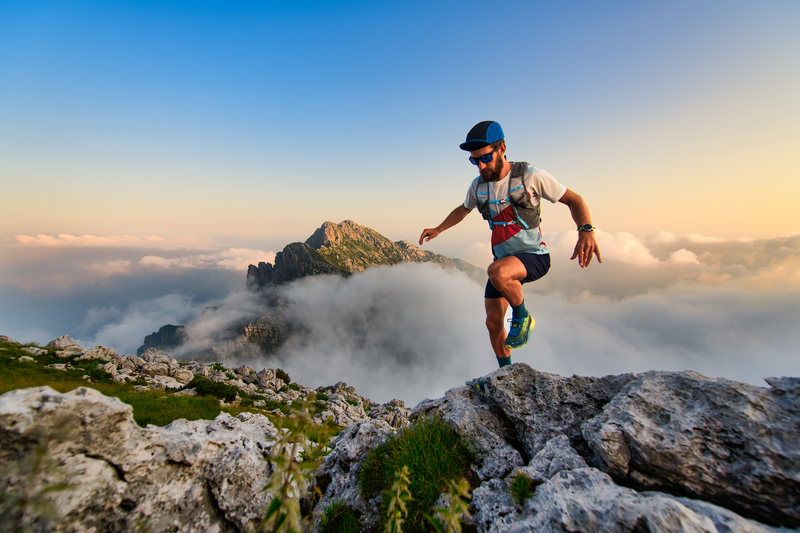
94% of researchers rate our articles as excellent or good
Learn more about the work of our research integrity team to safeguard the quality of each article we publish.
Find out more
PERSPECTIVE article
Front. Environ. Sci. , 25 January 2023
Sec. Conservation and Restoration Ecology
Volume 11 - 2023 | https://doi.org/10.3389/fenvs.2023.1119094
Impact or risk assessments of alien species can use qualitative criteria (such as verbally described categories) or quantitative criteria (numerically defined threshold values of empirically measurable quantities). According to a common misconception, the use of qualitative criteria in invasion biology is justified by uncertainty in the available data. Yet qualitative criteria have the effect of increasing uncertainty. In contrast, assessments using quantitative criteria are testable, transparent, highly repeatable and comparable. Most of these characteristics do not even depend on the availability of numerical data. Although quantitative criteria do not necessarily make assessments correct, they do make them correctable, which is the benchmark of science.
Ecological risk assessments can be carried out using quantitative or qualitative criteria. IUCN’s (2012) Red List criteria are an example of a quantitative set of criteria. A species may for instance be listed as endangered (EN) or vulnerable (VU), depending on whether its area of occupancy (AOO) is below or above 500 km2.
In contrast, many assessment schemes addressing the ecological impact of alien (invasive) species are still based on qualitative sets of criteria (for an overview, see e.g. Roy et al., 2018). However, there is no obvious reason why red-listing of threatened species and impact assessments of alien species should follow different scientific standards, and the need for quantitative sets of criteria for alien species assessments has been stated before (e.g. Lodge et al., 2006; Suedel et al., 2007). I would here like to reinforce this conviction by clarifying the advantages of, and refuting some worries about, quantitative criteria. For example, it has been stated that “the lack of [numerical] data” and “challenges in interpretation and communication” constitute “Major hurdles preventing the use of [...] quantitative risk assessment method[s]” (BNZ, 2006:26; Roy et al., 2018:528). However, such statements confound quantitative criteria with the quantitative nature of the data used.
An assessment criterion is here regarded as quantitative if and only if it provides a numerically defined threshold value of an empirically measurable quantity for each assessment category. In the Red List example, according to criterion B2, an AOO of 500 km2 is the threshold value distinguishing species in the EN category from species in the VU category (IUCN, 2012). Because such threshold values are defined for all assessment categories (CR, EN, VU, NT, LC) for all assessment criteria (A–E) and sub-criteria, the Red List constitutes a fully quantitative set of criteria.
A set of criteria that does not meet this definition is here referred to as qualitative. This obviously includes cases where the assessment categories are described using a scale of subjective terms such as ‘low’, ‘limited’, ‘moderate’, ‘medium’, ‘high’, ‘severe’, without any translation into numerical thresholds.
Sometimes, ‘semi-quantitative’ sets of criteria are said to occupy a middle-ground. From the perspective taken here, they do not represent a separate category, however. They are either a mixture of quantitative and qualitative criteria; or they use categories that are referred to by numbers (scores) but defined verbally.
It is thus important to understand that the use of numerical scores does not make a criterion quantitative: Measurement theory distinguishes between different scale types, for which different operations are admissible (Houle et al., 2011). For instance, an AOO of 300 km2 is three times larger than an AOO 100 km2, but a score of three does not need to be three times as substantial as a score of 1. Ignoring these differences can lead to erroneous or meaningless inferences (Wolman, 2006).
Conversely, a set of criteria can be quantitative even though it uses verbal descriptors for its categories, as long as the underlying thresholds are numeric. For instance, referring to ecological effects as ‘local’ or ‘large-scale’, while defining the threshold between these terms as affecting “less than” or “at least 5% of the population size or AOO”, respectively (Sandvik et al., 2019), is fully compatible with a quantitative set of criteria.
Yes/no questions may or may not be quantitative criteria. “Is the species a parasite,” may be regarded as quantitative, because the answer can be established empirically. The question whether a species has “prolific seed production” is quantitative only if a numerical definition of ‘prolific’ is provided (e.g. 1,000 seeds m−2 a−1; Gordon et al., 2010).
Risk assessments, as all other scientific activities, are imbued with uncertainty. Uncertainty may be epistemic (e.g. natural variation, observation error, measurement error, estimation error) and semantic (e.g. linguistic vagueness) in its nature (Akçakaya et al., 2000; Regan et al., 2002). Although uncertainty in risk assessments of alien species has received quite some attention (McGeoch et al., 2012; Latombe et al., 2019; Clarke et al., 2021), and even though some of these studies acknowledge that uncertainty is partly due to criterion delimitations being “a matter of degree” (Clarke et al., 2021, p. 13), the nature of the criteria has been conspicuous by its absence from recommendations for reducing uncertainty. However, quantitative criteria take an obvious step towards reducing semantic uncertainty, i.e. towards reducing the scope of potential interpretations that can be given to the questions posed, definitions used, and criteria applied. This is an important gain, even though the epistemic portion of uncertainty is not affected by the nature of the criteria.
This gain is visualized in Figure 1: epistemic uncertainty about the expansion speed (Sandvik, 2020) of three alien species is illustrated using the lengths of ellipses, while semantic uncertainty is symbolized by the borders between categories (scores). In a quantitative set of criteria (Figure 1A), some species will unambiguously fit into a certain category (A and B), while the category may be uncertain for others (C). In other words, although uncertainty is present in all three cases, it only affects the classification of species C. If one chooses a qualitative set of criteria instead (Figure 1B), species A may still fit into one score and C will be uncertain; in addition, however, it has become unclear whether species B should be scored as 2 or 3 (or even 1). As can be seen from Figure 1A, this uncertainty is not due to poor data; it is due to fuzzy thresholds, i.e., scores allowing different interpretations. Some experts may even argue, based on Figure 1B, that C fits perfectly into score 4. In the latter case, qualitative criteria, by increasing semantic uncertainty, result in an underestimate of the epistemic uncertainty.
FIGURE 1. Concept figure illustrating three alien species in an (A) quantitative and a (B) qualitative set of criteria. Species are assigned to one of four scores based either (A) on the estimated numerical value of their expansion speed (in square brackets, meters per year) or (B) on expert judgment. The length of the ellipses illustrates epistemic uncertainty (e.g. natural variation and measurement error), whereas semantic uncertainty is illustrated by the (A) sharpness or (B) fuzziness of the thresholds between scores.
A quantitative set of criteria is at its best when it can be combined with quantitative data, i.e. when numerical estimates are available for the parameters underlying the criteria. However, this is a not a prerequisite for using quantitative criteria. If good data are absent, estimates have to be replaced by ‘expert judgments’, based on the personal scientific expertise of specialists. As expert judgments are often associated with qualitative criteria, it is important to point out that expert judgments are fully compatible with a quantitative set of criteria, provided that the judgments are based on the threshold values. This means that the experts do not need to produce a numerical point estimate; it suffices that they substantiate that the true parameter value is likely to lie between two specified threshold values.
For example, an expert judgment is based on the threshold values of Figure 1A when the expert assesses an alien species to have an expansion speed between 50 and 160 m per year, even if no specific expansion speed (e.g. 144 m a−1) has been estimated. Although this judgment is subjective and may turn out to be erroneous, it has an important advantage over a qualitative one: the result is testable. The search for more knowledge can thus concentrate on the question: are there any empirical findings suggesting that the species may have an expansion speed of less than 50 or more than 160 m per year?
It is comparatively easy to envisage quantitative criteria for aspects of invasiveness, such as expansion speed or likelihood of establishment (Sandvik et al., 2019). However, even ecological effects, such as interactions with native species, can be quantified if expressed e.g. in terms of the reduction in population growth rate, carrying capacity or AOO (Laska and Wootton, 1998). It is true that such quantification requires extensive long-term study (Doak et al., 2008; Novak and Wootton, 2008) and is thus difficult in practice. However, quantitative criteria do not presuppose that numerical data are actually available, but only that it is possible in principle to obtain such data and that the effect criteria, therefore, can be defined numerically. For example, defining a ‘local impact’ as “affecting less than 5% of the population size” provides the expert with a clear guideline for her judgment. For many species, it will be unknown whether more or less than 5% of the population are actually affected. However, the precisely defined threshold permits an unambiguous understanding of the criterion in question.
Figure 2 shows the nature of the results obtained, given a certain set of criteria and a certain type of data. A quantitative set of criteria does not guarantee that the results are repeatable (for this to be the case, the additional requirement of numerical data needs to be fulfilled). However, it does guarantee that the results are testable. A qualitative set of criteria can neither guarantee repeatability nor testability—not even when numerical data are available. In combination with numerical data, the quantitative set of criteria even allows a quantification of the uncertainty (see Figure 1A), an option that does not exist in the qualitative alternative.
FIGURE 2. The nature of the results obtained (repeatable and/or testable), given a certain set of criteria (quantitative or qualitative) and a certain type of data (numerical or expert judgment).
Preferring a qualitative set of criteria because numerical estimates are not always available, essentially amounts to maintaining (in terms of Figure 2): “When results can have low repeatability, they do not need to be testable either.” Which is equivalent to stating (in terms of Figure 1): “When the epistemic uncertainty is large, we can just as well increase the semantic uncertainty.” However, there is no conceivable reason to accept such reasoning. All means of reducing uncertainty ought to be welcome.
The advantages of quantitative over qualitative sets of criteria may be summarized as increasing testability, repeatability, comparability, and transparency.
1) Testability.—An assessment is testable if there are objective criteria for deciding whether it is compatible with or contradicted by empirical findings. If, for example, a species has been classified as endangered based on its AOO, it is obvious what kind of information would be required to change this conclusion: if novel findings indicate that the AOO is actually larger than 500 km2, the previous assessment has been refuted. In contrast, if the AOO of a species is qualitatively assessed to be ‘small’, it is far from obvious what kind of information would be needed to change or refute this supposition.
2) Repeatability.—An assessment is repeatable if different experts would have ended up with the same result, given that the same information was available to them. For example, different experts will agree that a species should be classified as endangered, given that the best estimate of its AOO is 100 km2. In contrast, different experts will not necessarily agree whether an AOO of 100 km2 qualifies as ‘limited’ or ‘moderate’, simply because different experts can have different understandings of such terms (Burgman, 2001). To be sure, repeatability is a matter of degree, since no quantitative method will ever reach a repeatability of strictly 100%, whereas methods are available for improving the repeatability of qualitative assessments (Burgman, 2001; McBride et al., 2012).
3) Comparability.—The interpretation of qualitative criteria is always affected by the reference framework of the experts carrying out the assessments (Tversky and Kahneman, 1974; Martin et al., 2012). What an expert means by saying that the expansion speed of an alien species is ‘moderate’, may for instance depend on whether she is an expert on birds or on snails. Methods to increase repeatability do not necessarily increase comparability, because reaching a consensus among e.g. bird experts is one thing (inter-subjectivity), whereas reaching consensus across bird and snail experts would be a rather different matter (objectivity).
4) Transparency.—The result of a quantitative assessment is transparent for all scientists and end users. In other words, it is obvious how the scores of each criterion were derived from the underlying data, and how the final category is derived from the scores. Likewise, it is possible to test whether and how specific assumptions (e.g. regarding detection rate; Christy et al., 2010; Sandvik, 2020) affect the assessment.
Of course, qualitative criteria are not necessarily characterized by the total absence of testability, repeatability, comparability, and transparency. The crucial point is that, other things being equal, quantitative criteria are more testable, repeatable, comparable, and transparent than qualitative ones. This plea for quantitative risk assessments may be summarized in two propositions.
• The advantage of a quantitative over a qualitative set of criteria is not that the risk assessment becomes certain, but that, by reducing one specific source of uncertainty, it becomes testable.
• The advantage of a quantitative over a qualitative set of criteria is not necessarily that the risk assessment becomes more correct, but that it becomes more correctable.
Testability is the benchmark of science (Popper, 1934). In conservation biology, Mace and Lande (1991) introduced testability to Red List assessments. It is about time to apply the same scientific standards to impact assessments of alien species. This does not necessarily require large changes. For example, EICAT’s (Hawkins et al., 2015) and SEICAT’s (Bacher et al., 2018) criteria might be turned into quantitative ones by providing numerical definitions of key terms such as ‘decline in population size’ (e.g. “a reduction of at least 15% in the population size of at least 1 native species over a 10-year period”; cf. Sandvik et al., 2019, p. 2806) or ‘irreversibility’ (e.g. a reversion requiring more than x US$ or y years). Several fully (Sandvik et al., 2019) and partially (e.g. Baker et al., 2005; Gordon et al., 2010; D’hont et al., 2015; USDA, 2019) quantitative sets of criteria are already available, and it is to be hoped for that other assessment schemes will follow by defining numerical threshold values for their criteria.
The original contributions presented in the study are included in the article/supplementary material, further inquiries can be directed to the corresponding author.
HS conceived of and wrote the article.
This work was supported by the Norwegian Biodiversity Information Centre (Artsdatabanken) and the Research Council of Norway through its Centres of Excellence funding scheme (project number 223257).
I gladly acknowledge my indebtedness to Karl Popper’s critical rationalism.
The author declares that the research was conducted in the absence of any commercial or financial relationships that could be construed as a potential conflict of interest.
All claims expressed in this article are solely those of the authors and do not necessarily represent those of their affiliated organizations, or those of the publisher, the editors and the reviewers. Any product that may be evaluated in this article, or claim that may be made by its manufacturer, is not guaranteed or endorsed by the publisher.
Akçakaya, H. R., Ferson, S., Burgman, M. A., Keith, D. A., Mace, G. M., and Todd, C. R. (2000). Making consistent IUCN classifications under uncertainty. Conserv. Biol. 14, 1001–1013. doi:10.1046/j.1523-1739.2000.99125.x
Bacher, S., Blackburn, T. M., Essl, F., Genovesi, P., Heikkilä, J., Jeschke, J. M., et al. (2018). Socio-economic impact classification of alien taxa (SEICAT). Methods Ecol. Evol. 9, 159–168. doi:10.1111/2041-210X.12844
Baker, R., Hulme, P., Copp, G. H., Thomas, M., Black, R., and Haysom, K. (2005). UK non-native organism risk assessment scheme user manual: Version 3.3. York: Great Britain Non-native Species Secretariat.
Burgman, M. A. (2001). Flaws in subjective assessments of ecological risks and means for correcting them. Aust. J. Environ. Manag. 8, 219–226. doi:10.1080/14486563.2001.10648532
Christy, M. T., Yackel Adams, A. A., Rodda, G. H., Savidge, J. A., and Tyrrell, C. L. (2010). Modelling detection probabilities to evaluate management and control tools for an invasive species. J. Appl. Ecol. 47, 106–113. doi:10.1111/j.1365-2664.2009.01753.x
Clarke, D. A., Palmer, D. J., McGrannachan, C., Burgess, T. I., Chown, S. L., Clarke, R. H., et al. (2021). Options for reducing uncertainty in impact classification for alien species. Ecosphere 12, e03461. doi:10.1002/ecs2.3461
D'hont, B., Vanderhoeven, S., Roelandt, S., Mayer, F., Versteirt, V., Adriaens, T., et al. (2015). Harmonia+ and Pandora+: Risk screening tools for potentially invasive plants, animals and their pathogens. Biol. Invasions 17, 1869–1883. doi:10.1007/s10530-015-0843-1
Doak, D. F., Estes, J. A., Halpern, B. S., Jacob, U., Lindberg, D. R., Lovvorn, J., et al. (2008). Understanding and predicting ecological dynamics: Are major surprises inevitable? Ecology 89, 952–961. doi:10.1890/07-0965.1
Gordon, D. R., Mitterdorfer, B., Pheloung, P. C., Ansari, S., Buddenhagen, C., Chimera, C., et al. (2010). Guidance for addressing the Australian weed risk assessment questions. Plant Prot. Q. 25, 56–74.
Hawkins, C. L., Bacher, S., Essl, F., Hulme, P. E., Jeschke, J. M., Kühn, I., et al. (2015). Framework and guidelines for implementing the proposed IUCN environmental impact classification for alien taxa (EICAT). Divers. Distrib. 21, 1360–1363. doi:10.1111/ddi.12379
Houle, D., Pélabon, C., Wagner, G. P., and Hansen, T. F. (2011). Measurement and meaning in biology. Q. Rev. Biol. 86, 3–34. doi:10.1086/658408
Laska, M. S., and Wootton, J. T. (1998). Theoretical concepts and empirical approaches to measuring interaction strength. Ecology 79, 461–476. doi:10.1890/0012-9658(1998)079[0461:TCAEAT]2.0.CO;2
Latombe, G., Canavan, S., Hirsch, H., Hui, C., Kumschick, S., Nsikani, M. M., et al. (2019). A four-component classification of uncertainties in biological invasions: Implications for management. Ecosphere 10, e02669. doi:10.1002/ecs2.2669
Lodge, D. M., Williams, S., MacIsaac, H. J., Hayes, K. R., Leung, B., Reichard, S., et al. (2006). Biological invasions: Recommendations for U.S. policy and management. Ecol. Appl. 16, 2035–2054. doi:10.1890/1051-0761(2006)016[2035:BIRFUP]2.0.CO;2
Mace, G. M., and Lande, R. (1991). Assessing extinction threats: Toward a reevaluation of IUCN threatened species categories. Conserv. Biol. 5, 148–157. doi:10.1111/j.1523-1739.1991.tb00119.x
Martin, T. G., Burgman, M. A., Fidler, F., Kuhnert, P. M., Low-Choy, S., McBride, M., et al. (2012). Eliciting expert knowledge in conservation science. Conserv. Biol. 26, 29–38. doi:10.1111/j.1523-1739.2011.01806.x
McBride, M. F., Garnett, S. T., Szabo, J. K., Burbidge, A. H., Butchart, S. H. M., Christidis, L., et al. (2012). Structured elicitation of expert judgments for threatened species assessment: A case study on a continental scale using email. Methods Ecol. Evol. 3, 906–920. doi:10.1111/j.2041-210X.2012.00221.x
McGeoch, M. A., Spear, D., Kleynhans, E. J., and Marais, E. (2012). Uncertainty in invasive alien species listing. Ecol. Appl. 22, 959–971. doi:10.1890/11-1252.1
Novak, M., and Wootton, J. T. (2008). Estimating nonlinear interaction strengths: An observation-based method for species-rich food webs. Ecology 89, 2083–2089. doi:10.1890/08-0033.1
Regan, H. M., Colyvan, M., and Burgman, M. A. (2002). A taxonomy and treatment of uncertainty for ecology and conservation biology. Ecol. Appl. 12, 618–628. doi:10.1890/1051-0761(2002)012[0618:ATATOU]2.0.CO;2
Roy, H. E., Rabitsch, W., Scalera, R., Stewart, A., Gallardo, B., Genovesi, P., et al. (2018). Developing a framework of minimum standards for the risk assessment of alien species. J. Appl. Ecol. 55, 526–538. doi:10.1111/1365-2664.13025
Sandvik, H. (2020). Expansion speed as a generic measure of spread for alien species. Acta Biotheor. 68, 227–252. doi:10.1007/s10441-019-09366-8
Sandvik, H., Hilmo, O., Finstad, A. G., Hegre, H., Moen, T. L., Rafoss, T., et al. (2019). Generic ecological impact assessment of alien species (GEIAA): The third generation of assessments in Norway. Biol. Invasions 21, 2803–2810. doi:10.1007/s10530-019-02033-6
Suedel, B. C., Bridges, T. S., Kim, J., Payne, B. S., and Miller, A. C. (2007). Application of risk assessment and decision analysis to aquatic nuisance species. Integr. Environ. Assess. Manag. 3, 79–89. doi:10.1002/ieam.5630030107
Tversky, A., and Kahneman, D. (1974). Judgment under uncertainty: Heuristics and biases. Science 185, 1124–1131. doi:10.1126/science.185.4157.1124
Keywords: impact assessment, invasive species, qualitative assessment, quantitative assessment, risk assessment, testability
Citation: Sandvik H (2023) For quantitative criteria in alien species assessment. Front. Environ. Sci. 11:1119094. doi: 10.3389/fenvs.2023.1119094
Received: 08 December 2022; Accepted: 11 January 2023;
Published: 25 January 2023.
Edited by:
Jaime A Collazo, North Carolina State University, United StatesReviewed by:
Lucian Pârvulescu, West University of Timișoara, RomaniaCopyright © 2023 Sandvik. This is an open-access article distributed under the terms of the Creative Commons Attribution License (CC BY). The use, distribution or reproduction in other forums is permitted, provided the original author(s) and the copyright owner(s) are credited and that the original publication in this journal is cited, in accordance with accepted academic practice. No use, distribution or reproduction is permitted which does not comply with these terms.
*Correspondence: Hanno Sandvik, aGFubm8uc2FuZHZpa0BuaW5hLm5v
Disclaimer: All claims expressed in this article are solely those of the authors and do not necessarily represent those of their affiliated organizations, or those of the publisher, the editors and the reviewers. Any product that may be evaluated in this article or claim that may be made by its manufacturer is not guaranteed or endorsed by the publisher.
Research integrity at Frontiers
Learn more about the work of our research integrity team to safeguard the quality of each article we publish.