- School of Statistics and Mathematics, Shandong University of Finance and Economics, Jinan, China
Transition risks caused by climate change are becoming an important issue in finance research. In this study, we first construct the transmission mechanism of “climate change–change in investor attention–financial stability” and analyze the impact of transition risks on Chinese financial market stability. Second, we construct a Climate Change Index (CCI). The CCI depicts changes in investors’ attention to the transition risks of climate change based on the Baidu Index. We also use the dynamic factor model (DFM) to construct a Financial Stress Index (FSI) that describes the Chinese financial market stability. The FSI can also effectively identify financial events within the sample interval. We then use the TVP-VAR model to empirically analyze the impact of the transition risks of climate change on Chinese financial market stability. We present the following results: in the short and medium terms, more financial market pressure will be caused by an increase in the CCI. In the long run, the impact of an increase in CCI on Chinese financial market pressure is uncertain. Finally, we present valuable countermeasures and suggestions from the different perspectives of investors, financial institutions, and regulators.
1 Introduction
In recent years, climate change has been considered as one of the most important factors affecting the stability of financial markets. It has received increasing attention from the international community. Francois Villeroy de Galhau, the governor of the Bank of France, noted that risks arising from climate change could jeopardize the role of central banks in price and financial stability. In severe cases, these risks can even threaten the economy of society. He named the risks caused by climate change the “green swan”. The 2019 International Monetary Fund’s Global Financial, 2005 Stability Report explored the relationship between climate change and financial stability (IMF, 2019). In 2020, the Bank for International Settlements reported that risks arising from climate change may have extreme and wide-ranging impacts. Under extreme circumstances, these risks may trigger systemic financial risks (Bolton et al., 2020).
Several studies have been conducted in various countries on the potential financial risks of climate change (Wang, 2021). The Bank of England began to assess the risk exposure of the banking and insurance markets caused by climate change. In addition, the BoE estimated the resilience of financial markets to climate risk through a scenario simulation. Under the Financial Stability Framework, the Federal Reserve announced that it would monitor and assess the vulnerability of financial markets to climate risks. At the 2021 Financial Street Forum meeting, Yi Gang, the governor of the People’s Bank of China, emphasized that “To manage climate-related transition risks, the People’s Bank of China is organizing climate risk stress tests. Financial institutions should actively assess and manage related risks through environmental risk analysis”. Therefore, assessing the impact of climate change transition risks on financial market stability will not only help financial markets pay more attention to transition risks, but also provide theoretical support for financial regulatory authorities to supervise the stability of the financial market.
In recent years, climate finance has been an important topic in finance research. Extensive studies have been conducted by institutions and scholars (Zhang et al., 2022). Carney divided climate risks into three categories: physical risks, transition risks, and liability risks (Carney, 2015). Because liability risks are often caused by physical risks, more scholars classify the impact of climate change on financial stability into two parts: physical risks and transition risks (G20 Green Finance Research Group, 2017; Chen et al., 2021). This study divides climate risks into physical and transition risks (Ma and Qin, 2021). Physical risks mainly include two categories: One refers to the influence of financial stability caused by extreme weather events such as hot waves, floods, and earthquakes induced by short-term climate change. The second category refers to the fundamental changes in environmental parameters. These changes are due to long-term changes in environmental conditions, such as average surface temperature and sea level elevation, as a result of climate change. Transition risks refer to changes in corporate operation methods and asset value levels, which in turn affect financial market stability. These changes are typically caused by green emission reduction policies, low-carbon technologies, and market preferences.
The impacts of climate change risks on financial markets are as follows:
First, physical risks change investors’ attention and behavior by impacting the operating conditions of enterprises and financial institutions, thereby influencing the financial market (Alfieri et al., 2016; Zanardo et al., 2019). Krogstrup and Oman (2019) pointed out that physical risks, such as sea-level rise, will cause real estate depreciation and increase the risk of mortgage loan portfolios. This affects investors’ capital investment and asset levels, leading to growing risks in real estate and banking markets. Bernstein et al. (2019) find that investors’ attention to global warming leads to fluctuations in property prices. Specifically, property prices, which are under the threat of the rise in sea level, are about 7% lower than those without threats. Furthermore, Wang et al. (2021) found that the level of financial development affects the marine system. For example, in China, the financial development of eastern and southern marine economic circles has a more significant positive impact on improving marine industrial efficiency.
Second, transition risks change investor attention by triggering fluctuations in asset prices, which then affect the stability of the financial market. Barro (2015) pointed out negative correlations between the optimal environmental investment level and the probability and typical size of environmental disasters. Barnett (2019) noted that the uncertainty of climate-policy implementation will change the subjective discount rate of investors and have a negative impact on oil spot prices and the value of oil firms. Furthermore, this increases the probability that oil reserves will become stranded assets. Based on a new systemic risk perspective that includes climate-policy uncertainty, Hsu et al. (2022) proved that the implementation of policies, such as environmental penalties, will lead to a decrease in corporate value as carbon emissions increase. Wang and Yi (2022) pointed out that the scale of financial industry development is positively related to emission quantity and negatively related to emission intensity. Given these circumstances, investors would change their investment decisions.
From the above research, we can conclude that climate change often affects the stability of financial markets by changing investor attention (individual and social investors). Many studies have found that online big data based on keyword searches can accurately reflect changes in investors’ attention (Costola et al., 2021). Da et al. (2015) first proposed a Google search index to represent changes in investor attention. In China, because Baidu is the largest online search engine, the Baidu Index is often used to measure changes in investors’ attention in the Chinese market. Yu and Zhang (2012) and Zhao et al. (2013) used the Baidu Index to represent investor attention and studied the impact of investor attention changes on the Chinese stock market. Wang et al. (2018) used the Baidu Index to analyze the link between investor attention and the Chinese stock index futures market. Liang et al. (2022) found that investors’ trading behavior characterized by the Baidu Index can greatly improve the prediction accuracy of China’s gold price volatility. Yang and Guo (2019) and Xiong et al. (2020) proposed that the Baidu Index can effectively reflect changes in investor attention and analyze its connection with the Chinese financial market.
At present, monitoring and measuring the volatility of a country’s financial market is of great significance for preventing the occurrence of systemic financial risks. These three methods are often used to measure fluctuations in the financial market. The first is the signalling approach, also known as the empirical approach, which is used to evaluate the possibility of another outbreak of systemic risk in countries where there has already been a financial crisis. Appropriate early warning indicators and models were selected using this approach based on the outbreak of a financial crisis (Duca and Peltonen, 2011). The second method was the model method. By establishing GARCH, CoVaR, and other models, it predicts the possibility of financial crisis outbreaks (Girardi and Ergün, 2013). The third method is the stress index method, which analyzes the financial stress value to evaluate the financial stability level. Because China has never experienced a financial crisis and the prediction results of the model method will be affected by the parameter settings, the stress index method is more suitable for measuring Chinese financial market stability. In 2011, Illing and Liu (2006) established a Canadian composite FSI to reflect the stability of the Canadian financial market. Canadian FSI includes banking, foreign exchange, bonds, and stock markets. Chinese scholars have begun extensive research on FSI. For example, Liu and Fang (2012) used the CDF-credit aggregate weighting method to construct a model system for measuring Chinese FSI in four major markets: banking, stock, foreign exchange, and insurance. Xu and Chen (2015) adopted the CRITIC empowerment method to measure Chinese financial stress from the banking, stock, real estate, and external market perspectives. Based on the principal component analysis method, Tao and Zhu (2016) constructed a comprehensive systemic FSI including stocks, bonds, currencies, foreign exchange, real estate, financial institutions, and government departments. They also identified the status and inflection points of the FSI, effectively linking macro-prudence with micro-prudence. Li and Liang (2021) used an empirical cumulative distribution function method to measure financial market stability. They used daily indicator data, which, to some extent, overcomes the lag problem of previous financial systemic risk monitoring.
It has been documented that the mechanism of transition risks caused by climate change affects financial stability. However, the role of investor attention has not been thoroughly analyzed. Moreover, the excessive adoption of static weights in the FSI synthesis process makes it difficult to effectively reflect the dynamic characteristics of the financial market. Under these circumstances, the main marginal contributions of this study are as follows. (ⅰ) Based on the comprehensive literature on climate finance and transition risks, we propose a transmission mechanism of “climate change–change in investor attention–financial stability”. We analyzed the impact of the transition risks of climate change on financial market stability. (ⅱ) From the perspective of transition risks, we use the term frequency-inverse document frequency (TF-IDF) method to analyze the text of the white paper “China’s Policies and Actions on Climate Change”. In addition, we combined the textual analysis results with the Baidu Index to construct the CCI. CCI is the core variable that describes the change in investor attention caused by transition risks. (ⅲ) We use DFM to construct the FSI, which includes six financial submarkets (stocks, bonds, insurance, banking, foreign exchange, and real estate markets). (ⅳ) The TVP-VAR model was used to quantitatively study the impact of climate change transition risks on financial market stability. The impact of stability includes two aspects: direction and extent.
2 Transmission mechanism
The transition risks of climate change may result in fluctuations in the production and sales capacity of enterprises, number of insurance claims, and scale of stranded assets. These changes affect investors’ attention and behavior, which further affects financial market stability. In this study, we construct the transmission mechanism of “climate change–change in investor attention–financial stability” and analyze the impact of transition risks caused by climate change on financial market stability (see Figure 1).
2.1 The transmission mechanism of green emission reduction policies to financial market stability
To achieve the “dual carbon” goal, the Chinese government have promulgated a series of green emission reduction policies. Chinese regulatory agencies have also promulgated green regulatory measures to guide the transformation of enterprises. These policies and measures have heterogeneous impacts on the operational levels of different enterprises. Furthermore, it affects the financial market and economy (Wang et al., 2020). For example, it promotes the development of low-carbon enterprises. However, the production and operation of carbon-intensive enterprises are under significant pressure. As one of the important reference factors in investors’ investment processes, the rate of return has a direct impact on investor behavior. Its fluctuation will result in a change in investors’ behavior in financial markets, such as stocks, bonds, insurance, and real estate, increasing pressure on the financial system. Unlike traditional policies, which only consider economic development, the green emission reduction policy also considers other aspects, such as sustainable development. Hence, implementing green policies has a more complicated impact on the financial market.
First, under the strong support of green emission reduction policies, new energy-related industries are key to future investments. Low-carbon innovation and digital transformation are general trends in the industrial field. Investors will obtain higher yields by investing in green industries such as new energy. Thus, they buy a large number of stocks in related industries, such as power equipment and new energy in the stock market, resulting in an increase in their stock prices. According to statistics, in 2011, the market value of power equipment and new energy industry stocks rose by as much as 92.22%, ranking first in the CITIC industry. In addition, the increasing number of carbon taxes and implementation of carbon trading policies will also change investors’ preferences. Under these new policies, investors would prefer stocks and bonds issued by new energy companies, which affects the stability of the financial market.
Second, in the process of implementing green emission reduction policies, green bonds have attracted the attention from a large number of many investors (Bachelet et al., 2019; Gianfrate and Peri, 2019; Flammer, 2020). In 2017, the China Securities Regulatory Commission issued the “Guiding Opinions on Supporting the Development of Green Bonds”. In 2021, the People’s Bank of China, the National Development and Reform Commission, and the China Securities Regulatory Commission jointly promulgated the “Catalogue of Green Bond Support Projects (2021 Edition)". It further clarifies the criteria for the identification of green bonds in China and strengthens the supervision of green bonds. It has won a respectable reputation. In addition, it lays a strong foundation for the standardized development of green bonds in the future. Moreover, more investors are attracted to investing in green bonds. According to statistics from the People’s Bank of China, the scale of the Chinese green bond market has expanded rapidly in recent years. By the end of 2020, the stock of green bonds reached 813.2 billion yuan, ranking second in the world.
Third, in the process of promoting the green emission reduction policy, as an important part of the financial market, the investment business in the insurance market will inevitably be affected by transition risks. The responsibility report issued by the Insurance Association of China emphasized that insurance institutions should actively develop liability insurance products in the field of environmental protection. Insurance institutions should also increase their investment and financing support for energy conservation, environmental protection, and new energy-related industries. Among them, environmental pollution liability insurance is the most relevant to a low-carbon transition. By paying premiums to the insurer, insured enterprises use environmental liability insurance to transfer the risk to the insurance company after a pollution accident. This leads to an increase in underwriting risk in the insurance market. Under the guidance of green emission reduction policies, insurance institutions are gradually shifting their investment focus from the traditional energy fields to the energy conservation, environmental protection, and cleaner production fields. There will be more investment focused on low-carbon sectors. Under the supervision of regulators and the media, if insurance companies still focus on high-carbon areas in the investment process, they will suffer reputation damage. Therefore, investment is reduced, increasing the volatility of the insurance market.
Finally, as traditional industries with high energy consumption and high emissions, the steel and cement industries will be subject to stricter control during the low-carbon transition. The Ministry of Ecology and Environment of the People’s Republic of China issued the “Guiding Opinions on Strengthening the Prevention and Control of Eco-environmental Sources for High Energy Consumption and High Emission Construction Projects”. The study emphasizes that to achieve carbon peak and carbon neutrality goals, it is necessary to change the development model of cement and the other “two high” industries. This adjustment increases the cost of the cement and other building materials. This will not only affect its profits, but also squeeze the profits of the closely related real estate industry. Moreover, this leads to less investment in the real estate sector by investors and adversely affects home sales. Thus, the real estate market is under great pressure.
2.2 The transmission mechanism of low-carbon technologies to financial market stability
Transition risks arising from the transition to low-carbon technologies can also impact investor behavior, thereby affecting financial market stability. Low-carbon technologies are currently divided into three main categories. The first is carbon reduction technology. It mainly refers to the reduction in the carbon emission level of high-energy-consuming and high-emission industries through cogeneration and waste heat utilization. The second is a carbon-free technology. It refers to the development of clean energy, such as wind energy, solar energy, biomass energy, etc., and would help in achieving the goal of zero carbon emissions. The third is decarbonization technology, which mainly refers to carbon capture and storage (CCS) technology. First, CCS separates carbon dioxide from industrial emissions. Then, it stores and transports carbon dioxide to places isolated from the atmosphere (such as the seabed and under-ground). During this process, the goal of decarbonization was achieved.
Carbon reduction technologies are mainly used in high-carbon enterprises in the low-carbon transition process. Consider the power industry as an example: The market share of Chinese traditional thermal power installed capacity has declined annually. The power industry is actively exploring and developing new thermal power technologies, including flue gas purification and coal conversion. During this process, successfully transformed enterprises continue to attract investment from investors and obtain funds for further research and development. They can also use funds to repay their bank loans. Enterprises that experience failure face the risk of a large-scale withdrawal of capital by investors. This makes it more difficult for them to repay their bank loans. The bank market liquidity risk increases (Delis et al., 2019).
Carbon-free technologies represented by energy vehicles are becoming increasingly popular in the automotive industry. Taking Tesla as an example, thanks to the transformation dividend brought about by technological changes, the global delivery of energy vehicles produced by the company continues to rise. Adam Jonas, an analyst at Morgan Stanley, expects Tesla EVs to account for 30% of the global EV sales market by 2030. Optimistic estimates of Tesla’s future development will attract more investors, which is conducive to further increasing the market value of Tesla’s stock. Moreover, thanks to the development of carbon-free technology, Tesla Inc. Earned $1.58 billion in revenue in 2020 alone by selling its carbon emissions shares in the carbon trading market. This revenue is twice as much as its annual net profit ($721 million). Tesla will further invest in the field of new energy research and development. This contributes to the fact that it attracts more investors with environmental, social, and governance (ESG) concepts in the stock market and improves its competitiveness. The credit rating of Tesla’s corporate bonds is conducive to increasing the yield of corporate bonds to attract more investment. Competitiveness in the bond market has also been enhanced.
To date, CCS is the representative decarbonization technology. This is considered the most effective way to slow global warming in the future. This huge potential has attracted significant investments. Microsoft announced a US$ 1 billion investment in CCS in 2020. The annual global CCS financing amount is expected to increase by more than 118% by 2021 over 2020. This significantly enhances the market attractiveness of listed CCS-related companies. Meanwhile, it stimulates investors to invest heavily in the stocks of such companies. Finally, the volatility of financial markets increases.
2.3 The transmission mechanism of market preference to financial market stability
First, in the low-carbon transition process, green and low-carbon assets are increasingly favored by the market. Carbon-intensive assets are sold on a large scale. For a long time, the impact of the low-carbon transition process on traditional markets has not been reflected in market valuation. Therefore, fossil fuels and other high-carbon industries have overinvested. With the continuous advancement of the low-carbon transition, market preferences have gradually changed. A change in market preferences means that consumers become more conscious of products related to concepts such as green, low-carbon, and environmental protection. It is becoming increasingly difficult for high-carbon products to attract consumers. This leads to changes in low-carbon and carbon-intensive assets. Specifically, a large number of high-carbon and stranded assets depreciated sharply. The HSBC report points out that investors play an important role in the development of high-carbon industries. When pressure on high-carbon industries increases, investors can promptly adjust the allocation of funds. Thus, they can invest in green products with greater development prospects and market potential. In the development field, changes in the direction of capital investment lead to fluctuations in the prices of stocks and bonds in related fields. Price volatility increases financial market stress. However, the global distribution of fossil fuels is uneven. The oil reserves in the Middle East are higher than those in the Asia-Pacific region, so changes in the price of stranded assets will have different impacts in these regions. When investors sell stranded assets on a large scale, they trigger fluctuations in the economic levels of the different countries. Consequently, the global trade pattern will change, and the volatility of the foreign exchange market will be aggravated.
With the continuous promotion of ESG investment, the market prefers environmentally friendly and sustainable brands. Therefore, companies with excellent ESG performance are more likely to attract investment (Görgen et al., 2020). This will lead investors to invest funds in more environmentally friendly green brands, improve their credit ratings, and help them obtain bank loans. Simultaneously, by incorporating ESG indicators into investment reference decision-making factors, investors can comprehensively construct investment strategies to avoid risks. By investing funds in environmentally friendly and sustainable development enterprises, we can obtain more stable income. This is beneficial for the sustainable development of the financial market.
Finally, market preferences are gradually shifting from traditional to low-carbon consumer goods. For example, investors prefer plant-based meat and milk. Changes in consumer market preferences can improve the profitability of low-carbon consumer-goods companies. With improvements in profitability, investors will enhance their confidence in the development of this kind of company. They make additional investments in these companies. In the stock market, many stocks of such companies are bought, which raises the stock price and exacerbates stock market volatility.
Through the above analysis, we find that the transition risks of climate change directly lead to fluctuations in the stock, bond, insurance, banking, foreign exchange, and real estate markets through changes in investor attention and behavior. Investors’ behavior is effective through a complex relationship network between financial markets. Hence, the transition risks of climate change will affect the stability of the entire financial system and even affect the entire real economy.
3 Materials and methods
3.1 Data
In this study, we analyzed the white paper “China’s Policies and Actions on Climate Change” to select the transition risk of climate change terms. Then we used Python to obtain the Baidu Index of PC and mobile terminals from January 2011 to December 2021 through a web crawler.
In addition, the choice of the basic financial indicator system is of great significance in accurately measuring the stability of the Chinese financial market. Based on the transmission mechanism of transition risks affecting the financial market, we also referred to Tao and Zhu (2016), Wanyan and Suo (2018), etc. To construct the financial stress indicator system (see Table 1). The sample interval was from January 2011 to December 2021, with monthly frequency. The main data sources are the Wind Financial Database and CEIC China Economic Database.
As presented in the above table, we finally selected six financial sub-markets of stocks, bonds, insurance, banking, foreign exchange, and real estate. There are a total of 17 financial indicators to measure the pressure of the Chinese financial market.
3.2 TF-IDF
Referring to Engle et al. (2020), this paper uses the TF-IDF textual analysis method to identify keywords related to transition risks of climate change.
The TF-IDF expresses the white paper content as a vector composed of n weighted terms and measures its importance by calculating the TF-IDF value of each term (Huang et al., 2011). The TF-IDF is formulated as:
where
3.3 Dynamic factor model
This paper uses DFM to construct the FSI. The DFM can be written in state-space representation as:
where
A finite order
is usually referred to as the “common component”, which reduces to
Further, formulas 2, 3 can be recast into a static state space by redefining the state vector. Let
when
with
3.4 TVP-VAR model
Since the TVP-VAR model does not have the assumption of homoscedasticity and has the characteristics of time-varying parameters, it can better capture the relationship and characteristics of economic variables in different eras. Therefore, this paper chooses the TVP-VAR model to analyze the impact of changes in investors’ attention to climate change on FSI. The TVP-VAR model defined as:
where the coefficient
We set
where
4 Results
4.1 Analysis of the climate change index
We use the TF-IDF textual analysis method to analyze the white paper “China’s Policies and Actions on Climate Change”. Referring to Engle et al. (2020), we selected terms with TF-IDF value exceeding 0.05 (see Table 2).
At the same time, referring to the climate-change-related vocabulary updated in 2021 in the Oxford English Dictionary, we added two words “global warming” and “greenhouse effect”. Finally, 11 climate-related words were identified.
In addition, extensive literature indicates that network big data can effectively measure investor attention and other index variables (Tian et al., 2020). In China, the market share of Baidu is greater than 90%. The Baidu Index, which is obtained by weighting and summing the search frequencies of keywords, is based on the massive search data of Baidu. This fully reflects the attention and behavior of Chinese investors. Therefore, we use the Baidu Index to characterize investor attention. To make the data smoother, we refer to Zhang et al. (2014) to deal with the Baidu Index as follows:
where
The above figure shows that from January 2011 to March 2019, the value of CCI fluctuated between 8.056 and 9.036. In April 2019, the CCI rose rapidly to 10.418 and then gradually declined. In September, it returned to around 9.066. The CCI experienced small fluctuations and began to gradually increase in September 2020. This indicates that the risks posed by climate change in China are receiving increasing attention.
Chinese investors’ attention to climate change was in its infancy until 2019. Financial investors are not yet fully aware of the pressure of climate change on the financial market; hence, the CCI was low. The reason for the rapid rise in the CCI in 2019 was that the Baidu Index for the word “green” increased significantly. This may be related to the UN Climate Summit and World Horticultural Exposition. In 2019, the UN Climate Summit, which highlighted that “green, low-carbon, environmental protection and energy saving have become the main theme of the times” stimulated domestic investors to pay attention to climate finance, especially the green industry. The Expo theme was “Live Green, Live Better”. This event drew people’s attention to the field of green environmental protection. The “green"-related Baidu Index during the expo increased rapidly. Later, at the general debate of the 75th United Nations General Assembly, China put forward the goal of “carbon peaking and carbon neutrality” for the first time. Considerable domestic attention has been paid to the field of climate change through this debate. CCI gradually increased simultaneously.
4.2 Analysis of the financial stress index
This paper uses the DFM to construct the Chinese FSI. Referring to Doz et al. (2011), we know that the Two-step estimation method and QML-EM estimation method can estimate DFM well. Therefore, this paper calculates the FSI using Gretl software based on these two estimation methods (see Figure 3).
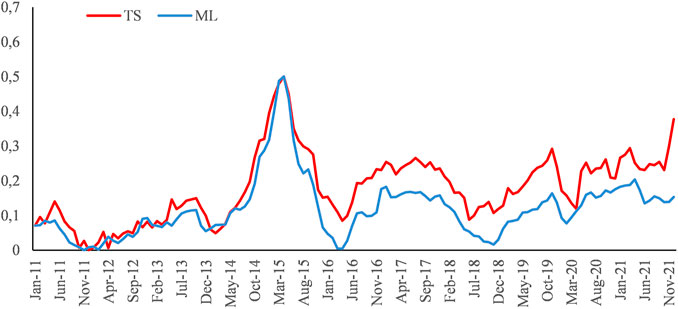
FIGURE 3. FSI trends. Note: TS represents the calculation result of the Two-step estimation method and ML represents the calculation result of the QML-EM estimation method.
From the above figure, we conclude the following. (ⅰ) In 2012, China’s financial market pressure increased due to the European debt crisis and the Chinese domestic local debt crisis. The FSI showed an upward trend. Subsequently, the FSI gradually climbed along with the diminishing impact of the European sovereign debt crisis, and the implementation of Chinese stability maintenance began to take effect. (ⅱ) With the advent of the “bull market,” the stress index of the Chinese stock market has gradually increased. The risk gradually spreads to other financial markets through the balance sheet and other channels. The FSI continued to rise until the Chinese stock price bubble burst in 2015, when the FSI began to decline. (ⅲ) In 2016, the Chinese A-share market experienced a circuit breaker and liquidity risks caused market panic. At the same time, housing prices in some cities have skyrocketed. This has caused the real estate market to enter a state of high pressure. The risks continued to spread among various markets, and the FSI increased again. (ⅳ) To promote healthy development of the financial market, China’s financial sector issued more than 20 important regulatory documents in 2017. Therefore, 2017 was called “the strictest year in history” by the financial industry. With the gradual implementation of regulatory policies, China’s FSI has gradually fallen. (ⅴ) In 2018, trade friction between China and the United States continued to intensify. On June 15, the United States issued a list of additional tariffs. China immediately counteracted this, and the international trade market became turbulent. Foreign exchange, banking, and other market risks immediately increased, resulting in a further increase in Chinese systemic risks. (ⅵ) In 2020, under the impact of the COVID-19 pandemic, both financial and real sectors suffered a huge blow. The FSI rose again.
The analysis above shows that the FSI established in this study can effectively identify financial market stress events in the region. It can also help monitor and evaluate the operations of the Chinese financial market.
4.3 Analysis of the TVP-VAR model
4.3.1 TVP-VAR model establishment and simulation
According to the analysis of the Chinese FSI, the FSI shows different fluctuation trends at different stages. The TVP-VAR model abandons the assumption of homoscedasticity, so it can well-reflect the change characteristics of the research variables at different times. This paper refers to the TVP-VAR model established by Nakajima to analyze the impact of climate change on financial stability (Nakajima, 2011). Since the climate will not only affect the stability of financial market, but also will cause the economic fluctuation in the real sector, the interaction between the real sector and financial market will lead to further expansion of the risk (Khanna et al., 2022). Therefore, we also introduce the industrial added value and consumer price index reflecting the macroeconomic performance into the TVP-VAR model.
We set the number of iterative simulations of the MCMC algorithm to be 10,000. The initial 1,000 samples are discarded. According to the LR information criterion, the optimal lag order was selected as 3 periods.
Table 3 shows the parameter estimation results of the TVP-VAR model. The Geweke test values are all less than 1.96, indicating that the acceptance parameters converge to the null hypothesis of the posterior distribution. The null factor values are all less than 62, indicating that the sampling is effective.
From the sample autocorrelation diagram in the first row of Figure 4, we know that the sample autocorrelation coefficient drops rapidly and fluctuates slightly around 0. This indicates that the sample data do not have autocorrelation. According to the sample path diagram in the second row, the stable sample path indicates that the sample data extracted are stable. From the posterior density map in the third row, the number of samples extracted by the MCMC algorithm is sufficient. Therefore, the MCMC algorithm draws enough samples, and the simulated estimation results are effective and robust.
4.3.2 Time-point impulse response analysis
According to the “climate change–change in investor attention–financial stability” transmission mechanism, the change in investors’ attention to transition risks can cause fluctuations in the Chinese financial market. Based on CCI and FSI, we further investigate the dynamic effects of the transition risks of climate change on financial market stability.
We select two representative time points to study the impact of climate change on the stability of the Chinese financial market. Time point 1 was the United Nations Climate Change Conference, held in December 2019. The participating countries emphasized that “China plays an important role in implementing the Paris Agreement and solving the world’s climate problems”. Time point 2 was the Leaders’ Climate Summit, held in April 2021, at which China once again emphasized that “China will achieve carbon peaking by 2030 and carbon neutrality by 2060". The convening of these two conferences is of great significance for China’s development of climate finance. Both these time points have been at a high position in the CCI in recent years. Figure 5 shows the changing trend of the FSI to a positive shock of one unit of CCI.
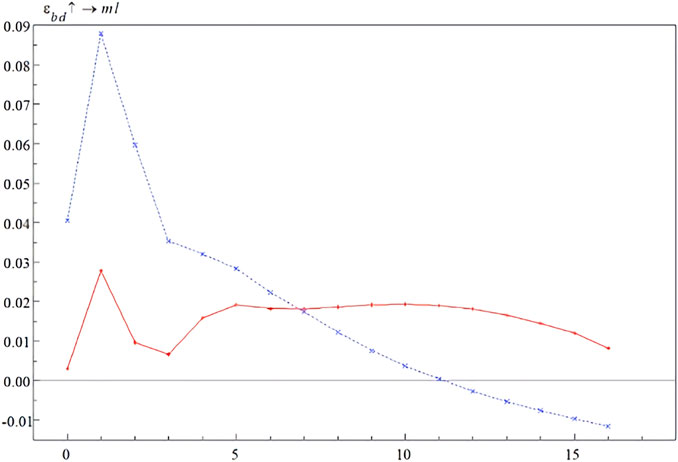
FIGURE 5. Time-point impulse response diagram. Note: The red line represents time point 1 (December 2019) and the blue line represents time point 2 (April 2021).
The analysis in Figure 5 shows the following: (ⅰ) The impact of the CCI at time point 1 on the FSI is positive in the short, medium, and long terms. This indicates that at this time, the increased attention of investors in the field of climate change will increase pressure on the financial market. In addition, the short-term response value of the FSI to the CCI was significantly larger than that in the medium- and long-term. This may be triggered by different stages of development in Chinese climate finance. In the early stage of development, investors lacked experience in dealing with transition risks, adversely affecting the financial market continuously. (ⅱ) At time point 2, in the short and medium terms, the impact of the CCI on the FSI was a positive response in the first 10 periods. Except for an upward trend at the beginning of the period, a downward trend was observed thereafter. In the long run, the impact of CCI on the FSI becomes a negative response within the sample interval and gradually increases. This shows that the spillover effect of climate change on financial market stability has obvious time-varying characteristics, and the impact differs in the short, medium, and long terms. This could be because, at the climate summit, many countries once again emphasized the importance of climate change. They stated that “climate change is a global challenge faced by the international community. However, this requires a global perspective. A new round of actions should be taken quickly to increase energy conservation and emission reduction, and vigorously develop clean energy”. This has a significant impact on both traditional high-emission industries and green and sustainable development industries, resulting in a rapid increase in the FSI. However, the growth rate of Chinese financial market pressure gradually slowed down based on the experience of investors and adjustments by financial regulatory authorities. (ⅲ) Comparing the impulse response trends, the fluctuation range of the impulse response graph at time point 2 was significantly higher than that at time 1 in the short term. However, the shock from time point 1 in the long term causes the FSI to continue to rise. The shock at time point 2 became negative after period 10. From this analysis, we can infer that Chinese financial market investors are gradually paying more attention to the transition risks caused by climate change. Investors can adjust their investment strategies on time when facing the pressure brought about by climate change on the financial market. For example, investors can withdraw their investments in high-emission industries and invest their funds in green development fields. These actions can help handle the financial risks brought about by climate change. They will also be conducive to maintaining the stability of China’s financial system and promoting the long-term development of China’s green finance.
5 Conclusions and recommendations
5.1 Conclusion
Based on the transmission mechanism of “climate change–change in investor attention–financial stability,” we theoretically analyze the change in investor attention caused by the transition risks of climate change and the impact of the change on financial market stability. At the empirical level, the TF-IDF method was used to analyze the text of Chinese white papers to determine terms related to the transition risks of climate change. We also crawled the Baidu Index from 2011 to 2021 to construct the CCI. This was used to measure the degree of change in investor attention during climate changes. Simultaneously, DFM was used to construct an FSI with 17 financial indicators in the stock, bond, insurance, banking, foreign exchange, and real estate markets. The TVP-VAR model was then used to quantitatively analyze the impact of climate change transition risks on Chinese financial market stability. The empirical results show that the FSI can effectively identify financial stress events during the sample period, which is helpful in monitoring the volatility of the Chinese financial market. Furthermore, the transition risks caused by climate change have a significant impact on financial market pressure, and the impact varies at different stages of climate finance development. In the early stage of climate finance development, due to the lack of investment experience and regulatory capacity, an increase in the CCI will lead to a continuous increase in pressure on the Chinese financial market. As more attention is paid to climate risks, the rise of the CCI will increase the pressure on the Chinese financial market in the short and medium terms, but will help reduce pressure on the Chinese financial market in the long run.
5.2 Recommendations
Based on theoretical and empirical analysis, we propose the following recommendations.
Individual and institutional investors should improve their ability to analyze and deal with the financial risks caused by climate change. Investors should pay close attention to information related to climate finance, which is disclosed by various environmental protection organizations and financial markets both at home and abroad. They should also continuously improve their ability to analyze and distinguish climate-related financial risks and reasonably analyze the impact of climate change on the stability of various financial submarkets. Simultaneously, investors should regard climate change as an important reference factor in investment decisions. They should try their best to reduce the negative impact of financial risks caused by climate change on investment returns continuously. These actions can gradually improve the returns and robustness of investment portfolios.
Furthermore, different financial institutions should be encouraged to incorporate climate change into their risk-management frameworks. First, financial institutions should incorporate climate risk analysis into their risk prevention and early warning mechanisms. Simultaneously, attention should be paid to the latest developments in climate change, and timely measures should be taken to respond to their impact on financial market volatility. In addition, they should actively promote quantitative analysis of the impact of climate risk on financial stability. The empirical measurement model should be comprehensively explored by comparing various evaluation methods suitable for the development status of climate finance in China. Based on the empirical results, appropriate climate finance-related products should be developed to reduce the adverse impacts of climate change on China.
Finally, regulators should accelerate the top-level design of climate investment and financing to systematically promote the development of climate finance in China. They can establish reasonable internal supervision and guidance mechanisms to encourage large enterprises and financial institutions to promptly disclose fluctuations in their business conditions caused by climate change. Moreover, differentiated regulatory policies should be implemented in different financial markets. Investors’ investment and financing behaviors should be gradually standardized during the different stages of climate finance development. It is very important to strictly adhere to the bottom line that systemic risk does not occur. Make rational use of international platforms such as the “Belt and Road” initiative and the green financial network. Economic and financial cooperation with countries around the world in the fields of climate protection and green development should also be strengthened. Through these efforts, we will actively fill gaps in the field of climate finance in China.
Data availability statement
The raw data supporting the conclusions of this article will be made available by the authors, without undue reservation.
Author contributions
QA was responsible for conceptualization, writing-original draft preparation, review, and editing. LZ was responsible for conceptualization, methodology, software, validation, formal analysis, resources, writing-original draft preparation, review, and editing. QL was responsible for methodology and software. CL was responsible for conceptualization, investigation and data curation.
Funding
This research was funded by the National Social Science Fund of China Program (General Project 21BTJ072), and was funded by the Shandong Soft Science Research Program (Major Project 2019RZB14008).
Conflict of interest
The authors declare that the research was conducted in the absence of any commercial or financial relationships that could be construed as a potential conflict of interest.
Publisher’s note
All claims expressed in this article are solely those of the authors and do not necessarily represent those of their affiliated organizations, or those of the publisher, the editors and the reviewers. Any product that may be evaluated in this article, or claim that may be made by its manufacturer, is not guaranteed or endorsed by the publisher.
References
Alfieri, L., Feyen, L., Salamon, P., Thielen, J., Bianchi, A., Dottori, F., et al. (2016). Modelling the socio-economic impact of river floods in Europe. Nat. Hazards Earth Syst. Sci. 16 (6), 1401–1411. doi:10.5194/nhess-16-1401-2016
Bachelet, M. J., Becchetti, L., and Manfredonia, S. (2019). The green bonds premium puzzle: The role of issuer characteristics and third-party verification. Sustainability 11 (4), 1098. doi:10.3390/su11041098
Barnett, M. D. (2019). A run on oil: Climate policy, stranded assets, and asset prices. University of Chicago. Doctoral dissertation.
Barro, R. J. (2015). Environmental protection, rare disasters and discount rates. Economica 82 (325), 1–23. doi:10.1111/ecca.12117
Bernstein, A., Gustafson, M. T., and Lewis, R. (2019). Disaster on the horizon: The price effect of sea level rise. J. financial Econ. 134 (2), 253–272. doi:10.1016/j.jfineco.2019.03.013
Bolton, P., Despress, M., da Silva, L. A. P., Samama, F., and Svartzman, R. (2020). The green swan—central banking and financial stability in the age of climate change. Basel: Bank for International Settlements.
Carney, M. (2015). Breaking the tragedy of the horizon–climate change and financial stability. Speech given A. T. Lloyd’s Lond. 29, 220–230.
Chen, G., Guo, J., and Zhao, X. (2021). The settlement of "carbon emissions trading" in RMB-explorations in China's pathway of "overtaking on the curve" in finance. Econ. Perspect. 131 (08), 131–174. doi:10.1007/978-981-33-6092-1_5
Costola, M., Iacopini, M., and Santagiustina, C. R. (2021). Google search volumes and the financial markets during the COVID-19 outbreak. Finance Res. Lett. 42, 101884. doi:10.1016/j.frl.2020.101884
Da, Z., Engelberg, J., and Gao, P. (2015). The sum of all FEARS investor sentiment and asset prices. Rev. Financ. Stud. 28 (1), 1–32. doi:10.1093/rfs/hhu072
Delis, M. D., De Greiff, K., and Ongena, S. (2019). Being stranded with fossil fuel reserves? Climate policy risk and the pricing of bank loans. Climate policy risk and the pricing of bank loans (september 10, 2019). EBRD Working Paper.
Doz, C., Giannone, D., and Reichlin, L. (2011). A two-step estimator for large approximate dynamic factor models based on Kalman filtering. J. Econ. 164 (1), 188–205. doi:10.1016/j.jeconom.2011.02.012
Duca, M. L., and Peltonen, T. A. (2011). Macro-financial vulnerabilities and future financial stress-Assessing systemic risks and predicting systemic events. Report.
Engle, R. F., Giglio, S., Kelly, B., Lee, H., and Stroebel, J. (2020). Hedging climate change news. Rev. Financial Stud. 33 (3), 1184–1216. doi:10.1093/rfs/hhz072
Flammer, C. (2020). Green bonds: Effectiveness and implications for public policy. Environ. Energy Policy Econ. 1 (1), 95–128. doi:10.1086/706794
Gianfrate, G., and Peri, M. (2019). The green advantage: Exploring the convenience of issuing green bonds. J. Clean. Prod. 219, 127–135. doi:10.1016/j.jclepro.2019.02.022
Girardi, G., and Tolga Ergün, A. T. (2013). Systemic risk measurement: Multivariate GARCH estimation of CoVaR. J. Bank. Finance 37 (8), 3169–3180. doi:10.1016/j.jbankfin.2013.02.027
Görgen, M., Jacob, A., Nerlinger, M., Riordan, R., Rohleder, M., and Wilkens, M. (2020). Carbon risk. Available at: https://papers.ssrn.com.
Hsu, P. H., Li, K., and Tsou, C. Y. (2022). The pollution premium. J. Finance. doi:10.2139/ssrn.3578215
Huang, C. H., Yin, J., and Hou, F. (2011). A text similarity measurement combining word semantic information with TF-IDF method. Chin. J. Comput. 34 (5), 856–864. doi:10.3724/sp.j.1016.2011.00856
Illing, M., and Liu, Y. (2006). Measuring financial stress in a developed country: An application to Canada. J. Financial Stab. 2 (3), 243–265. doi:10.1016/j.jfs.2006.06.002
Khanna, M., Gusmerotti, N. M., and Frey, M. (2022). The relevance of the circular economy for climate change: An exploration through the theory of change approach. Sustainability 14 (7), 3991. doi:10.3390/su14073991
Krogstrup, S., and Oman, W. (2019). Macroeconomic and financial policies for climate change mitigation: A review of the literature. Report.
Li, M., and Liang, S. (2021). Monitoring systemic financial risks: Construction and state identification of China’s financial market stress index. Financial Res. 6, 21–38.
Liang, C., Wei, Y., Ma, F., and Li, W. (2022). A study on the impact of investor attention on Chinese gold volatility. Syst. Eng. – Theory & Pract. 42 (02), 320–332.
Liu, X., and Fang, L. (2012). Construction and effectiveness test of financial stress index—empirical analysis based on China data. J. Industrial Eng. Eng. Manag. 26 (03), 1–6. doi:10.13587/j.cnki.jieem.2012.03.010
Ma, Z., and Qin, F. (2021). Research on the transmission mechanism of climate change affecting financial stability. J. Financial Dev. Res. 02, 35–43. doi:10.19647/j.cnki.37-1462/f.2021.02.005
Nakajima, J. (2011). Time-varying parameter VAR model with stochastic volatility: An overview of methodology and empirical applications. Report.
Tian, J., Wang, D., and Kong, X. (2020). Investors’ attention and heterogeneity of concept stocks: Influence mechanism and multidimensional measure—matching test based on behavior big data. J. Appl. Statistics Manag. 39 (02), 354–367. doi:10.13860/j.cnki.sltj.20191021-002
Wang, S., Lu, B., and Yin, K. (2021). Financial development, productivity, and high-quality development of the marine economy. Mar. Policy 130, 104553. doi:10.1016/j.marpol.2021.104553
Wang, S., and Yi, X. (2022). Can the financial industry ‘Anchor’Carbon emission reductions? —the mediating and moderating effects of the technology market. Energy & Environ., 0958305X211061810. doi:10.1177/0958305X211061810
Wang, S., Zhao, D., and Chen, H. (2020). A spatial analysis of corruption, misallocation, and efficiency. Environ. Sci. Pollut. Res. 27 (29), 36845–36856. doi:10.1007/s11356-020-09707-y
Wang, X. (2021). Managing the financial risks relating with climate change prudentially. China Finance 04, 41–42. doi:10.1142/s259172932197004x
Wang, X., Ye, Q., Zhao, F., and Kou, Y. (2018). Investor sentiment and the Chinese index futures market: Evidence from the internet search. J. Futur. Mark. 38 (4), 468–477. doi:10.1002/fut.21893
Wanyan, R., and Suo, L. (2018). China’s perspective on insurers and systemic risks: Theories and evidences. Insur. Stud. 11, 3–16. doi:10.13497/j.cnki.is.2018.11.001
Wei, L., Ma, Y., and Yang, B. (2022). Corporate solvency, economic uncertainty and monetary policy an empirical study based on TVP-VAR model. Financ. Dev. Rev. 02, 1–16. doi:10.19895/j.cnki.fdr.2022.02.007
Xiong, X., Xu, K., and Shen, D. (2020). Investor sentiment and futures market functions: Evidence from CSI300 index futures market. Syst. Eng. – Theory & Pract. 40 (09), 2252–2268.
Xu, D., and Chen, S. (2015). Research on systematic financial risk measurement based on financial stress index. Econ. Perspect. 04, 69–78.
Yang, T., and Guo, M. (2019). Investor attention and the stock market: A new perspective on PM2.5 concept stocks. J. Financial Res. 467 (5), 190–206.
Yu, Q. J., and Zhang, B. (2012). Limited attention and stock performance: An empirical study using baidu index as the proxy for investor attention. J. Financial Res. 386, 152–165.
Zanardo, S., Nicotina, L., Hilberts, A. G., and Jewson, S. P. (2019). Modulation of economic losses from European floods by the north atlantic oscillation. Geophys. Res. Lett. 46 (5), 2563–2572. doi:10.1029/2019gl081956
Zhang, J., Liao, W., and Zhang, R. (2014). The effect of ordinary investors’ attention on volume and price of stock market: Empirical evidence based on Baidu index. Account. Res. 8, 52–59.
Zhang, S., Lu, L., Zhang, X., and Wang, H. (2022). Climate risk in financial system: Assessment, pricing, and management. Chin. Rev. Financial Stud. 14 (01), 99–120+124.
Keywords: climate change, the Baidu Index, financial stress index, dynamic factor model, TVP-VAR model
Citation: An Q, Zheng L, Li Q and Lin C (2022) Impact of transition risks of climate change on Chinese financial market stability. Front. Environ. Sci. 10:991775. doi: 10.3389/fenvs.2022.991775
Received: 11 July 2022; Accepted: 25 July 2022;
Published: 26 August 2022.
Edited by:
Muhammad Mohiuddin, Laval University, CanadaCopyright © 2022 An, Zheng, Li and Lin. This is an open-access article distributed under the terms of the Creative Commons Attribution License (CC BY). The use, distribution or reproduction in other forums is permitted, provided the original author(s) and the copyright owner(s) are credited and that the original publication in this journal is cited, in accordance with accepted academic practice. No use, distribution or reproduction is permitted which does not comply with these terms.
*Correspondence: Lin Zheng, zhenglin@mail.sdufe.edu.cn