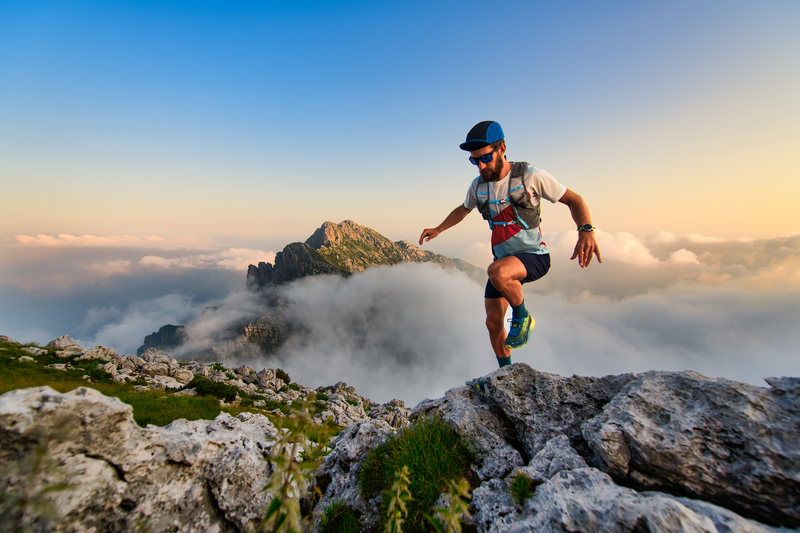
94% of researchers rate our articles as excellent or good
Learn more about the work of our research integrity team to safeguard the quality of each article we publish.
Find out more
ORIGINAL RESEARCH article
Front. Environ. Sci. , 07 February 2023
Sec. Drylands
Volume 11 - 2023 | https://doi.org/10.3389/fenvs.2023.1097049
This article is part of the Research Topic Hydrological Connectivity and Sustainable Watershed Management in a Changing Environment View all 13 articles
Comparative analysis of the impacts of precipitation and land use on streamflow from adjacent catchments is critical to exploring pathways toward water security and sustainable development. In this work, two adjacent catchments (the Mayinghe and Xidahe, abbreviated as MYC and XDC, respectively) in northwestern China were selected to compare the impacts of precipitation change and land use alteration on streamflow change during 1956–2019 using field observation streamflow data and satellite data. An opposite trend of streamflow was found for the two catchments from 1956 to 2019: the streamflow decreased significantly in the MYC (-0.63 × 107m3/10a, p < 0.05), while it increased in the XDC (0.71 × 107m3/10a, p < 0.05). Land conversion dominated the streamflow reduction in the MYC, with a contribution of about 68.3%. In the XDC, precipitation was confirmed to be the major factor driving the increase in streamflow. The streamflow for farmland irrigation reached 27.97 × 104 m3/km2 in the MYC, which was the leading factor of streamflow reduction in the basin. The findings obtained from this work can shed light on the quantitative understanding of streamflow changes in small catchments and offer a scientific basis for sustainable water management in other inland river basins.
Climate change and human activities have affected hydrology in several ways and to varying degrees (Piao et al., 2010; Cooley et al., 2021). Global warming leads to the acceleration of the hydrological cycle, increasing the frequency of extreme hydrological events such as floods and droughts (Piao et al., 2010; Asadieh and Krakauer, 2015; Duan et al., 2022). It also affects the temporal and spatial distribution of water resources, and thus threatens the stability of the ecosystem and thereby of human society (Dey and Mishra, 2017; Lebek et al., 2019; Palmer and Ruhi, 2019; Satge et al., 2019; Darvini and Memmola, 2020). Intensive human activities have caused changes in the underlying surface, such as the conversion from grassland and forest land to farmland and cities, in turn increasing the uncertainty of the availability of water resources, often leading to water resource shortages (Rust et al., 2014; Senbeta and Romanowicz, 2021). Streamflow is one of the main components of the hydrological process, and it plays an important role in water resource security. It is also a key factor in controlling vegetation distribution and species diversity in basins (Dey and Mishra, 2017; Palmer and Ruhi, 2019). Streamflow is affected by various factors including climate, land use, topography, human activities, and so on (Beck et al., 2015; Pourmokhtarian et al., 2017; Guzha et al., 2018; Williamson and Barton, 2020). Climate variability and land use change are considered to be two key factors that control streamflow dynamics in many regions (Fenta et al., 2017; Anache et al., 2018; Lebek et al., 2019; Darvini and Memmola, 2020). For example, precipitation change directly leads to the variation of streamflow, and there is a strong correlation between extreme precipitation and extreme flood (Pall et al., 2011; Duan et al., 2022). And land use change affects the utilization efficiency of water resources, while increasing farmland area leads to an increase in streamflow consumption, although the implementation of water conservation measures could mitigate some of these negative impacts. For sustainable water management and exploring pathways toward water security, understanding streamflow dynamics under precipitation and land-use change is essential.
Numerous researches have reported on streamflow variation, based on long-term observation data, remote sensing data, hydrological simulation data (Zhang et al., 2015; Liu et al., 2017), and even analyzed the driving mechanism behind the observed variation trends (Huo et al., 2021; Su et al., 2021). Models such as the Soil and Water Assessment Tool (SWAT) and the Budyko-based models (Yang et al., 2014; Dey and Mishra, 2017; Zhai and Tao, 2017), the paired catchment method (Rangecroft et al., 2019) and statistical methods based on the double mass curve (Gao et al., 2017) are now available, to elucidate the role of climate change and anthropogenic stressors in shaping the streamflow hydrograph. Comparative studies have also been carried out in many regions throughout the world to further explore the relative and combined effects of climate change and anthropogenic stressors on streamflow (Liu et al., 2017; Cheng and Yu, 2019). These studies have conducted in-depth research on streamflow variations, but there remains a constant need for a better quantitative understanding of this subject through a comparative study of small adjacent catchments experiencing different agricultural intensities, for the following reasons. First, most available comparison researches have been conducted at the mesoscale, but compensating effects in complex watersheds with a variety of land use types, and the impacts of land use changes on hydrology, are relatively less significant at large scales, while they are much more pronounced at smaller scales (Cao et al., 2009; Bieger et al., 2015). Secondly, few comparative studies have been conducted in arid or semi-arid regions, even though the driving mechanisms of streamflow variation have been widely reported in northwestern China (Yang et al., 2017; He et al., 2019; Xue et al., 2021). Furthermore, these driving mechanisms present complex characteristics because it is difficult to ensure the consistency of the natural background in comparative studies, as there are obvious differences in geographical location, terrain and climate, among various catchments (Yang et al., 2014; Darvini and Memmola, 2020).
Northwestern China has an arid or semiarid continental climate, with an average annual rainfall of 130 mm (about 15% of the average annual precipitation globally) (Arora 2001; Shen et al., 2013). It is one of the areas where ecological resources are restricted mainly by water resources, and where streamflow changes have had significant effects on environmental and socioeconomic resources, so that ill-advised utilization of streamflow may lead to the degradation of natural vegetation and aggravate desertification (Lesk et al., 2016; Fereidoon and Koch, 2018). As one of the region most sensitive to climate change (Chen et al., 2015), Northwestern China is highly vulnerable to variabilities in precipitation and temperature; yet it is a place where future streamflow dynamics are complex and uncertain (Ning et al., 2020). In addition, rapid population growth and urbanization have led to oasis expansion and increasing demand for farmland, significantly changing the land cover over the past several decades, and changes such as farmland expansion and grassland loss have led to the redistribution of streamflow in the region (Brath et al., 2006; Sharma et al., 2019). Although many studies have been conducted on streamflow changes and its influencing factors in Northwest China (Wang et al., 2006; Zhang et al., 2014; Yang et al., 2017; Xue et al., 2021), most of them were concentrated in single watershed such as Heihe River Basin (HRB) and Shiyang River Basin (SRB), lacking comparative studies on small catchments. Therefore, a comparative analysis of the causes of streamflow variation in small catchments in Northwestern China is critical to the management of water resources in this region.
The purpose of this work is to analyze the impacts of precipitation change and land use alteration on streamflow of two small adjacent catchments in arid northwestern China, the Mayinghe catchments (MYC) and Xidahe catchments (XDC). The objectives were i) to compare streamflow variations and land use change during 1956–2019 in the MYC and XDC; ii) to quantify the impacts of land use alteration and precipitation change on streamflow; and iii) to estimate water consumption by farmland in different periods from 1956 to 2019. The study could shed light on the understanding of the hydrological process in inland river basins, and provide a valuable reference for coordinating the relationship between human activities and water resources in the context of climate change.
The MYC (100°56′35″-101°18′7″ E, 37°54′58″-38°30′4″ N) and XDC (101°7′34″-101°29′19″ E, 37°47′24″-38°4′34″ N) are first-order catchments of two larger adjacent inland river basins (HRB and SRB, respectively) of arid northwestern China (Figure 1). Both of them originate from the northern part of the middle Qilian Mountains in Shandan County, Gansu Province, and share similar geographical settings (climate, soil and vegetation, et al.), but are under different levels of anthropogenic stressors from agriculture. The advantage of this selection is to ensure the consistency of the natural background of the selected catchments as much as possible, to reduce the potential uncertainties involved in the comparison analysis. The mainstream lengths of XDC and MYC are 30.6 km and 153km, and the areas are 660 km2 and 1163 km2, respectively. The terrain of both the catchments is the high elevation in the south and low in the north: it ranges from 2157 m above sea level (m asl.) in the mountain valley to 4423 m asl. at the peak. Both catchments are continental alpine plateaus with a semi-arid climate, with the characteristics of cold, but still distinctive four seasons, concentrated rainfall and obvious vertical zonation (Zhang and Kang, 2021). The precipitation is mostly concentrated in the summer, with the highest temperatures from June to September, a period that also accounts for about 80% of the total annual precipitation. The main recharge source of the surface runoff is precipitation, followed by ice and snow melt.
FIGURE 1. Geographical location, river and topographic characteristics and locations of meteorological and hydrological stations of XDC (Xidahe Catchment) and MYC (Mayinghe Catchment).
In the MYC, the annual average precipitation and evaporation were 395 mm and 2153.6 mm from 1956 to 2019, respectively. The annual average streamflow was 6.16 × 107 m3 and the annual temperature varied from -29.8°C to 39.8°C with a mean of 7°C, the average depth of frozen soil was generally between 1.2 m and 1.6 m, and the annual frost-free period was 214 days. The total amount of water resources available in the basin is 0.91 × 108 m3, and the per capita water resources are less than 0.06 × 108 m3, which is lower than the internationally recognized water shortage warning line of 0.17 × 108 m3. The upstream section of the MYC is home to the Shandan Army Horse Breeding Farm, the oldest such farm in the world, and the largest in Asia. It is also an important water conservation area of the MYC. However, it is greatly affected by human activities, as large areas of land reclamation have changed the land cover pattern and also significantly affected streamflow in the region. From 1967 to 2000, the conversion of forest and grassland to farmland in the MYC resulted in the reduction of the annual streamflow by 28% (Wang et al., 2006). These developments and the resulting utilization of water resources have resulted in serious ecological deterioration and led to a water resource crisis in the entire basin. The vegetation is vertically distributed in the mountains, with scrub-steppe zones and forest-steppe zones appearing from the foothills to the tops of the mountains in turn. In the corridor plains, it is mainly covered by scrubs and sparse trees. And the main soil types are chestnut and calcareous soil. There are 53,300 people in MYC, and the population is mainly concentrated in the downstream plain, while the upstream is relatively small.
In the XDC, the annual temperature varies from - 27°C to 35.3°C with a mean of 5.7°C from 1956 to 2019, the average depth of frozen soil was generally between 1.4 m and 1.7 m, and the annual frost-free period was 134 days, and annual average precipitation and evaporation were 395 mm and 2098.8 mm, respectively. The annual average streamflow was 1.68 × 108 m3 during 1956–2019, the implementation of a water diversion project since 2003 has introduced an average of 0.25 × 108 m3 per year into the total streamflow of the basin. The annual average sediment concentration was 0.26 kg/m3, and the annual sediment discharge was 41,600 kg. Contrary to the situation of MYC, the XDC was relatively less affected by human activities, and its land cover has remained a relatively pristine state. It is mainly covered by scrubs, the middle and high mountains are covered by trees, shrubs and grasslands, and some areas in the middle reaches are covered by grasslands and forests. The soil is mostly mountain grey cinnamon soil and subalpine meadow soil. The terrain of the basin is mainly mountainous and the population is relatively small.
The products of land use in the Qilian Mountains from 1985 to 2019 were provided by National Tibetan Plateau Data Center (http://data.tpdc.ac.cn). The dataset was produced by using Landsat data. There were 10 land use categories in the products, and we classified them into six categories. The spatial resolution was 30 m, and the accuracy reached 92.2% as evaluated by Google Earth images and field survey data (Zhong and Jue, 2019). Six satellite images, Landsat MSS (1975), Landsat TM (1985, 1995, 2005), and Landsat OLI (2015, 2019), obtained from USGS through Google Earth Engine (GEE) were also used to interpret previous land use changes, and land use categories were identified based on spectral characteristics of the watershed using supervised classification. Finally, all land use types were classified into six categories, namely forest, grassland, farmland, residential, barren land, and water. To improve the accuracy of the final land use change data, we combined the land use data of the Qilian Mountains with the supervised data and then calibrated them using visual interpretation and the third national land survey data of China.
Streamflow and precipitation data were observed data, with a period of 64 years from 1956 to 2019, acquired from the Shandan Hydrology Bureau. Hydrological stations were built above the dams of the Liqiao and Xidahe reservoirs, and these accurately record the inflow and outflow of the catchments. There are five meteorological stations involved in the precipitation data. The precipitation of MYC involved four meteorological stations, the data of Huocheng, Maying and Liqiao Reservoir were used to represent the precipitation status in the middle and lower reaches of MYC. Since there was no meteorological station in the upper reaches of MYC, we selected the precipitation data of Biandukou, which was the nearest to MYC, to represent the upstream precipitation. Finally, the average of these four stations represents the precipitation status of the whole MYC. There were relatively few meteorological stations involved in the XDC, only Xidahe Reservoir.
Digital Elevation Model (DEM) data were downloaded from the Geospatial Data Cloud (http://www.gscloud.cn/), the data type was ASTER GDEM, and the spatial resolution was 30 m, and it was used to extract the catchment.
A change point indicates the starting time of an abrupt change in streamflow and precipitation. The non-parametric Mann-Kendall (M-K) test (Mann 1945; Kendall 1975) was used to detect change points of annual streamflow and precipitation in the XDC and MYC. It is based on the correlation between the rank of a time series and the time order, which can handle non-normalcy with high asymptotic efficiency and is commonly used to analyze the trend and abrupt points in various hydrological and meteorological series. The test is given as follows:
Following the assumption that xi is an independent and identically distributed random variable, the expected value E (Si) and variances var (Si) are given by (Hamed 2008; Bao et al., 2012):
Then, the curve of UFi and UBi can be shown after calculating UBi based on the inverse time series xn, xn-1, … , x1 of UFi and the UBi = - UFi, i = n, n-1, … , 1. The change year can be found if there was an intersection of two curves and the series trend is statistically significant.
In this study, Pearson correlation was used to evaluate the linear correlation between runoff, precipitation and various land use types. The equation is given as follows:
where x, y were the variables, such as runoff and precipitation, and ‾x, ‾y were the mean values of the two variables. The significance level of the correlation was obtained by querying the correlation coefficient table, and n is the number of observations in x and y variables.
In the MYC, the main soil types are Chestnut and Calcareous soil, the soil structure and water permeability are relatively poor, and both types have obvious calcium accumulation and a high content of carbonate, which is not conducive to streamflow leakage; hence any water loss caused by river channel and soil water infiltration can be ignored. Therefore, the XDC rainfall-streamflow linear regression was considered as the reference model to estimate the streamflow consumption of the MYC under farmland expansion. First, the chi-square test was applied to verify whether precipitation, streamflow and land use data are independent of each other, if they are independent of each other, the rainfall-streamflow model of the XDC was established through linear regression. Then the precipitation of the MYC was brought into the model to obtain the streamflow without the effects of farmland reclamation. The streamflow for farmland irrigation was obtained by calculating the difference between the observed streamflow and the estimated streamflow. The specific method is as follows:
where QX and PX are the streamflow and precipitation of the XDC, respectively; a is the regression coefficient and b is the regression intercept. Qestimated and Qobserved are the estimated streamflow and observed streamflow in the MYC, respectively; A is the farmland area during the different periods in the MYC, and Qfarmland is the streamflow for farmland irrigation.
Human activities and climate variability were considered to be the two main factors impacting streamflow. Since in water-limited regions, annual streamflow is more sensitive to precipitation than to other climate factors (Donohue et al., 2011; Liang and Liu, 2014), we applied linear regression to estimate the impacts of precipitation and land use (Koster and Suarez, 1999; Sankarasubramanian et al., 2001; Milly and Dunne, 2002), which was displayed by:
where ∆Q is the streamflow difference before and after the division point, Q1 is the streamflow before the division point, Q2 is the streamflow after the division point; and ∆Qprecipitation and ∆Qland use are the streamflow changes caused by precipitation change and land use change, respectively.
The division points were set based on the corresponding years of existing land use data and 10-year intervals, and a total of seven periods are divided from the initial year. In the study of a period, the years before the division was regarded as pre-division, and the years after the division point and before the next division point were considered as post-division. The streamflow change of the pre-division point was a reference period for considering the initial land use, and the post-division year was a period after the land use change occurred. First, it was assumed that precipitation change had the same effects on streamflow variability in both of the two periods, and that streamflow change was therefore only impacted differently, during the two periods, by land use changes (Zhang et al., 2014; Chen et al., 2020). Hence the relationship (R1) between annual precipitation and annual streamflow at the pre-division point was established by linear regression, and the hypothetical Q,2 (streamflow changes without the effects of land use change) can be determined by substituting the annual precipitation after the division point period into R1. Before applying these assumptions, it was necessary to verify them based on available data. One step was to check whether the change of regression coefficients in pre- and post-division periods was not significant, another step was to check whether any land use class changed significantly than others. If these conditions were true, the assumptions can be applied to the study. Subsequently, separating the impacts of precipitation variability and land use change can be given by:
Finally, the contributions of the two factors can be quantified by:
where Cprecipitation and Cland_use are the contributions of precipitation change and land use change to streamflow variation.
Increasing trends were detected in the XDC and MYC precipitation data collected during the period of 1956–2019 (Figure 2), with change rates of 19.37 mm/10a and 4.33 mm/10a, respectively. The annual mean precipitation amounts were 395.54 mm and 395.28 mm in the two catchments, respectively. The abrupt point of precipitation was determined as 1981 in the XDC, while there was no remarkable abrupt point in the MYC. Increasing trends in precipitation during the period of 1956–1980 (with mean precipitation of 351.81 mm and a change rate of 14.01 mm/10a) were lower than those of the period of 1981–2019 (423.58 mm and 11.09 mm/10a), in the XDC. A significant increasing trend appeared in the annual streamflow of the XDC during 1956–2019, while there was a significant decreasing trend in the MYC (p < 0.05) with streamflow change rates of 0.071 × 108 m3/10a and 0.063 × 108 m3/10a, and annual mean streamflows of 1.68 × 108 m3 and 0.62 × 108 m3 in the XDC and MYC, respectively. In the XDC, the abrupt point of streamflow change was determined as 2003, with the decreasing trends during the period of 1956–2002 (with mean streamflow of 1.55 × 108 m3 and change rate of 0.03 × 108 m3/10a) being lower than those of the 2003–2019 period (2.02 × 108 m3 and 0.21 × 108 m3/10a). In the MYC, the abrupt point of streamflow was considered to be 1991, with the increasing trends during the period of 1956–1990 (with mean streamflow of 0.74 × 108 m3 and change rate of 0.006 × 108 m3/10a) being higher than those of the period of 1991–2019 (0.46 × 108 m3 and 0.008 × 108 m3/10a).
FIGURE 2. Trends of precipitation and streamflow (left column); M-K tests of precipitation and streamflow (right column): (A) precipitation M-K test of MYC; (B) precipitation M-K test of XDC; (C) streamflow M-K test of MYC; (D) streamflow M-K test of XDC. The slope is the regression coefficient of linear regression, which means that streamflow and precipitation change with time.
The dominant land use types in the MYC are farmland and grassland (Figure 3), which together account for more than 80% of the area. The grassland area is the largest, accounting for 58.8% and 55.3% in 1975 and 1985, respectively, while it was less than 50% of the area during 1995–2019. Although the farmland area was larger than forest, water or residential land in the MYC, its proportion ranged from only 21%–37% of the entire area. In the XDC, forest and grassland were the main land uses, accounting for about 58% and 35%, respectively, while only 1% of its area was used for agriculture. A significant change was detected in MYC farmland, grassland and residential (p < 0.05) while the change in forest, water and barren were not significant. And there were two main trends of land use changes in the MYC during 1975–2019: a decrease of grassland and an increase of farmland and construction land. The farmland increased by 181 km2 while the grassland decreased by 187 km2 from 1975 to 2019. The grassland area increased by 13.76 km2 in the XDC, and no other land use types there increased by more than 5 km2. The land conversions in the MYC and XDC were relatively small from 1975 to 2019 (Figure 3), accounting for only 25.9% (300.76 km2) and 12.0% (79.48 km2) of the catchments, respectively. There were four main types of land area change (all more than 5%), of which the grassland conversion area was the largest, reaching 226.5 km2, and accounting for 75.3% of the conversion area. Conversions from forest land and grassland were the main forms of land use change in the XDC. The area converted from forest land to grassland was 23.91 km2, from grassland to forest land, 13.46 km2, which accounted for 30.1% and 16.9% of the area, respectively.
FIGURE 3. Distribution of land use and major land use conversions during 1975–2019 in the MYC and XDC (A), 1975; (B), 1985; (C), 1995; (D), 2005; (E), 2015; (F), 2019; (G), major land use conversions from 1975 to 2019.
Significantly correlated relationships were detected between streamflow and both farmland and grassland in the MYC (Table 1). In particular, there was a significant negative correlation between streamflow and farmland (p < 0.01). However, a significantly correlated positive relationship was detected between streamflow and precipitation in the XDC. Streamflow variation and the contributions of land use change and precipitation change were different during various periods between 1956 and 2019 in the MYC (Figure 4). The streamflow variation was largest during the periods of 1986–1995 and 1996–2005, although during the period of 1996–2005 the streamflow decreased by 14.01 × 106 m3 more than it did during the previous period. And the minimum streamflow change was only 0.92 × 106 m3 in the period of 1966–1975, compared to the other periods. The streamflow variation caused by precipitation change was highest in the period of 2006–2019, especially during 2006–2015 (17.99 × 106 m3), while the highest streamflow difference caused by land use change occurred during the period of 1986–2019, especially in 1996–2005 and 2006–2015, which were 25.19 × 106 m3 and 19.76 × 106 m3, respectively.
FIGURE 4. Streamflow difference before and after division point and contributions of land use change and precipitation change in various periods from 1956 to 2019 in the MYC. (∆Q is the streamflow change based on the comparison of before and after the division point; ∆Qprecipitation and ∆Qland use are the streamflow changes caused by precipitation change and land use change, respectively).
The contributions of precipitation change and land use alteration to streamflow variation were 31.7% and 68.3%, respectively, during the overall study period in the MYC. Land use change first increased streamflow and then decreased it, and the contribution of land use change was more than 60% during the period of 1956–2005, with a maximum of 98.9% during the period of 1976–1985, after which it began to decrease. After 2015, the contribution rate of land use change was less than that of precipitation. The estimated streamflow was highest (162.42 × 106 m3 and 167.43 × 106 m3, respectively) during the periods of 2006–2015 and 2016–2019; the smallest was 156.18 × 106 m3, during the period of 1956–1975 (Figure 5). The biggest differences between estimated and measured streamflow were found during the two periods of 2006–2015 and 2016–2019 (117.36 × 106 m3 and 118.32 × 106 m3), respectively. Streamflow for farmland irrigation first increased and then decreased, from 1956 to 2019, with a change rate of 27.97 × 104 m3/km2. Farmland had the greatest impact on streamflow reduction during the period of 1956–1975 (33.5 × 104 m3/km2). However, the least impact of farmland expansion on streamflow reduction occurred during 1986–1995 (26.07 × 104 m3/km2).
FIGURE 5. Differences (∆Q) between observed streamflow (Qobserved) and estimated streamflow (Qestimated), and streamflow for farmland irrigation (Qfarmland) in various periods during 1956–2019 in the MYC.
Streamflow was generally affected by many factors, such as farmland reclamation, urban expansion, geomorphic properties, climate warming and so on, but on the whole, it is mainly affected by climate change and land use (Cooley et al., 2021; Huo et al., 2021; Su et al., 2021). An opposite trend of streamflow was detected for the MYC and XDC during 1956–2019. We compared the correlations between streamflow and precipitation for these two adjacent small catchments and noticed that the increase of annual precipitation should be the dominant reason for the increase of streamflow in the XDC. Xue (2021) showed that the significant increase in streamflow was mainly affected by the increased precipitation of XDC from 1961 to 2018, which also supported our results. Along with the increased precipitation in northwestern China over the past decades (Qin et al., 2021), the increased temperature caused by global warming is also expected to bring more streamflow from glacier melt (Harper et al., 2012; Winkelmann et al., 2012; Zhang et al., 2017; Smith et al., 2020; Azam et al., 2021; Zhang and Kang, 2021). In contrast to the XDC, the correlation between streamflow and precipitation was no longer significant in the MYC. Our results indicated that significant changes occurred in farmland (the area increased by 15.6%) and grassland (the area decreased by 16%) in the MYC during this period, but the land use change detected in the XDC was almost negligible (the increase in farmland area was less than 1%) compared with that in the MYC. Considering that both catchments have experienced the same climate change background, the different streamflow regimes between the MYC and the XDC appear to have been caused mainly by their different land use histories (Table 1).
By comparing the abrupt change points detected in the measured time series, the above conclusions could be further enhanced. For example, a sharp decrease of about 0.34 × 108 m3 was detected in streamflow of the MYC around 1991. This time was roughly coincident with the implementation of household contract responsibility system (in the 1980s), which stimulated agricultural land reclamation from other types of land use (Huang et al., 2014), and thus reduced streamflow through irrigation-intensive farming (Bao and Fang, 2007; Xie et al., 2018). The cumulative contribution of land-use changes to streamflow reduction in the MYC reached 68% during the past 64 years, and the contribution even exceeded 90% during 1976–1985, when farmland expanded rapidly (Figure 4). A previous study also showed that farmland expansion has been the main cause of streamflow change in the MYC from 1967 to 2000 with a contribution of 77%–80% (Wang et al., 2006). Human activities also have a great impact on streamflow in the other regions of inland river basins of Northwestern China, such as the whole HRB, Taohe Upstream Basin and SRB (Wang et al., 2007; Yang et al., 2017; Xue et al., 2021). Although the contribution of land-use change to streamflow has gradually decreased in recent years due to the policy of returning farmland to forest and grassland, land-use change is still the main reason for streamflow reduction in the MYC, primarily because compensating effects are much more pronounced at smaller scales (Cao et al., 2009; Zhang et al., 2014; Bieger et al., 2015). In the XDC, a sharp increase of about 0.47 × 108 m3 was detected in streamflow around 2003, which was coincident with the implementation of a water diversion project (in 2003), which increased streamflow via input from other rivers. These shifts also prove that land use history has a great impact on streamflow variations. This mutation time was also consistent with Xue’s result (Xue et al., 2021), which confirmed the reliability of our results.
Although the general global climate change trend is toward warmer and wetter weather, and glacier melt has accelerated in the Qilian Mountains (Guo et al., 2018), there is no obvious response of streamflow change in the XDC, which further indicates that other factors besides human activities also affect this result, such as permafrost coverage, catchment elevation and groundwater level (Niu et al., 2011; He et al., 2019). Viola et al. (2017) pointed out that the amount of rainfall converted into streamflow in a high-altitude catchment is higher than that in a low-altitude catchment, which indicates that the XDC, with an average altitude of 3465 m, has great potential for converting precipitation into streamflow. In addition, the groundwater level rose under the influence of the policy of prohibiting overexploitation of groundwater, reducing streamflow infiltration of the XDC and maintaining a normal circulation of surface water (Hochmuth et al., 2015; Huang et al., 2017). Moreover, the proportion of grassland and forest land was considered as the main factor affecting the annual streamflow of basins in temperate continental climate regions (Huo et al., 2021), and the XDC, with a total proportion of grassland and forest land accounting for 93% of the area, may not be greatly affected by the warming and wetting climate. All of these influences affect streamflow variations and increase the complexity of hydrological processes, and indicate that human activities and climate change play different roles in different regions and at different scales.
Although we quantified the impacts of precipitation change and land use change on streamflow, uncertainties remain in the assessment of their respective contributions. Uncertainties could be induced by data representation. For example, the sparsely distributed stations may ignore the hydrological processes in upstream of MYC. Considering that Biandoukou was close to MYC and its altitude was similar to that of the upstream of MYC, it is acceptable to replace the upstream precipitation from this meteorological station when there is no suitable data available. The hydrological data and land use data are not well matched in terms of their spatial scales and resolution. The hydrological data (i.e., precipitation and streamflow) were collected at individual stations, while the land use data were extracted from satellite images (Zhu et al., 2018; Duan et al., 2021). Given that the evaluation of streamflow and land use change was largely based on trend analysis rather than data points, the errors introduced by the data resolution could be largely ignored. Multi-sourced data (i.e., Landsat data, products of land use in the Qilian Mountains) were used to classify land use. In addition, we assumed that streamflow change is only influenced by land use and precipitation, the effect of evapotranspiration and other climate variables may not be representative. This assumption also may not be applicable to catchments with large land area, low gradient, slow water flows and rich water, but it could be considered plausible for the XDC and MYC, with their high gradients and limited water resources, because annual streamflow is more sensitive to precipitation than to evapotranspiration in these regions (Donohue et al., 2011; Berghuijs et al., 2016; Zhang et al., 2021). Moreover, in the water consumed by human activities, only farmland irrigation was considered, while domestic and industrial production use were neglected, which may have led to calculation errors in streamflow consumption. Considering, however, that the proportions of industrial production and domestic water use in these catchments are small and mainly come from groundwater, these errors could be largely ignored.
Streamflow guarantees regional ecosystem stability and ecological protection in arid northwestern China (Dey and Mishra, 2017; Palmer and Ruhi, 2019). But streamflow also serves as the primary source of irrigation water for agriculture (which in turn is the dominant source of livelihood in arid regions like the study area). Because each of the systems, including vegetation, water, soil and atmosphere, will be affected in a variety of ways by reduced streamflow, if too much water is used for irrigation and streamflow continues to decrease, irreversible ecological disasters are probable, although rarely does one know just when such an event will happen (Palmer and Ruhi, 2019; Morganstein and Ursano, 2020). Balancing water use between human food production and the needs of the environment is extremely important for local communities and governments, but also complex and difficult to achieve in practice (Qin et al., 2022). Thus, an explicitly formulated strategy for streamflow forecasting and management is needed, especially in the climate change context. In streamflow management, water allocation among various ecosystems should be coordinated to prevent excessive water use in any given ecosystem, especially in agriculture, which may reduce the water available for natural vegetation, causing desertification (Satge et al., 2019). It is necessary to find the threshold of ecological water demand in different regions, to realize the maximum benefit by using the minimum amount of water for human needs. And the water resources should be reasonably allocated in the upper, middle and lower reaches of a river, through a series of policies and the coordination of various departments, to alleviate the contradiction between the supply and demand of water resources and prevent downstream interruption caused by excessive water use in the upper and middle reaches.
In the context of global climate change, the uncertainty of precipitation and snowmelt in arid northwestern China leads to instability in streamflow, which in turn could lead to an increase in the number of extreme events such as floods and droughts, posing a threat to both the natural environment and human life (Lesk et al., 2016; Fereidoon and Koch, 2018; Duan et al., 2022). For example, drought, flood and unpredictable rainfall distribution threaten food security by increasing the uncertainty of agricultural production, and an increase in drought frequency and intensity will increase desertification and lead to serious land degradation (Akbari et al., 2020; Qin et al., 2022). Although climate change is not considered to be the main driving factor of streamflow variation in some regions, glacier retreats and the melting of previously frozen soil caused by climate change have important impacts on streamflow regulation (Niu et al., 2011; He et al., 2019). The reduction of large-scale snow cover will aggravate spring droughts in the future, and the reliability of snow melt for river runoff will be greatly reduced (Qi et al., 2022). Therefore, the multi-scale variation characteristics of hydrological processes such as precipitation, streamflow and the melting of alpine snow and glaciers should be the focus of future research, to increase the accuracy of predictions and help humans prepare for possible water insecurity, in the future. In addition, vegetation can play an important role in ameliorating streamflow instability (Zhang et al., 2012; Aires et al., 2020; Huo et al., 2021). The impact of vegetation patterns and spatial allocation modes, on the temporal and spatial variation of streamflow, should be studied, to regulate the dynamic process of streamflow. The possible impact of the construction of large-scale restoration projects on the decrease of natural streamflow in arid Northwest China should be considered. One suggestion is that vegetation restoration should focus on restoring natural areas, and that artificial vegetation restoration projects should be properly controlled (Zastrow, 2019). Appropriate vegetation coverage, matching the sustainable utilization of water and soil resources in the basin, should be based on the water-carrying capacity, to give full play to the ecological, economic and social benefits of vegetation.
In this study, hydrological and satellite data were used to compare the streamflow variations, the contributions of precipitation change and land use alteration to the streamflow, and the water consumption attributable to farmland expansion for two adjacent basins in the Qilian Mountains, in northwestern China. Opposite trends in streamflow were detected in the two catchments during the period of 1956–2019; the streamflow in the MYC decreased significantly (-0.63 × 107m3/10a) while it increased in the XDC (0.71 × 107m3/10a). A sharp decrease of about 0.34 × 108 m3 in streamflow was detected in the MYC around 1991, while an abrupt increase of about 0.47 × 108 m3 was detected in the XDC around 2003. The land conversions within the MYC were relatively small, accounting for only 25.9% (300.76 km2) of the streamflow changes in the catchment, yet land conversion was the dominant reason for streamflow reduction in the MYC, as its contribution reached 68.3%. The streamflow consumed by farmland irrigation first increased and then decreased, from 1956 to 2019, with a change rate of 27.97 × 104 m3/km2. The greatest impact of farmland irrigation on streamflow occurred during 1956–1975 (33.5 × 104 m3/km2). In the XDC, however, changes in precipitation were confirmed to be the dominant factor driving the increase of streamflow. We conclude that although streamflow is affected by both human activities and climate change, these influences vary greatly among different regions. The relationship between the intensity of human activities and the availability of water resources should be studied more closely, and anthropogenic stressors and water resource allocation should be coordinated to maintain water resource security and prevent regional water resource crises.
The original contributions presented in the study are included in the article/Supplementary Material, further inquiries can be directed to the corresponding authors.
XB, WZ, HL, and YZ contributed to conception and writing. QY, JL, and XC interpreted the data. XB wrote the first draft of the manuscript. All authors contributed to manuscript revision, read, and approved the submitted version. WZ and HL received funding for the project detailed in the Funding.
This work was jointly supported by the Strategic Priority Research Program of the Chinese Academy of Sciences (XDA2003010102), the National Key Research and Development Program of China (2019YFC0507400), and the National Natural Science Foundation of China (42171117).
The authors declare that the research was conducted in the absence of any commercial or financial relationships that could be construed as a potential conflict of interest.
The reviewer CZ declared a shared affiliation with the author XC to the handling editor at time of review.
All claims expressed in this article are solely those of the authors and do not necessarily represent those of their affiliated organizations, or those of the publisher, the editors and the reviewers. Any product that may be evaluated in this article, or claim that may be made by its manufacturer, is not guaranteed or endorsed by the publisher.
Aires, U. R. V., da Silva, D. D., Moreira, M. C., Ribeiro, C. A. A. S., and Ribeiro, C. B. M. (2020). The use of the normalized difference vegetation index to analyze the influence of vegetation cover changes on the streamflow in the manhuacu River Basin, Brazil. Water Resour. Manag. 34 (6), 1933–1949. doi:10.1007/s11269-020-02536-1
Akbari, M., Shalamzari, M. J., Memarian, H., and Gholami, A. (2020). Monitoring desertification processes using ecological indicators and providing management programs in arid regions of Iran. Ecol. Indic. 111, 106011. doi:10.1016/j.ecolind.2019.106011
Anache, J. A. A., Flanagan, D. C., Srivastava, A., and Wendland, E. C. (2018). Land use and climate change impacts on runoff and soil erosion at the hillslope scale in the Brazilian Cerrado. Sci. Total Environ. 622, 140–151. doi:10.1016/j.scitotenv.2017.11.257
Arora, V. K. (2001). Streamflow simulations for continental-scale river basins in a global atmospheric general circulation model. Adv. Water Resour. 24 (7), 775–791. doi:10.1016/s0309-1708(00)00078-6
Asadieh, B., and Krakauer, N. Y. (2015). Global trends in extreme precipitation: Climate models versus observations. Hydrol. Earth Syst. Sci. 19 (2), 877–891. doi:10.5194/hess-19-877-2015
Azam, M. F., Kargel, J. S., Shea, J. M., Nepal, S., Haritashya, U. K., Srivastava, S., et al. (2021). Glaciohydrology of the himalaya-karakoram. Science 373 (6557), eabf3668. doi:10.1126/science.abf3668
Bao, C., and Fang, C. (2007). Water resources constraint force on urbanization in water deficient regions: A case study of the hexi corridor, arid area of NW China. Ecol. Econ. 62, 508–517. doi:10.1016/j.ecolecon.2006.07.013
Bao, Z. X., Zhang, J. Y., Wang, G. Q., Fu, G. B., He, R. M., Yan, X. L., et al. (2012). Attribution for decreasing streamflow of the Haihe River basin, northern China: Climate variability or human activities? J. Hydrol. 460, 117–129. doi:10.1016/j.jhydrol.2012.06.054
Beck, H. E., Roo, A. D., and Dijk, A. V. (2015). Global maps of streamflow characteristics based on observations from several thousand catchments. J. Hydrometeorol. 16 (4), 1478–1501. doi:10.1175/JHM-D-14-0155.1
Berghuijs, W. R., Woods, R. A., Hutton, C. J., and Sivapalan, M. (2016). Dominant flood generating mechanisms across the United States. Geophys. Res. Lett. 43 (9), 4382–4390. doi:10.1002/2016gl068070
Bieger, K., Hoermann, G., and Fohrer, N. (2015). The impact of land use change in the Xiangxi Catchment (China) on water balance and sediment transport. Reg. Envir. Chang. 15 (3), 485–498. doi:10.1007/s10113-013-0429-3
Brath, A., Montanari, A., and Moretti, G. (2006). Assessing the effect on flood frequency of land use change via hydrological simulation (with uncertainty). J. Hydrol. 324 (1-4), 141–153. doi:10.1016/j.jhydrol.2005.10.001
Cao, W., Bowden, W. B., Davie, T., and Fenemor, A. (2009). Modelling impacts of land cover change on critical water resources in the motueka river catchment, New Zealand. Water Resour. Manag. 23 (1), 137–151. doi:10.1007/s11269-008-9268-2
Chen, H., Fleskens, L., Baartman, J., Wang, F., Moolenaar, S., and Ritsema, C. (2020). Impacts of land use change and climatic effects on streamflow in the Chinese loess plateau: A meta-analysis. Sci. Total Environ. 703, 134989. doi:10.1016/j.scitotenv.2019.134989
Chen, Y., Zhi, L., Fan, Y., Wang, H., and Deng, H. (2015). Progress and prospects of climate change impacts on hydrology in the arid region of Northwest China. Environ. Res. 139, 11–19. doi:10.1016/j.envres.2014.12.029
Cheng, Z., and Yu, B. (2019). Effect of land clearing and climate variability on streamflow for two large basins in Central Queensland, Australia. J. Hydrol. 578, 124041. doi:10.1016/j.jhydrol.2019.124041
Cooley, S. W., Ryan, J. C., and Smith, L. C. (2021). Human alteration of global surface water storage variability. Nature 591 (7848), 78–81. doi:10.1038/s41586-021-03262-3
Darvini, G., and Memmola, F. (2020). Assessment of the impact of climate variability and human activities on the runoff in five catchments of the Adriatic Coast of south-central Italy. J. Hydrol.-Reg. Stud. 31, 100712. doi:10.1016/j.ejrh.2020.100712
Dey, P., and Mishra, A. (2017). Separating the impacts of climate change and human activities on streamflow: A review of methodologies and critical assumptions. J. Hydrol. 548, 278–290. doi:10.1016/j.jhydrol.2017.03.014
Donohue, R. J., Roderick, M. L., and McVicar, T. R. (2011). Assessing the differences in sensitivities of runoff to changes in climatic conditions across a large basin. J. Hydrol. 406 (3-4), 234–244. doi:10.1016/j.jhydrol.2011.07.003
Duan, W., Maskey, S., Chaffe, P., Luo, P., He, B., Wu, Y., et al. (2021). Recent advancement in remote sensing technology for hydrology analysis and water resources management. Remote Sens. 13 (6), 1097. doi:10.3390/rs13061097
Duan, W., Zou, S., Christidis, N., Schaller, N., Chen, Y., Sahu, N., et al. (2022). Changes in temporal inequality of precipitation extremes over China due to anthropogenic forcings. npj Clim. Atmos. Sci. 5 (1), 33–13. doi:10.1038/s41612-022-00255-5
Fenta, A. A., Yasuda, H., Shimizu, K., and Haregeweyn, N. (2017). Response of streamflow to climate variability and changes in human activities in the semiarid highlands of northern Ethiopia. Reg. Envir. Chang. 17 (4), 1229–1240. doi:10.1007/s10113-017-1103-y
Fereidoon, M., and Koch, M. (2018). SWAT-MODSIM-PSO optimization of multi-crop planning in the Karkheh River Basin, Iran, under the impacts of climate change. Sci. Total Environ. 630, 502–516. doi:10.1016/j.scitotenv.2018.02.234
Gao, P., Li, P., Zhao, B., Xu, R., Zhao, G., Sun, W., et al. (2017). Use of double mass curves in hydrologic benefit evaluations. Hydrol. Process. 31 (26), 4639–4646. doi:10.1002/hyp.11377
Guo, H., Bao, A., Liu, T., Jiapaer, G., Ndayisaba, F., Jiang, L., et al. (2018). Spatial and temporal characteristics of droughts in Central Asia during 1966-2015. Sci. Total Environ. 624, 1523–1538. doi:10.1016/j.scitotenv.2017.12.120
Guzha, A. C., Rufino, M. C., Okoth, S., Jacobs, S., and Nóbrega, R. (2018). Impacts of land use and land cover change on surface runoff, discharge and low flows: Evidence from East Africa. J. Hydrol.-Reg. Stud. 15, 49–67. doi:10.1016/j.ejrh.2017.11.005
Hamed, K. H. (2008). Trend detection in hydrologic data: The Mann-Kendall trend test under the scaling hypothesis. J. Hydrol. 349 (3-4), 350–363. doi:10.1016/j.jhydrol.2007.11.009
Harper, J., Humphrey, N., Pfeffer, W. T., Brown, J., and Fettweis, X. (2012). Greenland ice-sheet contribution to sea-level rise buffered by meltwater storage in firn. Nature 491 (7423), 240–243. doi:10.1038/nature11566
He, Y., Jiang, X., Wang, N., Zhang, S., Ning, T., Zhao, Y., et al. (2019). Changes in mountainous runoff in three inland river basins in the arid Hexi Corridor, China, and its influencing factors. Sust. Cities Soc. 50, 101703. doi:10.1016/j.scs.2019.101703
Hochmuth, H., Thevs, N., and He, P. (2015). Water allocation and water consumption of irrigation agriculture and natural vegetation in the Heihe River watershed, NW China. Environ. Earth Sci. 73 (9), 5269–5279. doi:10.1007/s12665-014-3773-9
Huang, S., Zhou, L., Chen, Y., and Lu, H. (2014). Impacts of polices on eco - environment of Minqin county during the past 60 years. J. Arid. Land Resour. Environ. 28 (7), 73–78.
Huang, S., Zhou, L., Feng, Q., Lu, Z., and Xiao, J. (2017). Evaluation of eco-environment effects of management policy implementing in inland River Basin of China: A case in the minqin oasis. J. Desert Res. 37 (3), 580–586.
Huo, J., Liu, C., Yu, X., Jia, G., and Chen, L. (2021). Effects of watershed char and climate variables on annual runoff in different climatic zones in China. Sci. Total Environ. 754, 142157. doi:10.1016/j.scitotenv.2020.142157
Koster, R. D., and Suarez, M. J. (1999). A simple framework for examining the interannual variability of land surface moisture fluxes. J. Clim. 12 (7), 1911–1917. doi:10.1175/1520-0442(1999)012<1911:asffet>2.0.co;2
Lebek, K., Senf, C., Frantz, D., Monteiro, J. A. F., and Krueger, T. (2019). Interdependent effects of climate variability and forest cover change on streamflow dynamics: A case study in the upper umvoti River Basin, south Africa. Reg. Envir. Chang. 19 (7), 1963–1971. doi:10.1007/s10113-019-01521-8
Lesk, C., Rowhani, P., and Ramankutty, N. (2016). Influence of extreme weather disasters on global crop production. Nature 529 (7584), 84–87. doi:10.1038/nature16467
Liang, L., and Liu, Q. (2014). Streamflow sensitivity analysis to climate change for a large water-limited basin. Hydrol. Process. 28 (4), 1767–1774. doi:10.1002/hyp.9720
Liu, J., Zhang, Q., Singh, V. P., and Shi, P. (2017). Contribution of multiple climatic variables and human activities to streamflow changes across China. J. Hydrol. 545, 145–162. doi:10.1016/j.jhydrol.2016.12.016
Mann, H. B. (1945). Nonparametric tests against trend. Econometrica 13, 245–259. doi:10.2307/1907187
Milly, P. C. D., and Dunne, K. A. (2002). Macroscale water fluxes - 2. Water and energy supply control of their interannual variability. Water Resour. Res. 38 (10), 24-1–24-9. doi:10.1029/2001wr000760
Morganstein, J. C., and Ursano, R. J. (2020). Ecological disasters and mental health: Causes, consequences, and interventions. Front. Psychiatry 11 (1), 1. doi:10.3389/fpsyt.2020.00001
Ning, T., Feng, Q., Li, Z., and Li, Z. (2020). Recent changes in climate seasonality in the inland river basin of Northwestern China. J. Hydrol. 590, 125212. doi:10.1016/j.jhydrol.2020.125212
Niu, L., Ye, B., Li, J., and Sheng, Y. (2011). Effect of permafrost degradation on hydrological processes in typical basins with various permafrost coverage in Western China. Sci. China Ser. D-Earth Sci. 54 (4), 615–624. doi:10.1007/s11430-010-4073-1
Pall, P., Aina, T., Stone, D. A., Stott, P. A., Nozawa, T., Hilberts, A. G. J., et al. (2011). Anthropogenic greenhouse gas contribution to flood risk in England and Wales in autumn 2000. Nature 470 (7334), 382–385. doi:10.1038/nature09762
Palmer, M., and Ruhi, A. (2019). Linkages between flow regime, biota, and ecosystem processes: Implications for river restoration. Science 365 (6459), eaaw2087. doi:10.1126/science.aaw2087
Piao, S., Ciais, P., Huang, Y., Shen, Z., Peng, S., Li, J., et al. (2010). The impacts of climate change on water resources and agriculture in China. Nature 467 (7311), 43–51. doi:10.1038/nature09364
Pourmokhtarian, A., Driscoll, C. T., Campbell, J. L., Hayhoe, K., Stoner, A. M. K., Adams, M. B., et al. (2017). Modeled ecohydrological responses to climate change at seven small watersheds in the northeastern United States. Glob. Change Biol. 23(2), 840–856. doi:10.1111/gcb.13444
Qi, W., Feng, L., Yang, H., and Liu, J. (2022). Warming winter, drying spring and shifting hydrological regimes in Northeast China under climate change. J. Hydrol. 606, 127390. doi:10.1016/j.jhydrol.2021.127390
Qin, J., Duan, W., Chen, Y., Dukhovny, V. A., Sorokin, D., Li, Y., et al. (2022). Comprehensive evaluation and sustainable development of water–energy–food–ecology systems in Central Asia. Renew. Sust. Energ. Rev. 157, 112061. doi:10.1016/j.rser.2021.112061
Qin, J., Su, B., Tao, H., Wang, Y., Huang, J., Li, Z., et al. (2021). Spatio-temporal variations of dryness/wetness over Northwest China under different SSPs-RCPs. Atmos. Res. 259, 105672. doi:10.1016/j.atmosres.2021.105672
Rangecroft, S., Van Loon, A. F., Coxon, G., Breña-Naranjo, J. A., Van Ogtrop, F., and Van Lanen, H. A. J. (2019). Using paired catchments to quantify the human influence on hydrological droughts. Hydrol. Earth Syst. Sci. 23 (3), 1725–1739. doi:10.5194/hess-23-1725-2019
Rust, W., Corstanje, R., Holman, I. P., and Milne, A. E. (2014). Detecting land use and land management influences on catchment hydrology by modelling and wavelets. J. Hydrol. 517, 378–389. doi:10.1016/j.jhydrol.2014.05.052
Sankarasubramanian, A., Vogel, R. M., and Limbrunner, J. F. (2001). Climate elasticity of streamflow in the United States. Water Resour. Res. 37 (6), 1771–1781. doi:10.1029/2000wr900330
Satge, F., Hussain, Y., Xavier, A., Zola, R. P., Salles, L., Timouk, F., et al. (2019). Unraveling the impacts of droughts and agricultural intensification on the Altiplano water resources. Agric. For. Meteorol. 279, 107710. doi:10.1016/j.agrformet.2019.107710
Senbeta, T. B., and Romanowicz, R. J. (2021). The role of climate change and human interventions in affecting watershed runoff responses. Hydrol. Process. 35, e14448. doi:10.1002/hyp.14448
Sharma, P. J., Patel, P. L., and Jothiprakash, V. (2019). Impact of rainfall variability and anthropogenic activities on streamflow changes and water stress conditions across Tapi Basin in India. Sci. Total Environ. 687, 885–897. doi:10.1016/j.scitotenv.2019.06.097
Shen, Y., Li, S., Chen, Y., Qi, Y., and Zhang, S. (2013). Estimation of regional irrigation water requirement and water supply risk in the arid region of Northwestern China 1989-2010. Agric. Water Manage. 128, 55–64. doi:10.1016/j.agwat.2013.06.014
Smith, B., Fricker, H. A., Gardner, A. S., Medley, B., Nilsson, J., Paolo, F. S., et al. (2020). Pervasive ice sheet mass loss reflects competing ocean and atmosphere processes. Science 368 (6496), 1239–1242. doi:10.1126/science.aaz5845
Su, X., Li, X., Niu, Z., Wang, N. a., and Liang, X. (2021). A new complexity-based three-stage method to comprehensively quantify positive/negative contribution rates of climate change and human activities to changes in runoff in the upper Yellow River. J. Clean. Prod. 287, 125017. doi:10.1016/j.jclepro.2020.125017
Viola, F., Caracciolo, D., Forestieri, A., Pumo, D., and Noto, L. V. (2017). Annual runoff assessment in arid and semiarid Mediterranean watersheds under the Budyko's framework. Hydrol. Process. 31 (10), 1876–1888. doi:10.1002/hyp.11145
Wang, G., Liu, J., Kubota, J., and Chen, L. (2007). Effects of land-use changes on hydrological processes in the middle basin of the Heihe River, northwest China. Hydrol. Process. 21 (10), 1370–1382. doi:10.1002/hyp.6308
Wang, G., Zhang, Y., Liu, G., and Chen, L. (2006). Impact of land-use change on hydrological processes in the Maying River basin, China. Sci. China Ser. D-Earth Sci. 49 (10), 1098–1110. doi:10.1007/s11430-006-1098-6
Williamson, T. N., and Barton, C. D. (2020). Hydrologic modeling to examine the influence of the forestry reclamation approach and climate change on mineland hydrology. Sci. Total Environ. 743 (15), 140605. doi:10.1016/j.scitotenv.2020.140605
Winkelmann, R., Levermann, A., Martin, M. A., and Frieler, K. (2012). Increased future ice discharge from Antarctica owing to higher snowfall. Nature 492 (7428), 239–242. doi:10.1038/nature11616
Xie, Y., Bie, Q., Lu, H., and He, L. (2018). Spatio-temporal changes of oases in the hexi corridor over the past 30 years. Sustainability 10 (12), 4489. doi:10.3390/su10124489
Xue, D. (2021). Attribution analysis of runoff changes in the Shiyang River Basin. Gansu, China: Northwest Normal University. doi:10.27410/d.cnki.gxbfu.2021.000174
Xue, D., Zhou, J., Zhao, X., Liu, C., Wei, W., Yang, X., et al. (2021). Impacts of climate change and human activities on runoff change in a typical arid watershed, NW China. Ecol. Indic. 121, 107013. doi:10.1016/j.ecolind.2020.107013
Yang, H., Qi, J., Xu, X., Yang, D., and Lv, H. (2014). The regional variation in climate elasticity and climate contribution to runoff across China. J. Hydrol. 517, 607–616. doi:10.1016/j.jhydrol.2014.05.062
Yang, L., Feng, Q., Yin, Z., Wen, X., Si, J., Li, C., et al. (2017). Identifying separate impacts of climate and land use/cover change on hydrological processes in upper stream of Heihe River, Northwest China. Hydrol. Process. 31 (5), 1100–1112. doi:10.1002/hyp.11098
Zastrow, M. (2019). China’s tree-planting drive could falter in a warming world. Nature 573 (7775), 474–475. doi:10.1038/d41586-019-02789-w
Zhai, R., and Tao, F. (2017). Contributions of climate change and human activities to runoff change in seven typical catchments across China. Sci. Total Environ. 605, 219–229. doi:10.1016/j.scitotenv.2017.06.210
Zhang, A., Zheng, C., Wang, S., and Yao, Y. (2015). Analysis of streamflow variations in the Heihe River Basin, northwest China: Trends, abrupt changes, driving factors and ecological influences. J. Hydrol.-Reg. Stud. 3, 106–124. doi:10.1016/j.ejrh.2014.10.005
Zhang, F., Zhang, M., Wang, S., Qiang, F., Che, Y., and Wang, J. (2017). Evaluation of the tourism climate in the hexi corridor of northwest China's Gansu Province during 1980-2012. Theor. Appl. Climatol. 129 (3-4), 901–912. doi:10.1007/s00704-016-1814-x
Zhang, L., Moges, E., Kirchner, J. W., Coda, E., Liu, T., Wymore, A. S., et al. (2021). Chosen: A synthesis of hydrometeorological data from intensively monitored catchments and comparative analysis of hydrologic extremes. Hydrol. Process. 35 (11). doi:10.1002/hyp.14429
Zhang, L., Podlasly, C., Ren, Y., Feger, K.-H., Wang, Y., and Schwaerzel, K. (2014). Separating the effects of changes in land management and climatic conditions on long-term streamflow trends analyzed for a small catchment in the Loess Plateau region, NW China. Hydrol. Process. 28 (3), 1284–1293. doi:10.1002/hyp.9663
Zhang, M., Wei, X., Sun, P., and Liu, S. (2012). The effect of forest harvesting and climatic variability on runoff in a large watershed: The case study in the Upper Minjiang River of Yangtze River basin. J. Hydrol. 464, 1–11. doi:10.1016/j.jhydrol.2012.05.050
Zhang, Q., and Kang, S. (2021). Glacier elevation change in the Western Qilian mountains as observed by TerraSAR-X/TanDEM-X images. Geocarto Int. 36 (12), 1365–1377. doi:10.1080/10106049.2019.1648563
Zhong, B., and Jue, K. (2019). Land cover dataset with 30m spatial resolution over Qilian Mountain area (1985-2017) V1.0. Beijing, China. National Tibetan Plateau Data Center. doi:10.11888/Geogra.tpdc.270130
Keywords: streamflow, inland river basin, northwestern China, land use alteration, precipitation change
Citation: Bai X, Zhao W, Liu H, Zhang Y, Yang Q, Liu J and Chang X (2023) Effects of precipitation changes and land-use alteration on streamflow: A comparative analysis from two adjacent catchments in the Qilian Mountains, arid northwestern China. Front. Environ. Sci. 11:1097049. doi: 10.3389/fenvs.2023.1097049
Received: 13 November 2022; Accepted: 17 January 2023;
Published: 07 February 2023.
Edited by:
Yiping Wu, Xi’an Jiaotong University, ChinaReviewed by:
Chunhua Zhang, Ludong University, ChinaCopyright © 2023 Bai, Zhao, Liu, Zhang, Yang, Liu and Chang. This is an open-access article distributed under the terms of the Creative Commons Attribution License (CC BY). The use, distribution or reproduction in other forums is permitted, provided the original author(s) and the copyright owner(s) are credited and that the original publication in this journal is cited, in accordance with accepted academic practice. No use, distribution or reproduction is permitted which does not comply with these terms.
*Correspondence: Wenzhi Zhao, emhhb3d6aEBsemIuYWMuY24=; Hu Liu, bGhheXoubGhAZ21haWwuY29t
Disclaimer: All claims expressed in this article are solely those of the authors and do not necessarily represent those of their affiliated organizations, or those of the publisher, the editors and the reviewers. Any product that may be evaluated in this article or claim that may be made by its manufacturer is not guaranteed or endorsed by the publisher.
Research integrity at Frontiers
Learn more about the work of our research integrity team to safeguard the quality of each article we publish.