- 1School of Accounting, Hebei Finance University, Baoding, China
- 2Department of Mathematics, Riphah International University (Lahore Campus), Lahore, Pakistan
- 3Department of Mathematics, College of Mathematical Sciences, Umm Al-Qura University, Makkah, Saudi Arabia
- 4College of Economics and Management, Hebei Agricultural University, Baoding, China
The digital green concept refers to the devotion to digital technology, i.e., techniques of procedures in the area of ecological or sustainable conservation. It contains leveraging digital techniques, procedures, and new tools to evaluate environmental problems and promote sustainable development. The major influence of this article is to evaluate the selection of the best digital green technology. For this, we aim to propose the idea of Maclaurin symmetric mean (MSM) operators based on interaction operational laws for T-spherical fuzzy (TSF) information, such as TSF interaction weighted averaging (TSFIWA), generalized TSF interaction weighted averaging (GTSFIWA), TSF interaction weighted geometric averaging (TSFIWGA), TSF interaction MSM (TSFIMSM), TSF interaction Bonferroni mean (TSFIBM), and TSF interaction weighted Maclaurin symmetric mean (TSFIWMSM) operators. Some dominant and reliable properties are also invented for evaluation. Moreover, to address the best digital green innovation (DGI) among the top five DGIs, we illustrate the procedure of the multi-attribute decision-making (MADM) technique under the presence of the derived operators. Finally, we demonstrate a numerical example for evaluating the comparative study between the proposed and existing or prevailing operators to enhance the worth of the derived theory.
1 Introduction
The term “digital green sustainable” describes how digital technology and procedures are used to support environmental sustainability and lower carbon emissions. It includes many facets of technology with an emphasis on reducing their environmental effect, including data centers, cloud computing, software applications, and gadgets. The goal of digital green sustainable practices is to maximize technology’s benefits while reducing its environmental harm. Organizations and people may help create a digital ecosystem that is more environmentally friendly and sustainable by using these practices. Moreover, to discover the best or finest decision from the collection of preferences, the theory of the multi-attribute decision-making (MADM) technique is very famous and reliable for evaluating the best pick from the array of choices, where the idea of the MADM approach is the sub-division of the decision-making procedure. However, in the presence of classical information, we faced a lot of complications because we have only two possibilities, such as 0 or 1, but not between unit intervals. Therefore, the theory of fuzzy set (FS) was proposed by Zadeh (1965), which described the membership whose values are contained in the unit interval. Furthermore, many scholars have raised the question of what would happen if we involved the falsity grade in the FS because the negative or falsity information is an initial and central part of every real-life problem. Therefore, Atanassov (1986) discovered the idea of intuitionistic FS (IFS). The IFS deals with yes or no types of theory, with the role that the sum of both degrees in the duplet should be contained in the unit interval. Furthermore, Yager (2013) improved or modified the theory of Pythagorean FS (PyFS). Moreover, Yager (2016) initiated the idea of q-rung orthopair FS (qROFS) with a valuable and robust condition such as
The most accurate way for an information alliance is an aggregate operator. Numerous writers have presented multiple aggregation operators over the past 10 years. The average mean (AM) operator is the most commonly used AO since it makes it simple to aggregate all the different data into a comprehensive form. Several practical AOs have also been developed that are useful for collecting data in ambiguous and complex fuzzy decision-making contexts, such as geometric mean (GM), Bonferroni mean (BM), and Heronian mean (HM). Other AM AOs for MADM have been created in past years. Any of the operators mentioned previously do not consider the relationships between the values being used. To resolve this issue, Yager developed the concept of power AO (Yager, 2001). Power AOs significantly impact the relationship of the data being aggregated. Maclaurin symmetric mean (MSM) operators in the aggregation theory are one of the research topics that have gained the most significant interest. The MSM was first proposed by Maclaurin (Jstor, 2022) and later made famous by Detemple and Robertson (Jstor, 2022). The relationship of numerous input arguments can be overcome by MSM, which defines its characteristics. The significant difference between MSM and BM is that MSM may represent interactions among more than input data arguments, but BM cannot. For the given statements, MSM monotonically decreases concerning the parameter value. MSM accepts the fact that opinions are transformed into crisp numbers.
However, Liu et al. (2020) investigated partitioned MSM operators for MADM applications within IFSs. Qin and Liu. (2014) introduced IF MSM (IFMSM) operators. In the technology domain, Wei and Lu. (2018) investigated the Pythagorean fuzzy MSM (PyFMSM) operators. By creating transactional PyFMSM operators for the applications of MADM, Yang and Pang. (2018) also enhanced the efficiency of PyFMSM operators. For the MADM, Wei et al. (2019) proposed the q-Rung orthopair fuzzy MSM (qROFMSM) operators. At the same time, Wang et al. (2019) enhanced the concept of the qROFMSM operator. MSM operations for linguistic variables were examined by Liu and Qin. (2017) using an IF layout, while Qin et al. (2015) introduced the MSM operators in hesitant fuzzy contexts. Further information and applications of MSM operators are shown in Wang et al. (2018); Yu et al. (2018); Ju et al. (2016); Ullah (2021); Ashraf et al. (2022).
In particular circumstances, the aggregating outcomes from IFS’s traditional operations are illogical, especially when there is zero NMD of the TSFS. Because operations of IFSs cannot consider interactions between truth and non-membership, He et al. (2014b) suggested the operational interaction laws of IFNs to solve this issue. When the NMD of IFNs is 0, the issues may be resolved, and these rules appropriately consider interactions between the membership and non-membership functions. Our proposed work is more appropriate than the that of existing operators because if any of the NMDs is 0, utilizing the existing operators affects all the other NMD values and gives zero aggregated value, whereas utilizing the proposed work zero does not affect the other ones. In our proposed work, we are using the TSFS as it is more useful than the other extensions of the FS because it gives independency to choose NMD by our own choice between
Green innovation” is the technological innovation that involves energy conservation, pollution prevention, waste recycling, green product design, or corporate environmental management. Digital technology is crucial for green innovation, and FS theory is typically the best option when it comes to assessing and modeling human knowledge during the process of digital green innovation (DGI). The purpose of this article is to discuss new developments in FS models and their use in DGI in engineering processes and practical accomplishments. Green innovation indicates all forms of invention that minimize green destruction and guarantee that biological supplies are utilized most effectively and efficiently as possible. A study on DGI can be seen in Yin et al. (2022); Yin and Yu (2022); Dong et al. (2023). Furthermore, fuzzy set theory is a valuable and dominant technique for modeling and evaluating human knowledge regarding DGI. Inspired by the aforementioned information, the major influence of this analysis is stated as follows:
1. To derive the theory of GTSFIWA, GTSFIA, TSFIWA, TSFIA, and TSFIWGA operators.
2. To discover the idea of TSFIMSM, TSFIBM, and TSFIWMSM operators.
3. To expose some valuable properties for the evaluated work.
4. To discuss the MADM technique under the presence of the derived operators.
5. To illustrate, a numerical example for evaluating the comparative study between proposed and existing or prevailing operators is applied to enhance the worth of the derived theory.
The rest of our work is divided into different sections. We primarily introduce the basic ideas of T-SFSs and interaction MSM operators in Section 2. We introduce the GTSFIWA, GTSFIA, TSFIWA, TSFIA, TSFIWGA, TSFIGA, and GTSFIWGA operators in Section 3. We defined the TSFIMSM and TSFIWMSM operators in Section 4 and described their properties. Using the proposed operators, we provide a new MADM technique in Section 5 and step-by-step instructions. In Section 6, we illustrate the effectiveness of the proposed MADM method and compare it with that of the recent techniques using a real-world application. The conclusions of this paper are presented in Section 7.
2 Preliminaries
Here, we aim to revise the theory of TSF information and its operational laws, such as algebraic and interaction laws, for TSF information. Throughout the paper,
Definition 1. (Ullah et al., 2018) A TSFS
With a valuable characteristic:
Under the presence of the aforementioned information, we derive the theory of neutral information
From now onward, the information in triplet
Definition 2. (Garg et al., 2018) Assume any two TSFVs be
Definition 3. (Naseem et al., 2022) Assume any two TSFVs be
Example 1. Let three TSFVs be
Definition 4. Let
The first two equations can be written in the respective ways shown in the following equations:
Example 2. Let three TSFVs be
3 Generalized TSF interaction aggregation operators
In the section on the development of green manufacturing engineering, we concentrate on utilizing the theory IMSM in the environment of TSF set theory, such as GTSFIWA, GTSFIA, TSFIWA, TSFIA, and TSFIWGA operators. Some dominant and reliable properties are also invented for evaluated work.
Definition 5. The GTSFIWA operator is particularized by
Theorem 1. Using the information in Eq. 16, we derive the following theory, such as
Proof: By using mathematical induction, we concentrate to derive the theory in Eq. 17. For this, we have the following procedure. When
Thus,
For
Then, we aim to derive it for
Hence, the theory in Eq. 17 is also valid for
Property 1. (Idempotency) Let
Furthermore, by setting the value of
Finally, for
Definition 6. A GTSFIWGA operator is particularized by
Theorem 2. Using the information in Eq. 22, we derive the following theory, such as
Proof: Omitted.
Example 3. Suppose five TSFVs be,
4 Interaction MSM operators for TSF information
In the section on the development of green manufacturing engineering, we concentrate on utilizing the IMSM theory in the environment of TSF set theory, such as TSFIMSM, TSFIBM, and TSFIWMSM operators. Some dominant and reliable properties are also invented for evaluated work.
Definition 7. (Maclaurin, 1729) Suppose
With various valuable properties, such as
Furthermore, we expose some special cases of the evaluated theory, such asWhile adding
While adding
While adding
While adding
Definition 8. A TSFIMSM operator is stated by
Furthermore, we derive that the following theory is valid, such as
Then, we obtain
According to the presence of the theory of the TSFIWA operator, we have
For
For
Then, we prove that the information in Eq. 35 is also valid for
Hence, we obtain our required result.
Theorem 3. Here, we derive the theory of the TSFIMSM operator in the presence of TSF information, such as
Proof: Consider
Then, we have
Therefore,
Property 4. When
Proof: Assume that
Property 5. Suppose
Proof: Using Theorem 6, the property of boundedness can be proven.Few specific cases of the
where
When
where
When every
Definition 9. A TSFIWMSM operator is particularized by
Theorem 5. Using the theory in Eq. 38, we derive the following information, such as
Example 4. Suppose

TABLE 4. Aggregated matrix of score values
5 Application in MADM technique
In this part, we describe the exact procedures for a novel MADM method using the suggested operators in a TSF environment. Suppose
Step 1: While calculating alternatives
where
Step 2: Add alternative ranking values
Step 3: Evaluate the score value of
Step 4: Utilizing the score function according to the technique in definition (3), rank
Step 5: The optimal alternative should be chosen after ranking the other options using
5.1 Application in digital green innovation
Green innovation is a process that involves developing green technologies, products, and processes as well as the accompanying companies, management, and systems. Digital innovation creates new possibilities for climate change mitigation and adaptation. Based on the Paris Agreement and the United Nations Framework Convention on Climate Change, a fundamental framework for the world’s response to climate change has been formed. Green innovation” is technological innovation that involves energy conservation, pollution prevention, waste recycling, green product design, or corporate environmental management. These innovations can significantly lessen the negative effects on the environment while also generating value for the company and its stakeholders. How to improve the green competitiveness and profit of enterprises in the environment of climate change is an important issue. In this case, we need to choose the most talented entrepreneurs from a large number of alternative projects to invest in. The criteria and methods presented in this paper are applicable to the selection of DGI investment projects.
Example 1: Pakistan has been listed as one of the top 10 nations in the world most impacted by climate change. The country’s environmental sustainability strategy is managed by the government. All people concerned must take responsibility for maintaining the ecosystem and its resources, both the governmental and private organizations and particular people. To encourage the development of ideas and solutions for some of the most serious issues we face, the National Incubation Center regularly hosts tech conferences and innovation challenges. It is an honor to have teamed up with Pak Mission Society to find solutions to issues related to climate change, given the situation of the environment at the moment. We will be asking Pakistan’s most talented entrepreneurs
Based on the theory, this study constructs a framework system for enterprises to choose DGI investment projects.
Solving the numerical example using decision-making steps is the best way to get the optimal solution.
Step 1: The TSFV is used to calculate the alternatives concerning the attributes, and the decision matrix
Step 2: The attribute weight vector is assumed to be. We utilize Eq. 26 to calculate each alternate collective evaluation value. In this case, we use
Figure 1 is the graphical representation of the TSFIWMSM operator using Table 6, which shows that when
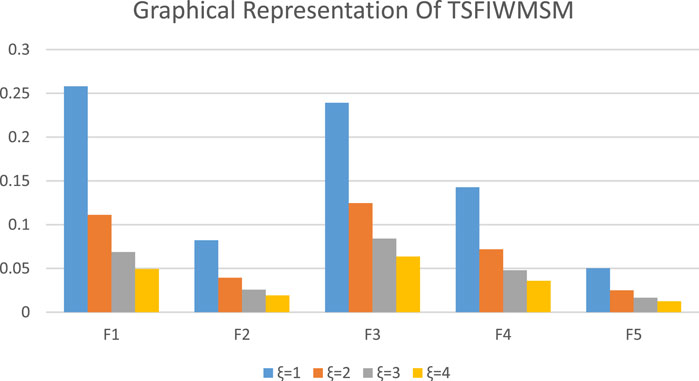
FIGURE 1. Demonstration of various
Figure 2 is the graphical representation of the TSFIMSM operator using Table 7, which shows that when
Step 3: Eq. 2 can be used to determine the scores
Step 4: According to the ranking of scores,
Step 5: Ranking of the alternatives would be
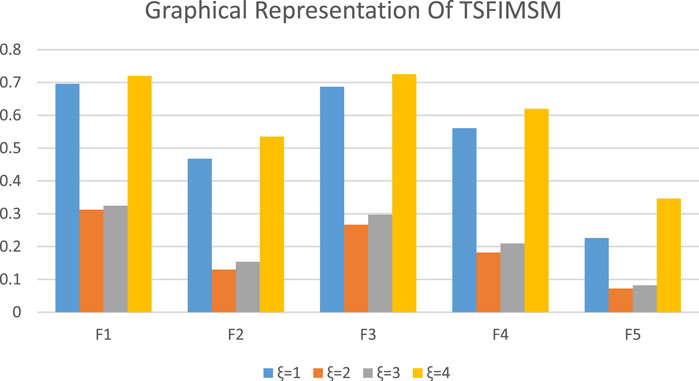
FIGURE 2. Graphical demonstration of various
5.2 Parameter sensitivity and comparative analysis
This section discusses the impact factor of various
5.3 Impact of the parameter
In the
Table 7 shows the outcomes of utilizing the
5.4 Sensitivity of the parameter
This section examines the sensitivity of the relevant parameter and how it affects the aggregation outcomes. To see the effects, we change the variable parameter and present the ranking outcomes in Table 11.
The TSFIMSM and TSFIWMSM operators also allow for the consideration of other
6 Comparison
The interrelation among membership, abstinence, and non-membership is not considered if operations of TSFVs in Definition 2 are utilized in the aggregation procedure. In Step 2, the aggregation operations mentioned previously are utilized, and the outcomes are shown in Table 12. It is clear from the outcomes that these differ from those of the TSFI operators, including the TSFIA, TSFIWA, TSFIGA, TSFIWGA, GTSFIA, and GTSFIWA operators, which are displayed in Table 12. With regards to the attribute
It is worth noticing that the results obtained using different operators are different. This is because of the nature of the operators and the parameters involved. For instance, the TSFIA operator has no associated weight vector, while the TSFIWA operator has a weight factor. Similar is the case with TSFIG and TSFWIG operators. However, the results of Tables 10–12 clearly show that the operators proposed in the paper have better results because of their advanced nature as the MSM operators proposed in Garg et al. (2022) have certain limitations which were discussed at the beginning of the paper. Furthermore, we have also compared the proposed work with Spearman’s rank correlation coefficient measures, which was proposed by Sedgwick [Sedgwick, P. (2014) Spearman’s rank correlation coefficient. Bmj, 349.] in 2014, which is very valuable and dominant measures based on classical set theory. For these measures, it is not possible to evaluate the proposed types of data because the arranged information is given in the shape of T-spherical fuzzy numbers, and the existing measure was computed based on the crisp set which is the special case of the proposed theory, but in the future, we aim to derive it for T-spherical fuzzy sets to improve the worth of the proposed theory.
Figure 3 is the graphical representation of the proposed and existing operators utilizing Table 12, making it easy to understand the optimal alternative. Figure 3 graphically shows that
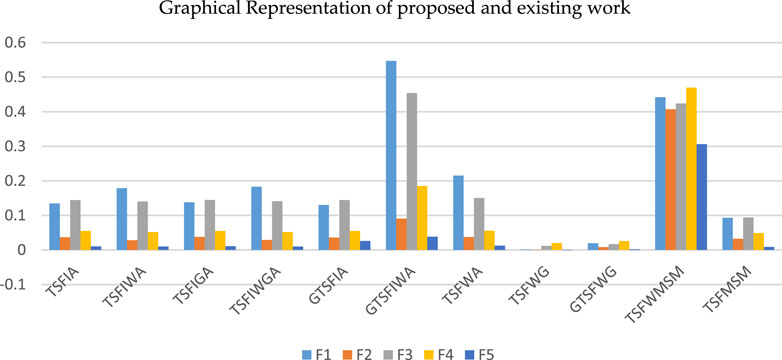
FIGURE 3. Graph illustrating the comparison between the proposed and existing operator utilizing the information in Table 12.
6.1 Advantages of the proposed operator
The following section will elaborate on the method’s superiority in dealing with situations of uncertainty by examining its properties in the context of some previous studies. As we all know, the traditional operator does not consider the interaction between MD, NMD, and AD with
Remark 1. The results obtained in Theorem 3 and Theorem 5 reduce to the framework of
1) SFSs if we place
2) PFSs if we place
3) qROFSs if we place
4) PyFSs if we place
5) IFSs if we place
7 Conclusion
In this section, we will learn about the following operators and techniques, such as
1. Based on the interaction operations for TSFVs, this research suggested several interaction MSM operators for TSFVs and generalized the MSM operator to TSFVs. After that, we talked about several of their desirable traits, like idempotency and commutativity.
2. An approach for the MADM problems was also proposed under the consideration of derived operators.
3. We further examined some particular examples of these operators and compared the presented results with those of many existing operators to justify the validity and supremacy of the stated approaches.
4. While comparing the current operators, we know that the proposed method is more extensive than several other existent methods.
The primary benefit is that they can capture the relationships between several flexible input arguments due to the parameter
Data availability statement
The original contributions presented in the study are included in the article/Supplementary Material; further inquiries can be directed to the corresponding authors.
Author contributions
All authors listed have made a substantial, direct, and intellectual contribution to the work and approved it for publication.
Acknowledgments
The authors would like to thank the Deanship of Scientific Research at Umm Al-Qura University for supporting this work by Grant Code: 22UQU4310396DSR66.
Conflict of interest
The authors declare that the research was conducted in the absence of any commercial or financial relationships that could be construed as a potential conflict of interest.
Publisher’s note
All claims expressed in this article are solely those of the authors and do not necessarily represent those of their affiliated organizations, or those of the publisher, the editors, and the reviewers. Any product that may be evaluated in this article, or claim that may be made by its manufacturer, is not guaranteed or endorsed by the publisher.
References
Akram, M., Ullah, K., and Pamucar, D. (2022). Performance evaluation of solar energy cells using the interval-valued T-spherical fuzzy Bonferroni mean operators. Energies 15, 292. doi:10.3390/en15010292
Ashraf, A., Ullah, K., Hussain, A., and Bari, M. (2022). Interval-valued picture fuzzy Maclaurin symmetric mean operator with application in multiple attribute decision-making. Rep. Mech. Eng. 3, 301–317. doi:10.31181/rme20020042022a
Atanassov, K. (1986). Intuitionistic fuzzy sets. Fuzzy Sets Syst. 20, 87–96. doi:10.1016/s0165-0114(86)80034-3
Chen, Y., Munir, M., Mahmood, T., Hussain, A., and Zeng, S. (2021). Some generalized T-spherical and group-generalized fuzzy geometric aggregation operators with application in MADM problems. J. Math. 2021, 1–17. doi:10.1155/2021/5578797
Cuong, B. C., and Kreinovich, V. (2014). Picture fuzzy sets. J. Comput. Sci. Cybern. 30, 409–420. doi:10.15625/1813-9663/30/4/5032
Dong, T., Yin, S., and Zhang, N. (2023). New energy-driven construction industry: Digital green innovation investment project selection of photovoltaic building materials enterprises using an integrated fuzzy decision approach. Systems 11, 11. doi:10.3390/systems11010011
Garg, H., Ahmad, A., Ullah, K., Mahmood, T., and Ali, Z. (2022). Algorithm for multiple attribute decision-making using T-spherical fuzzy Maclaurin symmetric mean operator. Iran. J. Fuzzy Syst. 19, 111–124. doi:10.22111/ijfs.2022.7215
Garg, H., Munir, M., Ullah, K., Mahmood, T., and Jan, N. (2018). Algorithm for T-spherical fuzzy multi-attribute decision making based on improved interactive aggregation operators. Symmetry 10, 670. doi:10.3390/sym10120670
Hussain, A., Ullah, K., Yang, M.-S., and Pamucar, D. (2022). Aczel-alsina aggregation operators on T-spherical fuzzy (TSF) information with application to TSF multi-attribute decision making. IEEE Access 10, 26011–26023. doi:10.1109/access.2022.3156764
Jstor 2022 ON generalized symmetric means of two variables on jstor. Available at:https://www.jstor.org/stable/43668126 (Accessed July 26, 2022).
Ju, Y., Liu, X., and Ju, D. (2016). Some new intuitionistic linguistic aggregation operators based on Maclaurin symmetric mean and their applications to multiple attribute group decision making. Soft Comput. 20, 4521–4548. doi:10.1007/s00500-015-1761-y
Karaaslan, F., and Al-Husseinawi, A. H. S. (2022). Hesitant T-spherical Dombi fuzzy aggregation operators and their applications in multiple criteria group decision-making. Complex & Intelligent Syst. 8, 3279–3297. doi:10.1007/s40747-022-00669-x
Karaaslan, F., and Dawood, M. A. D. (2021). Complex T-spherical fuzzy Dombi aggregation operators and their applications in multiple-criteria decision-making. Complex Intell. Syst. 7, 2711–2734. doi:10.1007/s40747-021-00446-2
Khan, M. R., Ullah, K., Pamucar, D., and Bari, M. (2022). Performance measure using a multi-attribute decision-making approach based on complex T-spherical fuzzy power aggregation operators. J. Comput. Cognitive Eng. 1 (3), 138–146. doi:10.47852/bonviewJCCE696205514
Liu, P., Chen, S.-M., and Wang, Y. (2020). Multiattribute group decision making based on intuitionistic fuzzy partitioned Maclaurin symmetric mean operators. Inf. Sci. 512, 830–854. doi:10.1016/j.ins.2019.10.013
Liu, P., and Qin, X. (2017). Maclaurin symmetric mean operators of linguistic intuitionistic fuzzy numbers and their application to multiple-attribute decision-making. J. Exp. Theor. Artif. Intell. 29, 1173–1202. doi:10.1080/0952813x.2017.1310309
Maclaurin, C. A. (1729). Second letter to martin folkes, esq.; concerning the roots of equations, with demonstration of other rules of algebra. Philos. Trans. R. Soc. Lond. Ser. A 1729, 59–96. doi:10.1098/rstl.1729.0011
Mahmood, T., and Ali, Z. (2020). Aggregation operators and VIKOR method based on complex Q-rung orthopair uncertain linguistic informations and their applications in multi-attribute decision making. Comp. Appl. Math. 39, 306. doi:10.1007/s40314-020-01332-2
Mahmood, T. A. (2020). Novel approach towards bipolar soft sets and their applications. J. Math. 2020, 4690808. doi:10.1155/2020/4690808
Mahmood, T., Ullah, K., Khan, Q., and Jan, N. (2019). An approach toward decision-making and medical diagnosis problems using the concept of spherical fuzzy sets. Neural Comput. Appl. 31, 7041–7053. doi:10.1007/s00521-018-3521-2
Naseem, A., Ullah, K., Akram, M., Božanić, D., and Ćirović, G. (2022). Assessment of smart grid systems for electricity using power Maclaurin symmetric mean operators based on T-spherical fuzzy information. Energies 15, 7826. doi:10.3390/en15217826
Özlü, Ş., and Karaaslan, F. (2022). Correlation coefficient of T-spherical type-2 hesitant fuzzy sets and their applications in clustering analysis. J. Ambient Intell. Humaniz. Comput. 13, 329–357. doi:10.1007/s12652-021-02904-8
Qin, J., and Liu, X. (2014). An approach to intuitionistic fuzzy multiple attribute decision making based on Maclaurin symmetric mean operators. J. Intelligent Fuzzy Syst. 27, 2177–2190. doi:10.3233/IFS-141182
Qin, J., Liu, X., and Pedrycz, W. (2015). Hesitant fuzzy Maclaurin symmetric mean operators and its application to multiple-attribute decision making. Int. J. Fuzzy Syst. 17, 509–520. doi:10.1007/s40815-015-0049-9
Ullah, K., Mahmood, T., and Jan, N. (2018). Similarity measures for T-spherical fuzzy sets with applications in pattern recognition. Symmetry 10, 193. doi:10.3390/sym10060193
Ullah, K. (2021). Picture fuzzy Maclaurin symmetric mean operators and their applications in solving multiattribute decision-making problems. Math. Problems Eng. 2021, 1–13. doi:10.1155/2021/1098631
Wang, J., Wei, G., Wang, R., Alsaadi, F. E., Hayat, T., Wei, C., et al. (2019). Some Q-rung interval-valued orthopair fuzzy Maclaurin symmetric mean operators and their applications to multiple attribute group decision making. Int. J. Intelligent Syst. 34, 2769–2806. doi:10.1002/int.22156
Wang, J., Yang, Y., and Li, L. (2018). Multi-criteria decision-making method based on single-valued neutrosophic linguistic Maclaurin symmetric mean operators. Neural Comput. Appl. 30, 1529–1547. doi:10.1007/s00521-016-2747-0
Wei, G., and Lu, M. (2018). Pythagorean fuzzy Maclaurin symmetric mean operators in multiple attribute decision making. Int. J. Intelligent Syst. 33, 1043–1070. doi:10.1002/int.21911
Wei, G., Wei, C., Wang, J., Gao, H., and Wei, Y. (2019). Some Q-rung orthopair fuzzy Maclaurin symmetric mean operators and their applications to potential evaluation of emerging technology commercialization. Int. J. Intelligent Syst. 34, 50–81. doi:10.1002/int.22042
Yager, R. R. (2016). Generalized orthopair fuzzy sets. IEEE Trans. Fuzzy Syst. 25, 1222–1230. doi:10.1109/tfuzz.2016.2604005
Yager, R. R. (2013). “Pythagorean fuzzy subsets,” in Proceedings of the 2013 Joint IFSA World Congress and NAFIPS Annual Meeting (IFSA/NAFIPS), Edmonton, AB, June 24-28, 2013, 57–61.
Yager, R. R. (2001). The power average operator. IEEE Trans. Syst. Man, Cybern. - Part A Syst. Humans 31, 724–731. doi:10.1109/3468.983429
Yang, W., and Pang, Y. (2018). New pythagorean fuzzy interaction Maclaurin symmetric mean operators and their application in multiple attribute decision making. IEEE Access 6, 39241–39260. doi:10.1109/ACCESS.2018.2856270
Yin, S., and Yu, Y. (2022). An adoption-implementation framework of digital green knowledge to improve the performance of digital green innovation practices for industry 5.0. J. Clean. Prod. 363, 132608. doi:10.1016/j.jclepro.2022.132608
Yin, S., Zhang, N., Ullah, K., and Gao, S. (2022). Enhancing digital innovation for the sustainable transformation of manufacturing industry: A pressure-state-response system framework to perceptions of digital green innovation and its performance for green and intelligent manufacturing. Systems 10, 72. doi:10.3390/systems10030072
Yu, S.-M., Zhang, H., and Wang, J. (2018). Hesitant fuzzy linguistic Maclaurin symmetric mean operators and their applications to multi-criteria decision-making problem. Int. J. Intelligent Syst. 33, 953–982. doi:10.1002/int.21907
Keywords: T-spherical fuzzy set, Maclaurin symmetric mean, interactive Maclaurin symmetric mean operators, digital green innovation, decision-making
Citation: Hou X, Naseem A, Ullah K, Naeem M, Akram M and Yin S (2023) Identification and classification of digital green innovation based on interaction Maclaurin symmetric mean operators by using T-spherical fuzzy information. Front. Environ. Sci. 11:1164703. doi: 10.3389/fenvs.2023.1164703
Received: 13 February 2023; Accepted: 03 July 2023;
Published: 22 August 2023.
Edited by:
Željko Stević, University of East Sarajevo, Bosnia and HerzegovinaReviewed by:
Faruk Karaaslan, Cankiri Karatekin University, TürkiyeNaeem Jan, Korea National University of Transportation, Republic of Korea
Said Broumi, University of Hassan II Casablanca, Morocco
Copyright © 2023 Hou, Naseem, Ullah, Naeem, Akram and Yin. This is an open-access article distributed under the terms of the Creative Commons Attribution License (CC BY). The use, distribution or reproduction in other forums is permitted, provided the original author(s) and the copyright owner(s) are credited and that the original publication in this journal is cited, in accordance with accepted academic practice. No use, distribution or reproduction is permitted which does not comply with these terms.
*Correspondence: Kifayat Ullah, a2lmYXlhdC5raGFuLmRyQGdtYWlsLmNvbQ==; Muhammad Naeem, bWZhcmlkb29uQHVxdS5lZHUuc2E=