/w10030284
Buytaert, W., Zulkafli, Z., Grainger, S., Acosta, L., Alemie, T. C., Bastiaensen, J., et al. (2014). Citizen science in hydrology and water resources: opportunities for knowledge generation, ecosystem service management, and sustainable development. Front. Earth Sci. 2, 26. doi:10.3389/feart.2014.00026
Carlson, T., and Cohen, A. (2018). Linking community-based monitoring to water policy: Perceptions of citizen scientists. J. Environ. Manag. 219, 168–177. doi:10.1016/j.jenvman.2018.04.077
Chase, S. K., and Levine, A. (2016). A framework for evaluating and designing citizen science programs for natural resources monitoring. Conserv. Biol. 30 (3), 456–466. doi:10.1111/cobi.12697
Chase, S. K., and Levine, A. (2018). Citizen science: exploring the potential of natural resource monitoring programs to influence environmental attitudes and behaviors. Conserv. Lett. 11 (2), e12382. doi:10.1111/conl.12382
Church, S. P., Payne, L. B., Peel, S., and Prokopy, L. S. (2019). Beyond water data: benefits to volunteers and to local water from a citizen science program. J. Environ. Plan. Manag. 62 (2), 306–326. doi:10.1080/09640568.2017.1415869
Colding, J., and Barthel, S. (2019). Exploring the social-ecological systems discourse 20 years later. Ecol. Soc. 24 (1), art2. doi:10.5751/es-10598-240102
Conrad, C. C., and Hilchey, K. G. (2011). A review of citizen science and community-based environmental monitoring: issues and opportunities. Environ. Monit. Assess. 176 (1-4), 273–291. doi:10.1007/s10661-010-1582-5
Crall, A. W., Newman, G. J., Stohlgren, T. J., Holfelder, K. A., Graham, J., and Waller, D. M. (2011). Assessing citizen science data quality: an invasive species case study. Conserv. Lett. 4 (6), 433–442. doi:10.1111/j.1755-263x.2011.00196.x
E/CN.3/2017/2 (2017). “Report of the Inter-Agency and Expert Group on Sustainable Development Goal Indicators (E/CN.3/2017/2),” in Annex III: revised list of global sustainable development goal indicators.
ECSA (European Citizen Science Association) (2015). Ten principles of citizen science. Berlin: Stockholm Environment Institute. doi:10.17605/OSF.IO/XPR2N
Eitzel, M., Cappadonna, J., Santos-Lang, C., Duerr, R., West, S. E., Virapongse, A., et al. (2017). Citizen science terminology matters: Exploring key terms. Citiz. Sci. Theory Pract. 2, 1–20. doi:10.5334/cstp.96
Fraisl, D., Campbell, J., See, L., Wehn, U., Wardlaw, J., Gold, M., et al. (2020). Mapping citizen science contributions to the UN sustainable development goals. Sustain. Sci. 15, 1735–1751. doi:10.1007/s11625-020-00833-7
Fraisl, D., Hager, G., Bedessem, B., Gold, M., Hsing, P. Y., Danielsen, F., et al. (2022). Citizen science in environmental and ecological sciences. Nat. Rev. Methods Prim. 2 (1), 64. doi:10.1038/s43586-022-00144-4
Fritz, S., See, L., Carlson, T., Haklay, M. M., Oliver, J. L., Fraisl, D., et al. (2019). Citizen science and the United Nations sustainable development goals. Nat. Sustain. 2 (10), 922–930. doi:10.1038/s41893-019-0390-3
Fritz, S., See, L. M., and Grey, F. (2022). The Grand Challenges Facing Environmental Citizen Science. Front. Environ. Sci. 10. doi:10.3389/fenvs.2022.1019628
Geoghegan, H., Dyke, A., Pateman, R., West, S., and Everett, G. (2016). “Understanding motivations for citizen science,” in Final report on behalf of UKEOF (York, UK: University of Reading, Stockholm Environment Institute (University of York) and University of the West of England).
Gharesifard, M., Wehn, U., and van der Zaag, P. (2019b). Context matters: a baseline analysis of contextual realities for two community-based monitoring initiatives of water and environment in Europe and Africa. J. Hydrology 579, 124144. doi:10.1016/j.jhydrol.2019.124144
Gharesifard, M., Wehn, U., and van der Zaag, P. (2019a). What influences the establishment and functioning of community-based monitoring initiatives of water and environment? A conceptual framework. J. Hydrology 579, 124033. doi:10.1016/j.jhydrol.2019.124033
Haklay, M. (2013). “Citizen science and volunteered geographic information – Overview and typology of participation,” in Crowdsourcing geographic knowledge: volunteered geographic information (VGI) in theory and practice. Editors D. Z. Sui, S. Elwood, and M. F. Goodchild, 105–122.
Haklay, M., Fraisl, D., Greshake Tzovaras, B., Hecker, S., Gold, M., Hager, G., et al. (2021b). Contours of citizen science: a vignette study. R. Soc. open Sci. 8 (8), 202108. doi:10.1098/rsos.202108
Haklay, M. M., Dörler, D., Heigl, F., Manzoni, M., Hecker, S., and Vohland, K. (2021a). “What is citizen science?,” in The challenges of definition in the science of citizen science (Cham: Springer), 13–33.
Haklay, M., Motion, A., Balázs, B., Kieslinger, B., Tzovaras, G., Bastian, , et al. (2020). ECSA's Characteristics of Citizen Science. Zenodo. doi:10.5281/zenodo.3758668
Hecker, S., Wicke, N., Haklay, M., and Bonn, A. (2019). How Does Policy Conceptualise Citizen Science? A Qualitative Content Analysis of International Policy Documents. Citiz. Sci. Theory Pract. 4 (1), 32. doi:10.5334/cstp.230
Heigl, F., Kieslinger, B., Paul, K. T., Uhlik, J., and Dörler, D. (2019). Toward an international definition of citizen science. Proc. Natl. Acad. Sci. 116 (17), 8089–8092. doi:10.1073/pnas.1903393116
Hicks, A., Barclay, J., Chilvers, J., Armijos, M. T., Oven, K., Simmons, P., et al. (2019). Global mapping of citizen science projects for disaster risk reduction. Front. Earth Sci. 7, 226. doi:10.3389/feart.2019.00226
HLPF (2018). 2018 HLPF Review of SDG implementation: SDG 6 – Ensure availability and sustainable management of water and sanitation for all. Available from: https://sustainabledevelopment.un.org/content/documents/195716.29_Formatted_2018_background_notes_SDG_6.pdf.
Jahn, S., Newig, J., Lang, D. J., Kahle, J., and Bergmann, M. (2022). Demarcating transdisciplinary research in sustainability science—Five clusters of research modes based on evidence from 59 research projects. Sustain. Dev. 30 (2), 343–357. doi:10.1002/sd.2278
Johnson, M. F., Hannah, C., Acton, L., Popovici, R., Karanth, K. K., and Weinthal, E. (2014). Network environmentalism: Citizen scientists as agents for environmental advocacy. Glob. Environ. Change 29, 235–245. doi:10.1016/j.gloenvcha.2014.10.006
Jollymore, A., Haines, M. J., Satterfield, T., and Johnson, M. S. (2017). Citizen science for water quality monitoring: Data implications of citizen perspectives. J. Environ. Manag. 200, 456–467. doi:10.1016/j.jenvman.2017.05.083
Kelly, R., Fleming, A., Pecl, G. T., von Gönner, J., and Bonn, A. (2020). Citizen science and marine conservation: a global review. Philosophical Trans. R. Soc. B 375 (1814), 20190461. doi:10.1098/rstb.2019.0461
Kirschke, S., Bennett, C., Ghazani, A. B., Franke, C., Kirschke, D., Lee, Y., et al. (2022). Citizen science projects in freshwater monitoring. From individual design to clusters? J. Environ. Manag. 309, 114714. doi:10.1016/j.jenvman.2022.114714
Kosmala, M., Wiggins, A., Swanson, A., and Simmons, B. (2016). Assessing data quality in citizen science. Front. Ecol. Environ. 14 (10), 551–560. doi:10.1002/fee.1436
Kullenberg, C., and Kasperowski, D. (2016). What is citizen science? A scientometric meta-analysis. PloS one 11 (1), e0147152. doi:10.1371/journal.pone.0147152
Land-Zandstra, A., Agnello, G., and Gültekin, Y. S. (2021). “Participants in citizen science,” in The science of citizen science 243, 259.
Lang, D. J., Wiek, A., Bergmann, M., Stauffacher, M., Martens, P., Moll, P., et al. (2012). Transdisciplinary research in sustainability science: practice, principles, and challenges. Sustain. Sci. 7, 25–43. doi:10.1007/s11625-011-0149-x
Larson, L. R., Cooper, C. B., Futch, S., Singh, D., Shipley, N. J., Dale, K., et al. (2020). The diverse motivations of citizen scientists: Does conservation emphasis grow as volunteer participation progresses? Biol. Conserv. 242, 108428. doi:10.1016/j.biocon.2020.108428
Lawrence, M. G., Williams, S., Nanz, P., and Renn, O. (2022). Characteristics, potentials, and challenges of transdisciplinary research. One Earth 5 (1), 44–61. doi:10.1016/j.oneear.2021.12.010
Le Coz, J., Patalano, A., Collins, D., Guillén, N. F., García, C. M., Smart, G. M., et al. (2016). Crowdsourced data for flood hydrology: Feedback from recent citizen science projects in Argentina, France and New Zealand. J. Hydrology 541, 766–777. doi:10.1016/j.jhydrol.2016.07.036
Lee, K. A., Lee, J. R., and Bell, P. (2020). A review of Citizen Science within the Earth Sciences: potential benefits and obstacles. Proc. Geologists' Assoc. 131 (6), 605–617. doi:10.1016/j.pgeola.2020.07.010
Lin Hunter, D. E., Newman, G. J., and Balgopal, M. M. (2023). What's in a name? The paradox of citizen science and community science. Front. Ecol. Environ. 21, 244–250. doi:10.1002/fee.2635
Lotfian, M., Ingensand, J., and Brovelli, M. A. (2020). A framework for classifying participant motivation that considers the typology of citizen science projects. ISPRS Int. J. Geo-Information 9 (12), 704. doi:10.3390/ijgi9120704
Lottig, N. R., Wagner, T., Henry, E. N., Cheruvelil, K. S., Webster, K. E., Downing, J. A., et al. (2014). Long-term citizen-collected data reveal geographical patterns and temporal trends in lake water clarity. PLoS One 9 (4), e95769. doi:10.1371/journal.pone.0095769
Lowry, C. S., and Fienen, M. N. (2013). CrowdHydrology: crowdsourcing hydrologic data and engaging citizen scientists. Groundwater 51 (1), 151–156. doi:10.1111/j.1745-6584.2012.00956.x
Mac Domhnaill, C., Lyons, S., and Nolan, A. (2020). The citizens in citizen science: demographic, socioeconomic, and health characteristics of biodiversity recorders in Ireland. Citiz. Sci. Theory Pract. 5 (1). doi:10.5334/cstp.283
MacPhail, V. J., and Colla, S. R. (2020). Power of the people: A review of citizen science programs for conservation. Biol. Conserv. 249, 108739. doi:10.1016/j.biocon.2020.108739
McKinley, D. C., Miller-Rushing, A. J., Ballard, H. L., Bonney, R., Brown, H., Cook-Patton, S. C., et al. (2017). Citizen science can improve conservation science, natural resource management, and environmental protection. Biol. Conserv. 208, 15–28. doi:10.1016/j.biocon.2016.05.015
MfN (2020). Our world – our goals: Citizen science for the sustainable development goals. Citizen science SDG conference declaration ‘knowledge for change. A decade of action of citizen science, 2020–2030. in support of the Sustainable Development Goals’. Available from: https://www.cs-sdg-conference.berlin/en/declaration.html.
Muenich, R., Peel, S., Bowling, L., Haas, M., Turco, R., Frankenberger, J., et al. (2016). The Wabash sampling blitz: a study on the effectiveness of citizen science. Citiz. Sci. Theory Pract. 1 (1), 3. doi:10.5334/cstp.1
Nath, S., and Kirschke, S. (2023). Groundwater Monitoring through Citizen Science: A Review of Project Designs and Results. Groundwater 61 (4), 481–493. doi:10.1111/gwat.13298
Newig, J., Jahn, S., Lang, D. J., Kahle, J., and Bergmann, M. (2019). Linking modes of research to their scientific and societal outcomes. Evidence from 81 sustainability-oriented research projects. Environ. Sci. Policy 101, 147–155. doi:10.1016/j.envsci.2019.08.008
Ngo, K. M., Altmann, C. S., and Klan, F. (2023). How the general public appraises contributory citizen science: Factors that affect participation. Citiz. Sci. Theory Pract. 8 (1), 3. doi:10.5334/cstp.502
Oliveira, S. S., Barros, B., Pereira, J. L., Santos, P. T., and Pereira, R. (2021). Social media use by citizen science projects: characterization and recommendations. Front. Environ. Sci. 9, 715319. doi:10.3389/fenvs.2021.715319
Oxford Dictionary (2020). Oxford Dictionary. Available at: https://en.oxforddictionaries.com/definition/citizen_science.
Pateman, R., Tuhkanen, H., and Cinderby, S. (2021). Citizen Science and the Sustainable Development Goals in Low and Middle Income Country Cities. Sustainability 13 (17), 9534. doi:10.3390/su13179534
Peter, M., Diekötter, T., Kremer, K., and Höffler, T. (2021). Citizen science project characteristics: Connection to participants’ gains in knowledge and skills. Plos one 16 (7), e0253692. doi:10.1371/journal.pone.0253692
Peter, M., Diekötter, T., and Kremer, K. (2019). Participant outcomes of biodiversity citizen science projects: a systematic literature review. Sustainability 11 (10), 2780. doi:10.3390/su11102780
Pocock, M. J., Roy, H. E., August, T., Kuria, A., Barasa, F., Bett, J., et al. (2019). Developing the global potential of citizen science: Assessing opportunities that benefit people, society and the environment in East Africa. J. Appl. Ecol. 56 (2), 274–281. doi:10.1111/1365-2664.13279
Quinlivan, L., Chapman, D. V., and Sullivan, T. (2020). Validating citizen science monitoring of ambient water quality for the United Nations sustainable development goals. Sci. Total Environ. 699, 134255. doi:10.1016/j.scitotenv.2019.134255
Ramírez, S. B., van Meerveld, I., and Seibert, J. (2023). Citizen science approaches for water quality measurements. Sci. Total Environ. 897, 165436. doi:10.1016/j.scitotenv.2023.165436
Ratnieks, F. L., Schrell, F., Sheppard, R. C., Brown, E., Bristow, O. E., and Garbuzov, M. (2016). Data reliability in citizen science: learning curve and the effects of training method, volunteer background and experience on identification accuracy of insects visiting ivy flowers. Methods Ecol. Evol. 7 (10), 1226–1235. doi:10.1111/2041-210x.12581
San Llorente Capdevila, A., Kokimova, A., Avellán, T., Kim, J., and Kirschke, S. (2020). Success factors for citizen science projects in water quality monitoring. Sci. Total Environ. 728, 137843. doi:10.1016/j.scitotenv.2020.137843
Sauermann, H., Vohland, K., Antoniou, V., Balázs, B., Göbel, C., Karatzas, K., et al. (2020). Citizen science and sustainability transitions. Res. Policy 49 (5), 103978. doi:10.1016/j.respol.2020.103978
Schade, S., Pelacho, M., van Noordwijk, T., Vohland, K., Hecker, S., and Manzoni, M. (2021). Citizen science and policy. Sci. Citiz. Sci., 351–371. doi:10.1007/978-3-030-58278-4_18
Schröter, M., Kraemer, R., Mantel, M., Kabisch, N., Hecker, S., Richter, A., et al. (2017). Citizen science for assessing ecosystem services: status, challenges and opportunities. Ecosyst. Serv. 28, 80–94. doi:10.1016/j.ecoser.2017.09.017
Shulla, K., Leal Filho, W., Sommer, J. H., Salvia, A. L., and Borgemeister, C. (2020). Channels of collaboration for citizen science and the sustainable development goals. J. Clean. Prod. 264, 121735. doi:10.1016/j.jclepro.2020.121735
Skarlatidou, A., and Haklay, M. (2021). Citizen science impact pathways for a positive contribution to public participation in science. J. Sci. Commun. 20 (06), A02. doi:10.22323/2.20060202
Somerwill, L., and Wehn, U. (2022). How to measure the impact of citizen science on environmental attitudes, behaviour and knowledge? A review of state-of-the-art approaches. Environ. Sci. Eur. 34 (1), 18–29. doi:10.1186/s12302-022-00596-1
Sprinks, J., Woods, S. M., Parkinson, S., Wehn, U., Joyce, H., Ceccaroni, L., et al. (2021). Coordinator perceptions when assessing the impact of citizen science towards sustainable development goals. Sustainability 13 (4), 2377. doi:10.3390/su13042377
Steffen, W., Persson, Å., Deutsch, L., Zalasiewicz, J., Williams, M., Richardson, K., et al. (2011). The Anthropocene: From global change to planetary stewardship. Ambio 40 (7), 739–761. doi:10.1007/s13280-011-0185-x
Steffen, W., Richardson, K., Rockström, J., Cornell, S. E., Fetzer, I., Bennett, E. M., et al. (2015). Sustainability. Planetary boundaries: guiding human development on a changing planet. Science 347 (6223), 1259855. doi:10.1126/science.1259855
Storey, R. G., Wright-Stow, A., Kin, E., Davies-Colley, R. J., and Stott, R. (2016). Volunteer stream monitoring: Do the data quality and monitoring experience support increased community involvement in freshwater decision making? Ecol. Soc. 21 (4), art32. doi:10.5751/es-08934-210432
Turbé, A., Barba, J., Pelacho, M., Mudgal, S., Robinson, L. D., Serrano-Sanz, F., et al. (2020). Correction: Understanding the Citizen Science Landscape for European Environmental Policy: An Assessment and Recommendations. Citiz. Sci. Theory Pract. 5 (1). doi:10.5334/cstp.308
UN Environment (2019). Assessment. Part A: state of the global environment. Available from: https://www.unenvironment.org/resources/assessment/part-state-global-environment (Accessed August 06, 2019).
UN Water (2018). Progress on ambient water quality. Piloting the monitoring methodology and initial findings for SDG indicator 6.3.2. Available from https://www.unwater.org/publications/progress-on-ambient-water-quality-632/.
United Nations Development Group (UNDG) (2011). Results-based management handbook. Harmonizing RBM concepts and approaches for improved development results at country level. New York, United States: UNDG.
van der Wal, R., Sharma, N., Mellish, C., Robinson, A., and Siddharthan, A. (2016). The role of automated feedback in training and retaining biological recorders for citizen science. Conserv. Biol. 30 (3), 550–561. doi:10.1111/cobi.12705
van Noordwijk, T., Bishop, I., Staunton-Lamb, S., Oldfield, A., Loiselle, S., Geoghegan, H., et al. (2021). Creating positive environmental impact through citizen science. Sci. Citiz. Sci., 373–395. doi:10.1007/978-3-030-58278-4_19
Wald, D. M., Longo, J., and Dobell, A. R. (2016). Design principles for engaging and retaining virtual citizen scientists. Conserv. Biol. 30 (3), 562–570. doi:10.1111/cobi.12627
Wehn, U., and Almomani, A. (2019). Incentives and barriers for participation in community-based environmental monitoring and information systems: A critical analysis and integration of the literature. Environ. Sci. Policy 101, 341–357. doi:10.1016/j.envsci.2019.09.002
Wehn, U., Gharesifard, M., Ceccaroni, L., Joyce, H., Ajates, R., Woods, S., et al. (2021). Impact assessment of citizen science: state of the art and guiding principles for a consolidated approach. Sustain. Sci. 16 (5), 1683–1699. doi:10.1007/s11625-021-00959-2
West, S. E., Pateman, R. M., and Dyke, A. (2021). Variations in the motivations of environmental citizen scientists. Citiz. Sci. Theory Pract. 6. doi:10.5334/cstp.370
Keywords: community-based monitoring, freshwater, impact assessment, regression analysis, SDG 6, voluntary monitoring
Citation: Kirschke S, Bennett C, Ghazani AB, Kirschke D, Lee Y, Loghmani Khouzani ST and Nath S (2023) Design impacts of citizen science. A comparative analysis of water monitoring projects. Front. Environ. Sci. 11:1186238. doi: 10.3389/fenvs.2023.1186238
Received: 14 March 2023; Accepted: 20 September 2023;
Published: 11 October 2023.
Edited by:
Erin Roger, Commonwealth Scientific and Industrial Research Organisation (CSIRO), AustraliaReviewed by:
Uta Wehn, University of Gothenburg, SwedenCopyright © 2023 Kirschke, Bennett, Ghazani, Kirschke, Lee, Loghmani Khouzani and Nath. This is an open-access article distributed under the terms of the Creative Commons Attribution License (CC BY). The use, distribution or reproduction in other forums is permitted, provided the original author(s) and the copyright owner(s) are credited and that the original publication in this journal is cited, in accordance with accepted academic practice. No use, distribution or reproduction is permitted which does not comply with these terms.
*Correspondence: Sabrina Kirschke, c2FicmluYS5raXJzY2hrZUBtZm4uYmVybGlu
Disclaimer: All claims expressed in this article are solely those of the authors and do not necessarily represent those of their affiliated organizations, or those of the publisher, the editors and the reviewers. Any product that may be evaluated in this article or claim that may be made by its manufacturer is not guaranteed or endorsed by the publisher.
Research integrity at Frontiers
Learn more about the work of our research integrity team to safeguard the quality of each article we publish.