- College of Applied Business Administration, King Saud University, Riyadh, Saudi Arabia
The Bootstrap Autoregressive Distributed Lag (BARDL) cointegration model used to examine whether there is a short and long-run relationship between health expenditure per capita Environmental Sustainability is measured by CO2 emissions per capita (CO2), GDP per capita (GDPPC), and Green Energy is measured by Electric Power Consumption per capita (GEPC) in Saudi Arabia using annual time-series data from 1995 to 2021. The significant results show no long-term cointegration relationship between Saudi Arabian variables. Therefore, the Bootstrap ARDL Bound Test offered significant empirical support for the validity of no cointegration between the variables. The empirical data show a unidirectional relationship between GDP per capita and health expenditure, green energy and CO2 emissions per capita. The data also show a bidirectional relationship between health expenditure and CO2 emissions and GDP per growth, whereas the same relation hold between green energy and economic growth. These findings would attract policymakers to expand economic growth and development’s environmental and health benefits. Moreover, to reduce the effects of global warming without affecting environmental health or the country’s long-term economic prosperity.
1 Introduction
Global precipitation and temperature elevation are influenced by climate change caused by rising greenhouse gas emissions. Climate change is an undeniable reality that severely threatens the long-term viability of humanity, society, the economy, and the environment. High greenhouse gas concentrations, climate change, and the impact of global warming on public health demonstrate a significant relation (Wang C.-M. et al., 2019). Urbanization, industrialization, and increased economic growth are associated with worsening air quality, resulting from the emission of various air pollutants into the environment. This environmental health deterioration brings detrimental impacts on people’s health. Recently, the association between economic growth, environmental degradation, and healthcare expenditure in developed and developing countries has received increased attention in the literature (Yue et al., 2021).
Because of the expanding international awareness of the strong relationship between economic growth and health, the contribution of health economics is becoming increasingly important. Furthermore, child mortality is essential for socio-economic development because childhood health is one of the most important indicators of later life health and productivity. Several upstream variables, such as the environment, economic area, and parents’ level of education, have been explored in the context of factors driving high child mortality worldwide. At the same time, nations have focused policies on healthcare expenditures, recognizing that countries with high healthcare spending have succeeded in decreasing mortality rates.
Inequality, unemployment, and low incomes are critical economic difficulties facing emerging nations and environmental protection organizations. Furthermore, leaders in emerging countries are attempting to boost low-income households, which will bring additional negative repercussions such as global warming, natural resource depletion, and contamination of the environment (Ahmed et al., 2022).
Saudi Arabia is the world’s second-biggest country in proved oil reserves, with 267.1 billion barrels, accounting for 17.2% of global oil reserves, expected to total 1.55 trillion barrels by 2020. Environmental protection challenges in Saudi Arabia are inextricably linked to the Saudi population’s energy and oil consumption behavior. Saudi Arabia’s total oil consumption is quickly increasing, with energy consumption per capita reaching 6.8 tons of oil equivalent in 2021, four times the global average (Alkhathlan & Javid, 2015). The Saudi Arabian economy depends on fossil fuel production. Globally, it ranks among the world’s ten largest emitters (Ahmed et al., 2016; Omri et al., 2022). Adverse health outcome is one of the consequences that encounter this development.
In 2016, CO2 emissions in Saudi Arabia had fallen after increasing, partially due to lower oil consumption in the electricity sector. As a result of the ' 'country’s effective use of energy, CO2 emissions have constantly increased alongside industrial, residential, agricultural, commercial, and transportation activities. The CO2 emissions fluctuated through the years; the maximum emission was 19.38 tons per capita in 2015, decreasing to 17 in 2021 (Figure 1).
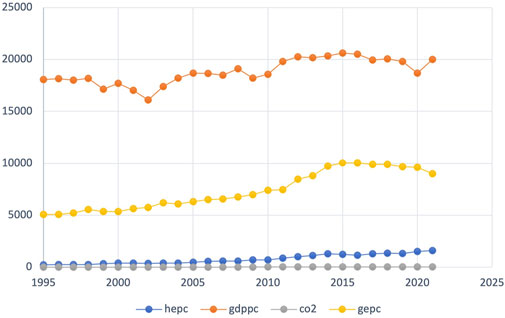
FIGURE 1. Relationship between CO2 emissions, Economic Growth (GDPPC), Health Expenditure (HEPC) and Green Energy (GEPC) for the period 1995–2021.
Saudi Arabia’s GDP per capita averaged 44,500.37 US dollars from 1990 to 2020, with a low of 38,170.22 US dollars in 2002 and a high of 48,921.21 US dollars in 2015. The most recent value from 2020 is 44,328.18 USD. In 2020, the world average based on 175 nations was 19,632.86 US dollars (https://data.worldbank.org/country/SA, 2021). The combined effect of an increase in both GDP and health expenditure caused changes in the ratio of health expenditures to GDP.
Increasing population and economic advancement have gradually increased energy consumption throughout time. As a result, these have become some of the most important factors of economic growth. Researchers, politicians, and governments have paid close attention to energy consumption. Since the 1970s, evaluations have focused on energy’s practical and theoretical elements. Power is a clear distinction, defined as a profitable commodity or a production factor required to keep the production process running (Ferguson et al., 2000). Because of the critical significance of energy in people’s and society’s lives, Saudi Arabia’s electricity sector has received particular attention as part of the country’s remarkable development renaissance (Abdel-Aal et al., 1997). Saudi Arabia’s primary energy resources are fuel and electricity, as it is primarily an oil-producing country. Saudi Arabia’s economy has progressed due to a focus on the component industries. Such a focus is ascribed to the more significant economic benefits of using electricity instead of other energy sources (Al-Garni et al., 1994).
Saudi Arabia was chosen as the subject for this study because its strong economic and industrial development has significantly increased health expenditure and CO2 emissions. In recent years during the COVID-19 pandemic, historically high international oil prices and substantial local fuel subsidies have influenced current economic development and high health expenditure. Furthermore, this research deals with the most important economic factors affecting the environmental deterioration represented by CO2 emissions, health expenditures, population, and GDP per capita. Therefore, this study includes the ecological dimension when analyzing economic relations.
Understanding the relationship between health expenditure, economic growth, and CO2 emissions as a measure of environmental degradation provides critical evidence of the impact of financial challenges. Developed and developing countries are susceptible to global warming consequences due to rising energy consumption and the resulting CO2 emissions. Aside from a small amount of research on the overall direction of the causal relationship between health expenditure, economic growth, green energy, and CO2 emissions, there has been no previous research on Saudi Arabia utilizing the bootstrap ARDL bound test.
As discussed the importance of health expenditures, Saudi Arabia is not a different case. Health is the basic element for increasing life expectancy; therefore, it is crucial to empirically test the key determinants that determine, deteriorate or even further boost health expenditures.
The main motivation of this study is based on 1) To empirically test the impact of income on health expenditures for Saudi Arabia from 1995 to 2021, 2) to test the role of green energy in terms of health expenditures 3) to check the role of carbon emissions and how it can be crucial in terms of health expenditures. To achieve these objectives, the current study should use the Bootstrap autoregressive distributed lags model (BARDL), a novel strategy approach developed by (McNown et al., 2018).
The paper is separated into five sections. Section 2 presents a review of the literature. The data and methods used are described in Section 3. Section 4 shows the results and discussion, and Section 5 concludes the research.
2 Literature review
Studies indicated a long-term cointegration link between health expenditure and economic growth. A study of twenty Pacific and Southeast Asian developing countries (Rizvi, 2019), discovered that increasing health expenditure leads to improved economic growth when adjusted for the quality of government expenditures (Sarpong et al., 2020). found that an increase in health expenditure led to a rise in economic development according to a study of 35 Sub-Saharan African countries.
In contrast, health spending does not impact child health in low-income and lower-middle-income countries. Research has also revealed that health expenditure influences mortality rates more significantly than private spending at lower development levels. In contrast, personal health spending has a favorable impact on child mortality at higher development levels.
In their study of more than 70 developing countries (Khaleghian & Gupta, 2005), found that health expenditure significantly influences the poor in low-income countries more than in high-income countries. The returns on health investment are greater in low-income countries. While economic growth is one of the main determinants of health outcomes in developing countries, government health expenditure represents a significant impact, according to (Bokhari et al., 2007). Several studies found a dependent causality between the variables regarding the relationship between population, CO2 emissions, and economic growth. According to (Begum et al., 2015), the effect of population increase on CO2 emissions in Malaysia is positive but small. In Malaysia, population expansion was not a critical factor influencing CO2 emissions.
Meanwhile, from 1970 to 1980, per capita GDP had a negative impact on per capita CO2 emissions in Malaysia. This indicates that an increasing per capita GDP reduces per capita CO2 emissions. However, from 1980 to 2009, CO2 emissions positively affected GDP per capita. This result suggests that per capita GDP grows with per capita CO2 emissions.
The autoregressive distributive lag (ARDL) model was used to analyze the dynamic links between CO2 emissions, health expenditures, and economic development in gross fixed capital creation and per capita trade for Pakistan from 1995 to 2017. Their findings reveal strong long- and short-term causal links in Pakistan between health expenditure, CO2 emissions, and economic development. Granger causality is seen in a bidirectional relation between health expenditures and CO2 emissions and health expenditures and economic growth. Short-term unidirectional causation connects carbon emissions to healthcare expenditure. The bidirectional causal link between carbon emissions and development is also explored (Wang Z. et al., 2019).
(Li et al., 2022) studied the association between health expenditures, CO2 emissions, and GDP variations in BRICS nations from 2000 to 2019 using the Fourier autoregressive distributed lag (ARDL) model. Small samples can benefit from tighter inspection findings thanks to the Fourier ARDL model’s bootstrap repeated simulation computations. They revealed that Brazil and China had cointegration linkages between health expenditure, CO2 emissions, and economic development in the long run. In the near term, there is a negative causal association between India’s CO2 emissions and health expenditure, with health expenditure and economic growth as independent variables; other nations only exhibit a one-way relationship between CO2 emissions, health expenditure, and economic growth.
From 1995 to 2013 (Chaabouni & Saidi, 2017), studied the causal association between CO2 emissions, health expenditure, and GDP growth in 51 countries (divided into low-, middle-, and high-income countries). This link is investigated using dynamic simultaneous-equations models and the Generalized Method of Moments (GMM). The findings are significant, showing that the three factors have causation. The empirical results reveal a bidirectional correlation between CO2 emissions, GDP per capita, health expenditure, and economic growth for the three groups of estimates. The findings also show a unidirectional correlation between CO2 emissions and health expenditure in low-income countries.
Researchers have also examined the causal linkages between healthcare expenditure and economic growth (Wu et al., 2020). (Piabuo & Tieguhong, 2017) discovered a bi-directional correlation between growth and health expenditure in nations with lower health expenditure but only unilateral causality in countries with higher health expenditure.
(Hassan & Kalim, 2012) used the Autoregressive Distribution Lag Model (ARDL) bound and Granger Causality tests to examine long-run association and triangular causality among real GDP per capita, education expenditures, and per capita health expenditures in Pakistan from 1972 to 2009. Their findings revealed that bidirectional granger causality exists between real GDP per capita, per capita education expenditures, and per capita health expenditures in the long term.
Environmental health, economic growth, and environmental quality are several factors that affect the health of a population. As world ecological quality deteriorates, healthy living poses a severe challenge (Ahmad et al., 2021). argued that the cost of health care is affected by the increased environmental pollution caused by CO2. Therefore, governments must fund outstanding health care, as health expenditures are steadily rising. Some studies (Dogan & Seker, 2016) (Shahzad et al., 2020) (Ullah et al., 2019; Ullah et al., 2020) have used the EKC framework to investigate the relationship between environmental and economic variables such as CO2, carbon footprints, trade openness, urbanizations, democracy, financial crises, and energy sources. Moreover, Shahbaz and Sinha (2019) performed a literature review on EKC for CO2 emissions, and the findings demonstrate that EKC estimation for CO2 emissions is still unclear (Shahbaz & Sinha, 2019).
In the case of Saudi Arabia (Samargandi, 2017), demonstrates the autoregressive distributed lags (ARDL) approach, disproving the Environmental Kuznets Curve (EKC). The results show that economic growth promotes CO2 emissions, and a quicker rise in CO2 emissions relates to value addition in the industrial and service sectors. Furthermore, significant technological advancements in the manufacturing process would reduce CO2 emissions while supporting economic growth.
Numerous studies have empirically explored the relationship between economic growth and environmental degradation (CO2 emission) (Al Mamun et al., 2014; Alam et al., 2016; Asumadu-Sarkodie & Owusu, 2017; Ahmad et al., 2018; Haberl et al., 2020; Mahmood et al., 2020; Petrović-Ranđelović et al., 2020; Azam et al., 2021; Karimi et al., 2021; Khan et al., 2021). (Halicioglu, 2009) investigated the relationship between CO2 emissions and Turkey’s economic growth using the ARDL bound test cointegration approach and Granger causality (Saboori et al., 2012). used an ARDL bound technique to examine the causal association between GDP growth and CO2 emissions in Malaysia and discovered unidirectional causation to identify a considerable linkage between CO2 emissions and economic development in Singapore and Thailand in the long run, which supported the EKC hypothesis.
In contrast (Goh et al., 2017), re-examines the energy-growth link for 22 OECD nations from 1966 to 2013 using a current bootstrap autoregressive distributed lag approach. For eight countries, the empirical findings reveal degenerate situations. Only Japan has a cointegrated economy. Studies have found a few causality patterns. On the other hand (Petrović-Ranđelović et al., 2020), found a bidirectional long-run causal relationship between CO2 emissions and GDP.
Using the novel bootstrap autoregressive-distributed lag (BARDL) technique and time-series data from 1990 to 2018 (Meirun et al., 2021), found a trade-off between financial development and environmental deterioration typically occurs for an economy to succeed in growth. For a nation like Singapore, which has seen rapid expansion and is recognized for its dense population, one must investigate the role of green technology innovation in achieving economic excellence at the lowest possible environmental cost. The results showed a positive and significant relationship between green technology innovation and economic growth and a negative and meaningful relationship with carbon emissions in the long and short run.
Most previous research looked at the bilateral association between CO2 emissions, GDP, and health expenditure. Over the last decade, various scholars have followed the Environmental Kuznets Curve (EKC) between economic growth and environmental quality, with the first empirical analysis based on (Grossman & Krueger, 1995). The empirical study’s second pairing indicates that many different types of research have been conducted on the relationship between health expenditure and GDP. Previous economists investigated ways to estimate healthcare demand and distribute resources based on income elasticity. Finally, the third linked empirical investigation examined the relationship between CO2 emissions and health expenditure (Apergis et al., 2018).
In studying the relationship between economic growth and CO2 emissions (Al Mamun et al., 2014), studied the causality between CO2 emissions per capita and economic development from 1980 to 2009. Environmental Kuznets Curve (EKC) is a global phenomenon, except in high-income countries. According to empirical findings, the transformation of different economies toward a service economy has produced more pollution in high-income countries and less pollution in low- and middle-income countries.
Many studies look for a one-way causal relationship between CO2 emissions and health expenditure and discover a positive correlation. However, only a few practical studies (Abdullah et al., 2016; Wang C.-M.et al., 2019; Bilgili et al., 2021) have focused on the two-way causal relationship between CO2 emissions and health expenditure, including some relevant and supported research on the relationship between CO2 emission and health expenditures. Greenhouse gas emissions raise health care expenses considerably, according to (Yahaya et al., 2016), who examined panel data from 125 countries (Metu et al., 2018); and (Abdullah et al., 2016) confirmed these results. Furthermore, a significant positive relationship between health expenditures and CO2 emissions was established by (Blázquez-Fernández et al., 2019; Chen et al., 2019; Gündüz, 2020).
Because of the increase in infectious illnesses caused by air pollution, the influence of climate change on health has lately become a topic of discussion (Khan A. et al., 2020; Khan S. A. R. et al., 2020). The body of knowledge regarding the factors of health expenditure is consistently growing. Climate change has undeniable detrimental health consequences.
Previous studies have looked at the relationship between economic growth and pollution. EKC research reveals much about the relationship between expansion and pollution (Bilgili & Ulucak, 2018). The association between health expenditure and environmental variables has been the focus of an essential aspect of the investigation. Researchers have paid less attention to this spectrum than to the EKC literature. For example (Zaidi & Saidi, 2018), established a two-way causal relationship between health expenditure and CO2 emissions.
(Khan et al., 2016) investigated health expenditure and per capita income. They stated that economic maturity occurs later in the economic cycle when diseases are less common. Their analyses revealed a connection between CO2 emissions and per capita health expenditure. Using the ARDL cointegration model (Wang C.-M. et al., 2019), investigated the relationship between health expenditure, CO2 emissions, and GDP per capita in 18 OECD countries from 1975 to 2017. They showed bidirectional causation between health expenditure and CO2 emissions.
In Chinese provinces, the short- and long-run relationship between economic growth and CO2 emissions was examined by (Sheng et al., 2020). They find different results depending on the level of development in each area. A positive short-run causality between the two variables was found in areas at low and high levels of development; on the other hand, a negative casualty relationship is found in areas at an intermediate level of development.
(Joshua & Bekun, 2020) use yearly data from South Africa to investigate the link between energy consumption, CO2 emissions, and economic growth to support the relationship between energy and growth (Ahmad et al., 2016; Bekun et al., 2019; Hanif et al., 2019; Zhang et al., 2019; Abbasi et al., 2020; Adedoyin et al., 2020; Wasti & Zaidi, 2020). Highlight the impact of energy consumption and economic growth on CO2 emissions.
(Radmehr et al., 2021) found a unidirectional flow from renewable energy to CO2 emissions using panel spatial simultaneous equations models using the European Union data from 1995 to 2014. They discovered that non-renewable energy increases CO2 emissions in OECD nations, although renewable energy decreases CO2 emissions.
To construct a panel-corrected standard error correction model and investigate the connection between energy consumption, CO2 emissions, and GDP Growth in OECD and “Belt and Road” initiative countries (Kongkuah et al., 2021) employed Paris-Winsten regression. The study discovered that although the OECD economic development rate is higher than that of the “Belt and Road” countries, CO2 emissions are also higher.
The relationship between energy consumption, carbon dioxide emissions, and economic growth in Thailand was examined by (Adebayo & Akinsola, 2021) employing wavelet coherence analysis on time-series data from 1971 to 2018. According to the findings, variations in economic development caused adjustments in the frequency of carbon dioxide emissions. Furthermore, GDP growth is strongly connected with short-term and long-term CO2 emissions.
Based on the initial review, determining the cause of increased CO2 emissions helps policymakers reduce CO2 emissions, improve the environment, and safeguard the health of individuals in the country (Ahmed et al., 2022).
The direction for the association between health expenditure per capita, Environmental Sustainability (CO2 emissions per capita), GDP per capita, and Green Energy (Electric Power Consumption per capita) showed dissimilarities between countries. Therefore, in this study, we contribute to the existing literature by providing an empirical perspective for Saudi Arabia using Bootstrap Autoregressive Distributed Lag (BARDL) cointegration model; and comparing its results to the available literature. Our results provide evidence for policy maker to develop and implement strategies to reduce Co2 emissions. Hence, achieve the carbon emission reduction goal by 2030.
3 Data and methodology
3.1 Data
This paper applies annual time-series data from the World Bank database (https://data.worldbank.org/country/SA, 2021) over the period of 1995–2021 for Saudi Arabia. The included variables have been used to explain the relationships between health expenditure per capita (HEPC), CO2 emissions per capita (CO2), GDP per capita (GDPPC), and Green Energy is measured by (Electric Power Consumption per capita) (GEPC).
3.2 Unit root tests
In this study, the Augmented Dicky Fuller (ADF) (Dickey & Fuller, 1981), (Dickey & Fuller, 1979) and Philips-Perron (PP) unit root tests (Phillips & Perron, 1986) (Phillips, 1987) (Phillips & Perron, 1988) are used to check the stationarity (Unit Root Test) of the time series data of health expenditure per capita (HEPC), CO2 emissions per capita (CO2), GDP per capita (GDPPC), and Green Energy is measured by (Electric Power Consumption per capita) (GEPC).
3.3 The ARDL bounds test approach
The autoregressive distributed lag (ARDL) bound test model was utilized. It is also significant as it is much more efficient in a limited sample size. The ARDL technique is used to investigate the long-run causal relationship between health expenditure per capita (HEPC), CO2 emissions per capita (CO2), GDP per capita (GDPPC), and Green Energy is measured by (Electric Power Consumption per capita) (GEPC), regardless of whether the variables are I (0) or I (1). Lags of dependent and independent (explanatory) variables are present in the ARDL model, a traditional least squares regression model with lags for the relationship between the variables. It is employed to investigate the cointegration relationship among the variables (Pesaran & Shin, 1995) (Pesaran et al., 2001). Therefore, the ARDL technique with three variables is as follows (Eq. (1)):
The first difference operator is ∆the natural logarithm, and (
3.4 The bootstrap (BARDL) bound test
This study employs a specific (ARDL) econometric methodology to examine the association between health expenditure, CO2 emissions, energy consumption, and economic growth in Saudi Arabia. Since (Pesaran et al., 2001) established their (ARDL) bounds test, it has undergone several transformations that have recently improved this test using bootstrap approaches (McNown et al., 2018). The newly created bootstrap (BARDL) bound test has various advantages over the traditional (ARDL) bound test (Pesaran et al., 2001).
(McNown et al., 2018) use the bootstrap approach to evaluate the ARDL cointegration tests, concluding that appropriate power and size attributes must be considered when running these tests. Such an approach is better for small samples. Also, it does not require all series to be integrated into the same order, either I(0) or I(1), in a long-term association. Moreover (McNown et al., 2018), improved the ARDL bound test by using a lagged independent variable to support the present (F-test and t-test) proposed by (Pesaran et al., 2001). The Bootstrap (BARDL) bound test is better than the classical ARDL approach based on power, properties, size, and the cointegration status of the series in the model. According to (McNown et al., 2018), the two degenerate situations are as follows:
1) If the F-test and t-test on the lagged independent variable are significant, the t-test on the lagged dependent variable is insignificant.
2) If the F-test and t-test on the lagged dependent variables are significant, the lagged independent variables are not.
3.5 Granger causality test based on
Cointegration indicates the presence of Granger causality, at least in one direction; it does not indicate the direction of causality between variables. In this case, the direction of causality can be detected through the error correction model (ECM) derived from the long-term cointegrating vectors.
The short-term causal effect can be interpreted through the F- statistics and t-statistics, while long-run causal effects can be analyzed through the t-statistics of error-correction term that applies to ECM only. The common causal effect can be seen through F-statistics, t-statistics of independent variables and t-statistics of error correction terms.
Unidirectional causality exists from X to Y if the set of estimated coefficients of the lagged X is significantly different from zero and the location of estimated coefficients of lagged Y is not significantly different from zero. Bidirectional causality occurs from X to Y if the set of estimated coefficients of the lagged X is very different from zero and vice versa. An independent basis exists from Y(X) to X(Y) if the estimated coefficients of the lagged Y(X) are not significantly different from zero. Causal relationships can be examined through the t-statistics of the regressor. If statistically significant, causality exists. It can also be determined by performing the Wald and Granger causality tests (Granger, 1980; Pesaran & Shin, 1995). The VECM approach with three variables is specified as follows (Eq. (2)):
4 Discussion and empirical results
Table 1 shows the descriptive statistics of the per capita health expenditure, CO2 emission per capita, GDP per capita, and Energy Consumption per capita in Saudi Arabia from 1995 to 2021. The results indicate that the per capita healthcare expenditure standard deviation (SD) is the largest and the lowest GDP per capita standard deviation (SD). According to the Jarque–Bera statistics, all study variables have a normal distribution.
Unit Root test results have been reported in the following (Table 2). The Augmented Dicky Fuller ADF test (Fuller, 1976) (Dickey & Fuller, 1981), (Dickey & Fuller, 1979) and Philips- Perron (PP) unit root tests (Phillips & Perron, 1986) (Phillips, 1987) (Phillips & Perron, 1988) results indicate that health expenditure per capita (HEPC), CO2 emissions per capita (CO2), GDP per capita (GDPPC), and Green Energy is measured by Electric Power Consumption per capita (GEPC) are stationary at the level I (0) and at the first difference I (1).
The findings of (Zivot & Andrews, 2002) unit root test in the presence of a structural break is presented in Table 3. Despite catching one endogenously determined gap in the data, the (Zivot & Andrews, 2002) unit root test result showed that all the variables under investigation possess unit root. Although all the variables were stationary at the first difference, this empirical evidence suggested that the series was non-stationary at the level. Therefore, given the associated breakpoints, it may be said that the (Zivot & Andrews, 2002) unit root tests pass.
It is necessary to determine the lag order selection criteria, such as the Akaike Information Criterion (AIC), Schwarz Bayesian Criterion (SC), or Hannan-Quinn Criterion, to select the appropriate ARDL bounds testing model of the long-run equation (HQ) (Table 4). shows that lag (4) is the best lag length for evaluating cointegration in the long-run ARDL bounds model. However, according to (AIC) (HQ) and (SBIC), we found that lag order 4 is suitable for our model data and is normally distributed.
Since all variables are integrated, we use the Bootstrap ARDL bound test approach to check the cointegration in the case of Saudi Arabia. In Table 5, the results of the Bootstrap ARDL cointegration test are shown. Bootstrap ARDL show that F/Pesaran, T/dependent, and F/dependent values are more significant than Bootstrap ARDL CV (McNown et al., 2018). The calculated statistical values of F/Pesaran, T/dependent, and F/dependent in the B-ARDL test of cointegration above the 5% CV, hence supporting the conclusion that the hypothesis (H0) of no cointegration is rejected at the level of 5% significance. In the Bootstrap ARDL Cointegration Test, Degenerated Case #2 discovered in Saudi Arabia, both the F-test and F*-test are statistically significant, but the t-test is not. Consequently, the bootstrap ARDL test results for the GDP, health spending, green energy, and CO2 emissions indicate no cointegration.
To determine the causal relationship between health expenditure, GDP growth, and CO2 emissions, we conduct a Granger causality test on Saudi Arabia’s variables based on the Bootstrap ARDL cointegration test findings. Table 6 shows a unidirectional relationship between GDP per capita and health expenditure, green energy and CO2 emissions per capita. The data also show a bidirectional relationship between health expenditure and CO2 emissions and GDP per growth, this holds true for green energy and economic growth.
Furthermore, we also included the diagnostic test in determining the model’s stability. Table 7 indicates that the (Jarque & Bera, 1987) statistics’ probability value is 64%, which means that the null hypothesis cannot be ruled out. It implies that the distribution of the residuals is normal. According to the (Breusch & Pagan, 1979) LM test, which displays the efficiently computed coefficients, the model does not have a serial correlation. Due to the homoscedasticity that the (Breusch & Pagan, 1979) test revealed, the standard errors and coefficient estimations are accurate. The null hypothesis cannot be rejected since the Ramsey Reset test’s probability value is more than 10%.
5 Conclusion and policy implications
The study aims to evaluate the possible determinants of health expenditure in the case of Saudi Arabia from 1995 to 2021. The study uses income as a primary determinant, carbon emissions, and green energy. The study employed a novel time series approach for primary estimation results, i.e., Bootstrap Autoregressive Distributed Lag (BARDL). This approach is robust and provides consistent results for time series data.
The empirical outcomes first confirm stationarity results for all variables. The results confirmed that health expenditure, carbon emissions, GDP and green energy have a mixed order of integration employing the unit root test, i.e., I (0) and I (1). Due to mixed order, the study uses Bootstrap ARDL bound approach. Based on the BARDL cointegration test results, the empirical data show a bidirectional relationship between health expenditure and GDP per capita, CO2 emissions per capita and GDP per capita, health expenditure and CO2 emissions and Green Energy. The data also show that causation is two-way. Green energy plays a significant role in reducing CO2 emissions. It reduces the effects of global warming without affecting environmental health or the country’s long-term economic prosperity. In Saudi Arabia, the government aims to generate 50% of its electricity from renewables and the other half from gas by 2030. The government and policymakers are currently developing and implementing effective policies to encourage investment in sustainable and innovative technologies, such as smart carbon-free cities and green space—a step toward the Saudi 2030 goals.
However, a significant challenge is the low capacity of green energy production and its efficiency level. This will need more facilities to match the growing power demand. A field that needs more research, policies, and investment to overcome these challenges. Therefore, the study recommends that investors should be encouraged in the green energy sector to boost health expenditures in case of Saudi Arabia.
5.1 Limitations of the study
The study is limited to Saudi Arabia and is based on time series data; therefore, the outcomes from this study cannot be generalized to other economies. However, future studies may extend not only the time period but also conduct similar studies for other countries. It will not only help to generalize policy implications but shall also provide a clearer picture in this regard. Further, a more robust technique with more novel relevant variables shall be employed.
Data availability statement
The original contributions presented in the study are included in the article/supplementary material, further inquiries can be directed to the corresponding author.
Author contributions
The author confirms being the sole contributor of this work and has approved it for publication.
Conflict of interest
The author declares that the research was conducted in the absence of any commercial or financial relationships that could be construed as a potential conflict of interest.
Publisher’s note
All claims expressed in this article are solely those of the authors and do not necessarily represent those of their affiliated organizations, or those of the publisher, the editors and the reviewers. Any product that may be evaluated in this article, or claim that may be made by its manufacturer, is not guaranteed or endorsed by the publisher.
References
Abbasi, M. A., Parveen, S., Khan, S., and Kamal, M. A. (2020). Urbanization and energy consumption effects on carbon dioxide emissions: Evidence from asian-8 countries using panel data analysis. Environ. Sci. Pollut. Res. 27 (15), 18029–18043. doi:10.1007/s11356-020-08262-w
Abdel-Aal, R. E., Al-Garni, A. Z., and Al-Nassar, Y. N. (1997). Modelling and forecasting monthly electric energy consumption in eastern Saudi Arabia using abductive networks. Energy, 22(9), 911–921. doi:10.1016/S0360-5442(97)00019-4
Abdullah, H., Azam, M., and Zakariya, S. K. (2016). The impact of environmental quality on public health expenditure in Malaysia. Asia Pac. J. Adv. Bus. Soc. Stud. (APJABSS) 2 (2), 365–379.
Adebayo, T., and Akinsola, G. (2021). Investigating the causal linkage among economic growth, energy consumption and CO2 emissions in Thailand: An application of the wavelet coherence approach. Int. J. Renew. Energy Dev. 10, 17–26. doi:10.14710/ijred.2021.32233
Adedoyin, F. F., Gumede, M. I., Bekun, F. V., Etokakpan, M. U., and Balsalobre-lorente, D. (2020). Modelling coal rent, economic growth and CO2 emissions: Does regulatory quality matter in BRICS economies? Sci. Total Environ. 710, 136284. doi:10.1016/j.scitotenv.2019.136284
Ahmad, A., Zhao, Y., Shahbaz, M., Bano, S., Zhang, Z., Wang, S., et al. (2016). Carbon emissions, energy consumption and economic growth: An aggregate and disaggregate analysis of the Indian economy. Energy Policy, 96, 131–143. doi:10.1016/j.enpol.2016.05.032
Ahmad, M., Akram, W., Ikram, M., Shah, A. A., Rehman, A., Chandio, A. A., et al. (2021). Estimating dynamic interactive linkages among urban agglomeration, economic performance, carbon emissions, and health expenditures across developmental disparities. Sustain. Prod. Consum. 26, 239–255. doi:10.1016/j.spc.2020.10.006
Ahmad, M., Khan, Z., Ur Rahman, Z., and Khan, S. (2018). Does financial development asymmetrically affect CO2 emissions in China? An application of the nonlinear autoregressive distributed lag (NARDL) model. Carbon Manag. 9 (6), 631–644. doi:10.1080/17583004.2018.1529998
Ahmed, A., Uddin, G. S., and Sohag, K. (2016). Biomass energy, technological progress and the environmental Kuznets curve: Evidence from selected European countries. Biomass Bioenergy 90, 202–208. doi:10.1016/j.biombioe.2016.04.004
Ahmed, F., Ali, I., Kousar, S., and Ahmed, S. (2022). The environmental impact of industrialization and foreign direct investment: Empirical evidence from asia-pacific region. Environ. Sci. Pollut. Res. 29, 29778–29792. doi:10.1007/s11356-021-17560-w
Al Mamun, M., Sohag, K., Mia, H. A., Uddin, G., and Ozturk, I. (2014). Regional differences in the dynamic linkage between CO2 emissions, sectoral output and economic growth. Renew. Sustain. Energy Rev. 38 (C), 1–11. doi:10.1016/j.rser.2014.05.091
Al-Garni, A. Z., Zubair, S. M., and Nizami, J. S. (1994). A regression model for electric-energy-consumption forecasting in Eastern Saudi Arabia. Energy 19 (10), 1043–1049. doi:10.1016/0360-5442(94)90092-2
Alam, M. M., Murad, M. W., Noman, A. H. M., and Ozturk, I. (2016). Relationships among carbon emissions, economic growth, energy consumption and population growth: Testing Environmental Kuznets Curve hypothesis for Brazil, China, India and Indonesia. Ecol. Indic. 70, 466–479. doi:10.1016/j.ecolind.2016.06.043
Alkhathlan, K., and Javid, M. (2015). Carbon emissions and oil consumption in Saudi Arabia. Renew. Sustain. Energy Rev., 48, 105–111. doi:10.1016/j.rser.2015.03.072
Apergis, N., Gupta, R., Lau, C. K. M., and Mukherjee, Z. (2018). US state-level carbon dioxide emissions: Does it affect health care expenditure? Renew. Sustain. Energy Rev. 91, 521–530. doi:10.1016/j.rser.2018.03.035
Asumadu-Sarkodie, S., and Owusu, P. A. (2017). Carbon dioxide emissions, GDP per capita, industrialization and population: An evidence from Rwanda. Environ. Eng. Res. 22 (1), 116–124. doi:10.4491/eer.2016.097
Azam, A., Rafiq, M., Shafique, M., Zhang, H., and Yuan, J. (2021). Analyzing the effect of natural gas, nuclear energy and renewable energy on GDP and carbon emissions: A multi-variate panel data analysis. Energy, 219, 119592. doi:10.1016/j.energy.2020.119592
Begum, R. A., Sohag, K., Abdullah, S. M. S., and Jaafar, M. (2015). CO2 emissions, energy consumption, economic and population growth in Malaysia. Renew. Sustain. Energy Rev. 41, 594–601. doi:10.1016/j.rser.2014.07.205
Bekun, F. V., Emir, F., and Sarkodie, S. A. (2019). Another look at the relationship between energy consumption, carbon dioxide emissions, and economic growth in South Africa. Sci. Total Environ., 655, 759–765. doi:10.1016/j.scitotenv.2018.11.271
Bilgili, F., Kuşkaya, S., Khan, M., Awan, A., and Türker, O. (2021). The roles of economic growth and health expenditure on CO(2) emissions in selected asian countries: A quantile regression model approach. Environ. Sci. Pollut. Res. 28 (33), 44949–44972. doi:10.1007/s11356-021-13639-6
Bilgili, F., and Ulucak, R. (2018). Is there deterministic, stochastic, and/or club convergence in ecological footprint indicator among G20 countries? Environ. Sci. Pollut. Res. 25 (35), 35404–35419. doi:10.1007/s11356-018-3457-1
Blázquez-Fernández, C., Cantarero-Prieto, D., and Pascual-Sáez, M. (2019). On the nexus of air pollution and health expenditures: New empirical evidence. Gac. Sanit. 33, 389–394. doi:10.1016/j.gaceta.2018.01.006
Bokhari, F., Gai, Y., and Gottret, P. (2007). Government health expenditures and health outcomes. Health Econ. 16 (3), 257–273. doi:10.1002/hec.1157
Breusch, T. S., and Pagan, A. R. (1979). A simple test for heteroscedasticity and random coefficient variation. Econometrica 47 (5), 1287–1294. doi:10.2307/1911963
Chaabouni, S., and Saidi, K. (2017). The dynamic links between carbon dioxide (CO2) emissions, health spending and GDP growth: A case study for 51 countries. Environ. Res. 158, 137–144. doi:10.1016/j.envres.2017.05.041
Chen, L., Zhuo, Y., Xu, Z., Xu, X., and Gao, X. (2019). Is carbon dioxide (CO2) emission an important factor affecting healthcare expenditure? Evidence from China, 2005–2016. Int. J. Environ. Res. Public Health 16 (20), 3995. doi:10.3390/ijerph16203995
Dickey, D. A., and Fuller, W. A. (1979). Distribution of the estimators for autoregressive time series with a unit root. J. Am. Stat. Assoc. 74 (366), 427–431. doi:10.1080/01621459.1979.10482531
Dickey, D. A., and Fuller, W. A. (1981). Likelihood ratio statistics for autoregressive time series with a unit root. Econometrica 49 (4), 1057–1072. doi:10.2307/1912517
Dogan, E., and Seker, F. (2016). Determinants of CO2 emissions in the European union: The role of renewable and non-renewable energy. Renew. Energy 94, 429–439. doi:10.1016/j.renene.2016.03.078
Ferguson, R., Wilkinson, W., and Hill, R. (2000). Electricity use and economic development. Energy Policy, 28(13), 923–934. doi:10.1016/S0301-4215(00)00081-1
Goh, S. K., Yong, J. Y., Lau, C. C., and Tang, T. C. (2017). Bootstrap ARDL on energy-growth relationship for 22 OECD countries. Appl. Econ. Lett. 24 (20), 1464–1467. doi:10.1080/13504851.2017.1284980
Granger, C. W. J. (1980). Testing for causality: A personal viewpoint. J. Econ. Dyn. Control, 2, 329–352. doi:10.1016/0165-1889(80)90069-X
Grossman, G. M., and Krueger, A. B. (1995). Economic growth and the environment. Q. J. Econ. 110 (2), 353–377. doi:10.2307/2118443
Gündüz, M. (2020). Healthcare expenditure and carbon footprint in the USA: Evidence from hidden cointegration approach. Eur. J. Health Econ. 21 (5), 801–811. doi:10.1007/s10198-020-01174-z
Haberl, H., Wiedenhofer, D., Virág, D., Kalt, G., Plank, B., Brockway, P., et al. (2020). A systematic review of the evidence on decoupling of GDP, resource use and GHG emissions, part II: Synthesizing the insights. Environ. Res. Lett. 15 (6), 065003. doi:10.1088/1748-9326/ab842a
Halicioglu, F. (2009). An econometric study of CO2 emissions, energy consumption, income and foreign trade in Turkey. Energy Policy 37 (3), 1156–1164. doi:10.1016/j.enpol.2008.11.012
Hanif, I., Faraz Raza, S. M., Gago-de-Santos, P., and Abbas, Q. (2019). Fossil fuels, foreign direct investment, and economic growth have triggered CO2 emissions in emerging Asian economies: Some empirical evidence. Energy, 171, 493–501. doi:10.1016/j.energy.2019.01.011
Hassan, M. S., and Kalim, R. (2012). The triangular causality among education, health and economic growth: A time series analysis of Pakistan. Available at: https://data.worldbank.org/country/SA.
Jarque, C. M., and Bera, A. K. (1987). A test for normality of observations and regression residuals. Int. Stat. Review/Rev. Int. Stat. 55 (2), 163–172. doi:10.2307/1403192
Joshua, U., and Bekun, F. V. (2020). The path to achieving environmental sustainability in South Africa: The role of coal consumption, economic expansion, pollutant emission, and total natural resources rent. Environ. Sci. Pollut. Res. 27 (9), 9435–9443. doi:10.1007/s11356-019-07546-0
Karimi, M. S., Ahmad, S., Karamelikli, H., Dinç, D. T., Khan, Y. A., Sabzehei, M. T., et al. (2021). Dynamic linkages between renewable energy, carbon emissions and economic growth through nonlinear ARDL approach: Evidence from Iran. PLOS ONE 16 (7), e0253464. doi:10.1371/journal.pone.0253464
Khaleghian, P., and Gupta, M. D. (2005). Public management and the essential public health functions. World Dev., 33(7), 1083–1099. doi:10.1016/j.worlddev.2005.04.001
Khan, A., Hussain, J., Bano, S., and Chenggang, Y. (2020). The repercussions of foreign direct investment, renewable energy and health expenditure on environmental decay? An econometric analysis of B&RI countries. J. Environ. Plan. Manag. 63 (11), 1965–1986. doi:10.1080/09640568.2019.1692796
Khan, H. N., Alrasheedi, M., and Shahzada, G. (2021). A dynamic multivariate causality analysis of energy–growth nexus using ARDL approach: A Malaysian energy policy perspective. Front. Energy Res. 9. doi:10.3389/fenrg.2021.735729
Khan, S. A. R., Zaman, K., and Zhang, Y. (2016). The relationship between energy-resource depletion, climate change, health resources and the environmental Kuznets curve: Evidence from the panel of selected developed countries. Renew. Sustain. Energy Rev. 62, 468–477. doi:10.1016/j.rser.2016.04.061
Khan, S. A. R., Zhang, Y., Kumar, A., Zavadskas, E., and Streimikiene, D. (2020). Measuring the impact of renewable energy, public health expenditure, logistics, and environmental performance on sustainable economic growth. Sustain. Dev. 28 (4), 833–843. doi:10.1002/sd.2034
Kongkuah, M., Yao, H., Fongjong, B. B., and Agyemang, A. O. (2021). The role of CO(2) emissions and economic growth in energy consumption: Empirical evidence from belt and Road and OECD countries. Environ. Sci. Pollut. Res. 28 (18), 22488–22509. doi:10.1007/s11356-020-11982-8
Li, F., Chang, T., Wang, M. C., and Zhou, J. (2022). The relationship between health expenditure, CO(2) emissions, and economic growth in the BRICS countries-based on the Fourier ARDL model. Environ. Sci. Pollut. Res. 29, 10908–10927. doi:10.1007/s11356-021-17900-w
MacKinnon, J. G. (1996). Numerical distribution functions for unit root and cointegration tests. J. Appl. Econ. Chichester. Engl. 11 (6), 601–618. doi:10.1002/(SICI)1099-1255(199611)11:6<601::AID-JAE417>3.0.CO;2-T
Mahmood, H., Alkhateeb, T. T. Y., and Furqan, M. (2020). Oil sector and CO2 emissions in Saudi Arabia: Asymmetry analysis. Palgrave Commun. 6 (1), 88. doi:10.1057/s41599-020-0470-z
McNown, R., Sam, C. Y., and Goh, S. K. (2018). Bootstrapping the autoregressive distributed lag test for cointegration. Appl. Econ. 50 (13), 1509–1521. doi:10.1080/00036846.2017.1366643
Meirun, T., Mihardjo, L. W. W., Haseeb, M., Khan, S. A. R., and Jermsittiparsert, K. (2021). The dynamics effect of green technology innovation on economic growth and CO2 emission in Singapore: New evidence from bootstrap ARDL approach. Environ. Sci. Pollut. Res. 28 (4), 4184–4194. doi:10.1007/s11356-020-10760-w
Metu, A., Kalu, C., Ezenekwe, U., and Maduka, O. (2018). Does greenhouse gas emission have any relevance to per capita health expenditure? Empirical evidence from Nigeria. Afr. J. Sustain. Dev. 7 (3), 17–36.
Omri, A., Kahouli, B., Afi, H., and Kahia, M. (2022). Environmental quality, healthcare and research and development in Saudi Arabia. Environ. Sci. Pollut. Res. Int. doi:10.1007/s11356-022-20314-x
Pesaran, M. H., Shin, Y., and Smith, R. J. (2001). Bounds testing approaches to the analysis of level relationships. J. Appl. Econ. Chichester. Engl. 16 (3), 289–326. doi:10.1002/jae.616
Pesaran, M., and Shin, Y. (1995). An autoregressive distributed lag modelling approach to cointegration analysis. https://EconPapers.repec.org/RePEc:cam:camdae:9514.
Petrović-Ranđelović, M., Mitić, P., Zdravković, A., Cvetanović, D., and Cvetanović, S. (2020). Economic growth and carbon emissions: Evidence from CIVETS countries. Appl. Econ. 52 (16), 1806–1815. doi:10.1080/00036846.2019.1679343
Phillips, P. C. B., and Perron, P. (1988). Testing for a unit root in time series regression. Biometrika 75 (2), 335–346. doi:10.1093/biomet/75.2.335
Phillips, P. C. B. (1987). Time series regression with a unit root. Econometrica 55 (2), 277–301. doi:10.2307/1913237
Phillips, P., and Perron, P. (1986). Testing for a unit root in time series regression. Biometrika 75 (2), 335–346. doi:10.1093/biomet/75.2.335
Piabuo, S. M., and Tieguhong, J. C. (2017). Health expenditure and economic growth - a review of the literature and an analysis between the economic community for central African states (CEMAC) and selected African countries. Health Econ. Rev. 7 (1), 23. doi:10.1186/s13561-017-0159-1
Radmehr, R., Henneberry, S. R., and Shayanmehr, S. (2021). Renewable energy consumption, CO2 emissions, and economic growth nexus: A simultaneity spatial modeling analysis of EU countries. Struct. Change Econ. Dyn. 57, 13–27. doi:10.1016/j.strueco.2021.01.006
Rizvi, S. A. F. (2019). Health expenditures, institutional quality and economic growth. Empir. Econ. Rev. 2 (1), 63–82. doi:10.29145/eer/21/020103
Saboori, B., Sulaiman, J., and Mohd, S. (2012). Economic growth and CO2 emissions in Malaysia: A cointegration analysis of the environmental Kuznets curve. Energy Policy, 51, 184–191. doi:10.1016/j.enpol.2012.08.065
Samargandi, N. (2017). Sector value addition, technology and CO2 emissions in Saudi Arabia. Renew. Sustain. Energy Rev. 78 (C), 868–877. doi:10.1016/j.rser.2017.04.056
Sarpong, B., Nketiah-Amponsah, E., and Owoo, N. S. (2020). Health and economic growth nexus: Evidence from selected sub-Saharan African (SSA) countries. Glob. Bus. Rev. 21 (2), 328–347. doi:10.1177/0972150918778966
Shahbaz, M., and Sinha, A. (2019). Environmental Kuznets curve for CO2 emissions: A literature survey. J. Econ. Stud. 46, 106–168. doi:10.1108/jes-09-2017-0249
Shahzad, K., Jianqiu, Z., Hashim, M., Nazam, M., and Wang, L. (2020). Impact of using information and communication technology and renewable energy on health expenditure: A case study from Pakistan. Energy 204, 117956. doi:10.1016/j.energy.2020.117956
Sheng, P., Li, J., Zhai, M., and Huang, S. (2020). Coupling of economic growth and reduction in carbon emissions at the efficiency level: Evidence from China. Energy, 213, 118747. doi:10.1016/j.energy.2020.118747
Ullah, I., Ali, S., Shah, M. H., Yasim, F., Rehman, A., and Al-Ghazali, B. M. (2019). Linkages between trade, CO(2) emissions and healthcare spending in China. Int. J. Environ. Res. Public Health 16 (21), 4298. doi:10.3390/ijerph16214298
Ullah, I., Rehman, A., Khan, F. U., Shah, M. H., and Khan, F. (2020). Nexus between trade, CO(2) emissions, renewable energy, and health expenditure in Pakistan. Int. J. Health Plann. Manage. 35 (4), 818–831. doi:10.1002/hpm.2912
Wang, C.-M., Hsueh, H.-P., Li, F., and Wu, C.-F. (2019). Bootstrap ARDL on health expenditure, CO2 emissions, and GDP growth relationship for 18 OECD countries. Front. Public Health 7, 324. doi:10.3389/fpubh.2019.00324
Wang, Z., Asghar, M. M., Zaidi, S. A. H., and Wang, B. (2019). Dynamic linkages among CO(2) emissions, health expenditures, and economic growth: Empirical evidence from Pakistan. Environ. Sci. Pollut. Res. 26 (15), 15285–15299. doi:10.1007/s11356-019-04876-x
Wasti, S. K. A., and Zaidi, S. W. (2020). An empirical investigation between CO2 emission, energy consumption, trade liberalization and economic growth: A case of Kuwait. J. Build. Eng. 28, 101104. doi:10.1016/j.jobe.2019.101104
Wu, C. F., Li, F., Hsueh, H. P., Wang, C. M., Lin, M. C., and Chang, T. (2020). A dynamic relationship between environmental degradation, healthcare expenditure and economic growth in wavelet analysis: Empirical evidence from taiwan. Int. J. Environ. Res. Public Health 17 (4), 1386. doi:10.3390/ijerph17041386
Yahaya, A., Nor, N. M., Habibullah, M. S., Ghani, J. A., and Noor, Z. M. (2016). How relevant is environmental quality to per capita health expenditures? Empirical evidence from panel of developing countries. SpringerPlus 5 (1), 925–1014. doi:10.1186/s40064-016-2505-x
Yue, X. G., Liao, Y., Zheng, S., Shao, X., and Gao, J. (2021). The role of green innovation and tourism towards carbon neutrality in Thailand: Evidence from bootstrap ADRL approach. J. Environ. Manage. 292, 112778. doi:10.1016/j.jenvman.2021.112778
Zaidi, S., and Saidi, K. (2018). Environmental pollution, health expenditure and economic growth in the Sub-Saharan Africa countries: Panel ARDL approach. Sustain. Cities Soc., 41, 833–840. doi:10.1016/j.scs.2018.04.034
Zhang, X., Zhang, H., and Yuan, J. (2019). Economic growth, energy consumption, and carbon emission nexus: Fresh evidence from developing countries. Environ. Sci. Pollut. Res. 26 (25), 26367–26380. doi:10.1007/s11356-019-05878-5
Keywords: health expenditure, environmental sustainability, economic growth, green energy, bootstrap ARDL test JEL: R11, I15, P18, Q56
Citation: Ageli MM (2022) Bootstrap ARDL on health expenditure, green energy, environmental sustainability, and economic growth nexus in Saudi Arabia. Front. Environ. Sci. 10:993634. doi: 10.3389/fenvs.2022.993634
Received: 13 July 2022; Accepted: 11 August 2022;
Published: 01 September 2022.
Edited by:
Asif Razzaq, Ilma University, PakistanReviewed by:
Zeeshan Khan, Curtin University Sarawak, MalaysiaDervis Kirikkaleli, European University of Lefka, Turkey
Copyright © 2022 Ageli. This is an open-access article distributed under the terms of the Creative Commons Attribution License (CC BY). The use, distribution or reproduction in other forums is permitted, provided the original author(s) and the copyright owner(s) are credited and that the original publication in this journal is cited, in accordance with accepted academic practice. No use, distribution or reproduction is permitted which does not comply with these terms.
*Correspondence: Mohammed Moosa Ageli, mageli@ksu.edu.sa