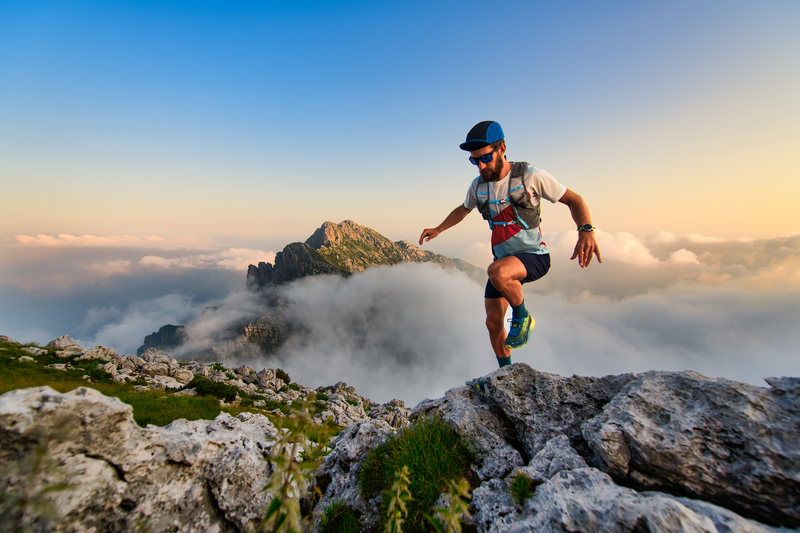
95% of researchers rate our articles as excellent or good
Learn more about the work of our research integrity team to safeguard the quality of each article we publish.
Find out more
ORIGINAL RESEARCH article
Front. Environ. Sci. , 30 January 2023
Sec. Environmental Systems Engineering
Volume 11 - 2023 | https://doi.org/10.3389/fenvs.2023.1055771
This article is part of the Research Topic Food-Energy-Water Systems: Achieving Climate Resilience and Sustainable Development in the 21st Century View all 21 articles
Integrated assessment models (IAMs) capture synergies between human development and natural ecosystems that have important implications for the food-energy-water (FEW) nexus. However, their lack of fine-scale representation of water regulatory structure and landscape heterogeneity impedes their application to FEW impact studies in water-limited basins. To address this limitation, we developed a framework for studying effects of global change on regional outcomes for food crops, bioenergy, hydropower, and instream flows. We applied the new methodology to the Columbia River Basin (CRB) as a case study. The framework uses the Demeter land-use and land-cover change (LULCC) downscaling tool, which we updated so that water rights are spatially integrated in the land allocation process. We downscaled two LULCC scenarios (SSP2-RCP 4.5 and SSP5-RCP 8.5) under three levels of irrigation expansion: no expansion (historical extent), moderate expansion (all land presently authorized by a water right is irrigated), and maximum expansion (new water rights are granted to cover all irrigable land). The downscaled scenarios were evaluated using a hydrology-cropping systems model and a reservoir model coupled in a linear fashion to quantify changes in food and bioenergy crop production, hydropower generation, and availability of instream flows for fish. The net changes in each sector were partitioned among climate, land use, and irrigation-expansion effects. We found that climate change alone resulted in approximately 50% greater production of switchgrass for bioenergy and 20% greater instream flow deficits. In the irrigation-expansion scenarios, the combination of climate change and greater irrigated extent increased switchgrass production by 76% to 256% at the cost of 42% to 165% greater instream flow deficits and 0% to 8% less hydropower generation. Therefore, while irrigation expansion increased bioenergy crop productivity, it also exacerbated seasonal water shortages, especially for instream use. This paper provides a general framework for assessing benchmark scenarios of global LULCC in terms of their regional FEW subsystem outcomes.
Food, energy, and water are essential for life. In modern society, it is difficult to imagine describing the process by which essential nutrients move from the earth to the ordinary person’s dinner plate without speaking anything of energy being used to make fertilizer, water being pumped to irrigate cropland, or fuel being used to move food to the grocery store. The interactions among food, energy, and water (FEW) in the face of scarcity define the FEW nexus (Hoff, 2011). Over the last decade, nexus thinking has become the paradigm for discussion around sustainable development and resource security on the global stage (Leck et al., 2015). Examples include the World Economic Forum’s Water Initiative report (WEF Water Initiative, 2011), the International Institute for Sustainable Development report on FEW security (Bizikova et al., 2013), and the U.N. Sustainable Development Goals (Weitz, 2014).
Mutual improvement in human wellbeing and ecosystem functioning will require more efficient use of resources and a greater understanding of tradeoffs and synergies among FEW sectors under societal, economic, and climatic pressures (Rasul and Sharma, 2016). Multi-sector, interdisciplinary computer models are useful to this end (Albrecht et al., 2018; Schull et al., 2020; Reed et al., 2022). These Integrated Assessment Models (IAMs) simulate complex interactions between socioeconomic and biophysical processes across multiple spatial and temporal scales (Fisher-Vanden and Weyant, 2020), and they bring together knowledge from many disciplines to generate decision-relevant information for making multi-objective policy (Harremoes and Turner, 2001).
An important aim of global-scale IAMs is to generate emission scenarios that describe changes in socioeconomic conditions, greenhouse gas emissions, and climate (Moss et al., 2010). The most recent generation of scenarios integrates Representative Concentration Pathways (RCPs) with Shared Socioeconomic Pathways (SSPs) (van Vuuren et al., 2014; Riahi et al., 2017). The RCPs are a set of greenhouse gas concentrations leading to different levels of radiative forcing by 2100 (van Vuuren, 2011). The SSPs, when coupled with climate policy assumptions, provide trajectories for reaching the greenhouse gas concentrations specified in the RCPs (van Vuuren et al., 2014). Each SSP has a storyline that describes the evolution of population, economy, energy, culture, and governance, and is characterized by relative ease of climate mitigation and adaptation (O’Neill et al., 2014; van Vuuren et al., 2014; O’Neill et al., 2017).
Land-use and land-cover change (LULCC) is an important outcome of scenario development and application, and it serves as an essential input for many modelling studies. This is because LULCC can have far-reaching effects on ecosystems (DeFries and Eshleman, 2004; Rickebusch et al., 2011), food security (Moore et al., 2012), and the terrestrial carbon cycle (Sohl et al., 2012). Moreover, LULCC often interacts with environmental factors that are site specific (Hibbard and Janetos, 2013). For example, conversion of natural grasslands to irrigated cropland in a region with declining groundwater could place undue strain on local water resources.
Global models may lack sufficient spatial detail to capture the most relevant features of a region. Accordingly, methods are needed to translate global-scale LULCC projections to regional-scale outcomes that reflect local climate, geography, culture, and institutions (Voisin et al., 2013; Le Page et al., 2016). In multi-scale modelling, this is accomplished by allocating land estimates produced by an aggregate model among smaller spatial units, typically grids that are tens of square kilometers, or even smaller. The downscaled LULCC maps can then be fed into a high-resolution model, such as an Earth system model, that is well suited to answer a set of research questions (West et al., 2014).
Many tools are available for spatial downscaling, including those that use cellular automata (Li et al., 2017), neural networks (Shi et al., 2021), and statistical models (Chakir, 2009). Multi-criteria methods are also commonly used in downscaling applications (Hellman and Verburg, 2011; Sakieh et al., 2015). Disaggregation of land use by multi-criteria procedures requires the identification and weighting of factors that make geographical locations suitable for a given land type (Fu et al., 2018). A suitability score is then computed from the weighted factors and combined with other decision rules to allocate land among grid cells (Ghadikolaei et al., 2012). An example of software that uses criteria-based downscaling algorithms is the Python-based package, Demeter (Vernon et al., 2018). Demeter applies user-defined transition rules and spatial constraints to disaggregate LULCC data from geopolitical regions and large water basins to the users’ desired grid size. Demeter has recently been used to evaluate bioenergy crops vs. afforestation in terms of their carbon sequestration costs and benefits (Cheng et al., 2022) and to study the impact of bioenergy cropland expansion on water security (Wild et al., 2021). In another application, Khan et al. (2020) integrated Demeter with a multi-model framework to explore the energy-water-land nexus implications of strengthening Uruguay’s beef, soy, and rice exports. Their results highlighted the importance of LULCC to the trajectory of food and energy production under different policy assumptions.
The strength of Demeter is its flexibility and ease of use. The multiple steps involved in downscaling are automated by Demeter so that results can be reproduced with minimal user error. Since it is open source, the code can be extended to include new functionality if desired (Vernon et al., 2018). One limitation of the current Demeter code is that hard constraints are not explicitly implemented. In agriculture-rich basins that rely heavily on irrigation, irrigated farmland should not be allocated where there are no water rights. Inclusion of a hard constraint for water rights is therefore an important part of downscaling land-use change in regions where irrigated agriculture is essential to the local economy. Likewise, cropland, both irrigated and non-irrigated, should be constrained by the availability of arable land.
In this paper, we refine Demeter downscaling software by incorporating hard constraints on cropland allocation based on extent of water rights and arable land. We then provide a proof-of-concept for the downscaling methodology within a wider framework featuring specialized cropping systems and reservoir models. The case study for the multi-model framework explores the potential impact of expanding irrigated extent to enhance crop yields, especially bioenergy crops, on hydropower generation and instream flows in the Columbia River Basin (CRB) under different LULCC scenarios.
The Columbia River is intensively managed for flood control, hydropower production, and maintenance of instream flows to support fish migration. Additionally, the Columbia River and its tributaries supply irrigation water for a thriving agricultural economy. These multiple beneficial uses must compete for limited available water. For example, diversion of streamflow for irrigation reduces the amount of water that can be stored in reservoirs for hydropower generation and reduces instream flows that support migratory fish species during critical stages of development. Another tradeoff that emerges under future scenarios of land-use change is between two forms of renewable energy: bioenergy and hydropower. Future climate mitigation scenarios project exponential growth in bioenergy crops as part of policy to reduce carbon emissions (Thomson et al., 2011). While ramping up hydropower would also reduce carbon emissions, constraints on available water may not allow both industries to grow concurrently. Competition for the river’s resources among agriculture, hydropower, and fish presents an ongoing water resources challenge for the CRB, and it is one that will likely intensify under climate change and LULCC.
Water regulation moderates the impact of LULCC on productivity of the basin’s FEW sectors by restricting the expansion of cropland under irrigation. State governments regulate water use in the CRB by issuing water rights that authorize beneficial use according to the doctrine of prior appropriation. Water right documents minimally specify priority date, purpose of use, point of diversion, place of use (POU), and water quantity (Benson, 1998). In the context of spatial downscaling, the most important of these features is water right POU because it restricts the spatial extent of cropland allowed to be irrigated, thereby mitigating the effect of streamflow depletion on instream uses.
Integrated modelling frameworks facilitate better understanding of FEW synergies and tradeoffs (Liu et al., 2017). Scenario testing with these tools can help elucidate implications of various policies aimed at mitigation, adaptation, or enhancing resource security (Howells et al., 2013). However, a persistent challenge is how to involve stakeholders, especially those tasked with resource planning and management, in the process of scenario assessment. One solution is to develop more user-friendly models for analyzing tradeoffs in the FEW landscape and to curate suitable FEW nexus models for stakeholders (Dargin et al., 2019). Decision-support type models include the Water-energy-food Nexus Tool (Daher and Mohtar, 2015) and CLEWs (Howells et al., 2013). Another approach, the one we pursue in this paper, is to compose a multi-model framework from loosely coupled sub-models (Liu et al., 2017). Researchers can use these frameworks to test scenarios and communicate scenario outcomes to stakeholders who can provide feedback, leading to higher quality assessments and crafting of scenarios better calibrated to stakeholder aims.
The objectives of this paper are: 1) to present a spatial downscaling approach for the assessment of global LULCC scenarios in terms of regional impacts on food, energy, and water resources, and 2) to make application of the framework in a case study that focuses on the interconnected hydropower, bioenergy, food crop, and instream flow sectors in a water-limited basin.
This paper contributes to the literature on scenario assessment and FEW impacts by linking benchmark socioeconomic and emissions scenarios to regional FEW subsystem outcomes. Incorporation of irrigation expansion into the assessment scheme highlights competition between sectors for available water, which has clear implications for the FEW nexus. Furthermore, we contribute to the spatial downscaling literature by demonstrating the importance of water rights to the allocation of irrigated cropland in downscaling applications. We do so by describing a highly adaptable downscaling software tool that has been updated to include water right POU as a hard constraint on the expansion of irrigated agriculture. The general framework described in this paper may also facilitate future studies of the influence that water regulatory activities have on FEW subsystems. These activities include, for example, water markets, water right curtailment, negotiation of transboundary treaties, and adjudication of Tribal water rights.
Our case study encompasses the CRB in addition to western Washington State and almost all of Oregon. Figure 1 shows the study area and important FEW sectors in the basin. The CRB covers a large part of four states in the U.S. Pacific Northwest, in addition to the Canadian province of British Columbia. Draining roughly 660,000 km2, the Columbia River is the largest river in the Pacific Northwest (Bureau of Reclamation, 2016) and the fourth largest river in North America by discharge (Ward and Ward, 2004). Hydrology and climate of the Pacific Northwest region are greatly influenced by topography, especially by the orographic effect of the Cascade Range on precipitation patterns (Leung and Ghan, 1998). The Columbia River hydrograph exhibits a strong snowmelt signature, with approximately 60% of runoff occurring in May through July (Kirschbaum and Lettenmaier, 1997).
FIGURE 1. Food, energy and water subsystems of the Columbia River Basin (CRB). The black boundary delineates the CRB, and the light grey area corresponds to the CRB inclusive of western Washington and most of Oregon (extended CRB). The density of irrigated area is in units of km2 per 1/16-degree grid cell. Critical habitat for ESA-listed salmonids are marked with the fish symbols. Locations of hydroelectric dams are shown with a red, crossed circle, with symbol size proportional to the square root of generating capacity.
The Columbia River is managed by a network of reservoirs and hydroelectric dams that provides flood control, generates hydropower, supplies water for irrigation, and maintains streamflow for navigation, recreation, and ecological benefits (BPA, 2001). Construction of the dams has blocked fish migration for the basins’ native salmonid species (Fish Passage Center, 2009), leading to severe declines in salmon from a high of 6–16 million at their peak in the 1880’s to less than 1 million today (Peery, 2012). This is particularly a concern to the basin’s Native American Tribes, for whom the fish have deeply rooted cultural significance and provide a stable food source (Taylor, 1999). Four species of trout and eight species of salmon in the CRB are protected under the Endangered Species Act (ESA) (NMFS, 2020).
Agriculture is an important industry in the CRB, annually generating $10 billion of revenue in Washington State (USDA, 2017) and $7 billion in Idaho (Mahler, 2019). Irrigation accounts for 85% of total water withdrawals in the Pacific Northwest states of Washington, Oregon, Idaho, and Montana (Dieter et al., 2018). The majority of irrigated cropland is located in the Yakima Basin and Columbia Basin Project of eastern Washington State, the Snake River Basin of southern Idaho, and Oregon’s Willamette Valley.
Energy production in the basin depends greatly on the many hydroelectric dams along the Columbia mainstem and its largest tributary, the Snake River. This cheap and clean source of energy accounts for approximately 50% of energy production in the Pacific Northwest (EIA, 2020). Biofuel constitutes a small percentage (<1%) of total production (EIA, 2020). To achieve carbon reductions in the CRB, the energy sector could assimilate more renewable feedstocks, like agricultural residues and dedicated bioenergy crops. Switchgrass has received attention as a potential bioenergy crop because it produces large quantities of biomass, can be grown on marginal lands, and requires relatively little agricultural inputs (McLaughlin and Kszos, 2005).
Water rights in the CRB are regulated according to prior appropriation. Under prior appropriation water law, states issue water rights that authorize water users to withdraw water from a source, over a limited season, for enumerated purpose(s) of use, in a given amount, and to apply it within specified place(s) of use (Benson, 1998). An essential tenant of prior appropriations is that water rights established earlier in time must be satisfied before rights established later in time (Schilling, 2018). State-adopted instream flow rules are enforced via curtailment of interruptible water rights (those with priority dates later than establishment of the flow rule) whenever streamflow falls short of the flow rule (Geller, 2014; Sessions, 2017).
We used a multi-model approach to simulate water for irrigation, water for hydropower, and water for instream flow. Each of these subsystems was simulated with specialized regional models. We included scenarios of irrigation expansion (see Section 2.3.1) to amplify one-way interactions among subsystems, and these interactions were evaluated by comparing changes in FEW metrics due to irrigation expansion, climate change, and LULCC (see Section 2.4.5). We simulated the food subsystem in terms of crop production for groups of major food crops grown in the CRB, the energy subsystem in terms of switchgrass crop production (bioenergy) and hydropower generation, and the water subsystem in terms of instream flow deficit and irrigation demand. The multi-model framework integrates the FEW subsystems by means of a soft coupling between a hydrology-cropping systems model and a reservoir model, i.e., water supply and demand from the former is used as input for the latter. Both models have been evaluated in previous publications (see Section 2.4.1 and Section 2.4.4).
Figure 2 shows the workflow used for scenario assessment, divided into three stages: global energy-economic modelling, LULCC downscaling, and FEW subsystem modelling. In the first stage, an IAM called the Global Change Analysis Model (GCAM) (Calvin et al., 2014; Calvin et al., 2019) was used to generate LULCC projections consistent with the SSP2-RCP 4.5 and SSP5-RCP 8.5 scenario storylines (Graham et al., 2020). A brief summary of GCAM and its applications can be found in the supplementary material (Supplementary Section S1). The second stage, shown in the purple-shaded box of Figure 2, illustrates scenario development and disaggregation of LULCC projections from regional to grid scale at 1/16th-degree (∼36 km2) resolution using the spatial downscaling software, Demeter (Vernon et al., 2018). The two socioeconomic and emissions scenarios from GCAM (SSP5-RCP 8.5 and SSP2-RCP 4.5) were combined with three irrigation scenarios (no, moderate, and maximum expansion) endogenous to Demeter. Storylines for each of the scenarios (two baselines and six integrated scenarios) are given in Section 2.3.1. Demeter is described in Section 2.3.2, including modifications made to accommodate hard constraints.
FIGURE 2. Spatial downscaling and multi-model workflow. Elements in the purple-shaded box illustrate downscaling LULCC projections from GCAM (grey hexagon) with Demeter (yellow hexagon). The scenarios shown in the Venn Diagram are constituted of LULCC scenarios from GCAM and irrigation scenarios endogenous to Demeter. FEW subsystems modelling is shown in the blue-shaded box. The cropping systems and hydrology model, VIC-CropSyst (green hexagon), simulates naturalized streamflow, irrigation demand, and crop yield. Naturalized streamflow and water demand drive the reservoir model, RColSim (orange hexagon). Outputs from the two models are then used to calculate FEW metrics.
The case study of FEW subsystems in the CRB is represented by the blue-shaded box in Figure 2. Demeter-downscaled maps were used to parameterize land use/cover in the grid-scale, hydrology and cropping systems model, VIC-CropSyst (Malek et al., 2017). The model was run twice for each scenario, once with irrigation turned off to simulate water supply and once with irrigation turned on to simulate irrigation demand and crop yield for food, bioenergy, and forage crops. VIC-CropSyst irrigation demands and estimates of consumptive municipal water use were partitioned between groundwater and surface water to estimate surface water demand (Section 2.4.2). When irrigation is turned off, the CropSyst portion is not invoked, and only the hydrological components of the model (VIC portion) are engaged. Surface runoff and base flow from VIC supply runs were routed to stream gauges throughout the CRB and bias-corrected at a weekly timestep to estimate naturalized flow (Section 2.4.3). Next, naturalized flow and surface water demand simulated with VIC-CropSyst were used to drive the reservoir model, RColSim (Malek et al., in review), which simulates regulated flow with irrigation withdrawals removed, from which instream flow deficit is derived, and hydropower generation on a weekly timestep (Section 2.4.4). The RcolSim model outputs were combined with crop production output from VIC-CropSyst to compute FEW metrics. These metrics were aggregated over the study area and adjusted to remove climate model bias. Finally, the changes in FEW metrics under the integrated scenarios were evaluated (Section 2.4.5).
We evaluated eight scenarios (two baselines and six integrated LULCC-irrigation scenarios) to demonstrate the role of LULCC, climate change, and irrigation expansion in the simulation of regional FEW systems (Table 1). Our approach to scenario-based analysis differed from an ensemble approach wherein results from multiple downscaling parameter sets and multiple climate models are jointly assessed to generate a prediction with quantified uncertainty. Rather than prediction, we tested how the FEW sectors would respond under specific storylines. Each of the scenarios are described in this section, along with storylines for the six integrated scenarios.
TABLE 1. Scenario implementation. There are two baseline scenarios which assume historical climate, irrigated extent, and land use. The remaining six scenarios assume combinations of three irrigation levels and two LULCC scenarios from GCAM over the period 2015-2100. Each of these scenarios was forced by an observed historical (GridMet/Livneh), modeled historical (CNRM-CM5 historical), and modeled future (CNRM-CM5 RCP 4.5/RCP 8.5) climate dataset.
The first baseline scenario assumes historical conditions of land use, irrigated extent, and climate. This scenario uses observed land-use data directly, without any downscaling. Land-use observations were derived from multiple sources, including the Cropland Data Layer (USDA-NASS, 2016) and the Agricultural Land Use dataset (WSDA, 2016). Historical irrigated extent for the U.S. portion of the study area was derived from IrrMapper (Ketchum et al., 2020) for the year 2018, as well as from the 2016 Agricultural Land Use dataset, which distinguishes between irrigated and dryland agriculture. For the Canadian portion of the study area, irrigated extent was determined following methodology used to create the 2017 MODIS Irrigated Agriculture Dataset (MIrAD) for the contiguous U.S. (Brown and Pervez, 2014) as reported in Hills et al. (2020).
The second baseline scenario is also based on historical conditions of land use, irrigated extent, and climate. However, the GCAM baseline scenario uses historical irrigated extent and 2015 land-use data that have been spatially downscaled and harmonized to match observed land use due to differences in land-use classification between the GCAM and high-resolution datasets; therefore, the GCAM baseline scenario does not perfectly match input from the observed baseline dataset (see Section 2.3.2.2). Additionally, we ran a simplified set of crops for sake of computational efficiency (see Section 2.4.1.4). We include both baseline scenarios to provide a sense of how these sources of error impacted our analyses.
The RCP 8.5 no expansion scenario is based on a high-emissions pathway without climate mitigation, and without any irrigation expansion. The heavy reliance on fossil fuels in the absence of climate policy in this scenario leads to greenhouse gas emissions and atmospheric concentrations consistent with the RCP 8.5 pathway (Riahi et al., 2011). The social and economic conditions underlying RCP 8.5 no expansion are consistent with SSP5 (Kriegler et al., 2017). Challenges for climate mitigation are high under SSP5 due to fossil-fuel based development and lack of environmental concern, but the challenges for adaptation are low due to rapid technological progress, strong investment in education, and high levels of global market integration (Kriegler et al., 2017; O’Neill et al., 2017). Both population and per capita calorie consumption increase in high-income nations, resulting in higher food demands. Agricultural growth, however, is driven mostly by replacement of traditional biomass with lingo-cellulosic feedstocks derived from non-food crops and agricultural residues (Kriegler et al., 2017). The addition of “no expansion” to the SSP5-RCP 8.5 storyline implies sustained interest among policymakers and the public in protecting or enhancing fish habitat and continuing fish recovery efforts. Accordingly, new land for dedicated bioenergy crops does not increase irrigated extent beyond historical levels.
The RCP 8.5 moderate expansion scenario follows the same pathways (SSP5 and RCP 8.5) and future climate conditions as RCP 8.5 no expansion. However, lower priority is placed on instream flow protection, and higher priority is placed on bioenergy production. As a result, irrigated area is allowed to expand to improve crop yields. Rather than irrigation being constrained to historical extent, it is permitted on all land currently authorized by a water right. There are multiple reasons why the area actually irrigated may not coincide with the POU printed on a water right document. These include land that has voluntarily been taken out of irrigated management as part of an instream water transfer or lease program, water rights that have not been perfected (i.e., the infrastructure is not yet in place), and water rights that have been fully or partially relinquished for non-use. Under moderate expansion, irrigated cropland can fill any parcel of land authorized by a water right. The locations of water right POUs were obtained from the databases of respective governments’ departments of ecology. These spatial datasets are the Geographic Water Information System (Ecology, 2018) for Washington State, the “Statewide Water Right Spatial Data” (OWRD, 2018) for Oregon, the “Place of Use: Water Right” dataset (IDWR, 2018) for Idaho, “Montana Water Rights” dataset (Montana DNRC, 2018) for Montana, and the “Land Parcels with Water Licenses” dataset for British Columbia (LWRS, 2018).
The RCP 8.5 maximum expansion scenario also follows the SSP5 pathway; however, it assumes there will be very little protection for instream flows, and bioenergy production is prioritized over fish and hydropower. The only restriction on irrigation is land suitability, meaning that all irrigable land is granted a water right. Land suitability for irrigation was determined from the Soil Survey Geographic (SSURGO) irrigated capability class (Soil Survey Staff(a), 2019). The maximum-irrigation scenario should not be considered a likely development but one which provides an upper bound for the impact of irrigation expansion.
The RCP 4.5 no expansion scenario follows a biomass-focused pathway to climate mitigation. It is consistent with stabilization of radiative forcing at 4.5 W/m2 (Thomson et al., 2011). The underlying social and economic conditions follow the SSP2 pathway, resulting in intermediate challenges for climate adaptation and mitigation. Climate policy incentivizes bioenergy production with carbon capture and storage (Fricko et al., 2017). Population growth is moderate and levels off in the second half of the century. Medium levels of human development and continued environmental degradation create obstacles to adaptation, while limited reliance on fossil fuels makes mitigation moderately difficult (O’Neill et al., 2017). Demand for bioenergy is met without expanding irrigation, reflecting the need to balance instream needs of fish and hydropower with consumptive needs of agriculture.
Socioeconomic, emissions, and climate trajectories in RCP 4.5 moderate expansion follow those of the RCP 4.5 no expansion scenario. There is large growth in irrigation to improve agricultural productivity and to increase the carbon capture and storage potential of dedicated bioenergy crops. However, no new water rights are granted, limiting potential streamflow impairment by new cropland.
The RCP 4.5 maximum expansion scenario follows the RCP 4.5 and SSP2 pathways. Large-scale expansion of bioenergy cropland in combination with unrestricted access to water rights creates large carbon sequestration benefits and optimal conditions for bioenergy production. This comes at the cost of water availability for instream use. In this scenario, pursuit of climate mitigation goals mostly precludes efforts to ensure fish survival or to maintain hydropower reliability.
The Historical baseline and GCAM baseline scenarios were evaluated with historical climate data derived from GridMet (Abatzoglou, 2013) for the U.S. portion of the study area and Livneh et al. (2013) for the Canadian portion. The Livneh dataset includes daily maximum and minimum temperatures, precipitation, and average wind speed. GridMet also provides shortwave solar radiation and daily minimum and maximum relative humidity. The reason for using GridMet rather than the Livneh dataset, where available, is that GridMet has a smaller cold-temperature bias in topographically complex landscapes like the Pacific Northwest (Behnke et al., 2016). The six integrated scenarios were evaluated under both historical and future climate conditions. Historical simulations were forced using the GridMet and Livneh datasets, while future simulations were forced using climate projections representing mid-range changes in precipitation and temperature. To find these mid-range values, we screened 17 global circulation models (GCMs) from the Multivariate Adaptive Constructed Analog downscaled climate dataset (Abatzoglou and Brown, 2012) and selected the GCM that produced mid-range climate projections in the study region, under both the RCP 8.5 and RCP 4.5 emissions pathways. The selection process consisted of first ranking each GCM with respect to temperature, precipitation, and runoff generation, then summing the ranks, and finally choosing the GCM corresponding to the median of the rank sums. The GCM we chose through this selection process was CNRM-CM5. Each GCM dataset comes with a historical reference (1950–2005) and a future (2006–2094) time series.
The GCAM-based LULCC projections were downscaled from regional scale (U.S. and Canadian regions of the extended CRB) to 1/16th-degree grid scale. We used Demeter, a Python-based downscaling software package that assimilates well into new multi-model workflows. Prior to downscaling with Demeter, land use in the base year is harmonized between the IAM and an observed historical land-use map so that the land categories match between the two data sources. The harmonized base-year land use is then compared to land demand from the next timestep, and target change is computed. Transition rules are used to distribute target land-use changes in each timestep at the resolution of the historical land-use map, subject to user-defined spatial constraints. The transition rules consist of treatment order, i.e., the order in which land classes are downscaled; transition priority, which assigns preferences for which types of land use convert to which; spatial constraints relating to suitability of land for a particular land use; kernel density reflecting the land-use composition of neighboring grid cells; and intensification (increase in a grid cell where the increasing land use exists) vs. expansion (increase in a grid cell where it did not exist previously). Once the required land-use transitions have been achieved via intensification and expansion, the downscaled land areas for the current timestep become the baseline land areas for the next 5-yr timestep. A full description of transition rules can be found in Le Page et al. (2016); Vernon et al. (2018). Refer to supplementary material for a summary of the Demeter model components (Supplementary Section S2; Supplementary Figure S1). In this section, we describe how hard constraints were added to the original code and discuss model parameterization.
Hard constraints restrict the area available for any given land use. Even if a grid cell is highly suitable for cultivation on average, the land could contain a mixture of poor and fertile soils such that most of the area is suitable, while a sizeable minority is not. In this case, the amount of land allocated to crops should not exceed the total amount of suitable area in that grid cell. Similarly, irrigated cropland should not be allocated in excess of land with a water right for irrigation. Implementation of hard constraints consists of four steps. First, the land area available for conversion to land use k in grid cell i (
The term
where
The term
where
The potential growth of land use k in grid cell i (
where the term
where
In the third step, target expansion of land use k is allocated among candidate grid cells using Eqs 1–8. However, for expansion, the candidate cells satisfy the following condition:
The difference from Equation 4 is that land use k does not exist in candidates for expansion, so
The above four-step procedure is repeated for all ‘convert-from’ land classes (m’s), either until the target growth of land class k has been achieved, or until there is no space available for land use k in any grid cell, whichever comes first. Then, the next land use in the treatment order with a positive target change (the next land use k) undergoes intensification and expansion according to the above procedure, and the process repeats until all required land-use transitions have been simulated for a given timestep. The processes of expansion and intensification with a hard constraint are illustrated in Figure 3.
FIGURE 3. Land-use transition with hard constraints in the updated Demeter code. Land use k (increasing) can replace land use m (contracting) only to the point at which the area of land use k equals the fraction of the grid cell satisfying conditions of the hard constraint (
The coefficient matrices used for mapping observed land types and GCAM land types to common land types are provided in Supplementary Tables S1, S2. The reclassified dataset derived from the observed land use/cover did not perfectly match the reclassified dataset derived from GCAM in terms of the total areas within each land class. The Demeter code contains an algorithm that rescales the GCAM data so that the two reclassified datasets match (see Chen et al. (2020) for details). However, we were working with an earlier version of the code that did not contain this rescaling algorithm. Instead, we harmonized the two datasets by manually adjusting the matrix coefficients until the reclassified land use/cover from observations were close to the reclassified land use/cover from GCAM.
The treatment order we chose gave highest priority to high-value crops, like corn, that are always irrigated. Next priority was given to medium-value crops that are sometimes irrigated, followed by low-value crops like wheat. Bioenergy crops were assigned the last treatment order among crop types because crop price data simulated with PRIMA (Kraucunas et al., 2015) indicate bioenergy crops will have lower value than food crops, even under RCP 4.5. Urban, shrubs, and forest were placed last in the treatment list (Supplementary Table S3). We chose transition priority rules consistent with West et al. (2014). This meant that when considering expansion of farmland, we prioritized conversion of grassland over forest and urban land (Supplementary Table S4). The most sensitive parameter in Demeter is the intensification ratio (Chen et al., 2019b). We used a ratio of 0.8 as suggested by West et al. (2014), meaning that 80% of the target growth was achieved via intensification.
We parameterized Demeter with the following spatial constraints: irrigated and dryland capability class, total arable land, total irrigable land, and total marginal land. The term “total” in the latter three constraints designates their operation as hard constraints. The first two constraints, together with kernel density, are the suitability factors (
Irrigated cropland and dryland crop suitability were determined from the land capability classifications of SSURGO (Soil Survey Staff(a), 2019). There are eight capability classes in order of decreasing suitability. Classes I-IV are generally suited to cultivation, while classes V and VI are only suited to some specialized crops and native plants, and classes VII and VIII are restricted in their use to recreation, wildlife, and grazing (Soil Conservation Service, 1961). Dryland crop suitability was supplemented with climate suitability. Climate suitability was calculated from GridMet climate data as the green-water availability ratio, which is the ratio of water supply (growing-season precipitation plus water-holding capacity) to water demand. Water demand for wheat and hay was determined as the actual evapotranspiration from a well-watered crop, following the method of Allen et al. (1998). We rescaled the suitability factors from 0 to 1 according to Supplementary Table S5. The criteria weights for the suitability factors are given in Supplementary Table S6.
The total area of land within non-irrigated capability classes I-VI or irrigated capability classes I-IV was used as a hard constraint on the expansion of arable land. The hard constraint for irrigated land area was formulated as the minimum of total land with a water right determined from the water right POU and the total area within irrigated capability classes I-IV. Marginal land was defined as any land in non-irrigated capability classes III-VI or irrigated capability classes IV-VI. Marginal land was imposed as a hard constraint for bioenergy crops since we assumed that they would not be competing with food crops for prime farmland. Hard constraint binary weights for each land type are given in Supplementary Table S7.
The hard constraint on irrigated cropland controls the split of irrigated vs. dryland crops. Some crops, such as corn and potatoes are always irrigated, so a hard constraint was enforced to prevent expansion into land without a water right, and these crops were assigned an irrigated fraction of 1. Other crops are rarely irrigated (e.g., oilseed crops), so their irrigated fraction was assumed to be zero. For the remaining crops that are not always irrigated (cereal crops, hay, fruits and vegetables), the maximum irrigated area allowed by the hard constraint was satisfied first, and then any remaining cropland was assumed to be non-irrigated.
Water supply and demand was simulated at a daily timestep and 1/16th-degree resolution using the tightly-coupled hydrology and cropping systems model, VIC-CropSyst. The model was developed to study the interplay between agricultural decision-making, climate, and hydrologic systems. The VIC portion of VIC-CropSyst (Liang et al., 1994) is a large-scale, process-based water and energy balance model. It has been used extensively in climate change studies in the Pacific Northwest (Hamlet et al., 2010; Mantua et al., 2010), as well as in the nearby Colorado River Basin (Christensen and Lettenmaier, 2007) and Sierra Nevada of California (Maurer, 2007). The CropSyst portion of VIC-CropSyst simulates growth and phenology of numerous annual and perennial crops under both irrigated and dryland conditions and under various management practices (Stöckle et al., 2003). A unique feature of VIC-CropSyst is its rigorous representation of cropping systems within a land surface model. The model has process-based representations of the hydrologic, carbon, and nitrogen cycles. The coupling of VIC with CropSyst is described by Malek et al. (2017). VIC-CropSyst has been used to study climate change impacts on irrigation demands and crop yields in the CRB (Rajagopalan et al., 2018), impacts of efficient irrigation technologies on performance of FEW sectors in the Yakima Basin (Malek et al., 2021), and climate change impacts on inter-annual crop yield variability and revenue volatility (Malek et al., 2020).
The data source for U.S. soils was STATSGO2 (Soil Survey Staff(b), 2019), and the soils data for the Canadian portion of the study area came from the Land Data Assimilation System (Mitchell et al., 2004). Historical land-use data were obtained from three sources: the 2016 Cropland Data Layer (USDA-NASS, 2016), the 2016 Washington Agricultural Land Use dataset (WSDA, 2016), and the Annual Crop Inventory Database (Agriculture and Agri-Food Canada, 2016), the first two being for the U.S. portion and the last being for the Canadian portion. Future land cover came from the Demeter-downscaled LULCC scenarios. VIC-CropSyst currently requires fixed land cover, so we ran all future scenarios with 2060 downscaled land-use/cover inputs. The VIC runs for generating water supply used a different land-cover parameterization than the runs for generating irrigation demands and crop yields. For VIC supply runs (irrigation turned off), the nearly 100 crop types in the Demeter-downscaled input files were aggregated to a single crop type to match VIC land categories, which identify all field crops as corn.
VIC has been calibrated and evaluated in the topographically complex, Pacific Northwest region (Elsner et al., 2010; Hamlet et al., 2013). The calibration and evaluation of streamflow for VIC is reported in Adam et al. (2022). Detailed crop calibration data and procedures for CropSyst used in this study are reported in Adam et al. (2022). However, that calibration set did not include bioenergy crops, therefore, we calibrated switchgrass (see supplementary material, Supplementary Section S3 and Supplementary Figure S2).
Historical and future climate forcing data were obtained in the manner described in Section 2.3.1.9.
VIC-CropSyst provides “top-of-crop” water demand, which is the water applied on the field. It does not include water lost in conveyance (e.g., seepage through canal lining). We excluded conveyance losses from the demand calculations, assuming that leakage from canals returns to the river network and becomes available for downstream in- and out-of-stream uses.
Crop yields and irrigation demands were calculated from VIC-CropSyst outputs using a two-step procedure. In the first step, area-weighted, average irrigation depth and yield were calculated for each of the CropSyst crop types according to Equation 10,
where
where
A portion of irrigation withdrawals are satisfied from a groundwater source and do not significantly impact streamflow. Thus, groundwater demands were removed from VIC-CropSyst irrigation demands and from consumptive municipal use prior to reservoir modelling and calculation of instream flow. See Supplementary Section S4 for a detailed description of how these splits were determined. The basin-wide average split determined by these methods for consumptive water use was 80% surface water and 20% groundwater.
Runoff and base flow simulated for each VIC grid cell were routed to 66 stream gauge locations selected from the Columbia Basin Climate Change Scenario Project (Hamlet et al., 2013). Daily streamflow was generated using the methodology of Lohmann et al. (1996). Bias in routed streamflow prediction owing to model structural uncertainties, as well as uncertainties in the meteorological inputs and calibration parameters, was corrected at monthly and annual timesteps to the no-regulation, no-irrigation (NRNI) dataset (BPA, 2014) using the methodology of Snover et al. (2003). The resulting bias-corrected, monthly flows were converted to weekly flows. This was achieved via multiplication of the daily routed flows by the ratio of monthly bias-corrected to monthly routed flows. Then, the resultant ‘bias-corrected’ daily flows were aggregated to a weekly timestep. The result of these steps was weekly, bias-corrected, naturalized streamflow.
The Columbia River hydrograph has been significantly altered by an extensive system of reservoirs and hydroelectric dams. The influence of these dams was modeled using a version of ColSim (Hamlet and Lettenmaier, 1999), a reservoir model for simulating operations of major dams to meet multiple objectives including flood control, hydropower production, maintenance of environmental flows, navigation, and recreation. The new version implements ColSim algorithms in the open-source computational software, R (Malek et al., in review). RColSim simulates the management of major storage and run-of-river dams along the Columbia River starting at Mica Dam, near the headwaters in British Columbia, and ending at Bonneville Dam in Oregon. It also includes dams along the Snake, Kootenai, Clark Fork, and Pend O’reille tributaries (see Supplementary Figure S3).
RColSim combines naturalized flow and surface water demand inputs with dam operating rules to produce regulated flow and hydroelectricity generation outputs. Dam operating rules for hydropower production, flood control, and flow targets are mostly the same as those used by Hamlet and Lettenmaier (1999), with minimal modification to capture important changes to the operating rules (Alan Hamlet, personal communication). The operating rule curves are chosen by the model based on the annual runoff forecast to ensure sufficient flood evacuation in winter and refill by end of summer. The mass balance for a reservoir in RColSim is calculated according to Equation 12,
where
The food sector was evaluated on median annual crop production of food crops aggregated to six groups (fruits, vegetables, potatoes, wheat, corn, and other grains) (more details on the calculation of mean or median can be found in the end of this section). We evaluated impacts on the energy sector by calculating mean monthly hydropower generation and median annual production of switchgrass. The water sector was evaluated on median monthly irrigation demand (including groundwater irrigation) and mean monthly Columbia River flow deficit calculated over a 30-year period.
The hydropower generation (
Where the hydropower generated at a single dam is calculated as the flow of water passing through the turbine (Q) multiplied by the product of net head (h), the specific weight of water (
Both hydropower generation and instream flow deficit were aggregated from a weekly to a monthly timestep, and the mean monthly value was calculated.
For all metrics, a median or mean of the metric values at the monthly or annual time scale was taken over the appropriate 30-yr time frame. For CNRM-CM5 historical climate data this was 1976–2005, for future CNRM-CM5 data it was 2046–2075, and for historical GridMet/Livneh data it was 1986–2015. Following calculation of the mean/median, we bias-adjusted the results according to the method described in the next sub-section.
The CNRM-CM5 data, like data from any GCM, has bias. We adjusted for the impact of bias on our metrics by using either the difference method (Equation 15) or the ratio method (Equation 16):
where M represents a given metric, the subscript i denotes the irrigation scenario (no expansion, moderate expansion, or maximum expansion), the subscript l denotes the land-use scenario (RCP 8.5/4.5). The future subscript denotes the irrigation and land-use scenario run with future CNRM-CM5 climate inputs, the historical subscript indicates that same scenario using historical CNRM-CM5 climate data, and the GridMet subscript indicates that scenario using observed GridMet/Livneh climate data. The prime (ʹ) designates the metric prior to bias-adjustment.
The difference method often produces negative values during low flows. To avoid this, we adjusted Columbia River instream flow deficits using the ratio method (Equation 16).
The changes in metrics for food, energy, and water were compared across scenarios to evaluate impacts of LULCC, climate, and irrigation expansion. We partitioned the net change in each of the food, energy, and water metrics into its climate (
where the baseline subscript denotes the GCAM baseline scenario (2015 SSP5-RCP 8.5 land use and historical climate forcing). All differences were normalized by the GCAM baseline scenario. It is noteworthy that
We created spatially downscaled, integrated scenarios of LULCC and irrigation expansion for the extended CRB. Since it provides necessary context for the downscaled scenarios, Section 3.1.1 presents trends in the GCAM LULCC data from 2015 to 2100. These include projections under the high-emissions (SSP5-RCP 8.5) and climate mitigation (SSP2-RCP 4.5) scenarios. Section 3.1.2 describes the spatial distribution of land-use/cover trends and irrigation intensity under the integrated scenarios. For simplicity, when discussing the integrated scenarios, we will refer to SSP2-RCP 4.5 as RCP 4.5 and SSP5-RCP 8.5 as RCP 8.5. However, when referring specifically to the LULCC scenarios from GCAM, we will keep the full name.
GCAM projected large increases in bioenergy across the study area, even for SSP5-RCP 8.5, which does not assume a global policy on carbon emissions. Bioenergy under this scenario increased from a baseline of zero in 2015 to approximately 40,000 km2 by 2060 and remained nearly constant afterward through 2100 (Figure 4). The corresponding increase under the mitigation scenario (SSP2-RCP 4.5) was larger, with approximately 50,000 km2 planted by 2060. The trend after 2060 was an exponential increase in bioenergy to approximately 240,000 km2 by 2100. This exponential increase was achieved by conversion of shrubs, grasslands, and forests. Therefore, forests and grasslands decreased exponentially after 2060 under SSP2-RCP 4.5.
FIGURE 4. Projected trends in land use/cover across the extended CRB over the 21st century. Results are shown for two GCAM LULCC scenarios: SSP5-RCP 8.5 and SSP2-RCP 4.5.
The amount of land allocated for food production followed trends unique to each SSP storyline. Under SSP2-RCP 4.5, the area steadily increased from 2015 to 2040, reaching a plateau of 33,000 km2. In contrast, the food cropland under SSP5-RCP 8.5 decreased from 30,000 km2 in 2015 to 28,000 km2 by 2040 but increased after 2050. The difference in trends was due to differences in the assumptions regarding population growth, agricultural technology, and per capita food consumption between the SSPs. Population is projected to grow until 2050 under both SSP2 and SSP5 (O’Neill et al., 2017); however, agricultural productivity increases rapidly in SSP5, leading to a decline in the area required to meet food demands. After 2050, population stabilizes in SSP2 (Fricko et al., 2017) but continues to increase slightly in SSP5 (Kriegler et al., 2017). This, combined with the greater per capita calorie consumption rates under SSP5, led the food cropland requirement under SSP5-RCP 8.5 to increase after 2050 and eventually catch-up by the end of the century.
Land dedicated to pasture and growing forage crops like alfalfa hay was projected under both GCAM scenarios to increase in a nearly linear fashion over the 21st century from 11,000 km2 in 2015 to 17,000 km2 by 2100 under SSP5-RCP 8.5 and to 20,000 km2 by 2100 under SSP2-RCP 4.5. These growth trends follow from assumptions of growth in demand for cattle feed to supply meat-rich diets, especially under SSP5 (Kriegler et al., 2017). The “other” land category includes land that could be cultivated in addition to non-arable land categories like rock/ice/desert. The trend for this category mirrored that of grass, shrubs, and forest, as marginal lands were converted to grow bioenergy crops.
Figure 5 shows spatially downscaled LULCC in each of the more than 20,000 model grid cells for the more than 100 VIC-CropSyst land categories grouped under forest, grasses and shrubs, forage, other, food, and bioenergy land categories. The highest food and forage crop densities were located in four major agricultural regions in the CRB: The Willamette Valley of Oregon, southern Idaho’s Snake River Basin, central and eastern Washington, and the Palouse dryland cropping region of eastern Washington and north central Idaho. While there was modest growth in food and forage in these regions, bioenergy crops contributed most to agricultural intensification, mainly by replacing grasslands, shrubs, and forest on marginal lands. The transition from forest to bioenergy was especially stark for RCP 4.5. By 2100, large swathes of forest in western Washington and Oregon were converted to trees harvested for biomass. It is important to note that GCAM includes two categories of biomass: grasses and trees. For simplicity and due to model limitations, we converted all the tree biomass to switchgrass for crop simulations.
FIGURE 5. Spatially downscaled LULCC trends and six major land-use/cover categories under the RCP 8.5 and RCP 4.5 pathways. Trends are given in terms of difference from the 2015 base year. The grey area is the extended CRB, which includes land in western Washington and Oregon lying outside the CRB boundary. The black boundary demarcates the CRB proper.
The spatial extent of water rights controls the area of land authorized for irrigation. Since the no-expansion scenario constrains total irrigated area to the 2018 level of 34,000 km2, change in the total amount of irrigated land under either RCP 4.5 no expansion or RCP 8.5 no expansion was negligible (see Figure 6). When the irrigated area was allowed to increase on land with an existing water right (moderate expansion), irrigation intensified in eastern Washington and the Snake River Basin (Figure 6A). After an initial 10,000 km2 spike in irrigated area due to maximizing irrigated area within existing POUs, irrigated extent continued increasing over time, from 44,000 km2 in 2020 to 50,000 km2 by 2100 under the RCP 8.5 moderate expansion scenario and from 44,000 km2 in 2020 to 53,000 km2 by 2100 under the RCP 4.5 moderate expansion scenario, driven largely by irrigated bioenergy crops for both scenarios (Figure 6B). The approximately 50% increase in irrigated area under moderate expansion compared to no expansion indicates large potential for irrigation expansion under existing water rights, without the need for issuing new ones.
FIGURE 6. Time series of total irrigated area under RCP 4.5 and RCP 8.5 LULCC scenarios and three irrigation expansion scenarios: no expansion (2018 irrigation), moderate expansion (constrained by water right place of use), and maximum expansion (constrained by SSURGO irrigated land capability class). Panel (A) shows the spatial distribution of changes in irrigated area from contemporary irrigated extent and panel (B) gives total irrigated area over the entire study area in 5-year increments from 2015 to 2100.
In addition to intensification of irrigated agriculture, Figure 6A also reveals some areas where the irrigated area is less under moderate expansion than under no expansion. This is most notable in the southwestern part of the basin, in Oregon’s Willamette Valley. This counterintuitive result is due to lack of agreement between estimates of water right POU extent and satellite-based estimates of irrigated extent. When estimating the historical irrigated extent, we assumed that high-value crops like fruits, vegetables, seed crops, and alfalfa are always irrigated. This resulted in assigning some agricultural lands to the irrigated category despite the absence of an existing water right. The implication is either that we misclassified non-irrigated land as irrigated by this process or that the POU data were incomplete. Since it would not be feasible to investigate each grid cell to definitively determine the source of error, we had to either adjust the data so that the two datasets matched or accept some level of uncertainty. Our approach was to accept small uncertainty and to determine POU extent and historical irrigated extent by independent methods. Consequently, there were notable hotspots where historical irrigated area exceeded POU area within individual grid cells. Across the extended CRB region, these hotspots, when defined as historical irrigated area exceeding POU area by more than 1% of a grid cell, occurred in 9% of irrigated grids. Had we chosen to harmonize the water right POU with the historical irrigation datasets, either by increasing the POU extent or by decreasing the historical irrigated extent, changes in the FEW metrics from baseline would have been equal to or greater in magnitude than we report in Section 3.2.
Under maximum-expansion scenarios, new land was brought under irrigation in areas formerly covered by shrubs, grassland, and forest. The maximum-expansion scenarios showed an almost 300% increase in irrigated area by 2040 for both RCP 8.5 and RCP 4.5 compared to baseline, driven by conversion of marginal lands in the main agricultural regions to irrigated switchgrass. The growth slowed after 2040 for RCP 8.5, resulting in 121,000 km2 by 2100, but it accelerated under RCP 4.5 due to exponential bioenergy growth, such that there was 190,000 km2 of irrigated land by 2100 (Figure 6B).
The spatially downscaled LULCC scenarios provided the necessary land-use/cover parameterization for conducting our case study, which evaluated climate and LULCC impacts on select Columbia FEW subsystems. Expanding irrigated acreage to increase food crop and bioenergy crop production resulted in a decrease by 0% to 8% in hydropower generation and an increase by 9% to 165% in Columbia River instream flow deficits, when also accounting for the effects of climate and land use.
We used statistically downscaled climate projections from the CNRM-CM5 GCM to simulate middle-of-the-road changes in temperature and precipitation. By the 2060’s, the mean annual temperature is projected to increase by 2.1°C to 3.6°C with a mean of 3.0°C under the RCP 8.5 emissions pathway across all grid cells in the study region and by 1.6°C to 2.9°C with a mean of 2.2°C under the RCP 4.5 pathway. The mean annual precipitation is projected to increase by 396 to −121 mm/year with a mean of 56 mm/year (RCP 8.5) and by 290 to 121 mm/year with a mean of 40 mm/year (RCP 4.5). The spatial variation in climate projections by the 2060’s is shown alongside historical temperature and precipitation in Supplementary Figure S4. Climate change in isolation generally led to higher crop yields, greater instream flow deficits, and less hydropower generation in summer and fall.
The response of food crop production to land use, climate, and irrigation varied considerably by crop category. Overall, the only crop showing an increase in production across all six future scenarios was wheat. Fruit, vegetable, and potato production declined under RCP 8.5 due to a decline in land area dedicated to those crops. Corn was the only crop for which the yield impact of climate was consistently negative.
The difference in food crop production between scenarios with different levels of irrigation expansion but the same land use (e.g., RCP 4.5 maximum expansion and RCP 4.5 no expansion) was most pronounced for crops, like wheat, that are grown under both irrigated and non-irrigated conditions (see Figure 7). Expanding irrigation resulted in a greater percentage of irrigated vs. dryland wheat, thus increasing wheat yields overall. In contrast, we assumed that crops like corn, potatoes, and fruit trees are always irrigated, leaving no room for their irrigated fractions to increase. While climate change (inclusive of elevated CO2) had a positive influence on all wheat yields (see Table 2),
FIGURE 7. Annual crop production for major food crops in the extended CRB. Total production, both irrigated and dryland, was aggregated from CropSyst crop types to the six crop groups, and the median annual production for each crop group was calculated over the appropriate 30-yr time frame for future scenarios (2046–2075) or baseline scenarios (1986–2015). The black horizontal line within (or above) each column marks the crop production value for that scenario using historical GridMet/Livneh climate data. The distance measurements show each component used to calculate
TABLE 2. Response of food crop production to climate change (
Total production of potatoes, fruits, and vegetables declined due to a reduction in their share of crop area. Namely,
Corn is unique from the other food crops because it has a C4 photosynthetic pathway, which allows it to efficiently fix CO2 from the atmosphere. Free-air CO2 enrichment experiments have shown that C4 plants are less responsive to elevated CO2 than are C3 plants (Kimball et al., 2002). Meanwhile, warming temperatures shorten the crop cycle length (Hatfield et al., 2011). As a result, corn yields declined under both RCP 8.5 (
Climate change alone caused both bioenergy crop production and hydropower generation to increase. The hydropower response to climate had a distinct seasonality. Hydropower generation decreased June through November because of large withdrawals during the irrigation season (April through October) combined with declines in summer water supply due to warming-induced shift in streamflow timing. Earlier streamflow peaks led to greater winter streamflow and a marked increase in winter hydropower generation. The net effect of climate on an annual timescale was a small increase in generation (
TABLE 3. Response of hydropower generation and bioenergy crop production to climate change (
These results have important implications for energy development in the region. The Northwest Power and Conservation Council is already planning to integrate more renewable energy in the form of solar and wind power (NWPCC, 2022). Climate mitigation policy to incentivize bioenergy could shift the composition of the energy sector away from hydropower and could result in downsizing or shuttering of some hydroelectric facilities, especially if reduced flows make hydropower less reliable.
The results for bioenergy crop production are shown in Figure 8. The large
FIGURE 8. Annual production of bioenergy crops, represented by switchgrass in the extended CRB. Switchgrass production, both irrigated and dryland, was summed over all grid cells and the median calculated over the years 2046–2075 (future scenarios) or 1986–2015 (historical scenarios). The horizontal black line within (or above) each column marks the crop production value for that scenario using historical GridMet/Livneh climate data. The distance measurements show each component used to calculate
The effects of climate, irrigation, and land use on hydropower are shown in Figure 9. Hydropower generation largely reflected seasonal trends in streamflow. The CRB has a snowmelt-dominant streamflow regime, with peak streamflow occurring historically in May or June. However, climate change is expected to shift streamflow timing earlier in the season due to a smaller percentage of precipitation falling as snow (Barnett et al., 2005). This should create conditions of greater winter water availability and reduced summer water availability (Hall et al., 2021). In support of this hypothesis, we found that
FIGURE 9. Hydropower generation at major hydroelectric dams in the CRB compared to the firm energy load. Mean monthly generation was calculated over 1986–2015 (baseline scenarios) or 2046–2075 (future scenarios). The black horizontal line within (or above) each column represents the hydropower generation for that scenario run with historical GridMet/Livneh climate data.
Expansion of water rights had greater influence on hydropower generation than climate, with annual
The overall changes in climate, irrigation expansion, and land use resulted in greater irrigation demands and flow deficits (Table 4). Climate change caused greater irrigation demand in most months but caused significant declines in July (Figure 10). Meanwhile, climate change led to smaller instream flow deficits April through June and greater deficits July through October (see Figure 11). The seasonal sensitivity of instream flow to climate change implies heightened competition between irrigation and fish survival in the months of July through September, even absent any increase in irrigation. The hypothesis that climate pressures on instream flows will increase is supported by Markoff and Cullen (2008), who similarly found that targets for instream flows along the Columbia River were missed more frequently under all future climate scenarios. Their results and ours suggest climate change will exacerbate the unintended consequences of expanding irrigation. Such consequences include substantial damage to the fishing industry and further strain on the survival of salmon: a centerpiece of Native American diet and custom. At present, several of these tribes have treaty rights to fish at “usual and accustomed places” (Bernholz and Weiner, 2008). Expanding irrigated agriculture in basins where flows are near critical levels would increase the risk of breaking treaty obligations.
TABLE 4. Response of irrigation demand and instream flow deficit to climate change (
FIGURE 10. Total monthly irrigation demand for the extended CRB. The water demand includes groundwater and surface water irrigation (excluding conveyance losses). Median demand was calculated over 1986–2015 (baseline scenarios) or 2046–2075 (future scenarios). The black horizontal line within (or above) each column represents irrigation demand for that scenario run with historical GridMet/Livneh climate data.
FIGURE 11. Columbia River regulated water supply (A) and instream flow deficit (B) measured at The Dalles, Oregon. The mean monthly deficit over 1986–2015 (baseline scenarios) or 2046–2075 (future scenarios) includes years with zero deficit in that month. The black horizontal line within (or above) each column represents deficit for that scenario run with historical GridMet climate data. Panel (A) shows the instream flow (ISF) rule along with regulated water supply (no irrigation) from The Dalles reservoir corresponding to historical GridMet climate and future RCP 8.5/4.5 climate.
The
Following harvest of most annual crops, irrigation requirements were driven by perennial crops like tree fruits and by crops with multiple cuttings, like alfalfa and switchgrass. Crops with multiple cuttings had a greater annual irrigation requirement under future climate conditions because biomass accumulated more quickly following each cut, and there were more cuts on average. This resulted in greater
The
Instream flow deficit for the Columbia River mainstem was heavily influenced by both climate change and irrigation expansion. Climate influenced crop water-use patterns and streamflow timing, leading to distinct seasonality in the instream flow response (Figure 11B). The role played by water supply in flow deficit is shown in Figure 11A by plotting regulated flow without irrigation, i.e., water supply under the influence of dams before any withdrawals have been made. The water supply for future climate scenarios was greater during the winter months and smaller during the summer months due to the smaller snowmelt contribution to total runoff (Figure 11A). As previously noted, climate change generally caused irrigation demands to increase in months other than July. The combination of lower summer supply and higher irrigation demands late in the summer contributed to large July and August flow deficits. On the annual timescale, climate contributed to increases in the flow deficit by 25% to 49% under RCP 8.5 and by 14% to 37% under RCP 4.5. The flow deficits were intensified by irrigation expansion, with
Similar studies that address the impact of bioenergy cropland expansion on water availability have likewise found that scenarios with a bioenergy-based pathway for climate mitigation exhibit greater water stress. For instance, Cheng et al. (2022) calculated a 2.7% decrease in annual runoff for the Pacific Northwest region under the SSP2-RCP 4.5 scenario. We observed an increase in flow deficit by 9% under the comparable RCP 4.5 no expansion scenario. Their study, and ours more directly, suggest bioenergy expansion could compromise water security in the CRB.
Here we highlight a couple key limitations in our analysis. The FEW metrics did not account for the influence of water right curtailment. The principal effect of curtailment on FEW metrics would be to reduce crop yields and to augment streamflow, resulting in smaller flow deficits and greater hydropower generation because a portion of irrigated cropland served by a junior water right would not receive water during shortages. While the difference in results would be minimal for the no-expansion scenarios, the differences could be quite large for the moderate- and maximum-expansion scenarios, since both involve increases in cropland with an interruptible water right. Curtailment would therefore dampen the effect of expanding irrigated acreage on consumptive water use. Curtailment has important implications for FEW subsystems because it can result in yield and revenue loss for farmers with a junior water right.
The modelling framework used in this study is not currently capable of simulating competition between bioenergy and other fuels in local energy markets, which competition will have a major impact on the land planted to biofuel crops. While the current framework relies upon the price-clearing model of GCAM to simulate markets on a global scale, the profitability of growing bioenergy crops will also be influenced by demand for alternative heating and transportation fuels among residents of the CRB. Therefore, a rigorous analysis of the costs and benefits of allocating water for irrigating bioenergy crops and hydropower generation would require simulation of prices with the aid of a regional economic model. Moreover, additional analysis is needed to translate depletion of streamflow into fish survivability metrics before tradeoffs between water for irrigation and water for fish can be communicated in practical terms.
In this paper, we assessed impacts of global change on regional FEW sector outcomes in a water-limited basin using a multi-model approach with spatial downscaling. Explicit representation of water rights in the downscaling module allowed for investigating the role of water right POU in moderating the impact of LULCC on water availability for food crops, bioenergy, hydropower, and instream flow.
In the case study, we found the net impact of climate change in all the scenarios to be greater yield for crops other than corn, greater irrigation demand for months other than July, larger summer flow deficits, smaller spring flow deficits, less June through November hydropower generation, and greater winter hydropower generation. While it had a large effect on total crop production, land-use change alone had very little impact on either irrigation demand or Columbia River instream flow deficit compared to climate change and irrigation expansion.
The case study showed a tradeoff between expanding irrigated extent to boost crop yields on the one hand and maintaining enough streamflow to support hydropower and instream flow needs on the other. Even when expansion was constrained to the extent of existing water right POUs (moderate expansion), the increased irrigated area, combined with climate change, led to a doubling of bioenergy crop production concurrent with ∼2% reduction in hydropower generation and ∼50% increase in instream flow deficits by the 2060’s. When all irrigable land was given access to a water right (maximum expansion), bioenergy crop production increased by 183% under RCP 8.5 and 256% under RCP 4.5, but this was achieved at the cost of reducing hydropower generation by 6% under RCP 8.5 and 8% under RCP 4.5 and increasing instream flow deficits by 134% under RCP 8.5 and 165% under RCP 4.5. These tradeoffs have important implications for the FEW nexus of the CRB.
Spatial downscaling forms a crucial bridge between IAMs and models used to study regional FEW sectors. Our case study highlighted competition among multiple uses of water, under the pressures of global human and environmental change. In basins with seasonal water scarcity, like the CRB, water law balances the requirements of instream and out-of-stream uses. It does so, in part, by controlling how much land can be irrigated. Bioenergy crops are expected to increase in many LULCC scenarios to meet rising demands for second-generation biofuels. Our results indicate bioenergy production would be greatly enhanced by expanding access to water rights, but this benefit would come at considerable cost to fish and hydropower. Basins worldwide are likely to face similar challenges to co-managing resources in the coming century. A fuller integration of water rights into FEW subsystem analysis would give greater insight into the role of water regulation in shaping each of the sectors and may help evaluate impacts of water policy on FEW security.
The raw data supporting the conclusions of this article will be made available by the authors, without undue reservation.
MY: First author responsible for writing the manuscript, creating figures and tables. Also responsible for refinements to Demeter downscaling code, RColSim model runs, VIC-CropSyst model runs, and FEW metric analyses. ML: VIC-CropSyst model calibration and preparation of model inputs. Major contributions to manuscript editing. FS: VIC-CropSyst calibration and minor manuscript editing. KR: Major contributions to manuscript editing. KM: Contributed to RColSim model development and minor manuscript editing. JB: Manuscript editing MH: Coordinated sharing of GCAM data between Pacific Northwest National Laboratory and Washington State University. MC: Contributed to development of original Demeter code and responsible for all GCAM model simulations, minor manuscript editing. JA: Contributed to direction and focus of the manuscript. Major contributions to manuscript editing.
This research was funded by the National Science Foundation (NSF) under Track 1 of the Innovations at the Nexus of Food, Energy, and Water Systems project (INFEWS): grant number 1639458 (Increasing regional to global-scale resilience in Food-Energy-Water systems through coordinated management, technology, and institutions).
We would like acknowledge our collaborators at the Joint Change Research Institute of Pacific Northwest National Laboratory in College Park, MD. They conducted all the GCAM model runs and shared all the land-use/cover change outputs. They also developed the Demeter downscaling platform.
KM is employed by Nutrien Ag Solutions, Inc.
The remaining authors declare that the research was conducted in the absence of any commercial or financial relationships that could be construed as a potential conflict of interest.
All claims expressed in this article are solely those of the authors and do not necessarily represent those of their affiliated organizations, or those of the publisher, the editors and the reviewers. Any product that may be evaluated in this article, or claim that may be made by its manufacturer, is not guaranteed or endorsed by the publisher.
The Supplementary Material for this article can be found online at: https://www.frontiersin.org/articles/10.3389/fenvs.2023.1055771/full#supplementary-material
Abatzoglou, J. T., and Brown, T. J. (2012). A comparison of statistical downscaling methods suited for wildfire application. Int. J. Climatol. 32 (5), 772–780. doi:10.1002/joc.2312
Abatzoglou, J. T. (2013). Development of gridded surface meteorological data for ecological applications and modelling. Int. J. Climatol. 33, 121–131. doi:10.1002/joc.3413
Adam, J. C., Yourek, M. A., Scarpare, F. V., Liu, M., McLarty, S., Asante-Sasu, C., et al. (2022). Technical supplement for the 2021 Columbia River Basin long-term supply and demand forecast: Publication No. 22-12-001. Olympia, WA: Washington Department of Ecology, 200.
Agriculture and Agri-Food Canada (2016). Annual crop inventory. Available at: https://open.canada.ca/data/en/dataset/ba2645d5-4458-414d-b196-6303ac06c1c9 (Accessed July 28, 2022).
Albrecht, T. R., Crootof, A., and Scott, C. A. (2018). The water-energy-food nexus: A systematic review of methods for nexus assessment. Environ. Res. Lett. 13, 043002. doi:10.1088/1748-9326/aaa9c6
Allen, R. G., Pereira, L. S., Raes, D., and Smith, M. (1998). Crop evapotranspiration (guidelines for computing crop water requirements: Food and agriculture organization of the united nations, irrigation and drainage paper No. 56. Rome, Italy: FAO, 300.
Barnett, T. P., Adam, J. C., and Lettenmaier, D. P. (2005). Potential impacts of a warming climate on water availability in snow-dominated regions. Nature 438, 303–309. doi:10.1038/nature04141
Behnke, R., Vavrus, S., Allstadt, A., Albright, T., Thogmartin, W. E., and Radeloff, V. C. (2016). Evaluation of downscaled, gridded climate data for the conterminous United States. Ecol. Appl. 26 (5), 1338–1351. doi:10.1002/15-1061
Benson, R. (1998). Maintaining the status quo: Protecting established water uses in the Pacific Northwest, despite the rules of prior appropriation. Environ. Law 28 (4), 881–918.
Bernholz, C. D., and Weiner, R. J. (2008). The Palmer and Stevens “usual and accustomed places” treaties in the opinions of the courts. Gov. Inf. Quaterly 25 (4), 778–795. doi:10.1016/j.giq.2007.10.004
Bizikova, L., Roy, D., Swanson, D., Venema, H. D., and McCandless, M. (2013). The water-energy-food security nexus: Towards a practical planning and decision-support framework for landscape investment and risk management. Winnipeg, Manitoba, Canada: International Institute for Sustainable Development, 24.
BPA (2014). The Bonneville Power Administration NRNI dataset (1929-2008). Available at: https://www.bpa.gov/p/Power-Products/Historical-Streamflow-Data/Pages/No-Regulation-No-Irrigation-Data.aspx (Accessed July 28, 2022).
BPA (2001). The Columbia River system inside story. 2nd Edition. Portland, OR: [publisher not identified].
Brenkert, A. L., Kim, S. H., Smith, A. J., and Pitcher, H. M. (2003). Model documentation for the MiniCAM: Battelle Memorial Institute publication number PNNL-14337. College Park, MD: Joint Global Change Research Institute, 119.
Brown, J. F., and Pervez, M. S. (2014). Merging remote sensing data and national agricultural statistics to model change in irrigated agriculture. Agric. Syst. 127, 28–40. doi:10.1016/j.agsy.2014.01.004
Bureau of Reclamation (2016). “Columbia River Basin,” in Secure Water Act section 9503(c) report to Congress (Denver, Colorado: Bureau of Reclamation, Policy and Administration), 307.
Bureau of Reclamation (2013). “Record of decision for the odessa subarea special study final environmental impact statement,” in Reclamation: Managing water in the west (Boise, ID: Bureau of Reclamation, Pacific Northwest Region), 25.
Calvin, K., Patel, P., Clarke, L., Asrar, G., Bond-Lamberty, B., Cui, R. Y., et al. (2019). GCAM v5.1: Representing the linkages between energy, water, land, climate, and economic systems. Geosci. Model. Dev. 12, 677–698. doi:10.5194/gmd-12-677-2019
Calvin, K., Wise, M., Kyle, P., Patel, P., Clarke, L., and Edmonds, J. (2014). Trade-offs of different land and bioenergy policies on the path to achieving climate targets. Clim. Change 123, 691–704. doi:10.1007/s10584-013-0897-y
Chakir, R. (2009). Spatial downscaling of agricultural land-use data: An econometric approach using cross entropy. Land Econ. 85 (2), 238–251. doi:10.3368/le.85.2.238
Chen, M., Vernon, C. R., Graham, N. T., Hejazi, M., Huang, M., Cheng, Y., et al. (2020). Global land use for 2015-2100 at 0.05° resolution under diverse socioeconomic and climate scenarios. Nat. Sci. Data 7, 320. doi:10.1038/s41597-020-00669-x
Chen, M., Vernon, C. R., Huang, M., Calvin, K., and Kraucunas, I. P. (2019b). Calibration and analysis of the uncertainty in downscaling global land use and land cover projections from GCAM using Demeter (v.1.0.0). Geosci. Model. Dev. 12, 1753–1764. doi:10.5194/gmd-12-1753-2019
Chen, Y., Liu, A., Zhang, Z., Hope, C., and Crabbe, J. (2019a). Economic losses of carbon emissions from circum-Arctic permafrost regions under RCP-SSP scenarios. Sci. Total Environ. 658, 1064–1068. doi:10.1016/j.scitotenv.2018.12.299
Cheng, Y., Huang, M., Lawrence, D. M., Calvin, K., Lombardozzi, D. L., Sinha, E., et al. (2022). Future bioenergy expansion could alter carbon sequestration potential and exacerbate water stress in the United States. Sci. Adv. 8, eabm8237. doi:10.1126/sciadv.abm8237
Christensen, N., and Lettenmaier, D. P. (2007). A multi-model ensemble approach to assessment of climate change impacts on the hydrology and water resources of the Colorado River Basin. Hydrol. Earth Syst. Sci. 11, 1417–1434. doi:10.5194/hess-11-1417-2007
Daher, B. T., and Mohtar, R. H. (2015). Water-energy-food (WEF) Nexus Tool 2.0: Guiding integrative resource planning and decision-making. Water Int. 40, 748–771. doi:10.1080/02508060.2015.1074148
Dargin, J., Daher, B., and Mohtar, R. H. (2019). Complexity versus simplicity in water energy food nexus (WEF) assessment tools. Sci. Total Environ. 650, 1566–1575. doi:10.1016/j.scitotenv.2018.09.080
DeFries, R., and Eshleman, K. N. (2004). Land-use change and hydrologic processes: A major focus for the future. Hydrol. Process. 18, 2183–2186. doi:10.1002/hyp.5584
Dieter, C. A., Maupin, M. A., Caldwell, R. R., Harris, M. A., Ivahnenko, T. I., Lovelace, J. K., et al. (2018). Estimated use of water in the United States 2015: U.S. Geological Survey circular 1441. Reston, Virginia: U.S. Geological Survey, 65. [Supersedes USGS Open-File Report 2017-1131.]. doi:10.3133/cir1441
Ecology (2018). Washington Department of Ecology, Geographic Water Information System. Available at: https://fortress.wa.gov/ecy/gispublic/DataDownload/wr/GWIS_Data (Accessed July 28, 2022).
Ecology (2021). Washington Department of Ecology, Water Rights Tracking System. Available at: https://appswr.ecology.wa.gov/waterrighttrackingsystem (Accessed July 28, 2022).
EIA (2020). State energy production estimates 1960 through 2020. Washington, DC: U.S. Energy Information Administration, 140.
Fish Passage Center (2009). Fish Passage Center 2008 annual report. Portland, OR: Fish Passage Center of the Columbia Basin Fish & Wildlife Authority, 740.
Fisher-Vanden, K., and Weyant, J. (2020). The evolution of integrated assessment: Developing the next generation of use-inspired integrated assessment tools. Annu. Rev. Resour. Econ. 12, 471–487. doi:10.1146/annurev-resource-110119-030314
Fricko, O., Havlik, P., Rogelj, J., Klimont, Z., Gusti, M., Johnson, N., et al. (2017). The marker quantification of the Shared Socioeconomic Pathway 2: A middle-of-the-road scenario for the 21st century. Glob. Environ. Change 42, 251–267. doi:10.1016/j.gloenvcha.2016.06.004
Fu, X., Wang, X., and Yang, Y. J. (2018). Deriving suitability factors for CA-Markov land use simulation model based on local historical data. J. Environ. Manag. 206, 10–19. doi:10.1016/j.jenvman.2017.10.012
Geller, L. (2014). Setting instream flows in Washington State. Lacey, Washington: Washington Department of Ecology, Publication Number 98-1813-WR, 4.
Ghadikolaei, M. B., Vaghefi, N., and Sulaiman, W. N. A. (2012). Land use suitability analysis using multi criteria decision analysis method for coastal management and planning: A case study of Malaysia. J. Environ. Sci. Technol. 5 (5), 364–372. doi:10.3923/jest.2012.364.372
Graham, N. T., Hejazi, M. I., Chen, M., Davies, E. G. R., Edmonds, J. A., Kim, S. H., et al. (2020). Humans drive future water scarcity changes across all Shared Socioeconomic Pathways. Environ. Res. Lett. 15, 014007. doi:10.1088/1748-9326/ab639b
Hall, S. A., Adam, J. C., Yourek, M. A., Whittemore, A. M., Yorgey, G. G., Scarpare, F. V., et al. (2021). 2021 Columbia River Basin long-term water supply and demand forecast: Publication No. 21-12-006. Olympia, WA: Washington Department of Ecology, 263.
Hamlet, A. F., Elsner, M. M., Mauger, G. S., Lee, S., Tohver, I., and Norheim, R. A. (2013). An overview of the Columbia Basin Climate Change Scenarios Project: Approach, methods, and summary of key results. Atmosphere-Ocean 51 (4), 392–415. doi:10.1080/07055900.2013.819555
Hamlet, A. F., Lee, S., Mickelson, K. E. B., and Elsner, M. M. (2010). Effects of projected climate change on energy supply and demand in the Pacific Northwest and Washington State. Clim. Change 102, 103–128. doi:10.1007/s10584-010-9857-y
Hamlet, A. F., and Lettenmaier, D. P. (1999). Effects of climate change on hydrology and water resources in the Columbia River Basin. JAWRA 35 (6), 1597–1623. doi:10.1111/j.1752-1688.1999.tb04240.x
Harremoes, P., and Turner, R. K. (2001). Methods for integrated assessment. Reg. Environ. Change 2, 57–65. doi:10.1007/s101130100027
Hatfield, J. L., Boote, K. J., Kimball, B. A., Ziska, L. H., Izaurralde, R. C., Ort, D. R., et al. (2011). Climate impacts on agriculture: Implications for crop production. Agron. J. 103 (2), 351–370. doi:10.2134/agronj2010.0303
Hellman, F., and Verburg, P. H. (2011). Spatially explicit modelling of biofuel crops in Europe. Biomass Bioenergy 35, 2411–2424. doi:10.1016/j.biombioe.2008.09.003
Hibbard, K., and Janetos, A. C. (2013). The regional nature of global challenges: A need and strategy for integrated regional modeling. Clim. Change 118, 565–577. doi:10.1007/s10584-012-0674-3
Hills, K., Pruett, M., Rajagopalan, K., Adam, J. C., Liu, M., Nelson, R., et al. (2020). Calculation of 2020 irrigation depletions for 2020 level modified streamflows. Pullman, WA: Washington State University, Water Research Center, 279.
Hoff, H. (2011). Understanding the nexus in Background paper for the Bonn 2011 Conference: The Water, Energy, and Food Security Nexus, Bonn, Germany, 16-18 November 2011 (Stockholm, Sweden: Stockholm Environment Institute), 51.
Howells, M., Hermann, S., Welsch, M., Bazilian, M., Segerström, R., Alfstad, T., et al. (2013). Integrated analysis of climate change, land-use, energy, and water strategies. Nat. Clim. Change 3, 621–626. doi:10.1038/nclimate1789
IDWR (2018). Idaho Department of Water Resources, maps and GIS data hub. Available at: https://data-idwr.opendata.arcgis.com/pages/gis-data#WaterRights (Accessed July 28, 2022).
IPCC (2007). Climate change 2007: The physical science basis. Contributions of working group 1 to the fourth assessment report of the Intergovernmental Panel on Climate Change. Cambridge, UK, and New York, NY: Cambridge University Press.
Ketchum, D., Jencso, K., Maneta, M. P., Melton, F., Jones, M. O., and Huntington, J. (2020). IrrMapper: A machine learning approach for high resolution mapping of irrigated agriculture across the Western U.S. Remote Sens. 20 (14), 2328. doi:10.3390/rs12142328
Khan, Z., Wild, T. B., Carrazzone, M., Gaudioso, R., Mascari, M. P., Bianchi, F., et al. (2020). Integrated energy-water-land nexus planning to guide national policy: An example from Uruguay. Environ. Res. Lett. 15, 094014. doi:10.1088/1748-9326/ab9389
Kimball, B. A., Kobayashi, K., and Bindi, M. (2002). Responses of agricultural crops to free-air CO2 enrichment. Adv. Agron. 77, 293–368. doi:10.1016/S0065-2113(02)77017-X
Kimura, E., Collins, H., and Fransen, S. (2015). Biomass production and nutrient removal by switchgrass under irrigation. Agron. J. 107, 204–210. doi:10.2134/agronj14.0259
Kirschbaum, R. L., and Lettenmaier, D. P. (1997). An evaluation of the effects of anthropogenic activity on streamflow in the Columbia River Basin: Technical report No. 156. Seattle, WA: Department of Civil Engineering, University of Washington, 131.
Kraucunas, I., Clarke, L., Dirks, J., Hathaway, J., Hejazi, M., Hibbard, K., et al. (2015). Investigating the nexus of climate, energy, water, and land at decision-relevant scales: The platform for regional integrated modeling and analysis (PRIMA). Clim. Change 129, 573–588. doi:10.1007/s10584-014-1064-9
Kriegler, E., Bauer, N., Popp, A., Humpenöder, F., Leimbach, M., Strefler, J., et al. (2017). Fossil-fueled development (SSP5): An energy and resource intensive scenario for the 21st century. Glob. Environ. Change 42, 297–315. doi:10.1016/j.gloenvcha.2016.05.015
Le Page, Y., Tris, W., Link, R., and Patel, P. (2016). Downscaling land use and land cover from the Global Change Assessment Model for coupling with Earth system models. Geosci. Model. Dev. 9, 3055–3069. doi:10.5194/gmd-9-3055-2016
Leck, H., Conway, D., Bradshaw, M., and Rees, J. (2015). Tracing the water-energy-food nexus: Description, theory, and practice. Geogr. Compass 9/8, 445–460. doi:10.1111/gec3.12222
Leung, R. L., and Ghan, S. J. (1998). Parameterizing subgrid orographic precipitation and surface cover in climate models. Am. Meteorological Soc. Mon. Weather Rev. 126, 3271–3291. doi:10.1175/1520-0493(1998)126<3271:PSOPAS>2.0.CO;2
Li, X., Chen, G., Liu, X., Liang, X., Wang, S., Chen, Y., et al. (2017). A new global land-use and land-cover change product at a 1-km resolution for 2010 to 2100 based on human-environment interactions. Ann. Am. Assoc. Geogr. 107 (5), 1040–1059. doi:10.1080/24694452.2017.1303357
Liang, X., Lettenmaier, D. P., Wood, E. F., and Burges, S. J. (1994). A simple hydrologically based model of land surface water and energy fluxes for general circulation models. J. Geophys. Res. 99 (D7), 14415–14428. doi:10.1029/94JD00483
Lindholm, G. F., and Goodell, S. A. (1986). Irrigated acreage and other land uses on the Snake River Plain, Idaho and eastern Oregon. Reston, Virginia: U.S. Geological Survey. doi:10.3133/ha691
Liu, J., Yang, H., Cudennec, C., Gain, A. K., Hoff, H., Lawford, R., et al. (2017). Challenges in operationalizing the water-energy-food nexus. Hydrological Sci. J. 62 (11), 1714–1720. doi:10.1080/02626667.2017.1353695
Livneh, B., Rosenberg, E. A., Lin, C., Nijssen, B., Mishra, V., Andreadis, K., et al. (2013). A long-term hydrologically based dataset of land surface fluxes and states for the conterminous United States: Update and extensions. J. Clim. 26, 9384–9392. doi:10.1175/JCLI-D-12-00508.1
Lohmann, D., Nolte-Holube, R., and Raschke, E. (1996). A large-scale horizontal routing model to be coupled to land surface parameterization schemes. Tellus 48A, 708–721. doi:10.1034/j.1600-0870.1996.t01-3-00009.x
LWRS (2018). British Columbia Ministry of Land, Water, and Resource Stewardship, land parcels with water licences. Available at: https://catalogue.data.gov.bc.ca/dataset/land-parcels-with-water-licences (Accessed July 28, 2022).
Madakadze, I., Coulman, B., Peterson, P., Stewart, K. A., Samson, R., and Smith, D. L. (1998). Leaf area development, light interception, and yield among switchgrass populations in a short-season area. Crop Sci. 38, 827–834. doi:10.2135/cropsci1998.0011183X003800030035x
Mahler, R. L. (2019). The impact of agriculture on the waters of the Idaho portion of the Snake River Basin, USA. Int. J. Sus. Dev. Plann. 14 (2), 93–104. doi:10.2495/SDP-V14-N2-93-104
Malek, K., Adam, J., Yoder, J., Givens, J., Stöckle, C., Brady, M., et al. (2021). Impacts of irrigation efficiency on water-dependent sectors are heavily controlled by region-specific institutions and infrastructures. J. Environ. Manag. 300, 113731. doi:10.1016/j.jenvman.2021.113731
Malek, K., Reed, P., Adam, J., Karimi, T., and Brady, M. (2020). Water rights shape crop yield and revenue volatility tradeoffs for adaptation in snow dependent systems. Nat. Commun. 11, 3473. doi:10.1038/s41467-020-17219-z
Malek, K., Stöckle, C., Chinnayakanahalli, K., Nelson, R., Liu, M., Rajagopalan, K., et al. (2017). VIC–CropSyst-v2: A regional-scale modeling platform to simulate the nexus of climate, hydrology, cropping systems, and human decisions. Geosci. Model. Dev. 10, 3059–3084. doi:10.5194/gmd-10-3059-2017
Malek, K., Yourek, M., Adam, J., Hamlet, A., Rajagopalan, K., and Reed, P. (2022). RColSim: A regional water management model to simulate the food-energy-water nexus in the Columbia River Basin. J. Open Res. Softw. (in review).
Mantua, N., Tohver, I., and Hamlet, A. (2010). Climate change impacts on streamflow extremes and summertime stream temperature and their possible consequences for freshwater salmon habitat in Washington State. Clim. Change 102, 187–223. doi:10.1007/s10584-010-9845-2
Markoff, M. S., and Cullen, A. C. (2008). Impact of climate change on Pacific Northwest hydropower. Clim. Change 87, 451–469. doi:10.1007/s10584-007-9306-8
Maurer, E. P. (2007). Uncertainty in hydrologic impacts of climate change in the Sierra Nevada, California under two emissions scenarios. Clim. Change 82 (3-4), 309–325. doi:10.1007/s10584-006-9180-9
McLaughlin, S. B., and Kszos, L. A. (2005). Development of switchgrass (Panicum virgatum) as a bioenergy feedstock in the United States. Biomass Bioenergy 28, 515–535. doi:10.1016/j.biombioe.2004.05.006
Mitchell, K. E., Lohmann, D., Houser, P. R., Wood, E. F., Schaake, J. C., Robock, A., et al. (2004). The multi-institution North American Land Data Assimilation System (NLDAS): Utilizing multiple GCIP products and partners in a continental distributed hydrological modeling system. J. Geophys. Res. 109, D07S90. doi:10.1029/2003JD003823
Montana DNRC (2018). Montana Department of Natural Resources and Conservation, Montana water rights. Available at: https://mslservices.mt.gov/Geographic_Information/Data/DataList/datalist_Details.aspx?did={0303D17E-BD0F-4180-A345-359C61E586F0} (Accessed July 28, 2022).
Moore, N., Alagarswamy, G., Pijanowski, B., Thornton, P., Lofgren, B., Olson, J., et al. (2012). East African food security as influenced by future climate change and land-use change at local to regional scales. Clim. Change 110, 823–844. doi:10.1007/s10584-011-0116-7
Moss, R. H., Edmonds, J. A., Hibbard, K. A., Manning, M. R., Rose, S. K., van Vuuren, D. P., et al. (2010). The next generation of scenarios for climate change research and assessment. Nature 463, 747–756. doi:10.1038/nature08823
NMFS (2020). Endangered Species Act (ESA) section 7(a)(2) biological opinion and Magnuson-Stevens Fishery Conservation and Management Act essential fish habitat response: NMFS consultation number: WCRO-2020-00113. Portland, OR: National Oceanic and Atmospheric Administration, National Marine Fisheries Service, 1496.
NWPCC (2022). The 2021 Northwest Power Plan. Portland, Oregon: Northwest Power and Conservation Council, 139.
O’Neill, B. C., Kriegler, E., Ebi, K. L., Kemp-Benedict, E., Riahi, K., Rothman, D. S., et al. (2017). The roads ahead: Narratives for Shared Socioeconomic Pathways describing world futures in the 21st century. Glob. Environ. Change 42, 169–180. doi:10.1016/j.gloenvcha.2015.01.004
O’Neill, B. C., Kriegler, E., Riahi, K., Ebi, K. L., Hallegatte, S., Carter, T. R., et al. (2014). A new scenario framework for climate change research: The concept of Shared Socioeconomic Pathways. Clim. Change 122, 387–400. doi:10.1007/s10584-013-0905-2
OWRD (2018). Oregon Water Resources Department, statewide water right spatial data. Available at: https://www.oregon.gov/owrd/access_data/pages/data.aspx (Accessed July 28, 2022).
Peery, C. (2012). “The effects of dams and flow management on Columbia River ecosystem processes,” in The Columbia River Treaty revisited. Editor B. Cosens (Corvallis, OR: Oregon State University Press).
Popp, A., Rose, S. K., Calvin, K., van Vuuren, D. P., Dietrich, J. P., Wise, M., et al. (2014). Land-use transition for bioenergy and climate stabilization: Model comparison of drivers, impacts, and interactions with other land-use based mitigation options. Clim. Change 123, 495–509. doi:10.1007/s10584-013-0926-x
Rajagopalan, K., Chinnayakanahalli, K. J., Stöckle, C. O., Nelson, R. L., Kruger, C. E., Brady, M. P., et al. (2018). Impacts of near-term climate change on irrigation demands and crop yields in the Columbia River Basin. Water Resour. Res. 54, 2152–2182. doi:10.1002/2017WR020954
Rasul, G., and Sharma, B. (2016). The nexus approach to water-energy-food security: An option for adaptation to climate change. Clim. Policy 16 (6), 682–702. doi:10.1080/14693062.2015.1029865
Reed, P. M., Hadjimichael, A., Moss, R. H., Brelsford, C., Burleyson, C. D., Cohen, S., et al. (2022). Multisector dynamics: Advancing the science of complex adaptive human-Earth systems. Earth’s Future 10, e2021EF002621. doi:10.1029/2021EF002621
Riahi, K., Rao, S., Krey, V., Cho, C., Chirkov, V., Fischer, G., et al. (2011). RCP 8.5 – a scenario of comparatively high greenhouse gas emissions. Clim. Change 109, 33–57. doi:10.1007/s10584-011-0149-y
Riahi, K., van Vuuren, D. P., Kriegler, E., Edmonds, J., O’Neill, B. C., Fujimori, S., et al. (2017). The Shared Socioeconomic Pathways and their energy, land use, and greenhouse gas emissions implications: An overview. Glob. Environ. Change 42, 153–168. doi:10.1016/j.gloenvcha.2016.05.009
Rickebusch, S., Metzger, M. J., Xu, G., Vogiatzakis, I. N., Potts, S. G., Stirpe, M. T., et al. (2011). A qualitative method for the spatial and thematic downscaling of land-use change scenarios. Environ. Sci. Policy 14, 268–278. doi:10.1016/j.envsci.2010.11.003
Sakieh, Y., Salmanmahiny, A., Jafarnezhad, J., Mehri, A., Kamyab, H., and Galdavi, S. (2015). Evaluating the strategy of decentralized urban land-use planning in a developing region. Land Use Policy 48, 534–551. doi:10.1016/j.landusepol.2015.07.004
Scarpare, F. V., Rajagopalan, K., Liu, M., Nelson, R. L., and Stöckle, C. O. (2022). Evaporation of irrigated crops under warming and elevated atmospheric CO2: What is the direction of change? Atmosphere 13, 163. doi:10.3390/atmos13020163
Schilling, K. (2018). Addressing the prior appropriation doctrine in the shadow of climate change and the Paris Climate Agreement. Seattle J. Environ. Law 8, 4.
Schull, V. Z., Daher, B., Gitau, M. W., Mehan, S., and Flanagan, D. C. (2020). Analyzing FEW nexus modeling tools for water resources decision-making and management applications. Food Bioprod. Process. 119, 108–124. doi:10.1016/j.fbp.2019.10.011
Sessions, C. (2017). An introduction to instream flows and instream flow rules. Lacey, Washington: Washington Department of Ecology, Publication Number 17-11-002, 2.
Shi, X., Matsui, T., Haga, C., Machimura, T., Hashimoto, S., and Saito, O. (2021). A scenario- and spatial-downscaling-based land-use modeling framework to improve the projections of plausible futures: A case study of the Guangdong–Hong Kong–Macao greater bay area, China. Sustain. Sci. 16, 1977–1998. doi:10.1007/s11625-021-01011-z
Snover, A. K., Hamlet, A. F., and Lettenmaier, D. P. (2003). Climate-change scenarios for water planning studies: Pilot applications in the Pacific Northwest. Bull. Am. Meteorological Soc. 84 (11), 1513–1518. doi:10.1175/BAMS-84-11-1153
Sohl, T. L., Sleeter, B. M., Zhu, Z., Sayler, K. L., Bennett, S., Bouchard, M., et al. (2012). A land-use and land-cover modeling strategy to support a national assessment of carbon stocks and fluxes. Appl. Geogr. 34, 111–124. doi:10.1016/j.apgeog.2011.10.019
Soil Conservation Service (1961). Land-capability classification: Agricultural handbook No. 210. Washington, DC: U.S. Department of Agriculture, Soil Conservation Service, 21.
Soil Survey Staff(a) (2019). Natural Resources Conservation Service, United States Department of Agriculture, Soil Survey Geographic (SSURGO) database. Available at: https://sdmdataaccess.sc.egov.usda.gov (Accessed Oct. 17, 2019).
Soil Survey Staff(b) (2019). Natural Resources Conservation Service, United States Department of Agriculture, U.S. General Soil Map (STATSGO2). Available at: https://sdmdataaccess.sc.egov.usda.gov (Accessed Oct. 17, 2019).
Stöckle, C. O., Donatelli, M., and Nelson, R. (2003). CropSyst, a cropping systems simulation model. Eur. J. Agron. 18 (3-4), 289–307. doi:10.1016/S1161-0301(02)00109-0
Taylor, J. E. (1999). Making salmon: An environmental history of the northwest fisheries crisis. Seattle, WA: University of Washington Press, 488.
Thomson, A. M., Calvin, K. V., Smith, S. J., Kyle, P. G., Volke, A., Patel, P., et al. (2011). RCP4.5: A pathway for stabilization of radiative forcing by 2100. Clim. Change 109, 77–94. doi:10.1007/s10584-011-0151-4
USDA (2017). 2017 Census of Agriculture. Volume 1, chapter 2, table 1. Washington, DC: U.S. Department of Agriculture.
USDA-NASS (2016). 2016 Cropland Data Layer. Washington, DC: U.S. Department of Agriculture, National Agricultural Statistics Service. Available at: https://nassgeodata.gmu.edu/CropScape/(Accessed Oct 27, 2021).
van Vuuren, D. P., Edmonds, J., Kainuma, M., Riahi, K., Thomson, A., Hibbard, K., et al. (2011). The Representative Concentration Pathways: An overview. Clim. Change 109, 5–31. doi:10.1007/s10584-011-0148-z
van Vuuren, D. P., Kriegler, E., O’Neill, B. C., Ebi, K. L., Riahi, K., Carter, T. R., et al. (2014). A new scenario framework for climate change research: Scenario matrix architecture. Clim. Change 122, 373–386. doi:10.1007/s10584-013-0906-1
Vernon, C. R., Le Page, Y., Chen, M., Huang, M., Calvin, K. V., Kraucunas, P., et al. (2018). Demeter – a land use and land cover change disaggregation model. J. Open Res. Softw. 6, 15. doi:10.5334/jors.208
Voisin, N., Liu, L., Hejazi, M., Tesfa, R., Li, H., Huang, M., et al. (2013). One-way coupling of an integrated assessment model and a water resources model: Evaluation and implications of future changes over the US Midwest. Hydrol. Earth Syst. Sci. 17, 4555–4575. doi:10.5194/hess-17-4555-2013
Ward, N. E., and Ward, D. L. (2004). Resident fish in the Columbia River Basin: Restoration, enhancement, and mitigation for losses associated with hydroelectric development and operations. Fisheries 29 (3), 10–18. doi:10.1577/1548-8446(2004)29[10:RFITCR]2.0.CO;2
WEF Water Initiative (2011). Water security: The water-food-energy-climate nexus. Washington, DC: Island Press, 243.
Weitz, N. (2014). Cross-sectoral integration in sustainable development goals: A nexus approach. Stockholm, Sweden: Stockholm Environment Institute, discussion brief, 8.
West, T. O., Le Page, Y., Huang, M., Wolf, J., and Thomson, A. M. (2014). Downscaling global land cover projections from an integrated assessment model for use in regional analyses: Results and evaluation for the US from 2005 to 2095. Environ. Res. Lett. 9, 064004. doi:10.1088/1748-9326/9/6/064004
Wild, T. B., Khan, Z., Zhao, M., Suriano, M., Bereslawski, J. L., Roberts, P., et al. (2021). The implications of global change for the co-evolution of Argentina’s integrated energy-water-land systems. Earth’s Future 9, e2020EF001970. doi:10.1029/2020ef001970
Wise, M. A., Calvin, K. V., Thomson, A. M., Clarke, L. E., Bond-Lamberty, B., Sands, R. D., et al. (2009). The implications of limiting CO2 concentrations for agriculture, land use, land-use change emissions and bioenergy: Battelle Memorial Institute publication number PNNL-17943. College Park, MD: Joint Global Change Research Institute, 42.
WSDA (2016). Washington State Department of Agriculture, agricultural land use dataset. Available at: https://agr.wa.gov/departments/land-and-water/natural-resources/agricultural-land-use (Accessed Oct. 27, 2021).
Keywords: water rights, land-use change, bioenergy, multi-model framework, instream flow, hydropower, land-use downscaling
Citation: Yourek M, Liu M, Scarpare FV, Rajagopalan K, Malek K, Boll J, Huang M, Chen M and Adam JC (2023) Downscaling global land-use/cover change scenarios for regional analysis of food, energy, and water subsystems. Front. Environ. Sci. 11:1055771. doi: 10.3389/fenvs.2023.1055771
Received: 28 September 2022; Accepted: 16 January 2023;
Published: 30 January 2023.
Edited by:
Donald Wuebbles, University of Illinois at Urbana-Champaign, United StatesReviewed by:
Anne Stoner, Texas Tech University, United StatesCopyright © 2023 Yourek, Liu, Scarpare, Rajagopalan, Malek, Boll, Huang, Chen and Adam. This is an open-access article distributed under the terms of the Creative Commons Attribution License (CC BY). The use, distribution or reproduction in other forums is permitted, provided the original author(s) and the copyright owner(s) are credited and that the original publication in this journal is cited, in accordance with accepted academic practice. No use, distribution or reproduction is permitted which does not comply with these terms.
*Correspondence: Matt Yourek, bWF0dGhldy55b3VyZWtAd3N1LmVkdQ==
Disclaimer: All claims expressed in this article are solely those of the authors and do not necessarily represent those of their affiliated organizations, or those of the publisher, the editors and the reviewers. Any product that may be evaluated in this article or claim that may be made by its manufacturer is not guaranteed or endorsed by the publisher.
Research integrity at Frontiers
Learn more about the work of our research integrity team to safeguard the quality of each article we publish.