- 1College of Economics and Management, China Jiliang University, Hangzhou, China
- 2College of Economics and Management, Zhejiang University of Water Resources and Electric Power, Hangzhou, China
This article aims to explore the inner relationship between targeted poverty alleviation and financing constraints of listed companies, and further reveal the underlying impact mechanism. Based on the data of listed companies in Shanghai and Shenzhen A-share main board participating in targeted poverty alleviation from 2017 to 2020, graded response models and mediation models are conducted. From the perspective of resource motivation, the study examined the relationship between the investment in targeted poverty alleviation and financing constraints of listed companies and introduces policy resources as mediator variable to reveal the underlying mechanism. The study found that the participation of listed companies in targeted poverty alleviation can significantly ease financing constraints, and policy resources can play an intermediary effect in the process. For enterprises with different equity nature, in state-owned enterprises, policy resources play a small intermediary role between poverty alleviation inputs and financing constraints. Meanwhile, in non-state-owned enterprises, government subsidies play a significant intermediary role, and tax incentives play a small intermediary role. In response to this, policy suggestions such as improving the disclosure of poverty alleviation information, expanding policy preferences, and increasing poverty alleviation subsidies have been suggested.
1 Introduction
In recent years, corporate social responsibility has attracted more and more attention. The Chinese President, Xi Jinping, pointed out that only caring wealth is truly meaningful wealth, and only companies that actively undertake social responsibilities are the most competitive and vital companies. Undertaking social responsibility requires enterprises to supervise and restrict their own behavior, and pay attention to serving the society while creating profits. The pyramid model proposed by Carroll (1991) divided corporate social responsibility into economic responsibility, legal responsibility, ethical responsibility and charitable responsibility. Elkington (1998) proposed that corporate behavior should meet the economic bottom line, social bottom line and environmental bottom line. So far, fulfilling social responsibility has become a general consensus. Ensuring corporate benefits and employee rights, participating in public welfare activities and social construction, environmental protection, among others; are the basic ways for enterprises to assume social responsibility. Specifically, contrary to extensive poverty alleviation, targeted poverty alleviation is defined as a poverty control method that uses scientific and effective procedures to accurately identify, assist and manage the targets of poverty alleviation in accordance with the environment of different poverty-stricken areas and the conditions of different poverty-stricken farmers. Enterprise participation in targeted poverty alleviation has been an important social responsibility in the context of the new era. It refers to the charitable behavior of enterprises freely and voluntarily using their disposable resources to relieve the poor under the guidance of government departments (Yang and Cheng, 2020). Listed companies are an important participant in China’s poverty alleviation work and have played an important role in the process of eliminating absolute poverty. According to the white paper on consolidating poverty alleviation and promoting rural revitalization by Chinese listed companies, since putting forward the targeted poverty alleviation strategy, many listed companies in China have carried out targeted assistance to poor areas. According to the annual report of A-share listed companies in 2020, a total of 1,514 listed companies have disclosed that they have participated in poverty alleviation and rural revitalization. Out of these companies, 1,244 have capital investment, with a total poverty alleviation investment of 88.998 billion yuan, and have achieved remarkable results in industrial support, employment and financial support. These enterprises integrate market resources through their own advantages, drive regional development with their market experience, complement each other’s advantages with the government’s poverty alleviation, boost the development of China’s poverty alleviation cause and promote the realization of common prosperity. These companies have specific motivations for participating in targeted poverty alleviation, and they can also bring specific benefits to them. Existing research shows that listed companies have three motivations for participating in targeted poverty alleviation: 1) Political motivation: in order to maintain and strengthen political connections, listed companies are more willing to fulfill their social responsibilities for targeted poverty alleviation (Yang and Cheng, 2020); 2) Economic motivation: in order to enhance the corporate image, pursue more hidden benefits, and then improve its profitability (Wang, 2020); 3) Resource motivation: in order to obtain more resources, such as government subsidies, tax incentives, lower financing costs, among others (Wang et al., 2020). In terms of economic consequences, the participation of listed companies in targeted poverty alleviation can significantly improve corporate financial performance (Zhang and Xing, 2019) and effectively ease their own financing constraints (Yi et al., 2020). Based on this motivation, enterprises will participate in poverty alleviation through industrial development, transfer of employment, relocation, ecological protection, education, medical treatment and other ways to increase income for the poor, drive industrial development and economic growth in poor areas, and achieve a win-win situation for enterprise interests, social benefits and economic benefits.
However, the ways in which companies participate in poverty alleviation are diversified, and a single-dimensional measurement is often difficult to reflect the comprehensive performance of poverty alleviation investment. For instance, some scholars regard industrial targeted poverty alleviation investment as enterprise poverty alleviation investment (Yin et al., 2021). Therefore, this article uses the item response theory (IRT) to measure poverty alleviation investment to improve its comprehensiveness and accuracy. In addition, as for the discussion of the mechanism behind the effect of poverty alleviation, scholars pay more attention to audit quality, financial performance, and enterprise innovation. For example, Guo et al. (2022) demonstrated that enterprises’ participation in targeted poverty alleviation has significantly improved audit quality; Zhang and Xing (2019) have proved that enterprises’ increasing investment in targeted poverty alleviation has a significant effect on improving their financial performance. Liu et al. (2020) found that the targeted poverty alleviation behavior of enterprises will improve the innovation output of enterprises, but the quality of enterprise innovation will decline under the same structure. However, existing research seldom pays attention to financing constraints. In view of this, this article has compiled the data of the Shanghai and Shenzhen A-share main board listed companies that participated in targeted poverty alleviation from 2017 to 2020. The investment in targeted poverty alleviation was regarded as a latent variable, and the item response theory model was used to comprehensively measure various poverty alleviation investments. From the perspective of resource motivation, using policy resources as an intermediary variable, we thoroughly examined the impact of targeted poverty alleviation investment on financing constraints. In the same vein, we reveal the mechanism and consequences of listed companies’ participation in targeted poverty alleviation and provide theoretical and practical evidence for deepening listed companies’ participation in relative poverty governance in the future.
The Item Response Theory (IRT) has a long history of application in educational testing and psychology, and is increasingly being used in economics and social sciences to infer potential characteristics. Currently, IRT models are used to study cognitive abilities, mental concepts, personality characteristics, attitudes, quality of life, patient satisfaction, and other potential attributes that cannot be directly measured. Recently, research in the fields of economics and social sciences has used this method to construct indexes that capture various potential characteristics. For example, Cappellari and Jenkins (2007) used information about family characteristics and the individual’s perception of purchasing specific items to construct a household poverty index; Ødegaard and Roos (2015) used the answers to health status and psychosocial environment questions to construct labor quality indicators; Pericoli et al. (2015) estimated the social capital index based on the answers to the questions, which indicate the degree of social capital to social networks, citizen participation, and neighbor relations. Abdul-Salam and Phimister (2017), and Gao and Niu (2019) constructed an IRT model to accurately estimate the ability of farmers to obtain information. Peng and Zhu (2019) measured consumer objective and supervisory financial literacy index based on IRT. Li (2020) employed the grade response model in item reflection theory to scientifically measure the concept of rights, authority and other complex political and psychological concepts. The basic idea of the IRT model used in these studies is that there are some potential characteristics or abilities, and the level of characteristics or abilities of the research object can be determined through observable responses, whether it is the answer to the question scale or the available data.
Many studies make use of the predicted potential index as an independent variable for their subsequent analysis. For example, Ødegaard and Roos (2015) used predictive values of potential labor quality in a DEA study to explain the production efficiency of large Swedish companies. Pericoli et al. (2015) used their index to explain the impact of social capital on consumer insurance and income fluctuations. Gao and Niu (2019) used estimated information acquisition capabilities to study its impact on farmers’ adoption of Green Control Technique (GCT). Li (2020) used the measured political psychology such as the concept of rights to study its influence on online political participation.
According to the definition of Fazzari et al. (1988), financing constraints refer to the situation where the external financing costs of the company are too high due to the incomplete market, and therefore the company’s investment cannot reach the optimal level. The typical consequence of financing constraints is to make a significant change in the matching status of corporate investment risks and returns compared with the situation in a complete market. To put it simply, companies are restricted in their external financing. Rajan and Zingales (1995) found that in the financing process, companies will cause “principle-agent” and “adverse selection” problems due to the existence of information asymmetry in the market. This leads to high external financing costs in the market and can only rely on internal funds with lower financing costs. However, internal funds are often very limited, so companies will be unable to meet financing needs due to the inability to pay high external financing costs. This indicates that companies are subject to financing constraints.
Current research reveals that financing constraints are mainly affected by the following factors: firstly, financial environment. In a sound financial environment with open markets, complete systems, and high resource allocation efficiency, corporate financing is easier and more convenient. Financial reform can promote the efficiency of the capital market, thereby alleviating the financing constraints faced by enterprises (Claessens and Laeven, 2003). Baum et al. (2011) inferred that the financial environment plays a vital role in reducing the barriers to external market financing, and that financing constraints are less in a good financial environment. Cheng et al. (2012) found that the opening of the capital market has a certain effect on alleviating financing constraints. Similarly, Wei et al. (2014) found that the optimization of the financial environment can effectively alleviate corporate financing constraints. Secondly, political connection. The government tends to favor politically connected companies and give them better credit resources and financing platforms. Cull et al. (2015) believe that political connections play an important role in explaining the financing conditions of Chinese companies, and political connections can significantly reduce financing constraints. Yu et al. (2012) found two mechanisms by which political connections can ease financing constraints. One of the mechanisms involves the use of political connections as a signal to reduce the information effect of information asymmetry. The other mechanism is the resource effect, this involves increasing the ability to obtain resources through political connections. Thirdly, internal factors of the enterprise. These include credit record, business performance, ownership property, ownership concentration, profitability, among others. Pagano et al. (1998) found that a company with a better credit record has a better reputation, which is conducive to the alleviation of financing constraints. Similarly, Cull and Xu (2003) believe that the better the business performance of the company, the more helpful it is to stabilize the bank’s expectations of its future cash flow, thereby making it easier for the company to obtain bank loans and alleviating financing constraints. In addition, Faccio (2006) believes that compared with private enterprises, state-owned enterprises face less financing constraints. In the same vein, Wang and Zhao (2010) found that the higher the ownership concentration, the lower the capital adequacy ratio, and the better the profitability, the smaller the financing constraints of companies. Moreover, from a geographical point of view, listed companies in the eastern region face smaller financing constraints than those in the central and western regions. Fourthly, information disclosure. Some scholars have found a correlation between information disclosure and the cost of capital. The more information disclosed by a company, the lower the cost of capital (Diamond and Verrecehia, 1991; Botosan, 1997; Healy and Palepu, 2001; Verrecchia, 2001; Zhang and Lv, 2007) degree of information asymmetry and ease financing constraints. In addition, they contend that the higher the market’s attention to companies, the more advantageous the company’s information mining and dissemination. This further reduces information asymmetry significantly thereby reducing corporate financing constraints. However, Qian et al. (2016) found that the disclosure of corporate social responsibility information has a negative effect on financing constraints. They also assert that the accounting conservatism of a company has a negative effect on financing constraints, and the roles of the two play an alternative relationship.
Corporate financing constraints are affected by many parties, of course, including social responsibility behaviors. According to existing research, investors are more inclined to invest funds in enterprises that fulfill social responsibilities (Roberts and Dowling, 2002; Baron, 2007), and fulfilling social responsibilities can alleviate financing constraints (Godfrey, 2005). Richardson and Welker (2001) found that corporate disclosure of social responsibility information can help deliver positive information to the market, reduce information asymmetry between investors and operators, reduce investors’ risk of predicting corporate-related financial information, thereby reducing capital costs and alleviating financing constraints. Similarly, Dhaliwal et al. (2011) found that companies can reduce the degree of information asymmetry by disclosing social responsibility information reports, thereby reducing their financing costs. In terms of the social responsibility of poverty alleviation, many scholars have found that enterprises’ participation in targeted poverty alleviation can ease financing constraints. For instance, Deng et al. (2020) used A-share listed companies as a research sample and found that companies actively carrying out targeted poverty alleviation activities can effectively alleviate their own financing constraints. In the same vein, Wang and Wang (2020) found that companies’ participation in targeted poverty alleviation and political connections can alleviate financing constraints, and the two have a synergistic effect on the impact of financing constraints.
The contributions of the research are as follows: First, there have been documents discussing the motivations and economic consequences of enterprises participating in precision poverty alleviation. When it comes to the calculation of precision poverty alleviation investment, it uses the absolute number of inputs or the proportion of relative assets and income, among others. However, the way in which each enterprise participates in poverty alleviation is different, and the above methods cannot be used to scientifically compare poverty alleviation investment. This article proposes a method for measuring poverty alleviation investment based on the Item Response Theory (IRT), which is more scientific and comparable. Second, the existing literature discussing the relationship between targeted poverty alleviation by enterprises and financing constraints mainly focuses on direct impacts. From the perspective of resource motives, this article adds political resources as an important factor to examine the reasons behind participating in poverty alleviation for enterprises to ease financing constraints mechanism. Finally, based on the conclusion that enterprises’ participation in targeted poverty alleviation will alleviate financing constraints, and policy resources play an intermediary role in it, this article puts forward suggestions to improve the disclosure of poverty alleviation information, expand policy preferences, and increase poverty alleviation subsidies. This will contribute to the government’s further optimization of the social responsibility disclosure system and regulatory mechanism, and help enterprises to actively carry out targeted poverty alleviation activities and disclose relevant information.
The remainder of this article is organized as follows. The “Literature Review” section reviews relevant literature. The section on “Theoretical Analysis and Research Hypothesis” outlines the relationship among listed companies’ targeted poverty alleviation, policy resources and financing constraints, and proposes hypotheses. The “Research Design” section describes the main variables and constructs the research model. The “Empirical Analysis” section examines the relationship between the investment in targeted poverty alleviation and financing constraints of listed companies, and introduces policy resources as an intermediary variable. It also highlights the underlying mechanism and further reveals the heterogeneity of poverty alleviation among enterprises of different property right. Finally, the article provides policy recommendations based on the research conclusion.
2 Theoretical analysis and research hypothesis
2.1 Targeted poverty alleviation and financing constraints
All listed companies face financing constraints, and information asymmetry is one of the important factors. According to the signaling theory, in the case of information asymmetry, well-run companies will transmit good news to the outside world, thereby attracting investors. Due to the limited resources of enterprises, when enterprises invest more resources in poverty alleviation, less resources will be used in the production and operation activities of enterprises, which may cause the decline of enterprise benefits and cause losses to stakeholders. However, companies actively fulfilling their social responsibilities for poverty alleviation can send a positive signal to the outside world, because poverty alleviation requires a lot of manpower and material resources to demonstrate corporate strength. This strengthens the willingness of stakeholders to provide resources to the enterprise and alleviates the problem of information asymmetry. At the same time, according to the Opinions on Giving Play to the Role of Capital Market to Serve the National Poverty Alleviation Strategy issued by the CSRC in 2016, the Shanghai Stock Exchange and Shenzhen Stock Exchange successively issued a notice on further information disclosure of poverty alleviation work, requiring listed companies to fully disclose relevant information such as poverty alleviation investment and poverty alleviation effect. The Notice on Improving the Information Disclosure of Listed Companies’ Performance of Poverty Alleviation Responsibilities issued by the Shanghai Stock Exchange in 2019 clearly stipulates that listed companies should fully disclose the specific situation of the company’s performance of its social responsibility for targeted poverty alleviation during the reporting period. Therefore, participation in poverty alleviation activities must be disclosed in the important matters chapter of the company’s annual report. This can establish a good social image for the company and improve its reputation, thereby effectively reducing the information asymmetry between the company and the outside world, and win the recognition by the investors, creditors and other stakeholders. This reduces the possibility and cost of negative events, thereby reducing corporate risks (Jo and Na, 2012). In short, participating in targeted poverty alleviation can improve confidence communication with the outside world, enhance the company’s image and reputation, and thereby increase the funding providers’ confidence in corporate financing. Studies have shown that companies with better social responsibility performance face lower financing constraints, and the transparency of corporate social responsibility performance is of great significance in reducing corporate financing constraints (Cheng et al., 2014). As a result, companies can obtain loans more easily from banks and other creditors. Although fulfilling social responsibilities will bring some extra costs to enterprises, it will also bring high-quality business environment, reduce costs in some aspects, and leave good social credit for enterprises (Wang et al., 2022). Based on this, this study formulates the first hypothesis as (Figure 1).
H1. The more a listed company invests in targeted poverty alleviation, the more it can ease financing constraints.
2.2 Targeted poverty alleviation and policy resources
In line with the resource dependence theory, companies need to find a way to obtain key resources in a stable manner. Political resources, including the government’s disposable financial funds, land and policies, as the key resources for the survival and development of enterprises, have a positive impact on the value of enterprises (Li et al., 2015). These key resources are usually allocated by the government, consequently, companies have certain motivations to respond to government policy guidelines. Participating actively in the government’s targeted poverty alleviation work facilitates enterprises and the government to establish close ties, and they are willing to bear some costs in exchange for more resources (Fan et al., 2013). Policy resources, as an important part of political resources, also play a key role for enterprises, especially the two major policies of government subsidies and tax incentives. In order to compensate enterprises for the cost of fulfilling their social responsibilities, the government usually adopts subsidies and preferential tax policies to increase the enthusiasm of enterprises to participate in targeted poverty alleviation. Yan and Tang (2020) found that the participation of listed companies in targeted poverty alleviation can significantly increase government subsidies. Similarly, Wang et al. (2020) believe that enjoying tax incentives is a major driving force for listed companies to participate in targeted poverty alleviation. Based on this, this study formulates the second hypothesis as (Figure 1).
H2. The more a listed company invests in targeted poverty alleviation, the more policy resources it can obtain.
2.3 Targeted poverty alleviation, policy resources and financing constraints
The signaling theory states that listed companies hope to send positive signals to the outside world by fulfilling their social responsibilities for poverty alleviation, reducing information asymmetry, and thereby reducing their financing constraints. However, precise poverty alleviation information is generated by companies, investors will question the authenticity of the information. However, due to the differences in the nature of enterprises, there are also differences in the possibility of being questioned. For state-owned enterprises, they have natural political advantages and their social responsibilities are more based on social obligations (Yi et al., 2020), and the intensity of internal audit and government supervision is slightly higher than that of non-state-owned enterprises. Therefore, investors rarely question the authenticity of information when participating in targeted poverty alleviation. Relatively speaking, non-state-owned enterprises tend to obtain more political resources when participating in targeted poverty alleviation that investors will be more suspicious of the authenticity of their information disclosure, such as questioning if companies are deliberately concealing unfavorable information for their own benefit and inflating investment in poverty alleviation. At the same time, the government’s policy resources for enterprises (such as government subsidies or tax incentives) can constitute an objective supervision mechanism, which is conducive for companies to send more true and objective positive signals to the outside world to enhance creditors’ confidence, thereby reducing financing constraints. Wu (2019) believes that government subsidies can eliminate the information asymmetry between the supply and demand sides of funds to a certain extent, and reduce corporate financing constraints. Yu and Yang (2016) found that small and medium-sized enterprises with more financing, and tax incentives; have a significant positive effect on corporate financing. Based on this, this study formulates the third hypothesis as (Figure 1).
H3. Policy resources play an intermediary role between the targeted poverty alleviation and financing constraints of listed companies.
3 Research design
3.1 Sample selection and data sources
The poverty alleviation data used were derived from the Chinese Research Data Services (CNRDS). The selected data is the amount of each sub-investment in the precision poverty alleviation table disclosed in the annual report of the Shanghai and Shenzhen A-share listed companies participating in precision poverty alleviation from 2017 to 2020. Other financial data comes from China Stock Market and Accounting Research Database (CSMAR). The data were screened according to the purpose of the research ensuring the exclusion of: 1) listed companies without specific investment amount; 2) listed companies in the financial industry. Due to the particularity of the financial industry, it has special sub-inputs, such as the amount of loans for targeted poverty alleviation projects, which are quite different from other industries; 3) ST and *ST listed companies with abnormal financial indicators; 4) samples that have been listed for less than 3 years and whose variables are missing. In addition, in order to eliminate the influence of extreme values, this study winsorizes all the continuous variables at 1% and 99% level. After the screening, 1,036 research samples were finally determined, with a total of 4,144 annual data.
3.2 Definition of variables
3.2.1 Dependent variable
In this study, financing constraints is regarded as the dependent variable. Previous studies have shown that there are various methods that can be used to measure financing constraints. The more representative methods include KZ index (Kaplan and Zingales, 1997), WW index (Whited and Wu, 2006) and SA index (Hadlock and Pierce, 2010). The Tobin Q value in the KZ index, which reflects the investment opportunities of enterprises, is prone to large measurement errors, resulting in inaccurate measurement of corporate financing constraints. Compared with the KZ index, the WW index excludes Tobin’s Q value, and in addition to the financial characteristics of the company itself, it also considers the characteristics of the industry in which it is located, which has broader economic significance. The specific model is as follows:
Among them,
3.2.2 Explanatory variable
The explanatory variable in this study is the investment in targeted poverty alleviation. Its original data comes from nine targeted poverty alleviation projects (See Table 1 for mean value statistics). Due to the diversification of poverty alleviation investment categories, the units of various indicators are not the same, and there may be some problems in fitting the composite index. However, the project response theory is a potential variable estimation method considering the difference of project weights. This article regards poverty alleviation investment as a potential structure. First, we use the quartile method to process the itemized input of poverty alleviation samples in each year, and convert the ordinal variables of 0, 1, 2, 3, and 4 to facilitate the parameter estimation of the grade response model (GRM). Second, we use the Bayesian expectation posterior estimation method to estimate the latent investment in targeted poverty alleviation.
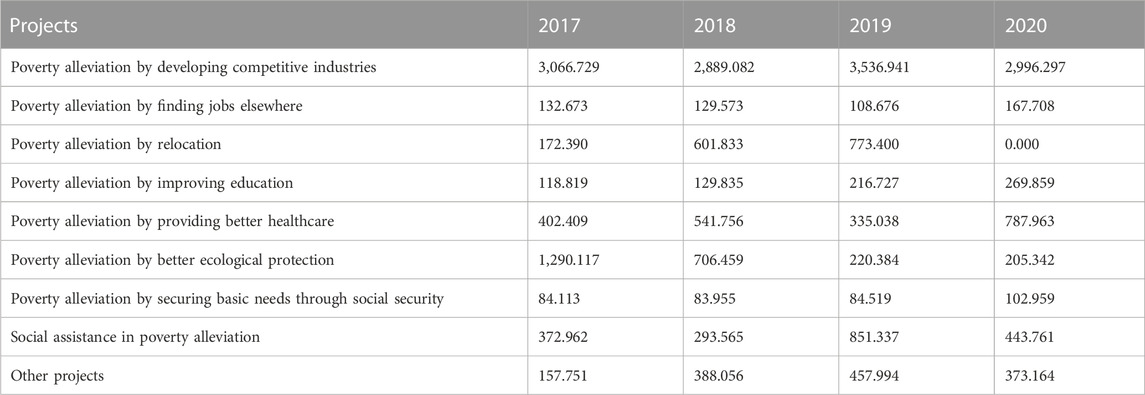
TABLE 1. Descriptive statistics of listed companies participating in targeted poverty alleviation (Unit: ten thousand Yuan).
Assuming that
Among them, D = 1.7, ai is the degree of discrimination of the i sub-item, and bik is the difficulty value of k points for the i sub-item. According to the study (Yang et al., 2008), the value of the discrimination parameter a is between [0.3, 4], and the difficulty parameter of the item is moderate when the value range of b is in the range of [−4, 4]. The research results show that the discrimination parameter and the diversity parameter conform to the above range.
On the basis of parameter estimation, the Bayesian posterior expectation estimate is used to estimate the poverty alleviation investment
Before parameter estimation, the single-dimension and local independence tests should be carried out. The single-dimensional test can be verified by factor analysis results. The results show that the characteristic roots of the first factor are 1.30, 1.33, 1.42, and 1.69 respectively from 2017 to 2020, and the characteristic roots of the second factor are 0.20, 0.17, 0.19, and 0.17 respectively. The characteristic roots of the first factor are 6.5 times, 7.82 times, 7.47 times, and 9.94 times of the characteristic roots of the second factor, all of which are greater than 3, meeting the single-dimensional test. On the other hand, R software provides Q3 program for local independence inspection. The results show that the absolute value of the correlation coefficient of all poverty alleviation projects is less than 0.3, meeting the assumption of local independence.
3.2.3 Mediating variable
The mediating variable in this study is the policy resources. These are measured by the most intuitive performance of policy resources—government subsidies and tax incentives received. Among them, the government subsidy data adopts the “Extraordinary profit and loss—government subsidies included in the current profit and loss” disclosed in the notes to the annual report, and the logarithm is used for processing. Tax incentives mainly consider income tax incentives, using the difference between the nominal corporate income tax rate and the actual tax rate and multiplying the total profit, and standardizing the subsequent measurement.
3.2.4 Control variables
Based on the existing research in related literature, the following control variables are selected: listing age, ownership concentration, asset-liability ratio, growth, cash flow, profitability, capital expenditure rate, as well as the control the impact of the Year, Industry, and Area. The variables and definitions are shown in Table 2.
3.3 Model setting
According to Wen et al.’s (2004) method, when studying the mediation effect, the model is constructed as follows.
Model a.
Model b.
Model c.
3.4 Empirical analysis
3.4.1 Descriptive statistics and correlation analysis
From Table 3, the mean value of financing constraints (WW) is −1.053 indicating that the scale of enterprise financing constraints has small difference. The mean value of poverty investment of enterprises is 0.173, and the standard deviation is 0.192. It can be seen that enterprises participating in targeted poverty alleviation have a small difference in poverty alleviation investment. From the perspective of the main control variables, the average of government subsidies (Sub) is 16.663, the standard deviation is 2.536, the median is 16.901, the minimum value is 0, and the maximum value is 22.876, indicating that there are certain differences in government subsidies to enterprises (Sub). The average age of enterprises is 14.935, the standard deviation is 7.284, the median is 15.000, the minimum value is 2, and the maximum value is 31, indicating that there are differences in the age of enterprises listed (Age). The standards of other indicators are small, the maximum and minimum values conform to the distribution law, and there are no extreme values and abnormal values in the observation sample, all within reasonable scope.
From the results in Table 4, it can be seen that the correlation between the main variables is more consistent with the hypothesis, and Hypotheses 1, 2, and 3 can be initially verified. In addition, the absolute value of the correlation coefficient of each variable is 0.426 at the maximum, which does not exceed 0.6. The test results of the variance inflation factor show that the maximum value of VIF is 1.63 and the average value is 1.19, indicating that there is no serious multiple collinearity problem.
3.4.2 Regression results and analysis
Table 5 reports the regression results of the three models. The results of model a show that the regression coefficient of investment in targeted poverty alleviation is −0.096, indicating that participation in targeted poverty alleviation can significantly reduce financing constraints. Hypothesis 1 has been verified. The results of model b show that the regression coefficients of poverty alleviation investment (Pov) are 1.894 and 0.086, respectively, which the participation of listed companies in targeted poverty alleviation can significantly increase policy resources. Hypothesis 2 is verified. Model c tests the mediation effect. The results show that the regression coefficients of poverty alleviation investment (Pov) are −0.082 and −0.089. The regression coefficient of government subsidies (Sub) is −0.007, and the regression coefficient of tax incentives (Tax) is −0.081, both of which can alleviate financing constraints at the 1% significance level. The absolute value of the coefficient of influence of poverty alleviation investment (Pov) on financing constraints (WW) is reduced from 0.096 to 0.082 and 0.089, indicating that policy resources play an intermediary role in the influence of poverty alleviation investment (Pov) on financing constraints (WW). Hypothesis 3 has been verified.
3.4.3 Robustness tests
Given that there is a two-way causal relationship between poverty alleviation investment and the latter two, that is, the more policy resources, the smaller the financing constraints, and the greater the investment in poverty alleviation. In order to solve this endogenous problem, the explanatory variables are lagging one period instead of the original independent variable for regression. Due to the limitation of data acquisition, 1 year’s data will be reduced compared to the original regression. The specific regression results are shown in Table 6. The regression coefficient of poverty alleviation investment (Pov) in model a is still significantly negative, the regression coefficient of poverty alleviation investment (Pov) in model b is still significantly positive, and the regression coefficients of poverty alleviation investment (Pov) and policy resources in model c are still significantly negative and the absolute value of the regression coefficient of poverty alleviation input (Pov) is smaller than the regression coefficient in model a, which supports Hypotheses 1, 2, and 3.
In addition, an alternative variable method was adopted, replacing the WW index, which measures financing constraints, with the SA index. Since the WW index measures financing constraints, it contains many endogenous variables, such as cash flow, leverage and others, and financing constraints are mutually determined by them. In order to avoid this endogenous influence, Hadlock and Pierce (2010) used the SA index constructed by two exogenous variables of company size (SIZE) and company establishment age (AGE) to measure financing constraints.
The model is as follows:
Since the SA index of the sample companies are all negative numbers, the absolute value of SA is taken as the logarithm to represent the financing constraint FC. The larger the value, the greater the financing constraint. The regression results in Table 7 are basically consistent with the previous regression results, indicating that the results of this study are more reliable.
In addition, the article also selected some sample companies for robustness testing. We selected regions with 100 or more listed companies for study and obtained 3,204 samples in total. The results show that the influence direction and significance of explanatory variables and intermediary variables have not changed. At the same time, we also selected some control variables for the robustness test, and found that the influence direction and significance of explanatory variables and intermediary variables did not change. Further, considering the error terms are correlated among groups, we adopted the “cluster adjustment standard error” (Petersen, 2009). We found that there is also no effect on the significance level of key empirical variables and the mediator variables. Therefore, we believe that the research results are robust.
3.4.4 Further study
In order to reveal the heterogeneity of poverty alleviation among enterprises of different equity natures, the samples were divided into state-owned enterprises and non-state-owned enterprises to perform regression estimation respectively. The results are shown in Tables 8, 9. The results show that the regression results of state-owned enterprises and non-state-owned enterprises are basically the same as the above results, in line with Hypotheses 1, 2, and 3. Further performing the Sobel test, the Z value is significant, that is, whether for state-owned enterprises or non-state-owned enterprises, policy resources have an intermediary effect in the relationship between targeted poverty alleviation and financing constraints of listed companies, but the intermediary effects are different. Regarding the policy resource of government subsidies (Sub), the mediation effect of state-owned enterprises accounted for 8.02% of the total effect, and the mediation effect of non-state-owned enterprises accounted for 22.79% of the total effect. The intermediary effect of non-state-owned enterprises is significantly greater than that of state-owned enterprises. In terms of tax incentives (Tax) as a policy resource, the mediation effect of state-owned enterprises accounted for 7.88% of the total effect, and the mediation effect of non-state-owned enterprises accounted for 3.42% of the total effect. The intermediary effect of state-owned enterprises is slightly higher than that of non-state-owned enterprises.
The explanation of this article is that state-owned enterprises have inherent advantages given that state-owned banks can provide guarantees for their financing. The demand for reducing financing constraints by participating in targeted poverty alleviation is lower, so the two intermediary effects are not high. Non-state-owned enterprises are more demanding for more policy resources, and are more likely to obtain more policy resources by participating in targeted poverty alleviation, thereby reducing financing constraints. However, non-state-owned enterprises have fewer channels to obtain government subsidies as a policy resource, and participation in targeted poverty alleviation is a very important aspect. There are more ways to obtain tax incentives, and it is not necessary to participate in targeted poverty alleviation. Therefore, the intermediary role of tax incentives is significantly less than that of government subsidies.
4 Discussion
First of all, the participation of listed companies in targeted poverty alleviation is a complex work, including nine different poverty alleviation projects or ways. Some previous studies only considered a poverty alleviation project, such as replacing targeted poverty alleviation with industrial targeted poverty alleviation (Yin et al., 2021), while others used the total amount of poverty alleviation investment or the proportion of poverty alleviation investment to assets (Du et al., 2019; Wang and Wang, 2020; Wang et al., 2022). These methods of measuring poverty alleviation investment are not comprehensive enough, especially when the same company participates in different poverty alleviation projects, adding up these poverty alleviation project input implies the equal weight of different poverty alleviation projects. In fact, the weight of different poverty alleviation projects may be different, because different companies attach different importance to and invest in different poverty alleviation projects. This article uses the grade response models to estimate the difficulty coefficient, which can reflect the differences of various poverty alleviation projects, and then provide more accurate evidence for measuring the potential poverty alleviation input variables.
Secondly, we examined the impact mechanism of participating in targeted poverty alleviation on alleviating corporate financing constraints. The research found that participating in targeted poverty alleviation can significantly reduce corporate financing constraints, which is consistent with other research conclusion (Deng, Tao, and Ji, 2020), because the government’s direct preferential policies and state-owned banks have encouraged enterprises to assume policy-oriented social responsibilities for incentive purposes (Yin et al., 2021). From the perspective of impact mechanism, participating in targeted poverty alleviation can help listed companies obtain policy resources, such as government subsidies and tax incentives, which can significantly alleviate the financing constraints of companies. Similarly, Deng, Tao, and Ji (2020) believed that by participating in targeted poverty alleviation, enterprises will receive more economic resources (such as credit support and government subsidies). However, Wang and Wang (2020) found that there is a certain lag in the impact of political connection on the financing constraint mitigation of targeted poverty alleviation.
Finally, policy resources have different impacts on enterprises of different nature. Specifically, the intermediary effect of policy resources on state-owned enterprises to alleviate financing constraints is small. We believe that state-owned listed companies have relatively more policy resources, and participating in targeted poverty alleviation is mainly due to political responsibility and social responsibility. Similarly, Wang and Wang (2020) believe that the mitigation effect of state-owned enterprise financing constraints came from political connection and targeted poverty alleviation. On the other hand, the intermediary effect of government subsidies on non-state-owned listed companies to ease financing constraints is large, while the intermediary effect of tax incentives is small.
5 Conclusion and suggestions
This article reports findings from a study that uses the Shanghai and Shenzhen A-share listed companies participating in targeted poverty alleviation from 2017 to 2020 as a research sample. It makes use of policy resources as an intermediary variable to explore the relationship between listed companies’ targeted poverty alleviation investment, policy resources and financing constraints. The study establishes that: 1) The investment in targeted poverty alleviation by listed companies has a significant negative impact on financing constraints, that is, the greater the investment in poverty alleviation, the smaller the financing constraints; 2) The investment in targeted poverty alleviation by listed companies has a significant negative impact on policy resources, that is, the greater the investment in poverty alleviation, the more policy resources they can obtain; 3) Listed companies’ investment in targeted poverty alleviation and policy resources have significant negative impact on financing constraints. Policy resources play an intermediary role between poverty alleviation investment and financing constraints. This means that listed companies can increase the policy resources they obtain by participating in targeted poverty alleviation, thereby reducing financing constraints. Further research shows that for enterprises with different equity nature, for instance, in state-owned enterprises; policy resources have a small intermediary role between poverty alleviation inputs and financing constraints. Meanwhile, in non-state-owned enterprises, government subsidies play a significant intermediary role, and tax incentives play a small intermediary role.
Based on the above conclusion, the following suggestions are put forward: First, the regulatory authorities should continuously improve the disclosure system of precise poverty alleviation information. Through institutional constraints, increase the openness and transparency of corporate poverty alleviation information disclosure, so as to give play to the signal transmission function of the securities market. In addition, there is need to provide institutional guarantees for guiding enterprises to participate actively in the governance of relative poverty. Second, the government should strengthen policy incentives to guide enterprises’ enthusiasm for participating in targeted poverty alleviation. The implementation of more preferential policies can help attract enterprises, such as broadening the scale of poverty alleviation special debt financing and the scope of green poverty alleviation debt. In order to strengthen government-enterprise cooperation, and promote the solution of problems such as relative poverty in the new stage and achieve the consolidation and expansion of poverty alleviation achievements and the effective connection with rural revitalization. Third, increase the incentives and subsidies for enterprise poverty alleviation development. In particular, increase the subsidy support for private enterprises to participate in poverty alleviation, thereby stimulating the enthusiasm of enterprises to participate in the governance of relative poverty in the new era.
Compared with the existing empirical research on listed companies participating in targeted poverty alleviation to alleviate financing constraints, this article introduces policy resources as an intermediary variable, which not only verifies the direct effect, but also the mechanism of action. However, this article still has some shortcomings. First, the consideration of endogenous problems is not comprehensive enough, and appropriate tool variables to deal with this are not found. Second, our study lacks analysis of the impact of macroeconomic factors. Finally, this article does not take into account the final outcome of poverty alleviation. These areas provide some directions for subsequent research, and future research will improve these deficiencies.
Data availability statement
The original contributions presented in the study are included in the article/supplementary material, further inquiries can be directed to the corresponding author.
Author contributions
ZH: Data curation; Methodology; Project administration; Software; Writing—review and editing. YX: Conceptualization; Data curation; Formal analysis; Project administration; Software; Visualization; Writing—original draft. DL: Formal analysis. MZ: Writing—review and editing; Funding acquisition. All authors contributed to the article and approved the submitted version.
Funding
This research was funded by Zhejiang soft science research program (Project No. 2023C25046), Humanities and Social Sciences Foundation of the Ministry of Education of China (Project No. 22YJAZH153).
Conflicts of interest
The authors declare that the research was conducted in the absence of any commercial or financial relationships that could be construed as a potential conflict of interest.
Publisher’s note
All claims expressed in this article are solely those of the authors and do not necessarily represent those of their affiliated organizations, or those of the publisher, the editors and the reviewers. Any product that may be evaluated in this article, or claim that may be made by its manufacturer, is not guaranteed or endorsed by the publisher.
References
Abdul-Salam, Y., and Phimister, E. (2017). Efficiency effects of access to information on small-scale agriculture: Empirical evidence from Uganda using stochastic frontier and IRT models. J. Agric. Econ. 68, 494–517. doi:10.1111/1477-9552.12194
Baron, D. P. (2007). Managerial contracting and corporate social responsibility. J. Public Econ. 92 (1/2), 268–288. doi:10.1016/j.jpubeco.2007.05.008
Baum, C. F., Schäfer, D., and Talavera, O. (2011). The impact of the financial system’s structure on firms’ financial constraints. J. Int. Money Finance 30 (4), 678–691. doi:10.1016/j.jimonfin.2011.02.004
Botosan, C. A. (1997). Disclosure level and the cost of equity capital. Account. Rev. 72 (3), 323–349.
Cappellari, L., and Jenkins, S. P. (2007). Summarizing multiple deprivation indicators. ISER working paper series.
Carroll, A. B. (1991). The pyramid of corporate social responsibility: Toward the moral management of organizational stakeholders. J. Bus. Horiz. 1991, 7–8.
Cheng, B., Ioannou, I., and Serafeim, G. (2014). Corporate social responsibility and access to finance. Strategic Manag. J. 35 (1), 1–23. doi:10.1002/smj.2131
Cheng, X., Zhang, J., and Dong, W. (2012). Has the opening of the capital market lowered corporate financing constraints?—based on an empirical study of Chinese listed companies. Secur. Mark. Her. 11, 32–38.
Claessens, S., and Laeven, L. (2003). Financial development, property rights, and growth. J. Finance 58 (6), 2401–2436. doi:10.1046/j.1540-6261.2003.00610.x
Cull, R., Li, W., Sun, B., and Xu, L. C. (2015). Government connections and financial constraints: Evidence from a large representative sample of Chinese firms. J. Corp. Finance 32, 271–294. doi:10.1016/j.jcorpfin.2014.10.012
Cull, R., and Xu, L. C. (2003). Who gets credit? The behavior of bureaucrats and state banks in allocating credit to Chinese state-owned enterprises. J. Dev. Econ. 71 (2), 533–559. doi:10.1016/S0304-3878(03)00039-7
Deng, B., Tao, C., and Ji, L. (2020). Firms’ targeted poverty alleviation and the ease of financial constraints. J. Finance Econ. 46 (12), 138–151. doi:10.16538/j.cnki.jfe.20200817.301
Dhaliwal, S. D., Li, O. Z., Tsang, A., and Yong, G. Y. (2011). Voluntary nonfinancial disclosure and the cost of equity capital: The initiation of corporate social responsibility reporting. Account. Rev. 86 (1), 59–100. doi:10.2308/accr.00000005
Diamond, D., and Verrecehia, R. (1991). Disclosure liquidity and the cost of capital. J. Finance 4, 1325–1359. doi:10.1111/j.1540-6261.1991.tb04620.x
Du, S. F., Shi, H. G., and Zhang, Y. Q. (2019). Research on the influencing factors of the precise poverty alleviation behavior of listed companies in China —based on the perspective of social responsibility. Public Finance Res. (02), 104–115. doi:10.19477/j.cnki.11-1077/f.2019.02.007
Elkington, J. (1998). Cannibals with forks: The triple bottom line of 21st century business. Environ. Qual. Manag. 81 (37)–51.
Faccio, M. (2006). Politically connected firms. Am. Econ. Rev. 96 (1), 369–386. doi:10.1257/000282806776157704
Fan, J., Wong, T., and Zhang, T. (2013). Institutions and organizational structure: The case of state-owned corporate pyramids. J. Law Econ. Organ. 29 (6), 1217–1252. doi:10.1093/jleo/ews028
Fazzari, S., Hubbard, R. G., Petersen, B. C., Blinder, A. S., and Poterba, J. M. (1988). Financing constraints and corporate investment. Brookings Pap. Econ. Activity 1, 141–195. doi:10.2307/2534426
Gao, Y., and Niu, Z. (2019). Risk aversion, information acquisition ability and farmers’ adoption behavior of green control techniques. Chin. Rural. Econ. 8, 109–127.
Godfrey, P. C. (2005). The relationship between corporate philanthropy and shareholder wealth: A risk management perspective. Acad. Manag. Rev. 30 (4), 777–798. doi:10.5465/amr.2005.18378878
Guo, H., Lv, X., and Wang, H. (2022). The impact of enterprises' participation in targeted poverty alleviation on audit quality. J. Southwest Petroleum Univ. Soc. Sci. Ed. 24 (06), 38–48.
Hadlock, C. J., and Pierce, J. R. (2010). New evidence on measuring financial constraints: Moving beyond the KZ index. Rev. Financial Stud. 23 (5), 1909–1940. doi:10.1093/rfs/hhq009
Healy, P. M., and Palepu, K. G. (2001). Information asymmetry, corporate disclosure, and the capital markets: A review of the empirical disclosure literature. J. Account. Econ. 31 (3), 405–440. doi:10.1016/S0165-4101(01)00018-0
Jo, H., and Na, H. (2012). Does CSR reduce firm risk? Evidence from controversial industry sectors. J. Bus. Ethics 110 (4), 441–456. doi:10.1007/s10551-012-1492-2
Kaplan, S. N., and Zingales, L. (1997). Do investment-cash flow sensitivities provide useful measures of financing constraints? Q. J. Econ. 112 (1), 169–215. doi:10.1162/003355397555163
Li, F. (2020). An analysis of the political psychological mechanism of online political participation: Measurement and analysis based on item response theory. J. Party Sch. Tianjin Comm. CPC 22 (2), 39–46.
Li, W., Wang, P., and Xu, Y. (2015). Philanthropy, political connection and debt finance: Reciprocal behavior of governments and private enterprises. Nankai Bus. Rev. 18 (1), 4–14.
Liu, C., Sun, L., and Cai, Q. (2020). Targeted poverty alleviation and enterprise innovation. Account. Econ. Res. 34 (05), 68–88. doi:10.16314/j.cnki.31-2074/f.2020.05.004
Ødegaard, F., and Roos, P. (2015). Measuring the contribution of workers' health and psychosocial work-environment on production efficiency. Prod. Operations Manag. 23 (12), 2191–2208. doi:10.1111/poms.12242
Pagano, M., Panetta, F., and Zingales, L. (1998). Why do companies go public? An empirical analysis. J. Finance 53 (1), 27–64. doi:10.1111/0022-1082.25448
Peng, X., and Zhu, X. (2019). Research on China consumer financial literacy index based on item response theory—empirical evidence from CFPS2014. Journal of. Hubei Univ. (Philosophy Soc. Sci. 2, 133–141. doi:10.13793/j.cnki.42-1020/c.2019.02.022
Pericoli, F. M., Pierucci, E., and Ventura, L. (2015). The impact of social capital on consumption insurance and income volatility in the UK: Evidence from the British Household Panel Survey. Rev. Econ. Househ. 13 (2), 269–295. doi:10.1007/s11150-013-9185-x
Petersen, M. A. (2009). Estimating standard errors in finance panel data sets: Comparing approaches. Rev. Financial Stud. 22 (1), 435–480. doi:10.1093/rfs/hhn053
Qian, M., Xu, G., and Shen, Y. (2016). Corporate social responsibility disclosure, accounting conservatism and financial constraints. Account. Res. 5, 9–17+95.
Rajan, R. G., and Zingales, L. (1995). What do we know about capital structure? Some evidence from international data. J. Finance 50 (5), 1421–1460. doi:10.1111/j.1540-6261.1995.tb05184.x
Richardson, A. J., and Welker, M. (2001). Social disclosure, financial disclosure and the cost of equity capital. Account. Organ. Soc. 26 (7), 597–616. doi:10.1016/s0361-3682(01)00025-3
Roberts, P. W., and Dowling, G. R. (2002). Corporate reputation and sustained superior financial performance. Strategic Manag. J. 23 (12), 1077–1093. doi:10.1002/smj.274
Verrecchia, R. E. (2001). Essays on disclosure. J. Account. Econ. 32 (1), 97–180. doi:10.1016/S0165-4101(01)00025-8
Wang, B. (2020). Research on the influencing factors of listed companies' participation in targeted poverty alleviation in Western China. West Leather 42 (14), 74.
Wang, C., Li, W., Wang, H., and Wang, Z. (2022). Research on the threshold effect of participating in targeted poverty alleviation on corporate performance of listed companies. Sci. Technol. Industry 22 (05), 39–45.
Wang, F., Tao, Y., and Ni, J. (2020). Research on investment efficiency and performance of listed companies under the background of precision poverty alleviation-based on samples of private enterprises. China Soft Sci. 6, 122–135.
Wang, J., and Zhao, L. (2010). An empirical analysis on the financing constraints of China’s listed companies and its factors. Industrial Econ. Res. 3, 77–84. doi:10.13269/j.cnki.ier.2010.03.005
Wang, S., and Wang, J. (2020). Targeted poverty alleviation, political connections and financing constraints. Finance Account. Mon. 20, 16–22.
Wei, Z., Zeng, A., and Li, B. (2014). Financial ecological environment and corporate financial constraints—evidence from Chinese listed firms. Account. Res. 5, 73–80+95.
Wen, Z., Zhang, L., Hou, J., and Liu, H. (2004). Testing and application of the mediating effects. Acta Psychol. Sin. 5, 614–620.
Whited, T. M., and Wu, G. (2006). Financial constraints risk. Rev. Financial Stud. 19 (2), 531–559. doi:10.1093/rfs/hhj012
Wu, L. (2019). Financial subsidies and financing constraints of small and medium-sized enterprises: Research on heterogeneity effect results and mechanisms. Commer. Res. 9 (8), 14–24. doi:10.13902/j.cnki.syyj.2019.08.003
Yan, R., and Tang, S. (2020). Can listed companies participate in targeted poverty alleviation to obtain support from government resources? Secur. Mark. Her. 11, 2–10.
Yang, Y. B., Miao, D. M., Tian, J. Q., Xiao, L. J., Sun, H., and Hong, X. (2008). Item analysis of the Chinese soldier personality questionnaire using item response theory. Acta Psychol. Sin. 40 (5), 611–617. doi:10.3724/sp.j.1041.2008.00611
Yang, Y., and Cheng, H. (2020). Political resources and targeted poverty alleviation of enterprises: Capital consideration under public welfare projects. Mod. Finance Economics-Journal Tianjin Univ. Finance Econ. 40 (9), 64–82. doi:10.19559/j.cnki.12-1387.2020.09.005
Yi, X., Wu, R., and Xie, Z. (2020). Nature of property rights, targeted poverty alleviation behavior of enterprises and capital market response. J. Guizhou Univ. Finance Econ. 205 (02), 98–104.
Yi, Y., Xie, B., Zhou, L., and Wei, Y. (2020). Does CSR affect the cost of equity capital: Empirical evidence from the targeted poverty alleviation of listed companies in China. Plos one 15 (2), e0227952. doi:10.1371/journal.pone.0227952
Yin, Z., Sun, M., and Wu, Y. (2021). Can the targeted poverty alleviation policy alleviate the financing constraints of Chinese enterprises: From the perspective of policy-oriented corporate social responsibility. J. Northeast. Univ. Sci. 23 (06), 32–39. doi:10.15936/j.cnki.1008-3758.2021.06.005
Yu, H., and Yang, Z. (2016). The influence of preferential tax policies on the investment and financing ability of small and medium sized enterprises. Public Finance Res. 12, 101–110. doi:10.19477/j.cnki.11-1077/f.2016.12.009
Yu, W., Wang, M., and Jin, X. (2012). Political connection and financing constraints: Information effect and resource effect. Econ. Res. J. 47 (9), 125–139.
Zhang, C., and Lv, W. (2007). Disclosure, analyst following and financial constraint. Account. Res. 11, 32–38+95.
Keywords: targeted poverty alleviation, listed companies, policy resources, financing constraints, mediator effect
Citation: Huo Z, Xia Y, Liu D and Zhang M (2023) The impact of listed companies’ participation in targeted poverty alleviation on financing constraints: Mediating effect based on policy resources. Front. Environ. Sci. 11:1115819. doi: 10.3389/fenvs.2023.1115819
Received: 04 December 2022; Accepted: 07 February 2023;
Published: 01 March 2023.
Edited by:
Muhammad Zahid Rafique, Center for Economic Research, Shandong University, ChinaReviewed by:
Zhibin Liu, North China Electric Power University, ChinaYonggen Luo, Guangdong University of Finance and Economics, China
Eduardo Moraes Sarmento, University of Lisbon, Portugal
Wei Wang, Sichuan Agricultural University, China
Guangwei Xu, Changzhou University, China
Copyright © 2023 Huo, Xia, Liu and Zhang. This is an open-access article distributed under the terms of the Creative Commons Attribution License (CC BY). The use, distribution or reproduction in other forums is permitted, provided the original author(s) and the copyright owner(s) are credited and that the original publication in this journal is cited, in accordance with accepted academic practice. No use, distribution or reproduction is permitted which does not comply with these terms.
*Correspondence: Mei Zhang, emhhbmdtQHpqd2V1LmVkdS5jbg==