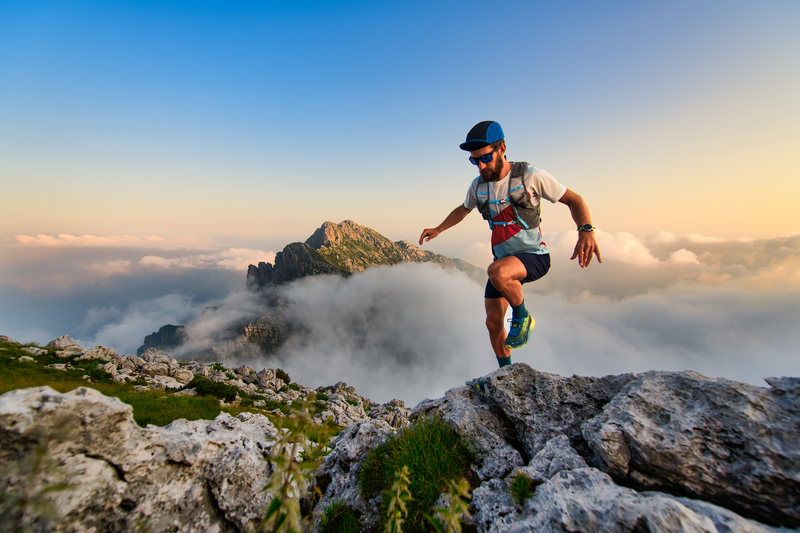
94% of researchers rate our articles as excellent or good
Learn more about the work of our research integrity team to safeguard the quality of each article we publish.
Find out more
ORIGINAL RESEARCH article
Front. Environ. Sci. , 19 August 2022
Sec. Environmental Economics and Management
Volume 10 - 2022 | https://doi.org/10.3389/fenvs.2022.993677
This article is part of the Research Topic Global Excellence: Environmental Issues and Global Value Chain in Asia View all 24 articles
In the context of the sharing economy, green consumption is becoming a trend. Shared accommodation represented by Airbnb has been extensively studied. Analysis of the emotional characteristics of green users is the key to promoting the sustainable development of Airbnb. The article obtains the online reviews of Airbnb green users, fully considers the deviation caused by the weight of feature words to the sentiment calculation, and combines the sentiment value with the weight value to obtain the comprehensive sentiment value of green users. Then, a spatial comparative analysis of green users’ emotional characteristics in Beijing, Shanghai and Hong Kong in China is carried out. The results show that in Airbnb, green users have a more obvious emotional tendency to human and geographical factors, followed by housing factors, and show lower tendency to environmental factors. The study provides a new perspective for optimizing the Airbnb scoring system and promoting the sustainable development of the shared accommodation market.
The boom in tourism has boosted the development of the travel accommodation market. In recent years, shared accommodation represented by Airbnb has been favored by travel consumers due to its shareability and affordability. After entering the Chinese market in 2015, Airbnb caused a boom in the shared accommodation market in China. Influenced by the traditional Chinese cultural concept of “home,” travelers are more inclined to choose Airbnb, which can provide them with personalized services and a sense of family experience (Chen Y. et al., 2020). However, due to factors such as the long transfer chain of resource use rights in domestic shared accommodation, the high value of shared housing and strong accommodation privacy, the barrier to trust among Airbnb entities is relatively high (Wang P. et al., 2019), resulting in the current situation of China’s shared accommodation market with great development potential but in short supply.
In the post-epidemic era, green consumption has gradually become a life attitude of consumers. Although some scholars have questioned the shareability of Airbnb (Demir and Emekli, 2021), domestic Airbnb hosts usually share the second and later houses they own. This reuse of underutilized assets proves that Airbnb is still a part of the sharing economy in China.
Some studies believe that participating in the sharing economy itself is a green consumption behavior (Curtis and Lehner, 2019), which remains to be discussed. Because greenness and sustainability are not the main motivation for users to participate in the sharing economy at this stage (Böcker and Meelen, 2017). Online reviews are the foundation of a trusting relationship between hosts and tenants. In the analysis of user experience through online reviews, researchers generally found that factors such as service, location, price and facilities influence user experience (Tussyadiah and Zach, 2017; Cheng and Jin, 2019; Luo and Tang, 2019; Kiatkawsin et al., 2020). However, most existing studies analyze Airbnb users as a whole, without considering users Type-induced differences in experience. For example, Lee et al. (2019) analyzed the online reviews of Airbnb users in London and explored the changes of Airbnb user experience over time spans and seasons. Kim et al. (2021) analyzed the online reviews of Airbnb users in multiple cities around the world and found that users’ purchase intentions are affected by the host’s response. The study by Serrano et al. (2021) indicates that green users are part of the Airbnb user group. In order to guide more travelers to green consumption, reduce the trust barriers among shared accommodation entities, and thus promote the development of the domestic shared accommodation market. It is necessary to study the emotional characteristics of green user groups in Airbnb.
When analyzing the emotional characteristics of users, researchers often find the emotional tendencies of users by calculating the sentiment value of reviews (Lawani et al., 2018; Yakubu et al., 2021). Some studies use supervised machine learning models such as deep learning to train labeled samples, so as to predict users’ emotional tendencies (Liu et al., 2021). These studies can accurately assess the emotional characteristics of individual consumers. However, when measuring the overall emotional characteristics of users, the weighting of subject terms that influence users’ emotional tendencies are often ignored. Luo and Tang (2019) proposed a modified latent aspect rating analysis (LARA), which suggests a way to measure weights. While the method does not consider the interaction effects between factors, which may bias the measurement results. To achieve an accurate analysis of user emotional characteristics, research must consider the interaction effect between factors. Analytic Network Process (ANP) model fully considers the interaction between factors, and provides a method for calculating weights (Saaty, 1996), which can effectively eliminate the influence of weight bias on the overall emotional tendency.
In addition, some scholars have already analyzed the current state of Airbnb in China, but mostly focused on exploring one city. For example, Sun et al. (2021) studied the influencing factors of Airbnb spatial distribution in Nanjing. Bao et al. (2022) used the text analysis method to discuss the user experience of Airbnb in Hangzhou, and found that Airbnb lacks entertainment. However, China has a huge territory and different consumption habits in different regions. At present, there is still a lack of comparative analysis of spatial characteristics of Airbnb customer experience in different provinces and cities.
The purpose of this study is to assess the emotional tendencies of green users during consumption by analyzing online reviews of green consumers in Airbnb. The specific objectives are to: 1) identify the topic factors that influence green users’ emotional tendencies by the Latent Dirichlet Allocation (LDA) model; 2) explore the interrelationships among the factors by multivariate statistical analysis; 3) determine the combined emotional values of green users and the role of environmental factors on green consumption; and 4) conduct a spatial comparison analysis of the emotional characteristics of green users in different cities. As China advocates the new development concept of green and sharing, this study explores the emotional tendencies of green consumers, which is in line with the trend of the times. Multiple regression analysis is used to verify the interaction effect between factors, which makes up for the neglect of the relationship between factors in previous studies. The study proposes to combine sentiment analysis with the DEMATEL-ANP method for the first time. This innovation improves the accuracy of sentiment feature measurement, and at the same time, through spatial feature analysis, it can provide a more detailed reference for optimization of the scoring system of the Airbnb platform, as well as an accurate reference for hosts when releasing property information. Therefore, the study of the emotional characteristics of green users in Airbnb needs to be further explored.
This paper selects Beijing, Shanghai and Hong Kong as representatives of cities in the northern, southern and special administrative regions of China, to obtain online consumer reviews from Airbnb users. Based on text sentiment analysis and the ANP model, this paper analyzes the emotional characteristics of green users in Airbnb under the influence of multi-factor interaction.
Green users refer to those who show pro-environmental and pro-social green consumption behavior in the consumption process (Hosta and Zabkar, 2021). With changes in social environment, green consumption has gradually shown a trend. The development of environmental technology can positively promote the green energy consumption (Gao et al., 2022). Users or organizations with green consumption behavior can usually show greater advantages in competition (Banytė et al., 2020). Möller and Herm (2021) investigated consumers’ views on green and non-green user entrepreneurs. The results show that consumers who identify with green user entrepreneurs have strong green values. Consumers with such green values will show more green consumption behavior in the process of consumption (Choi and Johnson, 2019).
The popularity of the Internet has distorted the way people interact with the environment, and promoted the transformation of individual attitudes and behaviors (Wang and Jeong, 2018). This transformation has spawned a large number of online green users under the guidance of digital technology. Chen B. et al. (2020) took Ant Forest as an example to explore the behavioral motivation of online green users through questionnaires, and found that environmental awareness and social motivation are positively promoting green user behavior. The rise of the Internet and digital technology has also contributed to the development of tourism. Hurley (2018) believes that green users tend to use words related to green consumption behavior during consumption. Both travelers’ consumption preferences and transportation environment-based factors have an impact on their green consumption behavior (Taube et al., 2018).
In other studies on the motivation of green users’ consumption behavior, it was found that external factors such as perceived responsibility and the environment promote green behavior by influencing the internal state of consumers (Wegmann and Jiao, 2017). Altruistic values, beliefs, and personal norms can effectively explain tourists’ pro-environmental behavior (Landon et al., 2018). In P2P accommodation, service composition complexity, network membership, reputation and innovation practice will have varying degrees of impact on green behavior (Ceptureanu et al., 2020). In addition, consumer knowledge, consumption object value, emotion, and rationality all have significant influence on green behavior prediction (Betzler et al., 2022).
The sharing economy, an economic model in which the public share the right to use an item without transferring ownership, has greatly alleviated the environmental problems caused by resource waste. By sharing cooperative consumption, social resources can be used more fully, from which consumers can obtain satisfaction with sustainable consumption behavior and even higher economic benefits (Hamari et al., 2016). In some clusters of the sharing economy, shared accommodation is the representative research object (Hossain, 2020; Sanasi et al., 2020). As a new type of accommodation model, Airbnb has an interdependent and competitive relationship with traditional hotels, factors such as the number and price of surrounding hotels will have a positive impact on the popularity of Airbnb (Yi et al., 2021). Meanwhile, Airbnb is a subversive innovation to traditional hotels (Yu et al., 2020). Its sharing nature is one of the focuses of public and media attention (Huertas et al., 2021). Research by Demir and Emekli (2021) shows that changes in business models have removed Airbnb from the sharing economy.
Airbnb’s direct stakeholders include platform companies, hosts, and renters. Airbnb platform connects hosts and renters, and in order to maintain stable development, it must respond accordingly to the market. To this end, von Richthofen and von Wangenheim (2021) propose strategies to promote the development of Airbnb through qualitative analysis, namely orientation, enablement, incentive and control. A study of Airbnb hosts found that economic motivation drives them to be more willing to share their listings (Canziani and Nemati, 2021). And by choosing Airbnb for their accommodation, consumers can avoid loneliness to some extent (Farmaki and Stergiou, 2019). Renters usually comment on their experience after consumption, and these review data can truly reflect the feelings and provide a great convenience for the research on consumer behavior (Ayar and Gürbüz, 2021).
The Internet drives the development of online consumption. Generally speaking, trust is the key to promoting online consumption growth. The Airbnb platform provides a large number of online reviews, providing reference information for the platform, hosts, and subsequent consumers, and is the key for marketers to formulate marketing strategies that meet the needs of tourists (Lalicic et al., 2021). Due to the huge amount of consumer review data, it is difficult for traditional econometric analysis to directly process text data. Therefore, the use of machine learning for text analysis of online reviews has attracted the attention of scholars (Li et al., 2019).
Extracting keywords that influence user experience from online reviews is a method for text processing. Common methods include factor analysis, Latent Semantic Analysis (LSA), etc. (Kim et al., 2021; Xu, 2021). The Latent Dirichlet Allocation (LDA) model is a relatively new and well-established tool, which is widely used by researchers. Ahani et al. (2021) used LDA to generate topics affecting the dimension of user satisfaction, on which the satisfaction of medical travelers was assessed by the expected maximum and neuro-fuzzy approach. Lucini et al. (2020) used LDA to identify 27 dimensions affecting airline customer experience, and then analyzed the impact of different dimensions on user satisfaction. Kiatkawsin et al. (2020) compared the review texts of Hong Kong and Singapore, and used LDA to classify the topics of users’ attention. It is found that there are differences in users’ attention between the two places. In the research on the impact of Airbnb user experience, Luo and Tang (2019) found five aspects that affect user experience through the analysis of online reviews: communication, experience, location, product, and price. Cheng and Jin (2019) analyzed the reviews of Sydney Airbnb users and found that users care about location, landlord attitude and accommodation facilities in the consumption process.
Calculating the sentiment value of reviews to observe users’ sentiment tendency is another research focus of text analysis. Lawani et al. (2018) used the sentiment dictionary to obtain the sentiment scores of reviews as a measure of user satisfaction, and explored the impact of price and distance on user satisfaction. Yakubu et al. (2021) used multi-gene genetic programming-based fuzzy regression (MGGPFR) to obtain the sentiment scores of reviews to analyze the sentiment tendencies of e-commerce users. It is worth noting that the emotional tendencies of a single user can be evaluated by sentiment values. When evaluating the overall user’s sentiment tendency, due to the different proportions of different feature words in the reviews, the interaction between different factors affecting the user experience may cause certain deviations to the results. Based on this, the following research hypothesis is proposed:
H1: Topic factors in online reviews have a significant impact on green users’ sentiment tendencies.
H2: There is an interaction effect between topic factors in online reviews, which comprehensively influences the sentiment tendencies of green users.
The data for this article comes from Inside Airbnb, which provides review information in many cities around the world. Taking Beijing as the main research object, 1376647 reviews were collected online. According to Hurley (2018), green sustainable lifestyles. Biswas and Roy (2015) found that the word “green” was often considered to sustainable in the consumption process. In some studies on sustainable consumption behavior, it is found that users who use “green,” “clean” and other words in reviews tend to make sustainable consumption behavior (Wang Y. et al., 2019; Serrano et al., 2021; Wang and Yu, 2021). To compile a list of search terms, the researchers iterated on related terms and found that only words such as “sustainability,” “green,” “organic,” “clean” and “conservation” could highlight the sustainable consumption behavior (Serrano et al., 2021). Therefore, this paper selects reviews with these words in the sentences to identify green users in Airbnb and conduct research.
Before data feature analysis, the data needs to be preprocessed. After cleaning the data with low research values such as English, repetition and system automatic response in the reviews, 52,786 preliminarily processed data are obtained, which indicates that the percentage of green users is about 38% among all users.
This study uses the Latent Dirichlet Allocation (LDA) model to classify the text. The purpose of the LDA model is to identify topics in text, also known as a three-layer Bayesian probability model, which includes a three-layer structure of words, topics, and documents. The document is formed by multiple topics, and one topic is also formed by multiple words. In LDA, if a word w belongs to topic t with high probability, then the document d containing that word also belongs to t with high probability.
The principle of LDA is shown in Figure 1. For each word in a document d, LDA determines the topic distribution θ of a document based on prior knowledge α, and then draws a topic t from the topic distribution θ corresponding to that document, followed by determining the word distribution φ of the current topic based on prior knowledge β, and then drawing a word w from the word distribution φ corresponding to topic t. Repeating this process N times, document d is produced. Therefore, for any document, the document-topic p (t|d) and the topic-vocabulary p (w|t) can be trained according to a large number of known document-vocabulary information p (w|d). The core formula of LDA is:
Since the computer cannot identify text data directly, the Bag-of-Words model and TF-IDF model are used for text vectorization transformation. In the classification process, the perplexity and topic consistency of the model are used to identify the quality of the model’s topic classification, and the topic consistency score is more helpful for judging the topic classification effect (Chi et al., 2021). In this paper, the number of topics is determined based on the topic consistency score. When the score is 10 (Figure 2), the model fits best.
The results of the LDA model are shown in Figure 3. It can be concluded that the topics of green users in Airbnb are traffic situation, geographical location, hygiene conditions, surrounding environment, performance-cost ratio, space area, housing facilities, service attitude, housing experience, room style and so on.
Based on the LDA model, this paper summarizes 10 characteristic factors in user reviews text: external environment, internal hygiene, performance-cost ratio, space area, supporting facilities, room style, traffic situation, location condition, service attitude, and housing experience. There are many words with similar meanings in Chinese vocabulary, and due to the different personal expression habits of consumers, the phenomenon of multi-word synonyms is inevitable. The analysis of similar words is helpful to count the number of comments with characteristic words. Based on this, Word2vec word vector method is used to calculate the word similarity. Table 1 shows the list of similar words compiled.
Referring to previous studies (Cheng and Jin, 2019; Luo and Tang, 2019; Kiatkawsin et al., 2020; Villeneuve and O'Brien, 2020), combined with the classification results of the LDA model, the summarized 10 characteristic elements were further refined to obtain four dimensions of green user emotional characteristics. External environment and internal hygiene are environmental factors that influence user emotional characteristics; performance-cost ratio, space area, supporting facilities, and room style are the housing factors; traffic situation and location condition are the geographic factors; service attitude and housing experience are the human factors. Among them, housing factors and human factors are internal conditions that influence user emotional characteristics, while environmental factors and geographical factors are external conditions. Accordingly, Table 2 shows the index system of green user emotional tendency.
In the process of sentiment value calculation, the study adopts the sentiment analysis dictionary released by Hownet, from which positive evaluation words and sentiment words, as well as negative evaluation words and sentiment words are imported. Combining the characteristics of the review data, new words such as “spacious” and “old” are added to the original sentiment dictionary to improve the accuracy of sentiment calculation.
The presence of negation in a review statement is likely to change the sentiment polarity of the entire review. Therefore, judging the presence of negatives in a review statement is crucial to ensure the accuracy of sentiment calculation. Based on this, this paper introduces a negation dictionary as a way to correct the sentiment tendency. If there are multiple negations in a comment statement, an odd number of negation words indicates negation, and an even number of negation words indicates affirmation. In practice, when judging the presence of negation in a review, it is necessary to consider two special cases of emotive words at the beginning of the review and in the second position of the review. If the sentiment word is at the beginning of the sentence, there is no negation. And if the sentiment word is in the second word, the presence of negation is judged by the word before it.
The sentiment value of each review is obtained by counting the sentiment words in the review statements and judging their sentiment polarity. To obtain the comprehensive sentiment value of each feature word, it is assumed that the sentiment value set of each review containing the feature word is {xi1, xi2, xi3... xin}, where i represents the feature word, and n is the number of reviews containing word i, then the combined sentiment value of the ith feature word is obtained by taking the mean value of all reviews. The formula is:
In order to verify the influence of geography, environment, human, and housing factors on the green user experience, and to test whether there is an interaction effect between the factors, this study conducts a multiple regression analysis. Among all review data, 22,580 reviews were randomly selected. The constructed econometric model is:
Referring to the research of Lawani et al. (2018), the sentiment value of each review obtained by sentiment analysis is used as a standard to measure the green user’s sentiment tendency. Referring to the research of Chi et al. (2019), the number of words of a certain element in user reviews can reflect the user’s tendency to this element. Therefore, the frequency of words related to each element in the review is counted and used as the measurement standard of the independent variables. Furthermore, the length of the reviews and the words related to green life appearing in the reviews may affect the results. Therefore, the length of the reviews and the green attitude are included as control variables, measured by the frequency of related words. The details of the variable definitions and measurements are shown in Table 3.
After defining the variables, import the data into Stata for descriptive statistics and correlation coefficient calculation. The analysis results are shown in Table 4. The green user emotion tendency (GUET) has a minimum value of −4 and a maximum value of 22, indicating that the sentiment of user reviews is more inclined to be positive. The independent variables are all count data, and the minimum value is 0, indicating that different users pay different attention to different factors. All the explanatory variables are related to the explained variables, and the correlation coefficient of each variable is lower than 0.75, indicating that there is no serious multi-collinearity among the variables, and regression analysis can be performed.
DEMATEL is an effective method for factor analysis. It mainly uses graph theory to calculate the degree of influence and the influenced of each factor on the other factors by constructing a direct influence matrix that responds to the logical relationship between the factors, and then calculates the centrality and causality of each factor.
The direct impact matrix X was constructed based on the proportional relationship among the reviews containing each topic term in the total reviews.
In order to analyze the indirect influence relationship between the elements, the integrated influence matrix T needs to be calculated, and the formula is
After obtaining the combined influence matrix, causality diagrams need to be constructed based on the centrality and causality of each factor. Table 5 reflects the degree of influence (D), degree of being influenced (R), centrality (D + R) and causality (D-R) of each factor.
As can be seen from Figure 4, the cause degree value of HU and GE indicators is greater than 0, which is cause index. And the cause degree of EN and HO indicators is less than 0, which is result index. This indicates that the indicator ability of human factors and geographical factors affect the indicator ability of environmental factors and housing factors. The value of centrality indicates the role of the indicator in the emotional tendency of green users. Therefore, in the DEMATEL method, the four indicators are ranked as housing, geography, human and environment.
FIGURE 4. The causal relationship between the indicators of green users’ emotional tendencies at the element level.
In addition, according to the integrated influence value of green users’ emotional tendency, it is known that the four indicators are mutually influenced and interrelated, which provides support for the construction of ANP model.
As an extension of the Analytic Hierarchy Process (AHP), Analytic Network Process (ANP) comprehensively takes into account the correlation within the indicators. In order to explore the influence of different factors on the overall emotional tendency of users more accurately, this paper uses the ANP model to calculate the weight of each influencing factor. Figure 5 reflects the ANP model of green user emotional tendency.
When determining the judgment matrix, this paper adopts the 1–9 scale to construct the judgment matrix. The importance of each element is determined according to the difference in the proportion of the number of reviews containing each feature word in the total reviews.
According to Table 6, it can be found that the index with the most significant proportion of reviews is housing experience, accounting for 19%, while the smallest is room style, accounting for 3.7%, and the difference between the two is 15.3%. According to the 9-level scale division, the inter-level scale division is carried out with 0.017, and the index of scale division is shown in Table 7.
After calculating the comprehensive sentiment value of each feature word, the results are shown in Table 8.
In this paper, the hierarchical multiple regression method is used for regression, and the results are shown in Table 9. Model 1 shows that both the green attitude and the length of the comment sentence have a significant positive impact on the emotional tendency of green users (β = 0.374, p < 0.001; β = 0.151, p < 0.001). Models 2–9 show that both independent variables and interaction terms have a significant impact on the emotional tendencies of green users. Model 10 presents the overall impact of independent variables and interaction terms on green user’s emotional tendencies. The results show that there is an interaction effect among the factors that influence the green users’ emotional tendencies. Both hypothesis one and hypothesis two are valid. Therefore, when calculating the overall emotional characteristics of green users, the interaction effects need to be taken into account.
After the construction of the judgment matrix, this paper uses Yaanp to calculate the weights. The judgment matrix of each level has passed the consistency test, and the weight results of each level index are shown in Table 10.
Based on the sentiment value of feature words and the weights of each index layer, the study measures the overall emotional characteristics of green users in Airbnb in Beijing. First, the emotional features of the index layer are calculated. The formula is
Then, the comprehensive emotional characteristics of the element layer are calculated, and the formula is:
By calculating the overall emotional characteristics of green users. It can be found that when Airbnb green users consume, the emotional tendency towards human factors is more obvious, with a score of 1.256, indicating that the overall experience of green users is more influenced by human factors such as housing experience and host service attitudes. The scores of geographical factors and housing factors are 0.162 and 0.036, both of which have a certain impact on the overall emotional characteristics of green users. The environmental factor has the lowest score in the comprehensive emotional tendency, with a score of 0.017. This is contrary to the perception that the consumption habits of green users should be affected by the environment.
Beijing is a representative city in northern China. The previous article analyzed the emotional characteristics of green users in Beijing Airbnb. Due to the vast territory of China, the consumption habits of different regions will be different. In order to explore the spatial distribution characteristics of green user sentiment in Airbnb, this paper further selects the online reviews of green users in Airbnb in Shanghai and Hong Kong for calculation. Among them, Shanghai is a representative city in southern China, and Hong Kong is a special administrative region of China. Selecting Beijing, Shanghai and Hong Kong for spatial feature analysis, the overall emotional tendencies of green users towards different factors can be observed more clearly. The spatial features are compared in Tables 13, 14
TABLE 13. Comparison of emotional characteristics of green users in Beijing, Shanghai and Hong Kong (I).
TABLE 14. Comparison of emotional characteristics of green users in Beijing, Shanghai and Hong Kong (II).
Through the comparison of spatial characteristics, the emotional tendencies of Airbnb green users in the three places are generally consistent. Human factors and geographic factors are the main factors influencing the consumer experience, followed by housing factors. Human factors are the primary factor influencing the green user experience of Airbnb in Beijing and Shanghai, followed by geographic factors. In Hong Kong, the geographical factor is in the first place, followed by the human factor. One possible explanation is that most of the renters who choose Airbnb in Hong Kong are travelers who have traveled long distances. When renting a house, these groups will first consider the convenience of transportation and the superiority of the geographical location to soothe their fatigue. It should be noted that in the comparison of the emotional characteristics of green users in the three places, environmental factors are always in the last position. Figure 6 visually shows the results of the spatial feature analysis.
Exploring the emotional characteristics of green users in Airbnb is essential to promoting green consumption and realizing the sustainable development of the Airbnb market. This paper obtains the online review data in Inside Airbnb, extracts four dimensions that influence user emotional characteristics through the LDA model: human factors, environmental factors, geographical factors and housing factors, and constructs an indicator system. Then, the sentiment value of online reviews is calculated, and the interaction effect of each factor was tested by multiple regression statistical analysis. Following that, the study uses the DEMATEL-ANP model to get the weight of each element. Finally, the emotional value and weight of each element are combined to calculate the overall emotional characteristics of green users in Airbnb under the influence of multi-factor interaction.
The results of research on Airbnb green users in Beijing show that human factors, namely housing experience and service attitude, are the most important factors influencing user experience. Environmental factors, especially the external environment of the housing, have the lowest score, indicating that environmental motivation is not the main motivation for green users to choose Airbnb. In the analysis of the spatial characteristics of Beijing, Shanghai and Hong Kong, it is found that the green users’ emotional tendencies are generally similar, and the environmental factors are in the last place, which shows that the public’s green consumption values still need to be further shaped and improved.
In general, the Airbnb platform, hosts and tenants act as a community of interests in the shared accommodation chain. Exploring the emotional characteristics of green users under the influence of multi-factor interaction can help the platform quickly identify users’ emotional tendencies in different dimensions and formulate appropriate market development strategies. The scoring algorithm should be optimized according to user online reviews, and the reviews of different dimensions should be given matching weights, so that accurate user scores can provide reference for hosts to publish listing information and tenants to select listings so as to realize the coordinated development among shared accommodation entities.
Uncovering the emotional characteristics of green users in Airbnb is an important aspect to promote the growth of the shared accommodation market. By constructing an evaluation index system influenced by multi-factor interaction, this paper analyzes the emotional characteristics of green users’ online reviews in Airbnb. And deeply understands users’ emotional tendencies in different dimensions, which fills the gap in the analysis of emotional characteristics under the influence of multi-factor interaction.
In order to effectively solve the influence of the weight of factors affecting user experience on the results, this paper obtains the weight of each element by DEMATEL-ANP on the basis of calculating the sentiment value of reviews, and integrates the sentiment value and weight to obtain the emotional characteristics of green users. It provides a new idea for the related research of text analysis. At the same time, the multivariate regression analysis was used to test the interaction effect between the factors, which verified the necessity of using the ANP model.
This paper analyzes the emotional characteristics of green users quantitatively from the perspective of the Airbnb platform. Few studies have explored the impact of environmental dimensions on user experience before. Therefore, this paper divides the dimensions that influence green user experience into four categories: housing, human, geography and environment. Through the calculation of the emotional characteristics of green users in Beijing, Shanghai and Hong Kong, it is found that the emotional inclinations of green users in the three places generally show the same trend, and the emotional inclinations towards environmental factors are relatively low.
The sharing economy is an important support for sustainable development, and attracting more consumers to participate in the sharing economy is a key part of promoting social development. Although the economic model of shared accommodation is better than traditional budget hotels (Nie et al., 2020). However, in the era of fast consumption, the market environment is changing rapidly, and the Airbnb platform must continuously improve user satisfaction and corporate competitiveness. This paper proposes a method to measure the overall emotional characteristics of green users under the influence of multi-factor interaction. Based on this, Airbnb platform managers can formulate new user rating strategies, optimize the scoring algorithm, and assign different weights to topics related to user experience in reviews, so as to accurately analyze the development direction of the platform.
According to the research in this paper, green users in Airbnb pay more attention to human factors and geographic factors in their housing experience, followed by housing factors, and have the lowest emotional inclination towards environmental factors. To guide more users to green consumption, Airbnb should strengthen its publicity on the environment, highlighting the sharing, affordability, and environmental protection of the listings. In addition, the platform can introduce a crowd sourcing mechanism to guide tenants to put forward specific needs before choosing a listing, and allow hosts to review these needs and provide personalized listing matching services. Provide appropriate economic incentives to hosts who are satisfied with their users and have excellent service attitudes to promote the virtuous cycle development of the Airbnb market, and can also effectively avoid offline transactions between hosts and tenants (Li and Mu, 2021).
In the descriptive statistical analysis of the sentiment value of users’ online reviews, it is found that the user’s positive sentiment tendency is relatively obvious, which is similar to some other findings (Hernández-López, 2019). This may be due to the fact that consumers can directly contact the hosts and the lack of anonymity of the comment mechanism (Bridges and Vásquez, 2018). Therefore, the platform should continue to improve the construction of the mutual evaluation mechanism between tenants and hosts to ensure anonymity in the process of mutual evaluation, to guide tenants to express their real experience opinions in the online reviews and improve the accuracy of the analysis of users’ overall emotional characteristics.
As the direct providers of listings, hosts are mostly economically motivated (Bremser and Wüst, 2021) to share their homes, which means that hosts want more and more tenants to choose their listings. Appropriate descriptions of listing information usually attract more tenants (Tao et al., 2022). The consumption habits of green users are more likely to establish a trusting relationship with hosts and drive the healthy development of the market. Hosts should pay attention to the emotional inclinations of green users on different dimensions in their online reviews, and adjust the focus of listing information. Users with green consumption intention can quickly locate the listings that meet their requirements based on the information when choosing listings. Therefore, exploring the emotional characteristics of green users under the interaction of multiple factors can achieve a win-win situation for platform companies, hosts, and tenants.
It should be noted that the overall experience of green users in Airbnb is less emotionally inclined to environmental factors. This shows that environmental motivation is not the main motivation for green users to participate in the sharing economy at this stage. With the change of social environment, green consumption has gradually become the mainstream of social development. As the regulator of macro policies, the government must take corresponding measures to promote green consumption and environmentally sustainable closed-loop development. On the one hand, the government should strengthen the supervision of shared accommodation platform companies, which is because the shared accommodation platform is the first gateway for consumers to choose, and the concept of the platform will have a greater impact on consumer behavior. Therefore, the government should urge enterprises to carry out green production and take green and sustainable development as part of the enterprise strategy. On the other hand, the government should promote consumers’ awareness of environmental protection, which requires the government to intensify its efforts to shape correct environmental values that are conducive to green consumption, and input them into the whole society through education, publicity and other channels. In addition, to reduce the barriers to trust between shared accommodation entities, the government should simplify the transfer process of the right to use shared accommodation resources, legalize the division of responsibilities between hosts and tenants, and ensure the steady progress of the policy of “housing without speculation.” As a result, more green consumption behaviors will be generated, and hosts are more willing to share their housing resources, so the shared accommodation market can achieve healthy development.
There are shortcomings in this paper: 1) The study obtained the weights of factors affecting the green user experience of Airbnb in China, combined with the emotional value of the overall emotional characteristics of green users. In the future, the research can measure the real ratings of the listings and comparing them with the ratings of existing listings. By which it could provide a fuller reference for tenants based on the optimization of listing ratings. 2) In the analysis of the emotional characteristics of green users of Airbnb in China, this paper only selects the review data of Beijing, Shanghai, and Hong Kong, which may make the results biased. In the future, if Inside Airbnb can provide data on more cities, further comparative analysis can be taken.
The original contributions presented in the study are included in the article/Supplementary Material, further inquiries can be directed to the corresponding author.
JW: Responsible for planning the overall structure of the article and revising the first draft of the article. CW: Responsible for data collection and collation, and for writing the first draft of the paper.
This research was funded by Humanities and Social Science Youth Program of the Ministry of Education of China, Grant Number 16YJC790099.
The authors declare that the research was conducted in the absence of any commercial or financial relationships that could be construed as a potential conflict of interest.
All claims expressed in this article are solely those of the authors and do not necessarily represent those of their affiliated organizations, or those of the publisher, the editors and the reviewers. Any product that may be evaluated in this article, or claim that may be made by its manufacturer, is not guaranteed or endorsed by the publisher.
Ahani, A., Nilashi, M., Zogaan, W. A., Samad, S., Aljehane, N. O., Alhargan, A., et al. (2021). Evaluating medical travelers’ satisfaction through online review analysis. J. Hosp. Tour. Manag. 48, 519–537. doi:10.1016/j.jhtm.2021.08.005
Ayar, I., and Gürbüz, A. (2021). Sustainable consumption intentions of consumers in Turkey: a research within the theory of planned behavior. SAGE Open 11 (3), 215824402110475. doi:10.1177/21582440211047563
Banytė, J., šalčiuvienė, L., Dovalienė, A., Piligrimienė, Ž., and Sroka, W. (2020). Sustainable consumption behavior at home and in the workplace: avenues for innovative solutions. Sustainability 12 (16), 6564. doi:10.3390/su12166564
Bao, Y., Ma, E., La, L., Xu, F., and Huang, L. (2022). Examining the Airbnb accommodation experience in Hangzhou through the lens of the experience economy model. J. Vacat. Mark. 28 (1), 95–116. doi:10.1177/13567667211024707
Betzler, S., Kempen, R., and Mueller, K. (2022). Predicting sustainable consumption behavior: knowledge-based, value-based, emotional and rational influences on mobile phone, food and fashion consumption. Int. J. Sustain. Dev. World Ecol. 29 (2), 125–138. doi:10.1080/13504509.2021.1930272
Biswas, A., and Roy, M. (2015). Green products: an exploratory study on the consumer behaviour in emerging economies of the East. J. Clean. Prod. 87, 463–468. doi:10.1016/j.jclepro.2014.09.075
Böcker, L., and Meelen, T. (2017). Sharing for people, planet or profit? analysing motivations for intended sharing economy participation. Environ. Innov. Soc. Transit. 23, 28–39. doi:10.1016/j.eist.2016.09.004
Bremser, K., and Wüst, K. (2021). Money or love - why do people share properties on Airbnb? J. Hosp. Tour. Manag. 48, 23–31. doi:10.1016/j.jhtm.2021.05.009
Bridges, J., and Vásquez, C. (2018). If nearly all Airbnb reviews are positive, does that make them meaningless? Curr. Issues Tour. 21 (18), 2065–2083. doi:10.1080/13683500.2016.1267113
Canziani, B., and Nemati, H. R. (2021). Core and supplemental elements of hospitality in the sharing economy: insights from semantic and tonal cues in Airbnb property listings. Tour. Manag. 87, 104377. doi:10.1016/j.tourman.2021.104377
Ceptureanu, E. G., Ceptureanu, S. I., Herteliu, C., and Cerqueti, R. (2020). Sustainable consumption behaviours in P2P accommodation platforms: an exploratory study. Soft Comput. 24 (18), 13863–13870. doi:10.1007/s00500-020-04681-6
Chen, B., Feng, Y., Sun, J., and Yan, J. (2020). Motivation analysis of online green users: evidence from Chinese “ant forest”. Front. Psychol. 11, 1335. doi:10.3389/fpsyg.2020.01335
Chen, Y., Liu, P., Zhang, J., Xiang, C., and Tang, L. (2020). Distant home: a study on the accommodation choice and experience of Chinese tourists in sharing accommodation. World Reg. Stud. 29 (1), 181–191. doi:10.3969/j.issn.1004-9479.2020.01.2018367
Cheng, M., and Jin, X. (2019). What do Airbnb users care about? An analysis of online review comments. Int. J. Hosp. Manag. 76, 58–70. doi:10.1016/j.ijhm.2018.04.004
Chi, M., Liu, S., Lu, X., and Luo, B. (2019). A study of the mechanism influencing hosts' willingness to sustain participation on shared accommodation platforms: a perspective of platform network effects. Nankai Manag. Rev. 22, 103–113.
Chi, M., Pan, M., and Wang, W. (2021). A cross-platform comparative study of shared accommodation and hotel user review texts: an LDA-based thematic social network and sentiment analysis. Libr. Intell. Works 65, 107–116. doi:10.13266/j.issn.0252-3116.2021.02.011
Choi, D., and Johnson, K. K. P. (2019). Influences of environmental and hedonic motivations on intention to purchase green products: an extension of the theory of planned behavior. Sustain. Prod. Consum. 18, 145–155. doi:10.1016/j.spc.2019.02.001
Curtis, S. K., and Lehner, M. (2019). Defining the sharing economy for sustainability. Sustainability 11 (3), 567. doi:10.3390/su11030567
Demir, E., and Emekli, G. (2021). Is Airbnb no longer a sharing economy platform? evidence from Europe's top 10 Airbnb destinations. Anatolia 32 (3), 470–488. doi:10.1080/13032917.2021.1890626
Farmaki, A., and Stergiou, D. P. (2019). Escaping loneliness through Airbnb host-guest interactions. Tour. Manag. 74, 331–333. doi:10.1016/j.tourman.2019.04.006
Gao, P., Meng, F., Ahmed, Z., Oláh, J., and Harsányi, E. (2022). A path towards green revolution: how do environmental technologies, political risk, and environmental taxes influence green energy consumption? Front. Environ. Sci. 10, 927333. doi:10.3389/fenvs.2022.927333
Hamari, J., Sjöklint, M., and Ukkonen, A. (2016). The sharing economy: why people participate in collaborative consumption. J. Assoc. Inf. Sci. Technol. 67 (9), 2047–2059. doi:10.1002/asi.23552
Hernández-López, M. D. L. O. (2019). Relational work in Airbnb reviews. Russ. J. Linguistics 23 (4), 1088–1108. doi:10.22363/2687-0088-2019-23-4-1088-1108
Hossain, M. (2020). Sharing economy: a comprehensive literature review. Int. J. Hosp. Manag. 87, 102470. doi:10.1016/j.ijhm.2020.102470
Hosta, M., and Zabkar, V. (2021). Antecedents of environmentally and socially responsible sustainable consumer behavior. J. Bus. Ethics 171 (2), 273–293. doi:10.1007/s10551-019-04416-0
Huertas, A., Ferrer-Rosell, B., Marine-Roig, E., and Cristobal-Fransi, E. (2021). Treatment of the Airbnb controversy by the press. Int. J. Hosp. Manag. 95, 102762. doi:10.1016/j.ijhm.2020.102762
Hurley, P. (2018). A concise introduction to logic. 13th Edition. Boston, United States: Cengage Learning.
Kiatkawsin, K., Sutherland, I., and Kim, J. (2020). A comparative automated text analysis of Airbnb reviews in Hong Kong and Singapore using latent Dirichlet allocation. Sustainability 12 (16), 6673. doi:10.3390/su12166673
Kim, D., Park, S., and Yi, S. (2021). Relevant and rich interactivity under uncertainty: Guest reviews, host responses, and guest purchase intention on Airbnb. Telemat. Inf. 65, 101708. doi:10.1016/j.tele.2021.101708
Lalicic, L., Marine-Roig, E., Ferrer-Rosell, B., and Martin-Fuentes, E. (2021). Destination image analytics for tourism design: an approach through Airbnb reviews. Ann. Tour. Res. 86, 103100. doi:10.1016/j.annals.2020.103100
Landon, A. C., Woosnam, K. M., and Boley, B. B. (2018). Modeling the psychological antecedents to tourists’ pro-sustainable behaviors: An application of the value-belief-norm model. J. Sustain. Tour. 26(6), 957–972. doi:10.1080/09669582.2017.1423320
Lawani, A., Reed, M. M. R., Mark, T., and Zheng, Y. (2018). Reviews and price on online platforms: evidence from sentiment analysis of Airbnb reviews in Boston. Reg. Sci. Urban Econ. 75, 22–34. doi:10.1016/j.regsciurbeco.2018.11.003
Lee, C. K. H., Tse, Y. K., Zhang, M., and Ma, J. (2019). Analysing online reviews to investigate customer behaviour in the sharing economy. Inform. Technol. Peopl. 33(3), 945–961. doi:10.1108/ITP-10-2018-0475
Li, X., Wu, C., and Mai, F. (2019). The effect of online reviews on product sales: a joint sentiment-topic analysis. Inf. Manag. 56 (2), 172–184. doi:10.1016/j.im.2018.04.007
Li, Y., and Mu, L. (2021). The role of offline trade in sharing accommodation. Decis. Sci., 12523. doi:10.1111/deci.12523
Liu, Y., Meng, L. K., Bao, J. G., and Zhao, C. D. (2021). A comparative study of sentiment computing methods: will machine learning Be overwhelming? Nankai Bus. Rev. 24 (05), 63–74.
Lucini, F. R., Tonetto, L. M., Fogliatto, F. S., and Anzanello, M. J. (2020). Text mining approach to explore dimensions of airline customer satisfaction using online customer reviews. J. Air Transp. Manag. 83, 101760. doi:10.1016/j.jairtraman.2019.101760
Luo, Y., and Tang, R. L. (2019). Understanding hidden dimensions in textual reviews on Airbnb: an application of modified latent aspect rating analysis (LARA). Int. J. Hosp. Manag. 80, 144–154. doi:10.1016/j.ijhm.2019.02.008
Möller, J., and Herm, S. (2021). Perceptions of green user entrepreneurs’ performance—is sustainability an asset or a liability for innovators? Sustainability 13 (6), 3580. doi:10.3390/su13063580
Nie, R., Tian, Z., Wang, J., and Chin, K. S. (2020). Hotel selection driven by online textual reviews: applying a semantic partitioned sentiment dictionary and evidence theory. Int. J. Hosp. Manag. 88, 102495. doi:10.1016/j.ijhm.2020.102495
Sanasi, S., Ghezzi, A., Cavallo, A., and Rangone, A. (2020). Making sense of the sharing economy: a business model innovation perspective. Technol. Anal. Strateg. Manag. 32 (8), 895–909. doi:10.1080/09537325.2020.1719058
Serrano, L., Ariza-Montes, A., Nader, M., Sianes, A., and Law, R. (2021). Exploring preferences and sustainable attitudes of Airbnb green users in the review comments and ratings: a text mining approach. J. Sustain. Tour. 29 (7), 1134–1152. doi:10.1080/09669582.2020.1838529
Sun, S., Zhang, S., and Wang, X. (2021). Characteristics and influencing factors of Airbnb spatial distribution in China’s rapid urbanization process: a case study of nanjing. PLoS One 16 (3), e0248647. doi:10.1371/journal.pone.0248647
Tao, D., Fang, W., Luo, B., and Wan, L. (2022). Which marketer-generated-content is more effective? an experimental study in the context of a peer-to-peer accommodation platform. Int. J. Hosp. Manag. 100, 103089. doi:10.1016/j.ijhm.2021.103089
Taube, O., Kibbe, A., Vetter, M., Adler, M., and Kaiser, F. G. (2018). Applying the Campbell Paradigm to sustainable travel behavior: compensatory effects of environmental attitude and the transportation environment. Transp. Res. Part F Traffic Psychol. Behav. 56, 392–407. doi:10.1016/j.trf.2018.05.006
Tussyadiah, I. P., and Zach, F. (2017). Identifying salient attributes of peer-to-peer accommodation experience. J. Travel Tour. Mark. 34 (5), 636–652. doi:10.1080/10548408.2016.1209153
Villeneuve, H., and O'Brien, W. (2020). Listen to the guests: text-mining Airbnb reviews to explore indoor environmental quality. Build. Environ. 169, 106555. doi:10.1016/j.buildenv.2019.106555
von Richthofen, G., and von Wangenheim, F. (2021). Managing service providers in the sharing economy: insights from Airbnb’s host management. J. Bus. Res. 134, 765–777. doi:10.1016/j.jbusres.2021.06.049
Wang, C. R., and Jeong, M. (2018). What makes you choose Airbnb again? an examination of users’ perceptions toward the website and their stay. Int. J. Hosp. Manag. 74, 162–170. doi:10.1016/j.ijhm.2018.04.006
Wang, J., and Yu, X. (2021). The driving path of customer sustainable consumption behaviors in the context of the sharing economy—based on the interaction effect of customer signal, service provider signal, and platform signal. Sustainability 13 (7), 3826. doi:10.3390/su13073826
Wang, P., Zhang, X. F., and Chi, M. M. (2019). A review of research on inter-subject trust in shared accommodation: an analysis based on the S-O-R framework. E-Government. 12, 108–116. doi:10.16582/j.cnki.dzzw.2019.12.011
Wang, Y., Xiang, D., Yang, Z., and Ma, S. S. (2019). Unraveling customer sustainable consumption behaviors in sharing economy: a socio-economic approach based on social exchange theory. J. Clean. Prod. 208, 869–879. doi:10.1016/j.jclepro.2018.10.139
Wegmann, J., and Jiao, J. (2017). Taming Airbnb: Toward guiding principles for local regulation of urban vacation rentals based on empirical results from five US cities. Land Use Policy 69, 494–501. doi:10.1016/j.landusepol.2017.09.025
Xu, X. (2021). What are customers commenting on, and how is their satisfaction affected? examining online reviews in the on-demand food service context. Decis. Support Syst. 142, 113467. doi:10.1016/j.dss.2020.113467
Yakubu, H., Kwong, C. K., and Lee, C. K. M. (2021). A multigene genetic programming-based fuzzy regression approach for modelling customer satisfaction based on online reviews. Soft Comput. 25 (7), 5395–5410. doi:10.1007/s00500-020-05538-8
Yi, B., Shi, D., Shi, F., and Zhang, L. (2021). Do the flipped impacts of hotels matter to the popularity of Airbnb? Int. J. Contemp. Hosp. Manag. 33 (6), 2239–2263. doi:10.1108/IJCHM-06-2020-0582
Keywords: green consumption, green users, Airbnb, emotional characteristics, multi-factor interaction, online reviews
Citation: Wang J and Wang C (2022) Analysis of airbnb’s green user emotional characteristics: How do human, geographical, housing, and environmental factors influence green consumption?. Front. Environ. Sci. 10:993677. doi: 10.3389/fenvs.2022.993677
Received: 14 July 2022; Accepted: 18 July 2022;
Published: 19 August 2022.
Edited by:
Shuhong Wang, Shandong University of Finance and Economics, ChinaCopyright © 2022 Wang and Wang. This is an open-access article distributed under the terms of the Creative Commons Attribution License (CC BY). The use, distribution or reproduction in other forums is permitted, provided the original author(s) and the copyright owner(s) are credited and that the original publication in this journal is cited, in accordance with accepted academic practice. No use, distribution or reproduction is permitted which does not comply with these terms.
*Correspondence: Juying Wang, d2FuZ2p1eWluZ0BvdWMuZWR1LmNu
Disclaimer: All claims expressed in this article are solely those of the authors and do not necessarily represent those of their affiliated organizations, or those of the publisher, the editors and the reviewers. Any product that may be evaluated in this article or claim that may be made by its manufacturer is not guaranteed or endorsed by the publisher.
Research integrity at Frontiers
Learn more about the work of our research integrity team to safeguard the quality of each article we publish.