- 1Department of Agricultural and Consumer Economics, University of Illinois Urbana-Champaign, Champaign, IL, United States
- 2Department of Agricultural and Consumer Economics, University of Illinois Urbana-Champaign, Champaign, IL, United States
- 3School of Civil and Environmental Engineering, University of Oklahoma, Norman, OK, United States
Introduction: Recent work examining the impact of climate-change induced extremes on food-energy-water systems (FEWS) estimates the potential changes in physical flows of multiple elements of the systems. Climate adaptation decisions can involve tradeoffs between different system outcomes. Thus, it is important for decision makers to consider the potential changes in monetary value attributed to the observed changes in physical flows from these events, since the value to society of a unit change in an outcome varies widely between thing like food and energy production, water quality, and carbon sequestration.
Methods: We develop a valuation tool (FEWSVT) that applies theoretically sound valuation techniques to estimates changes in value for four parameters within the food-energy-water nexus. We demonstrate the utility of the tool through the application of a case study that analyzes the monetary changes in value of a modelled heat wave scenario relative to historic (baseline) conditions in two study regions in the United States.
Results: We find that food (corn and soybeans) comprises the majority (89%) of total changes in value, as heatwaves trigger physical changes in corn and soybeans yields. We also find that specifying overly simplified and incorrect valuation methods lead to monetary values that largely differ from FEWSVT results that use accepted valuation methods.
Discussion: These results demonstrate the value in considering changes in monetary value instead of just physical flows when making decisions on how to distribute investments and address the many potential impacts of climate change-induced extremes.
1 Introduction
New work studies the impacts of climate change and climate-induced extremes on physical features of a linked food, energy, water system (C-FEWS) (Vörösmarty et al., 2023; this issue). In order to use that research to guide future policy and infrastructure decisions, decision makers need to understand what tradeoffs exist between elements of the system (Parkinson et al., 2019) and what those tradeoffs mean in terms of human wellbeing (Antle and Capalbo 2010). Economic valuation methods have been developed for several elements of food-energy-water systems; examples include crop production, air pollutant emissions, and water quality benefits (Alston et al., 1998; Muller and Mendelsohn, 2007; Johnston et al., 2017); these methods have been sufficiently validated to be used extensively by the US Environmental Protection Agency in formal cost-benefit analysis of proposed regulations (Petrolia et al., 2021).
Research shows how estimating the monetized values of changes in multiple ecosystem service flows can enable meaningful comparison between them and guide decisions about policy and management. For example, Naime et al. (2020) find that forest restoration yields greater total benefits if it prioritizes regulating over provisioning services because the value of carbon sequestration can be twice as large as the use values people gain from forest use. However, there currently exists no resource that consolidates methods for valuing multiple C-FEWS elements into one tool for researchers to use. This study addresses that gap by developing a valuation tool that harnesses several elements of economics methodology to estimate the monetized social value of changes in five C-FEWS portfolio elements from user-provided data. The resulting instrument empowers scientists who are not experts in economics to carry out important ecosystem service valuation of common features of FEWS. We then harness the functionality of the tool to identify several core features of changes in multiple ecosystem services from climate changes.
This paper describes the methodological foundations for a C-FEWS Valuation (FEWSVT) tool that produces valid rapid-response estimates of the value to society of changes in food-crop yields, water quality, electricity generation, carbon sequestration, and air quality. The tool is spatially and temporally flexible and accommodates any user-defined alternative scenarios. The analyses use two different types of economic valuation approaches. Microeconomic market analysis (Alston et al., 1998; Manning and Ando 2022) is used to estimate changes in monetized consumer and producer surplus for crops and electricity when a climate shock changes production costs by altering crop yields and increasing input costs. In contrast, the values of changes in air quality, water quality, and net carbon flux are estimated using non-market valuation methods (Hanley and Czajkowski 2019; Bateman and Kling 2020) that estimate society’s willingness to pay (WTP) for improvements in environmental quality.
We then explore several important principles of C-FEWS valuation by applying this tool to data representing hypothetical but plausible climate changes in the Northeastern and Midwestern United States. We show which changes in climate change-attributable physical flows are likely to have the largest monetary impacts to society. Finally, we show the importance of using theoretically sound economic methods for valuation rather than simple products of market prices and quantities of goods produced.
2 Materials and methods
2.1 C-FEWS valuation (FEWSVT) tool
As the world continues to grow in population and economic development, demand for elements of the food-energy-water (FEW) nexus will continue to strain the engineered and natural infrastructure on which these elements depend. Global crop demand is projected to double from current levels by 2050, while global water demand is estimated to rise by 20%–30% per year by 2050 (Tilman et al., 2011; WWAP & UN-Water, 2018). Research on FEW systems has fueled a push to develop market and non-market valuation methods that monetize the economic ramifications of scenarios on the FEW nexus.
Previous research has refined and validated methods to estimate the social value of changes in food-energy-water system (FEWS) elements. For example, food production has been studied extensively to examine how a certain change influences crop production, which in turn can be monetized using market data. Examples include the effect of irrigation on food supply (Jin et al., 2012; Xu et al., 2020), the impacts of climate change shocks on crop yields (Islam et al., 2012; Lobell et al., 2013; Nelson et al., 2014; Adhikari et al., 2015; Niyogi et al., 2015), and the effects of farm subsidies on a crop’s productivity levels (Unisfera International Centre 2003; Lencucha et al., 2020). Research has also monetized the impacts of scenario changes on electricity generation per sector using price and cost data. Fonseca et al. (2021) evaluated the impacts of climate change on the electric power sector in the Southeast region of the US, and provided monetary estimates as to the increase in investment costs that would result from accounting for climate change effects on both supply and demand. Other studies have examined how climate change conditions impact specific generating units (Mideksa and Kallbekken 2010; Solaun and Cerda, 2019).
Much research in economics has also developed and validated tools for quantifying FEWS portfolio elements whose impacts are not reflected through a market mechanism like price. Both air pollutant emissions and water quality have effects on human health and wellbeing that are monetized with non-market valuation techniques. Primary non-market valuation methods such as hedonic pricing and travel cost studies can be used to capture the values people place on an ecosystem service. Benefit transfer methods can take values estimated for environmental goods in one place with primary research and apply them to estimate the values of similar goods in other settings. For air pollutant emissions, integrated assessment models (IAM) have been developed to estimate marginal damages associated with rising power plant-level emissions (Muller and Mendelsohn, 2007; Heo et al., 2016a; U.S. Environmental Protection Agency, 2018). The policy consequences of changing power plant-level emissions have also been examined with respect to the transportation industry (Holland et al., 2020).
Research examining the value of water quality improvements ranges from site-specific studies valuing individuals’ WTP to use a clean water body for one specific use to more holistic studies using benefit transfer methods to quantify use and non-use values from restoring the water quality of a range of water bodies (Houtven et al., 2007; Kauffman 2019; Johnston and Bauer 2020). Recent literature has also developed valuation methods to quantify the “social costs of nitrogen” (SCN), which is a spatially explicit measure of the monetary damages of nitrogen release to nearby surface waters from fertilizer application (Keeler et al., 2016; Gourevitch et al., 2018).
Despite the ever-growing literature on the values of individual elements of FEWS, no resource has yet consolidated methods for valuing multiple FEWS elements in a single tool. Adaptations to climate change can involve tradeoffs between elements of the system, so it is vital to be able to compare the social value of multiple changes in the same analysis in order to evaluate which course of action would have the most favorable net value (Goldstein et al., 2012). To meet that need, this study presents a valuation tool (Chang 2021) that adapts economic valuation methods to take user-input data and produce monetary estimates for multiple FEWS portfolio elements: food (corn and soybeans) production, power plant-level electricity generation, net carbon dioxide emissions (including carbon sequestration), and water quality (total nitrogen and total phosphorus).1
As shown in Figure 1, the tool accepts user-provided inputs for these FEWS portfolio elements and employs a variety of economic valuation techniques to produce monetary outputs, available in three different formats. The values of marketed goods like food and electricity are estimated with a social-surplus calculation approach. We use information on changes in market prices, output quantities, and features of production that help us trace out the shapes of demand and supply curves to calculate how the difference between the value to consumers and production costs to producers change in respond to a shock, like climate change. In contrast, the values of non-marketed outcomes like net carbon flux and air and water pollution are found by drawing on previous research that estimates social WTP for environmental quality, and applying those numbers to the changes in environmental quality reflected in the scenarios being analyzed.
Complete technical documentation of the functions of FEWSVT is readily available online in Chang. Below we explain the approach used in this specific paper to value changes in elements of a FEWS portfolio with the FEWSVT tool.
2.1.1 Food crop production
The food production component of the FEWSVT tool estimates changes in social surplus due to changes in crop yield for a particular commodity. These values are derived using economic surplus methods that, given initial demand and supply, quantify the magnitude of the vertical shift in a commodity’s supply curve due to a change in yield (Alston et al., 1998). This shift in supply, along with the observed change in yield, dictates the magnitude of the change in total surplus for the commodity. In Figure 2, area a-b-c-d represents this monetary measure, due to a vertical supply curve shift (b-a).
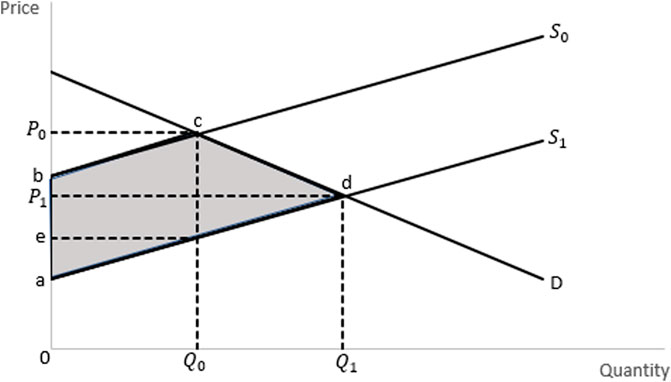
FIGURE 2. Basic economic surplus change model Note: P0 and Q0 are initial price and quantity, P1 and Q1 are final price and quantity, S0 and S1 are initial and final supply, D is demand (unchanged), and the change in total surplus is represented by shaded region abcd.
This vertical shift in a commodity’s supply curve, herein designated as parameter “K”, can be derived in one of two manners. If there is available price and quantity data for both a baseline scenario and alternative scenario, one can use Eq. 1 from Manning and Ando (2022), where P0 and P1 are baseline and alternative scenario prices, Q0 and Q1 are baseline and alternative scenario quantity, and
However, there may be cases in which one does not have a reasonable expectation as to how prices and quantities will change from its baseline level. Letourneau et al. (2015) developed an alternative calculation for K which is driven by changes in crop yield instead of prices and quantities. Eq. 2 displays this analytically, where Y0 and Y1 are baseline and alternative scenario yields. Based on the inputs provided, FEWSVT can use either method to calculate K.
Once the shift in the supply curve is quantified, changes in value can be determined. The impacts of this shift depend on change in price and quantity, as well as supply and demand elasticities. In addition, the size of the market in question determines if changes to commodity yields within the region are large enough to influence international market price. If this is the case, trade effects with any region which the study area exports to, herein designated as the “Rest-of-World” (ROW), must be considered to accurately reflect the underlying effects of the changes in yield for the studied commodity. ROW price-elasticities for the commodity must also be considered to capture all market behavior.
Therefore, FEWSVT can model two kinds of cases dependent on spatial resolution. The first case assumes the study area in question does not have a large enough market share of the commodity in which changes to its crop yields would influence international market price for that crop. If yields within the study area changed over time, international market price would remain unchanged, as producers in the study area act as price takers. The underlying equations adapted from Alston et al. (1998) are as follows:
Here, Z is the price change relative to its initial value and
If the specified study area does have a large enough market share of the commodity to influence market price, trade effects with the ROW must be considered. One example of a study areas that fulfills this case is the Midwest corn market, which accounts for an approximately 50% share of U.S. corn production, with the United States. being the world’s largest corn exporter (Roberts and Schlenker 2013). There are no numerical thresholds within the tool that determine whether inputs fall under this case; rather, spatial location is the sole determinant used by the tool. In these instances, the underlying equations (adapted from Alston et al., 1998) are as follows, for both the study area (subscript “DOM”) and areas who receive exports from it (subscript “ROW”):
Here, CA,0 is initial domestic (study area) consumption, QA,0 is initial domestic production, CB,0 is initial ROW consumption, QB,0 is initial ROW production, and μ/ε are the price-elasticities of demand (absolute value) and supply for the study area (subscript D) and ROW (subscript B). For domestic (study area) producers, we assume price elasticities account for demand and supply for both domestic and ROW consumption. For ROW areas, we assume price elasticities only account for exports received from the study area. The Z parameter is calculated in the same manner as the first case.
Trade effects are only captured by incorporating ROW supply and demand price elasticities for the commodity, and we assume that there are no trade-distorting policies that may otherwise influence market price. There are several reasons why we argue that this assumption will not lead to false estimates of surplus. Policies such as ethanol subsidies mainly impact demand for the commodity (in this case, corn), and since the shock that is fed into the tool is to supply, the effects of these policies are largely not felt for the desired parameters of interest (Babcock and Fabiosa 2011). Furthermore, many of the largest export destinations for United States. corn maintain free trade agreements under which exports enter at low or zero tariffs, which mitigates concerns over the impacts of trade distortions on model estimates (USTR 2021). Thus, given baseline values of commodity price and quantity and alternative-scenario quantities, the valuation tool uses Eq. 8 to calculate changes in total surplus.
In this paper, we input crop yield and harvested area data as described in Section 3.2 into FEWSVT Version 1.0, which assigns elasticity values as specified in Table 1. While the tool contains multiple measures of domestic and ROW elasticities, this study applies domestic elasticities from Roberts & Schlenker (2013) and ROW elasticities from Reimer et al., 2012.2
2.1.2 Electricity generation
The electricity generation component of FEWSVT estimates changes in value of power plant level production due to changes in electricity generation and marginal cost for a given technology. Understanding how measures of value change for certain electricity sectors are useful in examining the impact of various scenarios, such as future climate shock events. Utilizing methods from Logan et al. (2021), the valuation tool takes in spatially explicit user inputs of electricity generation (MWh) and marginal cost ($/MWh), and uses built-in electricity price data to output measures of present value of generation for any given electricity sector at an annual timescale. Given data inputs from both a baseline and alternative scenario, the tool can also calculate the change in value between scenarios.
For any given electrical sector, the valuation tool uses the following underlying equation, adapted from Logan et al. (2021):
Here, PVj is the present monetary value of electricity generation under conditions j, Gj is electricity generation (MWh) under conditions “j,” W is the wholesale electricity price ($/MWh) received for the given sector, and M is the marginal cost of electricity (MWh) for the given sector. The marginal cost term considers the capital (e.g., fuel) and variable operation and maintenance (O&M) costs of producing one additional unit of generation for a given electricity-generating technology.
Conditions j refers to the specific spatial and temporal input received by the tool for the electrical sector of interest. For example, the National Renewable Energy Laboratory’s (NREL) Regional Energy Deployment System (ReEDS) Model produces generation and cost projections by “balancing area” for the contiguous United States, and typically breaks down these parameters into 17 time slices that represent specific seasons and time-of-day throughout the calendar year (Brown et al., 2020).
Besides electricity generation and marginal cost, the user can also choose to specify their own electricity prices to produce monetary estimates per their own preferences. However, the tool does contain default historical wholesale electricity prices obtained from online independent system operator (ISO) databases for the Northeast and Midwest regions of the United States. We input electricity generation and price data as described in Section 3.2 and apply baseline marginal cost values as specified in Chang (2021).
2.1.3 Net CO2 flux
Greenhouse gas emissions are a uniformly mixed pollutant, such that the damages of such pollution do not depend on spatial location. Rather, damages are uniformly distributed across space regardless of the source of pollution and are quantified solely as a simple function of the total net quantity emitted. Examples of such pollutants include carbon dioxide (CO2) and chlorofluorocarbons (CFCs).
We use this component of the valuation tool to estimate changes in social wellbeing due to changes in net CO2 flux, where CO2 is emitted from power plants and absorbed through terrestrial sequestration. The valuation tool uses the following underlying equation:
Here, TD is the total net damages from carbon emissions, SCC is the social cost of carbon ($/ton), CO2PP (tons) is the quantity of carbon dioxide emissions from power plants, and CO2SQ (tons) is carbon sequestration levels. It is important to note that carbon sequestration data is typically measured in mass units of carbon. Thus, the valuation tool first converts mass carbon units into its carbon dioxide equivalent by multiplying by the ratio of the molecular weight of carbon dioxide to that of carbon. If the user does not have carbon sequestration data, the CO2SQ term is simply dropped from the equation and the resulting monetary estimate is changed to the total gross damages from just carbon dioxide emissions.
The valuation tool contains several default values of the SCC taken from literature that allows a user the flexibility to produce either a low, middle, or high monetary estimate depending on their preferences Hänsel et al. (2020) (Interagency Working Group on Social Cost of Greenhouse Gases, 2016; Nordhaus 2017). As shown in Table 2, all SCC values used by the tool assume a 3% discount rate and are updated to have uniform units of 2021 United States. dollars (USD) per ton of carbon dioxide emitted. The valuation tool also allows a user to input their own estimate which takes precedent over the default values within the tool.3 This paper uses estimates of net carbon flux from both carbon dioxide emissions from power plants and net carbon sequestration from multiple land cover types for the Midwest and Northeast regions in the United States and applies a SCC value of $51/ton CO2 as estimated by Interagency Working Group on Social Cost of Greenhouse Gases, 2016.
2.1.4 Water quality
The water quality component of the valuation tool estimates changes in consumer WTP to use a water body due to changes to its water quality. The valuation tool first takes in water pollutant concentration inputs to produce a “Water Quality Index” (WQI) estimate, and then employs a benefit transfer methodology to calculate monetary values associated with people’s WTP for a water body’s WQI (Johnston et al., 2005; Alvarez et al., 2016; Johnston et al., 2017; Johnston and Bauer 2020).
The WQI is a standardized 100-point index that relates water pollutant concentrations to water body suitability for wildlife and human usage (Johnston and Bauer 2020). Higher WQI values indicate a water body of higher quality, with water pollutant concentrations at a level low enough to support aquatic life, recreational use, or even drinking water consumption. The WQI is calculated using pollutant concentration data inputs, which are translated into subindex values that are combined with an assigned index weight to produce an aggregate WQI estimate. The resulting WQI equation is as follows (adapted from Walsh and Wheeler 2013):
where Qi is the water quality subindex for water pollutant i, and Wi is the assigned index weight of water pollutant i. Table 3 shows all assigned subindex weights for water pollutants in freshwater water bodies. For each water pollutant, shown in Table 4, subindex values are first calculated on a 10–100 scale dependent on pollutant concentration. Once these subindex values are derived, Eq. can be used to generate a WQI estimate for any water body.
A benefit transfer methodology is then applied to estimate the monetary value of changes to the initial WQI estimate. The tool uses a meta-regression model developed by Johnston et al., 2017, using 140 unique observations from 51 stated preference studies published between 1985 and 2013 that estimated WTP for changes to WQI as a function of independent variables (Berrgstrom and Taylor 2006) that characterize features of the study site, water bodies, and nearby affected population from studies in the analysis. The variables used in this model are listed in Table B1 of Chang (2021) and the coefficients on those variables resulting from a random effects regression are summarized in Table B2 of Chang (2021). These coefficients are matched with any geographic features of the water body, as well as the socioeconomic characteristics of those who reguarly utilize it. For example, if the water body in question was located in the United States. Department of Agriculture Northeast region, the user would assign a binary value of “1” to the variable “Northeast_United States.” Once all variable coefficients have been matched with its selected value, household WTP is calculated using standard Consumer Price Index (CPI) adjustment for a specified increase in WQI for the desired water body. Household data is then used to calculate region-wide WTP.
This paper inputs nitrogen concentrations (mg/L) for water bodies located in the Midwest and Northeast United States. For the other five water pollutants that comprise the WQI, the tool will assign a default subindex value of 50 for those pollutants. Thus, the resulting changes in WQI across the climate scenario described in Section 3.2 only reflects changes in nitrogen concentrations.
2.2 Climate scenario data
This study is within the structure of the C-FEWS framework (Vörösmarty et al., 2023 this issue), where all climate scenarios are based on the North American Land Data Assimilation Phase 2 (NLDAS-2) and its intensified modification. The input datasets are mostly from the model simulations within the C-FEWS framework that follow the same climate scenarios of historical and intensified extremes to produce the food production, carbon sequestration, electricity generation, etc.
2.2.1 Data sources
The NLDAS-2 data was obtained from NASA Goddard Earth Sciences Data and Information Services Center (DES DISC, https://disc.gsfc.nasa.gov/), that covers the CONUS from 1980 to 2019. The historical NLDAS-2 data and intensified climate data were fed into a) the Terrestrial Ecosystem Model (TEM) to produce the carbon sequestration data (Kicklighter et al., 2023 this issue), b) the Integrated Science Assessment Model (ISAM) to produce the food production data (Lin et al., this issue), c) the Water Balance Model (WBM) coupled with Thermoelectric Power & Thermal Pollution Model (TP2M) to produce electricity production data as well as CO2 emission data (Zhang et al., this issue), and d) the SPAtially Referenced Regression On Watershed attributes (SPARROW) model to produce the water pollution data (Maxfield et al., 2023 this issue). All model simulations were aggregated into each state within the C-FEWS framework defined NE and MW regions (Figure 3, Vorosmarty et al., 2023 this issue). The brief description of the model simulation data that was used in this study are listed in Table 5.
For the historical baseline and heat wave scenarios, we received data from several sources to apply as inputs to the FEWSVT tool. Food production data was obtained from Lin et al. (2023 this issue) for corn and soybeans via the Integrated Science Assessment Model (ISAM), a process-based dynamic crop and vegetation model that simulates the productivity of food and bioenergy crops (Niyogi et al., 2015).
Annual electricity generation (MWh), electricity price ($/MWh) and carbon dioxide emissions (metric tons) data were obtained from the Thermoelectric Power and Thermal Pollution (TP2M) model, which is a simulation model that simultaneously quantifies thermal pollution from rivers and estimates efficiency losses in electricity generation (Zhang et al., 2023 this issue) were obtained from Zhang et al., 2023. Marginal cost of electricity production ($/MWh) data was taken from Chang (2021) and were matched by both time and space to the TP2M generation data.
Annual carbon sequestration (Tg C) data was obtained from Kicklighter et al. (2023 this issue) via the Terrestrial Ecosystem Model (TEM), which is a process-based biogeochemistry model that uses spatially referenced information to estimate fluxes of carbon in multiple land cover types (Felzer et al., 2004).4
Annual water quality data for the baseline scenario are obtained from Maxfield et al. (2023 this issue) via the United States. Geological Survey’s Spatially Referenced Regression on Watershed Attributes (SPARROW) model, which estimates long-term average values of water characteristics by synthesizing monitoring and geographic data (Bergamaschi et al., 2014). Water quality data for the heat wave scenario are calculated based on the following equation, in which TNriver is total nitrogen concentration at a given reach, TNland is overland nitrogen concentration moving to a river, and T is water temperature in degrees Celsius.
For the baseline and heat wave scenarios, we only input annual nitrogen concentrations (mg/L) by county. The other four water pollutants that comprise the WQI estimate (TP, DO, BOD, TSS, FC) are assumed to hold a subindex value of 50 and are unchanged from one scenario to the next. Thus, as detailed in Section 3.1.3, the resulting monetary estimates reflect the change in WTP due to a change in WQI resulting only from a change in nitrogen concentrations. We also received additional information regarding the water body type(s) and geographic location of each region, which dictates the usage of benefit transfer coefficients that are used to calculate monetary values.
All annual inputs for food production, electricity generation, and net CO2 flux are fed into the FEWSVT tool at the state level for the years 2010–2019. Water quality inputs are provided at the county-level for the aggregated period from 2010–2019. In other words, water pollutant concentrations were provided as the average concentration across 2010 to 2019, as opposed to individual annual values from 2010 to 2019.
2.2.2 Scenarios
The C-FEWS framework contains multiple non-climate and climate scenarios to investigate and quantify the sensitivity of a FEW system (Vorosmarty et al., 2023 this issue). This paper focuses only on the difference between the baseline scenarios and one out of 4 extreme climate scenarios to demonstrate the characteristics of the valuation tool. The baseline scenario is the 40 years historical climate recorded in NLDAS-2 dataset that drove all the C-FEWS models to produce the datasets needed for this study.
The intensified extreme climate scenarios (IECS) that were described in Approach B in Vorosmarty et al. (2023 this issue), where the most climate extreme year in each of four types (heat wave, cold wave, heavy precipitation, and drought) within the last decade (2010–2019) and its 2 sub-sequential years were selected to repeat three times to replace 9 years of climate data from 2010 to 2019. In this study, the focus is the heat wave. The extreme heat wave years 2012–2014 for MW and 2016–2018 for NE were selected to repeat three times and replace the 2010–2018 climate data from the baseline. The intensified extreme climate datasets were then used to drive all the C-FEWS models to generate the data for this study. Figure 4 shows the air temperatures of the baseline scenario and the heat wave IECS from 1980–2019 to show how the latter is constructed. As heat wave has been predicted to be more frequent in the future (Raghavendra et al., 2019; Lorenzo et al., 2021), the scenario chosen in this study is relevant to likely future climate outcomes.
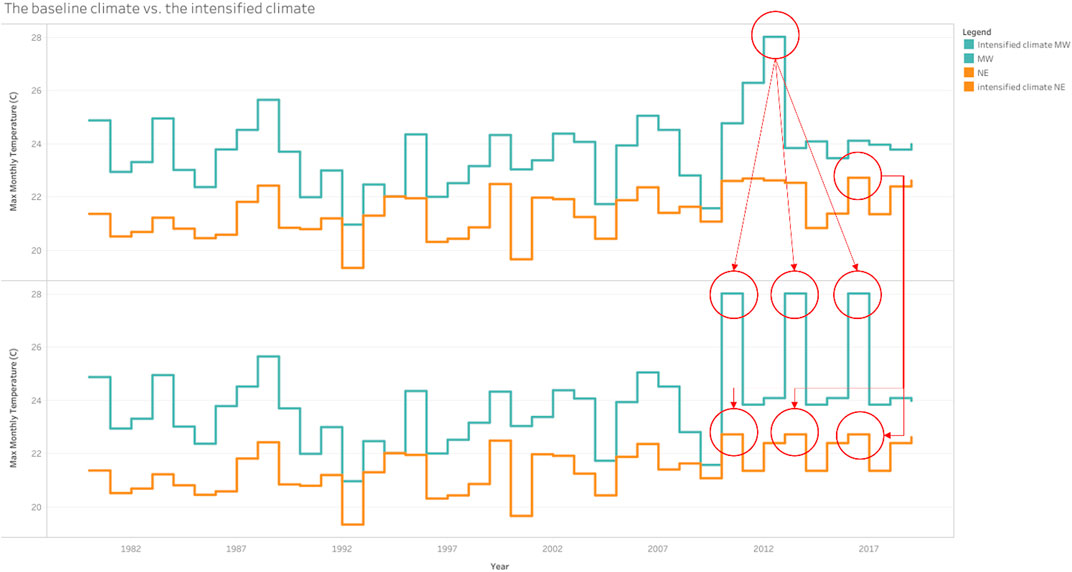
FIGURE 4. Demonstration of creation of extreme climate scenarios. Note: The extreme heat wave event from 2010–2019 was identified and replicated three times across the last decade of C-FEWS framework of studies.
This paper examines the monetary implications of heat wave conditions as specified in Vörösmarty et al., 2023 compared to historically typical (baseline) climate conditions for two defined geographic regions in the United States (Midwest (MW) and Northeast (NE))5. The heat wave data received from sources described in Section 3.2.1 reflect one of several climate extreme scenarios described in Vörösmarty et al., 2023. Results from FEWSVT indicate the monetary implications of changing meteorological conditions related to a modeled heat wave scenario from historical conditions as a result of changes in physical flows in food production, electricity generation, carbon dioxide emissions, and water quality (total nitrogen concentrations).
2.3 Analyses
We apply the FEWSVT tool to outcome simulations for the heat wave climate extreme scenario described in Section 3.2.2. We analyse the results to answer policy-relevant questions related to the impact of climate extremes on ecosystem service flows. When climate extremes hit, which changes in food-energy-water physical outcomes are likely to have the largest monetized effects on society? How important is it to use theoretically sound economics models for valuation?
2.3.1 Ranking monetized impacts of a climate-extreme event
We use valuations of the heat wave scenario results to show which changes in climate change-attributable physical flows are likely to have the largest monetized value. We first use FEWSVT to value the monetary impacts due to changes in physical flows for each FEWS parameter. Then we rank all FEWS parameters by magnitude to examine which elements contain the highest monetary impacts directly attributed to the modelled changes in physical flows.
2.3.2 Importance of using theoretically sound economic models for valuation
Researchers outside economics often use simple approaches to estimate the value of changes in sectors of the economy that yield marketable goods. If a scenario change yields a change in the quantity of a good like electricity or crops produced, it is tempting to represent the value of that change as the change in the market revenue from selling that output assuming a fixed price. However, as pointed out by Letourneau et al. (2015), that kind of simple revenue-change calculation can produce highly inaccurate measures of the actual changes in social wellbeing. If output falls because of an increase in production costs, the revenue-change calculation will understate the loss to society because it doesn’t account for increased costs of producing the quantity still produced. On the other hand, the revenue-change calculation can overstate the loss to society because it does not subtract production costs from the social value of the quantity of good no longer produced.
The FEWSVT tool estimates the value to society of changes in production costs and market outcomes for two kinds of goods sold in marketplaces: electricity and crops. In this part of the analysis, we conduct simple revenue-change calculations for each of the changes in outputs associated with the change to the extreme scenario by multiplying the original market price by the change in quantity. We then compare those values to the theoretically sound values produced by the models in the FEWSVT tool.
2.3.3 Utility of monetization for decision making
Managers and policy makers may need to make choices between policies and investments that have trade-offs between physical outcomes. For example, investing further in tile drainage in the Midwest could improve crop production in the face of increasing wet springs, but worsen water pollution because of increased nitrogen runoff. Policy makers could create an index of normalized physical changes (like percentage changes) in qualitatively different physical flows and pick the investments that do best in terms of that index. Alternatively, they could monetize the changes and pick the investment that does best in terms of total monetized value. This section uses data from the baseline and the heat wave scenario in a simple demonstration of how monetization can affect one’s judgment regarding the relative merits of investments that lead to one set of outcomes versus another.
3 Results
3.1 Ranking monetized impacts of a climate-extreme event–results
Table 6 displays all summary results by study region representing the change in value due to FEWS portfolio element inputs changing from conditions set forth in the baseline scenario to those in the heat wave scenario. The reported values indicate the monetary impacts due to changes set forth under a heat wave climate event relative to historic conditions. For all FEWS portfolio elements except water quality, values in Table 6 represent the yearly average (2010–2019) of all annual results. For water quality, since data inputs reflect county-level water pollutant concentrations across the 2010–2019 decade, results simply reflect outputs for that aggregate time period.
These FEWSVT outputs offer an illustrative example of the potential monetary implications of a modeled heat wave scenario on changes in food production, electricity generation, air pollutant emissions, and water quality. From the summary results posted in Table 6, food (corn and soybeans) production accounts for the largest share of the resulting changes, with a change in value of approximately $291 billion (89% of the entire changes in value for both regions). Changes in values in the MW region dwarf NE region estimates, with the NE region accounting for less than one percent of the total changes in values for corn and soybeans production. This observation is unsurprising, as the MW region of the United States is a significant producer of both corn and soybeans and accounts for a large share of total exports for both crops. This is also substantiated by the raw data inputs; for instance, total harvested acres for corn production in the MW region was approximately twenty times that of the NE region.
Changes in value for the remaining three FEWS portfolio elements are smaller in magnitude across both regions, ranging from $206 million (water quality) to $28.5 billion (net CO2 flux). These results indicate that under the modelled heat wave conditions, the food production sector would potentially be most affected relative to other elements considered within the food-energy-water nexus.
3.2 Importance of using theoretically sound economic models for valuation–results
As stated in Section 3.3.2, it is vital to use theoretically sound methods when valuing changes in economic value. To demonstrate the level of magnitude of differences in changes in value, we compare the food and electricity results from Section 4.1 with an alternative simplified methodology that simply calculates economic value by multiplying price and quantity under the baseline and heat wave scenarios. For food, we multiply corn and soybeans production levels by their respective prices (omitting crop-specific market relationships at the state level), while for food we multiply electricity generation by its received price (omitting application of the marginal cost of electricity production).
Table 7 displays these values, the magnitude of differences in changes in value, and the percent difference between applying theoretically sound methods versus overly simplified methods. We observe that the simplified methodology underestimates the true change in value for food production and overestimates the true change in value for electricity generation. The magnitude of changes in value between both metrics using the simplified method are quite similar, but when using theoretically sound economic valuation techniques, we find food production to have much higher potential economic implications under the modelled heat wave scenario.
3.3 Utility of monetization for decision making—results
As stated in Section 3.3.3, it is useful to compare how changes in physical flows of food-energy-water metrics relate to corresponding monetary changes in value. It is often not appropriate to assume that observed changes in physical flows will linearly scale with change in monetary value. The economic valuation techniques specified throughout Section 3 often compute changes in value that relate changes in physical flows in a non-linear manner.
Using the results presented in Table 6 as reference, while elements like net carbon dioxide flux show a proportional relationship between change in physical flows and change in monetary flows, other elements such as water quality do not. For example, a 5% change in physical flows (nitrogen concentrations) results in a 1.5% change in monetary flows, and a 10% change results in a 3.8% change in monetary flows. This is expected, as the valuation calculation for water quality is complex and non-linear, while other elements like net carbon dioxide emissions values are calculated linearly.
For these metrics, it is apparent that one cannot assume without calculation that a change in physical flows by some fraction will correspond to the same change in monetary value. Thus, it is useful to understand the value in employing these theoretically sound valuation techniques, as it adds additional context for decision makers when comparing how observed changes in physical flows compare to corresponding monetary impacts.
4 Discussion
There are several key takeaways from this analysis that all speak to the potential monetary implications of a modelled climate change extreme scenario. We find that the vast majority (89%) of total changes in value across the Midwest and Northeast United States attributed to changes in physical flows for various food-energy-water parameters come from changes in corn and soybean yields. We find that for the two study regions, relative to changes in value stemming from changes in physical flows related to electricity generation, net carbon dioxide flux, and water quality, changes to food and soybean yields have the most implications on potential monetary impacts under the modelled heat wave scenario. Observations like this can help decision makers understand what food-energy-water metrics have significant monetary impacts to society, and provides additional context to the observed measured changes in physical flows. For this analysis, it is clear that stakeholders should focus on the food sector when deciding on how to distribute investments to combat the effects of heat wave-related climate extreme events.
This analysis also emphasizes the importance of utilizing theoretically sound economic valuation techniques as opposed to back of the envelope linear calculations. We find that when changes in physical flows for food production and electricity generation are simply multiplied by its market price, the resulting monetary impacts for both metrics are quite close in magnitude. This observation may lead decision makers to incorrectly determine that investments to curb detrimental effects of heat wave-related climate extreme events should be equally distributed across these two sectors. As shown in our application of theoretically sound economic valuation techniques applied through the FEWSVT tool, investments should be primarily devoted to food production. We also find the magnitude of changes in value to be quite different when we apply the simplistic methodology, further emphasizing the importance of utilizing accepted valuation methods to appropriately characterize the impacts of heat waves on these sectors.
These findings demonstrate the value in considering the monetary impacts of climate change-related events in addition to impacts on physical flows within elements of the food-energy-water nexus. However, when considering the implications of these results, it is important to note the limitations and uncertainties of each valuation technique utilized in the CFEWSVT tool. For instance, there may be uncertainties with the point estimate inputs provided to the CFEWSVT tool, which could potentially overstate or understate the associated monetary outputs. Users can address this by conducting additional runs to produce a range of possible monetary impacts instead of one point estimate.
Each valuation methodology executed in the CFEWSVT tool have their own limitations as well. For instance, the economic surplus methods utilized in the food production component of the tool currently do not consider benefits due to technological improvements or mitigation strategies (such as changing input use or crop choice), which may overstate the net costs of a heat wave scenario. The tool also does not consider general equilibrium effects nor impacts on downstream markets beyond the study area(s) of interest. Finally, the tool does not report results with statistical error bounds, as there are currently no statistical methods to assign pertaining error bounds around the module estimates. With these limitations in mind, while the CFEWSVT tool provides a convenient outlet for users to produce preliminary results without having to execute highly technical analysis, the users should not consider the monetary outputs from the tool as more sophisticated than the results of more complex models more tailored to site-specific characteristics.
The FEWSVT tool offers a convenient way for decision makers to apply observed changes in food-energy-water metrics to estimate corresponding changes in monetary value, whilst also allowing them to compare how these values correlate with changes in physical flows. Considering both changes to physical and monetary flows calculated with sound economic valuation techniques allows for well-informed decisions on how to best distribute investments in response to anticipated climate change-related events.
Data availability statement
Publicly available datasets were analyzed in this study. This data can be found here: The datasets analyzed for this study can be found in the CFEWS data repository: https://cloud.environmentalcrossroads.net/s/sxNPYebRyJrDDDz/download.
Author contributions
JC and AA contributed to conception and design of the study. JC created the valuation tool. MC organized the data used in the analyses. JC performed the valuation analyses. All authors wrote sections of the manuscript, contributed to manuscript revision, read, and approved the submitted version.
Funding
Major funding for this paper came from the U.S. National Science Foundation’s Innovations at the Nexus of Food, Energy and Water Systems (INFEWS/T1 Grant #1856012). This paper is also based in part on work funded by the USDA-NIFA W4133 Multistate Research Grant #1008843.
Acknowledgments
This work benefitted particularly from discussion with members of the CFEWS team involved in running domain models (Atul Jain, David Kicklighter, Tzu-Shun Lin, Nicolas Maxfield, Richard Smith, Jerry Melillo, and Jiaqi Zhang) and from discussions with Ben Gramig, Ehsan Najafi, William Ridley, and Charles Vörösmarty.
Conflict of interest
The authors declare that the research was conducted in the absence of any commercial or financial relationships that could be construed as a potential conflict of interest.
Publisher’s note
All claims expressed in this article are solely those of the authors and do not necessarily represent those of their affiliated organizations, or those of the publisher, the editors and the reviewers. Any product that may be evaluated in this article, or claim that may be made by its manufacturer, is not guaranteed or endorsed by the publisher.
Footnotes
1The tool presented in Chang (2021) also quantifies damages from local air pollution released by electricity-generating power plants (nitrous oxide and sulfur dioxide).
2While FEWSVT only contains elasticity values for corn and soybeans, the user can input their own estimates of crop price and elasticity for other commodities of interest.
3FEWSVT only contains SCC values for one uniformly mixed air pollutant (carbon dioxide). However, FEWSVT can still operate for other uniformly mixed pollutants given the desired air pollutant’s emissions and social cost estimate.
4Before subtracting these sequestration estimates from the emissions level, the FEWSVT tool uses molecular weights of carbon and carbon dioxide to first convert mass units’ carbon into its carbon dioxide equivalent.
5The MW and NE study regions are comprised of nine and thirteen states, respectively within the contiguous United States.
References
Adhikari, U., Nejadhashemi, A., and Woznicki, S. (2015). Climate change and eastern africa: A review of impact on major crops. Food Energy Securaa 4 (2), 110–132. doi:10.1002/fes3.61
Alston, J. M., Norton, G. W., and Pardey, P. G. (1998). Science under scarcity. Washington, DC, USA: CAB International, 207–299.
Alvarez, S., Aşçi, S., and Vorotnikova, E. (2016). Valuing the potential benefits of water quality improvements in watersheds affected by non-point source pollution. Water 8, 112. doi:10.3390/w8040112
Antle, J. M., and Capalbo, S. M. (2010). Adaptation of agricultural and food systems to climate change: An economic and policy perspective. Appl. Econ. Perspect. Policy 32 (3), 386–416. doi:10.1093/aepp/ppq015
Babcock, B. A., and Fabiosa, J. F. (2011). The impact of ethanol and ethanol subsidies on corn prices: Revisiting history. CARD Policy Brief 11-PB 5. Ames, IA: CARD. Retrieved from https://www.card.iastate.edu/.
Bateman, I. J., and Kling, C. L. (2020). Revealed preference methods for nonmarket valuation: An introduction to best practices. Rev. Environ. Econ. Policy 14 (2), 240–259. doi:10.1093/reep/reaa009
Bergamaschi, B. A., Smith, R. A., Sauer, M. J., Shih, J.-S., and Ji, L. (2014). “Terrestrial fluxes of nutrients and sediment to coastal waters and their effects on coastal carbon storage in the Eastern United States,” in Baseline and projected future carbon storage and greenhouse-gas fluxes in ecosystems of theEeastern United States (Reston, VA, USA: U.S. Department of the Interior), 85–114.
Brown, M., Wesley, C., Kelly, E., Becker, J., Bielen, D., Chernyakhovskiy, I., et al. (2020). “Regional energy deployment system (ReEDS) model documentation: Version 2019,”. NREL/TP-6A20-74111 (Golden, CO, USA: National Renewable Energy Laboratory). Retrieved from https://www.nrel.gov/docs/fy20osti/74111.pdf.
Chang, J. W. (2022). Fewsvt. Available at: https://zenodo.org/badge/latestdoi/540868267.
Chang, J. W. (2021). Valuation tool for food-energy-water portfolio outcomes. M.Sc. Thesis (Gramig, Benjamin: University of Illinois Urbana-Champaign). https://www.ideals.illinois.edu/items/120926.
Felzer, B., Kicklighter, D. W., Melillo, J., Wang, C., Zhuang, Q., and Prinn, R. (2004). Effects of ozone on net primary production and carbon sequestration in the conterminous United States using a biogeochemistry model. Tellus B 56 (3), 230–248. doi:10.1111/j.1600-0889.2004.00097.x
Fonseca, F., Craig, M., Jaramillo, P., Berges, M., Severnini, E., Loew, A., et al. (2021). Effects of climate change on capacity expansion decisions of an electricity generation fleet in the Southeast U.S. Environ. Sci. Technol. 55 (4), 2522–2531. doi:10.1021/acs.est.0c06547
Goldstein, J. H., Caldarone, G., Duarte, T. K., Ennaanay, D., Hannahs, N., Mendoza, G., et al. (2012). Integrating ecosystem-service tradeoffs into land-use decisions. Proc. Natl. Acad. Sci. 109 (19), 7565–7570. doi:10.1073/pnas.1201040109
Gourevitch, J., Keeler, B., and Ricketts, T. (2018). Determining socially optimal rates of nitrogen fertilizer application. Agric. Ecosyst. Environ. 254, 292–299. doi:10.1016/j.agee.2017.12.002
Hanley, N., and Czajkowski, M. (2019). The role of stated preference valuation methods in understanding choices and informing policy. Rev. Environ. Econ. Policy 13 (2), 248–266. doi:10.1093/reep/rez005
Hänsel, M. C., Drupp, M. A., Johansson, D. J. A., Nesje, F., Azar, C., Freeman, M. C., et al. (2020). Climate economics support for the UN climate targets. Nature Climate Change 10, 781–789. doi:10.1038/s41558-020-0833-x
Heo, J., Adams, P., and Gao, H. (2016a). Public health costs of primary PM2.5 and inorganic PM2.5 precursor emissions in the United States. Environ. Sci. Technol. 50 (11), 6061–6070. doi:10.1021/acs.est.5b06125
Holland, S., Mansur, E. T., Muller, N., and Yates, A. (2020). Decompositions and policy consequences of an extraordinary decline in air pollution from electricity generation. Am. Econ. J. Econ. Policy 12, 244–274. doi:10.1257/pol.20190390
Houtven, G., Powers, J., and Pattanayak, S. (2007). Valuing water quality improvements in the United States using meta-analysis: Is the glass half-full or half-empty for national policy analysis? Resour. Energy Econ. 29 (3), 206–228. doi:10.1016/j.reseneeco.2007.01.002
Interagency Working Group on Social Cost of Greenhouse Gases (2016). Technical support document: Technical update of the social cost of carbon for regulatory impact analysis under executive order 12. Retrieved from https://www.epa.gov/sites/default/files/2016-12/documents/sc_co2_tsd_august_2016.pdf.
Islam, A., Ahuja, L., Garcia, L., Ma, L., Saseendran, A., and Trout, T. (2012). Modeling the impacts of climate change on irrigated corn production in the Central Great Plains. Agric. Water Manag. 110, 94–108. doi:10.1016/j.agwat.2012.04.004
Jin, S., Yu, W., Jansen, H., and Muraoka, R. (2012). The impact of irrigation on agricultural productivity: Evidence from India. Trienn. IAAE Conf. 8 (3). doi:10.14445/23942568/IJAES-V8I3P102
Johnston, R. J., and Bauer, D. (2020). Using meta-analysis for large-scale ecosystem service valuation: Progress, prospects, and challenges. Agric. Resour. Econ. Rev. 49, 23–63. doi:10.1017/age.2019.22
Johnston, R. J., Besedin, E. Y., Iovanna, R., Miller, C., Wardwell, R. F., and Ranson, M. (2005). Systematic variation in willingness to pay for aquatic resource improvements and implications for benefit transfer: A meta-analysis. Can. J. Agric. Econ. 53, 221–248. doi:10.1111/j.1744-7976.2005.04018.x
Johnston, R. J., Besedin, E. Y., and Stapler, R. (2017). Enhanced geospatial validity for meta-analysis and environmental benefit transfer: An application to water quality improvements. Environ. Resour. Econ. 68, 343–375. doi:10.1007/s10640-016-0021-7
Kauffman, G. (2019). Economic benefits of improved water quality in the Delaware River (USA). River Res. Appl. 35, 1652–1665. doi:10.1002/rra.3484
Keeler, B., Gourevitch, J. D., Polasky, S., Isbell, F., Tessum, C., Hill, J. D., et al. (2016). The social costs of nitrogen. Sci. Adv. 2, e1600219. doi:10.1126/sciadv.1600219
Kicklighter, D. W., Lin, T.-S., Zhang, J., Chen, M., Vörösmarty, C. J., Jain, A., et al. (2023). Influence of forest infrastructure on the responses of ecosystem services to climate extremes in the Midwest and Northeast United States from 1980 to 2019. Front. Environ. Sci.
Lencucha, R., Pal, N., Appau, A., Thow, A., and Drope, J. (2020). Government policy and agricultural production: A scoping review to inform research and policy on healthy agricultural commodities. Glob. Health 16 (11). doi:10.1186/s12992-020-0542-2
Letourneau, Deborah K., Ando, Amy W., Jedlicka, Julie A., Narwani, Anita, and Barbier, Edward (2015). Simple-but-sound methods for estimating the value of changes in biodiversity for biological pest control in agriculture. Ecol. Econ. 120, 215–225. doi:10.1016/j.ecolecon.2015.10.015
Lin, T.-S., Jain, A., Kheshgi, H. S., Song, Y., and Vörösmarty, C. J. (2023). Which crop has the highest bioethanol yield and water use efficiency in the United States of America? Front. Environ. Sci.
Lobell, D. B., Hammer, G. L., McLean, G., Messina, C., Roberts, M. J., and Schlenker, W. (2013). The critical role of extreme heat for maize production in the United States. Nat. Clim. Change 3, 497–501. doi:10.1038/nclimate1832
Logan, L., Gupta, R., Ando, A., Suski, C., and Stillwell, A. (2021). Quantifying tradeoffs between electricity generation and fish populations via population habitat duration curves. Ecol. Model. 440, 109373. doi:10.1016/j.ecolmodel.2020.109373
Lorenzo, N., Díaz-Poso, A., and Royé, D. (2021). Heatwave intensity on the iberian peninsula: Future climate projections. Atmos. Res. 258, 105655. doi:10.1016/j.atmosres.2021.105655
Manning, D., and Ando, A. (2022). Ecosystem services and land rental markets: Producer costs of bat population crashes. J. Assoc. Environ. Resour. Econ. 9 (6), 1235–1277. doi:10.1086/720303
Maxfield, N. E., Smith, R. A., Chang, J., Ando, A. W., Lin, T.-S., and Vörösmarty, C. J. (2023). Modeling the impact of technology, management, and policy-related strategies on CONUS water quality and ecosystem services. Front. Environ. Sci. this issue.
Mideksa, T., and Kallbekken, S. (2010). The impact of climate change on the electricity market: A review. Energy Policy 38 (7), 3579–3585. doi:10.1016/j.enpol.2010.02.035
Muller, N., and Mendelsohn, R. (2007). Measuring the damages of air pollution in the United States. J. Environ. Econ. Manag. 54, 1–14. doi:10.1016/j.jeem.2006.12.002
Naime, J., Mora, F., Sánchez-Martínez, M., Arreola, F., and Balvanera, P. (2020). Economic valuation of ecosystem services from secondary tropical forests: Trade-offs and implications for policy making. For. Ecol. Manag. 473, 118294. doi:10.1016/j.foreco.2020.118294
Nelson, G. C., Valin, H., Sands, R. D., Havlik, P., Ahammad, H., Deryng, D., et al. (2014). Climate change effects on agriculture: Economic responses to biophysical shocks. Proc. Natl. Acad. Sci. 111 (9), 3274–3279. doi:10.1073/pnas.1222465110
Niyogi, D., Liu, X., Andresen, J., Song, Y., Jain, A. K., Kellner, O., et al. (2015). Crop models capture the impacts of climate variability on corn yield. Geophys. Res. Lett. 42 (9), 3356–3363. doi:10.1002/2015gl063841
Nordhaus, W. (2017). Revisiting the social cost of carbon. Proc. Natl. Acad. Sci. 114, 1518–1523. doi:10.1073/pnas.1609244114
Parkinson, S., Krey, V., Huppmann, D., Kahil, T., McCollum, D., Fricko, O., et al. (2019). Balancing clean water-climate change mitigation trade-offs. Environ. Res. Lett. 14 (1), 014009. doi:10.1088/1748-9326/aaf2a3
Petrolia, D. R., Guignet, D., Whitehead, J., Kent, C., Caulder, C., and Amon, K. (2021). Nonmarket valuation in the Environmental Protection Agency's regulatory process. Appl. Econ. Perspect. Policy 43 (3), 952–969. doi:10.1002/aepp.13106
Raghavendra, A., Dai, A., Milrad, S. M., and Cloutier-Bisbee, S. R. (2019). Floridian heatwaves and extreme precipitation: Future climate projections. Clim. Dyn. 52, 495–508. doi:10.1007/s00382-018-4148-9
Reimer, J., Zheng, X., and Gehlhar, M. (2012). Export demand elasticity estimation for major U.S. crops. J. Agric. Appl. Econ. 44 (4), 501–515. doi:10.1017/s107407080002407x
Roberts, M. J., and Schlenker, W. (2013). Identifying supply and demand elasticities of agricultural commodities: Implications for the US ethanol mandate. Am. Econ. Rev. 103 (6), 2265–2295. doi:10.1257/aer.103.6.2265
Solaun, K., and Cerda, E. (2019). Climate change impacts on renewable energy generation. A review of quantitative projections. Renew. Sustain. Energy Rev. 116, 109415. doi:10.1016/j.rser.2019.109415
Tilman, D., Balzer, C., Hill, J., and Befort, B. (2011). Global food demand and the sustainable intensification of agriculture. Proc. Natl. Acad. Sci. 108 (50), 20260–20264. doi:10.1073/pnas.1116437108
Unisfera International Centre (2003). “The economic and environmental impacts of agricultural subsidies: An assessment of the 2002 US farm bill and doha round,” in Prepared for the second North American symposium on assessing the environmental effects of trade (Mexico, MX, USA: Commission for Environmental Cooperation).
U.S. Environmental Protection Agency (2018). Environmental benefits mapping and analysis program. Retrieved from https://www.epa.gov/benmap/benmap-ce-manual-and-appendices.Community Edition
USTR (2021). Free trade agreements. Retrieved from https://ustr.gov/issue-areas/industry-manufacturing/industrial-tariffs/free-trade-agreements.
Vörösmarty, C. J., Melillo, J., Wuebbles, D. J., Jain, A. K., Ando, A., Tuler, S., et al. (2023). A framework to study climate-induced extremes on food, energy, and water Systems (C-FEWS): The role of engineered and natural infrastructures, technology, and environmental management in the U.S. Midwest and Northeast. Front. Environ. Sci.
Walsh, J. P., and Wheeler, W. J. (2013). Water quality indices and benefit-cost analysis. J. Benefit-Cost Analysis 4 (1), 81–105. doi:10.1515/jbca-2012-0005
WWAP (United Nations World Water Assessment Program) & UN-Water (2018). The united nations world water development report 2018: Nature-based solutions for water. Paris, France: UNESCO.
Xu, Z., Chen, X., Liu, J., Zhang, Y., Chau, S., Bhattarai, N., et al. (2020). Impacts of irrigated agriculture on food–energy–water–CO2 nexus across metacoupled systems. Nat. Commun. 11, 5837. doi:10.1038/s41467-020-19520-3
Keywords: economic valuation, food-energy-water nexus, climate change, consumer surplus, food production, electricity generation, water quality
Citation: Chang JW, Ando AW and Chen M (2023) Valuing changes in the portfolio of service flows from climate-induced extremes on a linked food, energy, water system (C-FEWS). Front. Environ. Sci. 11:1069483. doi: 10.3389/fenvs.2023.1069483
Received: 13 October 2022; Accepted: 23 January 2023;
Published: 10 February 2023.
Edited by:
Richard Lawford, Morgan State University, United StatesReviewed by:
Chong Huang, Shandong University of Finance and Economics, ChinaChrysi S. Laspidou, University of Thessaly, Greece
Copyright © 2023 Chang, Ando and Chen. This is an open-access article distributed under the terms of the Creative Commons Attribution License (CC BY). The use, distribution or reproduction in other forums is permitted, provided the original author(s) and the copyright owner(s) are credited and that the original publication in this journal is cited, in accordance with accepted academic practice. No use, distribution or reproduction is permitted which does not comply with these terms.
*Correspondence: Joseph W. Chang, am9zZXBod2VpY2hhbmdAZ21haWwuY29t
†Present Address: Joseph W. Chang, Industrial Economics Inc., Cambridge, MA, United States