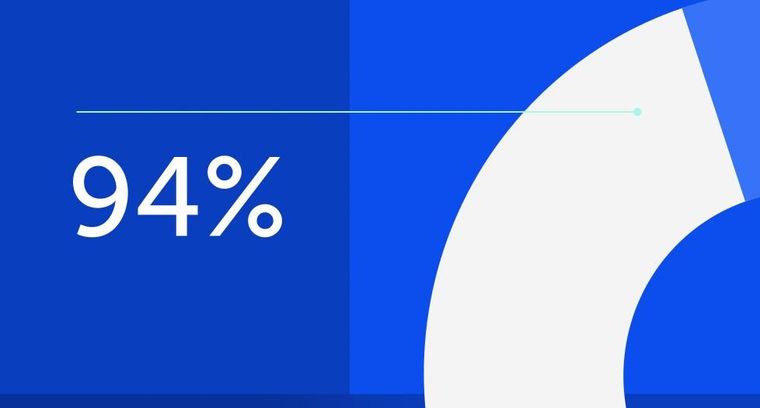
94% of researchers rate our articles as excellent or good
Learn more about the work of our research integrity team to safeguard the quality of each article we publish.
Find out more
ORIGINAL RESEARCH article
Front. Environ. Sci., 03 October 2022
Sec. Atmosphere and Climate
Volume 10 - 2022 | https://doi.org/10.3389/fenvs.2022.987272
The 24th Winter Olympics was held in Beijing, and the air quality in the Beijing area has become the focus of the world’s attention. The Beijing government has taken a series of strict measures to control pollutant emissions during the Winter Olympics, which also provides us with a valuable opportunity to study the impact of meteorological conditions on pollutants. We defined November, December, January, February, and March as the polluted period in Beijing, and used the T-PCA method to divide the circulation types (CTs) affecting Beijing into six kinds (CT1-CT6). It was found that under the control of the western high pressure (CT1) and the northwest high pressure (CT4), the concentrations of PM2.5, NO2, SO2 and CO in Beijing were lower; while under the control of the northern high pressure (CT2), eastern high pressure (CT5), southeast high (CT3) and northeast low pressure (CT6), the concentration of PM2.5, NO2, SO2 and CO is higher. By analyzing the daily CTs, wind field and pollutant concentration changes in the Beijing area during the Beijing Winter Olympics, it was found that when two pollution events occurred during the Winter Olympics, the Beijing area was mainly prevailed by CT2, CT3, and CT6. Comparing the frequency of occurrence of six CTs during the 2022 Winter Olympics and the same period from 2014 to 2021, it was found that the proportion of CT1 and CT4 increased significantly during the Winter Olympics. Finally, the FLEXPART-WRF model was used to analyze the 48-h backward footprint distribution of pollutant particles in Beijing during the Winter Olympics. It further showed that the circulation in the Beijing area during the Winter Olympics was generally conducive to the dispersion of pollutants, and the air quality was better.
The 24th Winter Olympics was held in Beijing, China, from February 4 to 20, 2022 (Wang et al., 2022b; Zhang et al., 2022). Being China’s capital city, the air quality in Beijing during the Winter Olympics has become the focus of global attention. In recent years, under the guidance of the Beijing-Tianjin-Hebei coordinated development strategy, the Beijing Municipal Government has further strengthened the governance of the air quality in the region (Chen et al., 2008; Cao et al., 2015; Miao et al., 2017; Zhai et al., 2019; Gong et al., 2022a; Gong et al., 2022b). It has taken a series of measures such as shutting down high-energy-consuming enterprises, restricting the use of motor vehicles, and widely using new energy (Miao et al., 2015; Su et al., 2015; Su et al., 2015; Ye et al., 2016; Yang et al., 2018; Sun et al., 2021). However, the emission of air pollution precursors from the Yangsha and North China Plain in the central and western regions of Inner Mongolia in autumn and winter still seriously affects the air quality in Beijing, resulting in the frequent occurrence of severe haze events in the region (Miao et al., 2020; Miao et al., 2021; Zhang et al., 2022). Air pollution remains one of the greatest challenges in the process of environmental improvement in the region.
Large-scale synoptic circulation determines the specific climate background of a region and provides the basic dynamic field for regional dispersion and transport of particulate matter (Zhao et al., 2019; Zhao et al., 2021a; Zhao et al., 2021b; Wang et al., 2022a; Wang et al., 2022b; Wang et al., 2022c). Several studies reveal the significance of weather circulation on air quality (Zhang J.P et al., 2012; Russo et al., 2014; Zhou et al., 2018; Zhou et al., 2019; Zhou et al., 2019; Zong et al., 2021). Studied the relationship between large-scale synoptic circulation and particle concentration in the Yangtze River Delta region and found that certain specific circulation conditions (such as weak high-pressure front, saddle field, and offshore low pressure) are not conducive to the dispersion of pollutants and caused poor air quality. studied the effects of weather circulation and straw burning on the PM2.5 concentration in Northeast China. It was found that a weak and stable high-pressure system led to poor dispersion conditions that increased the PM2.5 concentration, and the front of the trough increased the PM2.5 concentration. The southwest warm and humid airflow enhanced the hygroscopic growth ability of aerosol particles, which also led to an increase in PM2.5 concentration. Miao et al., 2020 studied the relationship between severe haze events in the Beijing-Tianjin-Hebei region and the planetary boundary layer and weather circulation and found that under the influence of the southeast high, northern low, and eastern high-pressure systems, warm air often suppresses the development of the boundary layer hight, resulting in the deterioration of air quality in the Beijing-Tianjin-Hebei region. Several studies have shown that local meteorological conditions have a strong dependence on weather circulation, which will affect the specific distribution of pollutants (Levy et al., 2008a; Levy et al., 2008b; Levy et al., 2010; Russo et al., 2016; Ye et al., 2016; Russo et al., 2018; Liu et al., 2019; Xu et al., 2019; Xu et al., 2020; Li X et al., 2021; Li Y et al., 2021; Sun et al., 2021; Wang et al., 2022a; Wang et al., 2022d). Sun et al., 2021 studied the formation and dissipation mechanism of pollution events and found that the wind speed and temperature in the formation stage of the pollution events were much lower than that in the dissipation stage. Additionally, the changes in pollutant concentrations were significantly affected by changes in relative humidity. Liu et al., 2019 studied the relationship between haze and meteorological conditions in Shanghai in autumn and winter and found that strong local atmospheric inversions often occurred, which inhibited the development of the boundary layer and caused the accumulation of pollutants under the influence of high pressures in the south and southwest region. When studying the causes of haze events in the North China Plain, Ye et al., 2016 found that local meteorological conditions with weak wind speed and high relative humidity often occur under the northern high, northeast high, and southeast high pressures. And the specific meteorological conditions are not conducive to the diffusion of matter but are conducive to the hygroscopic growth of particulate matter, resulting in serious and persistent haze events.
Several studies (Zhang Y et al., 2012; Zhai et al., 2019; Zhai et al., 2019) have shown that the autumn and winter seasons (November to March) are periods of poor air quality in Beijing (referred to as the polluted period in this study). In this study, the meteorological conditions in the Beijing area over the last 20 years were considered as the research objects, and the T-mode principal component analysis (T-PCA) method was used (Richman, 1981; Compagnucci and Richman, 2008; Huth et al., 2008). The circulation patterns that primarily affected the area during this period were classified into six types. The FLEXPART-WRF model was used to analyze the footprint distribution of pollutants in the areas under the six circulation patterns. Combined with local meteorological conditions, the pollutant concentrations corresponding to each circulation pattern were analyzed, and the relationship between weather circulation, local meteorological conditions, and air quality in Beijing was studied. The study also explored the positive impact of weather conditions on the improvement of air quality in the Beijing area during the Beijing Winter Olympics. The distribution of pollutant imprints during the Winter Olympics was simulated using the FLEXPART-WRF model, where it was found that favorable meteorological conditions were significant for the improvement of air quality in the area during the Beijing Winter Olympics.
This study used mean sea level pressure field data (SLP) from the ERA5 reanalysis dataset from the European Centre for Medium-Range Weather Forecasts (ECMWF)to determine the diurnal circulation pattern (Kalnay et al., 1996). The data’s spatial range was within 32°N-48°N, 108°E-124°E, and the time range considered was from 1 November 2000, to 31 March 2022. The ERA5 reanalysis data assimilates radiosonde data and other data at 00:00 UTC every day, and has the best reduction effect on the weather field. The study, therefore, used the SLP data at 00:00 UTC to determine the daily circulation pattern (Zhang J.P et al., 2012; Zhao et al., 2019).
At the same time, the daily data of the ERA5 boundary layer height at 00:00 UTC was used to analyze the characteristics of the boundary layer height of each circulation pattern in the study area. The data’s spatial range was within 36°N-45°N, 111°E-121°E, and the time range considered was from 1 January 2014, to 31 March 2022.
The local meteorological conditions under each circulation pattern were statistically analyzed using the temperature, humidity, wind direction, and wind speed data from the weather station at Beijing Capital International Airport from 2015 to 2022. These observational data are released with high precision after quality control (https://quotsoft.net/air/). Additionally, considering the geographical location of the selected station is far from the urban area and is less affected by urban buildings and human factors, the data accurately reflects the meteorological elements in the area. Figure 1 presents the specific locations and surrounding topography of the meteorological observation stations in Beijing.
FIGURE 1. The location of Beijing (A) and the surrounding terrain of Beijing (B). The black dots represent the locations of the environmental monitoring stations, and the red star represents the location of the meteorological observation stations.
The air quality data were extracted from the China National Environmental Monitoring Centre (http://www.cnemc.cn). Due to the low ozone concentration in winter, there is generally no ozone pollution. Therefore, only four types of atmospheric pollutants were considered in this study (PM2.5, NO2, SO2, and CO), using hourly monitoring data and daily average data from 2015 to 2022. The air quality monitoring stations are located in representative locations such as the suburban and urban areas of the city. These stations observe and record the air quality parameters at their locations hourly and are released after quality control by the Air Quality Monitoring Center of the Ministry of Environmental Protection, China. Figure 1 presents the specific locations of the air quality monitoring sites in Beijing.
The principal component analysis of T-mode tilt rotation (T-PCA) was used to carry out the circulation classification of the daily sea level pressure field in Beijing and its surrounding areas from 2000 to 2022. Compared with other objective circulation classification methods, T-PCA can better generalize the main modes contained in the data, and the classification results obtained have higher temporal and spatial stability. The circulation patterns that primarily affect Beijing were divided into six types based on the main weather characteristics in autumn and winter in Beijing. At present, the T-PCA method has been integrated into a software package cost733class, which can be downloaded from the website http://cost733.met.no/.
The WRF model provides accurate weather field variables for the FLEXPART-WRF model in particle trajectory simulations. Two levels of nesting were used in this simulation. The first level comprised a horizontal resolution of 15 × 15 km, covering the entire North China Plain, and the second level comprised a resolution of 4 × 4 km, covering the entire Beijing and surrounding areas. The main parameterization schemes considered were the Mellor-Yamada-Janjic (Eta) TKE boundary layer parameterization scheme (Janjic, 2001), Noah land surface scheme (Chen et al., 2006), WSM 3-class simple ice microphysical process scheme (Hong et al., 2004), Kain-Fritsch (new Eta) cumulus convection parameterization scheme (Kain, 2004), Monin-Obukhov (Janjic Eta) near-surface layer parameterization scheme (Nakanishi and Niino, 2006), RRTM longwave radiation scheme (Mlawer et al., 1997) and Dudhia shortwave radiation scheme (Dudhia, 1989). The initial and boundary conditions of the model used the NCEP FNL reanalysis data prepared operationally every 6 hours (horizontal resolution 0.25×0.25), and the model outputs results every 30 min.
The study uses the FLEXPART-WRFV3.3 version (Brioude et al., 2013) of the Lagrangian particle diffusion model that uses the function of backward simulation of particle trajectories. The center of Beijing was selected as the particle release point (38.9°N, 116.4°E). First, the heavy pollution periods (PM2.5 concentration is greater than the national primary standard of 75 μg/m3) in Beijing were screened under six circulation patterns from November 2021 to March 2022. The model releases particles evenly every 1 h during the heavy pollution period (48 h) under six circulation patterns in Beijing and releases 50,000 particles throughout the simulation period. A total of five vertical layers (10, 100, 500, 1,000, and 5,000 m) were set up, where the particle cluster release height was 10–1,000 m, and the horizontal resolution was 0.125◦×0.125◦. The model comprehensively considers the process of emission transport, turbulent diffusion, and wet and dry deposition and simulates particle dispersion and aggregation, possible source distribution, etc., by calculating the backward transport trajectory of large-scale particles. In this study, the 48-h particle imprints (Zhang Y et al., 2012; Shen et al., 2021) of the entire atmosphere were superimposed under several circulation patterns to obtain a comprehensive imprint distribution which helps in analyzing the source of pollutants and determining the factors affecting the diffusion of pollutants.
Figure 2 presents the proportions of the six circulation patterns in the 5-months polluted period from 2000 to 2022. Figure 3A presents the results of the weather circulation classification in Beijing during the polluted period from 2000 to 2022. The six circulation patterns are recorded as high pressure in the west with a large pressure gradient (CT1), high pressure in the north (CT2), high pressure in the south (CT3), high pressure in the northwest (CT4), high pressure in the northeast (CT5), high pressure in the southeast and northeast Low-pressure with small pressure gradient (CT6). Among them, CT1 was the most common type, with a frequency of 23.76%, followed by CT2 and CT4, demonstrating a frequency of 23.32% and 21.09%, respectively. From Figure 2 and Figure 3A, it may be observed that CT1, CT2, and CT4 often appear in winter (CT1 often appears in December, CT4 in January, and CT2 in February). The Beijing area was primarily affected by the cold high-pressure system under the control of the three CTs (CT1, CT2, and CT4). The high-pressure centers were located in the west (CT1), north (CT2), and northwest (CT4) of Beijing, far from the Beijing area. The frequency of CT3, CT5, and CT6 demonstrated an initial decreasing and then increasing trend from November to March and often appeared in late autumn (November) and early spring (March). The Beijing area was primarily located at the back of the cold high pressure and was closer to the high-pressure center under the control of CT3, while the area was primarily affected by the offshore high-pressure system and was located at the back of the high-pressure area with a small pressure gradient under the control of CT5 and CT6.
FIGURE 2. The monthly proportion of six circulation patterns in Beijing during the polluted period from 2000 to 2022.
FIGURE 3. (A) Six main weather circulation patterns affecting the Beijing area, (B) local wind field under the six circulation patterns (unit: m/s), (C) height (unit: m) distribution of planetary boundary layer under the six circulation patterns, (D) 48 h backward footprint (unit:
Figure 3B presents the distribution of the local wind directions and wind speeds under six CTs in Beijing from 2014 to 2022, and Figure 3C presents the distribution of the boundary layer height under the six CTs from 2014 to 2022. Table 1 presents the temperature, relative humidity, wind speed, and boundary layer height under the six CTs from 2014 to 2022. CT1 and CT4 were mainly associated with strong northwesterly wind (average wind speed was 2.3 m/s (CT1) and 2.55 m/s (CT4), respectively). And at this time, the average boundary layer height in the Beijing area was higher (average boundary layer height was 591.97 m (CT1) and 458.46 m (CT4)). Low temperature (average temperature of -2.06 (CT1) and -0.78 (CT4), respectively), dry environment (average relative humidity of 39.47% and 41.86%, respectively), and good horizontal and vertical diffusion conditions make pollutants less likely to accumulate when CT1 and CT4 prevailed. CT2, CT3, CT5, and CT6 were mainly related to the weak northeasterly winds (average wind speeds were 1.69 m/s, 1.36 m/s, 1.54 m/s, 1.34 m/s, respectively), which are not conducive to the horizontal diffusion of pollutants. At the same time, under CT2, CT3, CT5, and CT6 prevailed, the average boundary layer height in the Beijing area was lower (the average boundary layer heights were 104.44 m (CT2), 62.5 m (CT3), 67.31 m (CT5) and 66.32 m (CT6), respectively), which would cause pollutants to accumulate on the surface layer and is not conducive to vertical diffusion. Among them, CT3, CT5, and CT6 are also related to the southwest warm and humid airflow. The temperature in Beijing (average temperature -0.30, 1.01, and 2.04) and the relative humidity (average relative humidity is 60.89%, 66.43%, and 65.25) are therefore relatively higher under CT3, CT5, and CT6 prevailed, respectively, which promotes the hygroscopic growth of particulate matter. The Beijing area was more prone to the accumulation of pollutants under CT3, CT5, and CT6 prevailed.
TABLE 1. The average concentrations of four major pollutants (PM2.5, NO2, SO2, and CO) as well as the average values of temperature, humidity, wind speed, and boundary layer height under the six circulation patterns from 2014 to 2022.
Figure 3D presents the 48-h backward imprint distribution of pollutants simulated by FLEXPART-WRF when severe pollution occurred under the six circulation patterns in Beijing from November 2021 to March 2022. Figure 4 shows the concentration distribution of the four major pollutants under the six circulation patterns in Beijing from 2014 to 2022. Combining Table 1 and Figure 3D, and Figure 4, it may be observed that the concentrations of PM2.5, NO2, SO2, and CO in Beijing are 48.75 μg/m³, 35.81 μg/m³, 7.85 μg/m³ and 0.87 mg/m3, respectively, under the CT1 prevailed, while the concentrations were 50.07 μg/m³, 38.23 μg/m³, 9.01 μg/m³ and 0.88 mg/m³, respectively under the prevail of CT4. Under the prevail of the above two circulation patterns (CT1 and CT4), most of the pollutants traveled from the north-northwest of Beijing, and the residence time was generally relatively small (less than 20 min). The concentrations of pollutants in the Beijing area were relatively low under the prevail of CT1 and CT4. The concentrations of PM2.5, NO2, SO2, and CO were 87.42 μg/m³, 54.24 μg/m³, 14.94 μg/m³, and 1.37 mg/m³, respectively, under CT2 prevailed and the concentrations were 90.54 μg/m³, 55.02 μg/m³, 12.75 μg/m³ and 1.30 mg/m³, respectively under CT6 prevailed. Under the above two circulation patterns (CT2 and CT6) prevailed, the pollutants generally traveled from two directions in the west and east of Beijing, and the pollutant residence time was long (approximately 1 h). The concentration of pollutants in Beijing was the highest at this time. Under CT3 prevailed, the residence time of pollutants was longer (more than 1 h), and the pollutants primarily traveled from the northwest direction. The concentration of pollutants was also relatively high at this time. The average concentrations of PM2.5, NO2, SO2, and CO were 82.67 μg/m³, 50.35 μg/m³, 16.01 μg/m³, and 1.29 mg/m³, respectively. Under CT5 prevailed, the pollutants primarily traveled from the east of Beijing, and the residence time was short (less than 40 min). The concentration of pollutants in Beijing was lower under CT5 prevailed at this time. The average concentrations were 76.12 μg/m³, 52.15 μg/m³, 11.02 μg/m³ and 1.25 mg/m³, respectively.
FIGURE 4. Concentration distributions of PM2.5, NO2, SO2, and CO under the six circulation patterns in Beijing from 2014 to 2022. The red triangles represent mean values. The bottoms and tops of the lines represent the values of the 5th and 95th percentiles, respectively; the lower sides of the rectangle represent the 25th percentile, the middle line represents the median, and the top lines represents the 75th percentile. The black dots represent the discrete values which are considered outliers to the distribution.
In general, the relationship between the concentrations of the four major pollutants and the CTs in Beijing was relatively consistent. The residence time of the pollutants was relatively small under CT1 and CT4 prevailed, and they traveled from the north-northwest direction. The diffusion conditions were better, and the average concentrations of the pollutants were the lowest. Under CT2 and CT6 prevailed, the pollutants in Beijing traveled from two directions at the same time, and the residence time was long, the diffusion conditions were poor, and the average concentration was high.
Figure 5 presents the concentration trends of the four major pollutants before, during, and after the Beijing Winter Olympics in February 2022, as well as the CTs and wind field variation time series. As presented in Figure 5, it can be observed that in February 2022, the overall air quality in Beijing was good, and the pollutant concentration was maintained within the national first-level standard for most of the time. Considering the changes in the concentrations of the four pollutants are similar, PM2.5 was taken as an example to explain. Although there were two pollution incidents (PM2.5 concentration exceeding 35 μg/m³) during the Winter Olympics (gray sections) from 9 February to 13 February and 17 February to 19 February, the PM2.5 concentration only briefly exceeded 75 μg/m³ on 11 February. The air quality was good throughout the Winter Olympics. To further study the influence of meteorological conditions on the changes in pollutant concentrations, a specific analysis of the changes in circulation patterns and wind fields was introduced during the Winter Olympics.
FIGURE 5. Hourly concentration changes of the four major pollutants (PM2.5, NO2, SO2, and CO) as well as the time series of circulation patterns and wind fields in Beijing in February 2022. The gray sections represent the Beijing winter Olympics (from 4 February 2022 to 20 February 2022), and the red sections represent the period of pollution events.
As can be seen from Figure 5, that before 8 February, the Beijing area was mainly prevailed by CT1 and CT4. The high-pressure area was located in the northwest of Beijing, far from the capital. The pressure gradient was large, and the pollutants would not accumulate under the north westerlies. At this time, the PM2.5 concentration was maintained below 20 μg/m³, the CO concentration was maintained below 0.4 mg/m³, the SO2 concentration was maintained below 3 μg/m³, and the NO2 concentration was maintained below 30 μg/m³. The overall air quality at this stage was considered to be good. The Beijing area was located at the rear of the high-pressure area from 8 February to 11 February under the CT3 and CT6 prevailed. The pressure gradient weakened, the wind speed became lower, and the southwest airflow appeared due to the high pressure at sea at this time. The meteorological conditions were favorable for the accumulation of pollutants. At this time, the PM2.5 concentration exceeded 35 μg/m³, the NO2 concentration exceeded 60 μg/m³, and the air quality was considered to be poor. From 11 February to 13 February, the Beijing area was prevailed by CT2. The high-pressure area was located in the north of Beijing. The east wind brought pollutants back to the capital. The accumulation of pollutants in a short period caused the pollutant concentration to rise. The PM2.5 concentration was maintained at a level higher than 35 μg/m³ for part of the period, and the CO concentration was higher than 1 mg/m³. The Beijing area was first prevailed by CT4 and then by CT2 from 14 February to 17 February. The air quality, therefore, deteriorated again, and the PM2.5 concentration gradually increased from below 20 μg/m³ to nearly 35 μg/m³. During the period from 17 February to 19 February, the southwesterly and northeasterly winds appeared when prevailed by CT2 and CT5, which once again caused a short-term accumulation of pollutants. The CO concentration was maintained at 0.4–0.8 mg/m³, PM2.5 concentration exceeded 50 μg/m³ for a short time, and NO2 concentration exceeded 35 μg/m³. From 19 February to 23 February, the air quality in Beijing was good and remained constant, with the PM2.5, NO2, SO2, and CO concentrations maintained within 20 μg/m³, 35 μg/m³, 3 μg/m³, and 0.4 mg/m³, respectively, when prevailed by CT1 and CT4. From 23 February to 25 February, the southwest airflow caused the pollutants to accumulate continuously under the influence of CT2, CT3, and CT6. The pollutant concentration peaked during the night of February 25, when the PM2.5 concentration reached 120 μg/m³, the NO2 concentration was close to 65 μg/m³, and CO concentration exceeded 1 mg/m³. On February 26, the strong northwest winds under the control of CT1 caused the accumulated pollutants to dissipate rapidly, and the concentrations of PM2.5, NO2, SO2, and CO remained within 10 μg/m³, 10 μg/m³, 2 μg/m³ and 0.2 mg/m³, respectively. From 27 February to 28 February, under CT3 prevailed, the northeasterly and southeasterly winds alternated, and the air quality deteriorated, with PM2.5 and NO2 concentrations approaching 80 μg/m³ and 70 μg/m³, respectively. Soon after 28 February, under CT4 prevailed, the pollutant concentrations decreased rapidly, the air quality improved, and the concentrations of PM2.5, NO2, SO2, and CO remained within 10 μg/m³, 10 μg/m³, 2 μg/m³, 0.1 mg/m³, respectively.
During the period of poor air quality during the Winter Olympics, the corresponding circulation patterns were found to be CT2, CT3, and CT6, indicating that unfavorable dispersion conditions were the main reasons for the poor air quality in some periods during the Winter Olympics.
Figure 6 presents the comparison of the frequency of the six circulation patterns during the Beijing Winter Olympics and during the same period from 2014 to 2021. As observed in Figure 6, the frequency of CT1 and CT4 (corresponding to good air quality) from February 2014 to February 2021 accounted for approximately 17% each, while the frequency of CT1 and CT4 during the 2022 Winter Olympics were approximately 25% and 28% (CT1 and CT4 occurred 7 and 8 times, respectively) each. CT1 and CT4 appeared for a total of 15 days during the Winter Olympics, accounting for more than 50% of the total. The frequency of CT2, CT5, and CT6 demonstrated a significant downward trend compared with the same period of the previous years. Among them, the proportion of CT2 and CT6 (corresponding to poor air quality) decreased by 11.6% and 3.6%, respectively.
FIGURE 6. Comparison of the frequency of the six circulation patterns during the 2022 Beijing Winter Olympics and the same period in 2014–2021.
In general, during the Winter Olympics, the total frequency of CT1 and CT4 increased significantly, and that of CT2, CT3, CT5, and CT6 decreased significantly compared with the same period of the previous years.
Figure 7 presents the contaminant backward footprint distribution during two small-scale pollution events (from 9 February to 13 February, from 17 February to 19 February), throughout the Winter Olympics, and during February 2022. It can be seen from Figure 7 that the pollutants during the period from 9 February to 13 February primarily traveled from the southeast, while those during the period from 17 February to 19 February mainly traveled from the southwest. This proves that the long-distance transport of pollutants from the sea and central China is the primary cause of these two pollution incidents. During the two small-scale pollution incidents, the particle residence time was generally longer, and the residence time was greater than the retention time during the entire Winter Olympics and February 2022. This proves that the pollutants from the southwest and southeast are affected by weather conditions that are not conducive to rapid transport, resulting in the accumulation of pollutants. During the entire Winter Olympics and 2022.02, the footprints were distributed in the northwest, southwest, southeast, and northeast, and the residence time was generally short (much less than 20 min). In particular, the residence time in the north to northwest was very short. This proved that the pollutants diffused quickly and did not accumulate during most of the period, leading to better air quality.
FIGURE 7. FLEXPART-WRF backward trajectory simulation of the entire Winter Olympics, 9 February to 13 February, 17 February to 19 February, and February 2022 48-h footprint distribution. The unit is s*kg/m3.
This study first defines the polluted period in the Beijing area. According to the sea level pressure field from 2000 to 2022, six weather circulation patterns were determined in the Beijing area that affected the polluted period. The local meteorological conditions of these six circulation patterns and their relationship with the concentrations of the four pollutants were analyzed. Finally, the changing trend of pollutants during the Winter Olympics was analyzed by combining the circulation pattern changes and footprint analysis. The following conclusions were drawn:
1) Higher pollutant concentrations are associated with specific circulation patterns. The concentration of pollutants was generally relatively low when the Beijing area was prevailed by CT1 and CT4. The footprints primarily traveled from the north-northwest direction, and the residence time was short. On the contrary, the air quality was poor when this area was prevailed by CT2, CT3, and CT6. The footprints traveled from both the western and eastern directions during this time, with the residence time being longer.
2) The obvious increase in the frequency of CT1 and CT4 during the Beijing Winter Olympics is the main reason for the overall improved air quality during that time.
3) Small-scale pollution events during the Beijing Winter Olympics were related to CT2, CT3, and CT6. The pollutants traveled through long-distance transportation in central China and the sea. The blocking conditions caused by the southwest and the eastern offshore airflow are the main reasons for the formation of pollution events.
The original contributions presented in the study are included in the article/supplementary material, further inquiries can be directed to the corresponding authors.
Data curation, JZ. Methodology, JZ. Project administration, CZ. Software, HD and WC. Visualization, HD and WC. Writing—original draft, TW. Writing—review and editing, CZ and ZZ.
This research was funded by [National Natural Science Foundation Committee of China] grant number (41875181, 41475021, 41375154 and 41405146), [National Key R&D Program of China] grant number (2018YFC0213600) and (Chinese Prime Minister Fund) grant number (DQGG0104).
The authors declare that the research was conducted in the absence of any commercial or financial relationships that could be construed as a potential conflict of interest.
All claims expressed in this article are solely those of the authors and do not necessarily represent those of their affiliated organizations, or those of the publisher, the editors and the reviewers. Any product that may be evaluated in this article, or claim that may be made by its manufacturer, is not guaranteed or endorsed by the publisher.
Brioude, J., Arnold, D., Stohl, A., Cassiani, M., Morton, D., Seibert, P., et al. (2013). The Lagrangian particle dispersion model FLEXPART-WRF version 3.1. Geosci. Model Dev. 6 (6), 1889–1904. doi:10.5194/gmd-6-1889-2013
Cao, Z., Sheng, L., Liu, Q., Yao, X., and Wang, W. (2015). Interannual increase of regional haze-fog in North China Plain in summer by intensified easterly winds and orographic forcing. Atmos. Environ. 122, 154–162. doi:10.1016/j.atmosenv.2015.09.042
Chen, F., Tewari, M., Kusaka, H., and Warner, T. (2006). “Current status of urban modeling in the community Weather Research and Forecast (WRF) model,” in paper presented at Joint Session with Sixth Symposium on the Urban Environment and AMS Forum: Managing our Physical and Natural Resources: Successes and Challenges, the 86th AMS Annual Meeting, AMS, Atlanta, Georgia, 28 January–3 February.
Chen, Z. H., Cheng, S. Y., Li, J. B., Guo, X., Wang, W., and Chen, D. (2008). Relationship between atmospheric pollution processes and synoptic pressure patterns in northern China. Atmos. Environ. 42 (24), 6078–6087. doi:10.1016/j.atmosenv.2008.03.043
Compagnucci, R. H., and Richman, M. B. (2008). Can principal component analysis provide atmospheric circulation or teleconnection patterns? Int. J. Climatol. 28 (6), 703–726. doi:10.1002/joc.1574
Dudhia, J. (1989). Numerical study of convection observed during the winter monsoon experiment using a mesoscale two-dimensional model. J. Atmos. Sci. 46, 3077–3107. doi:10.1175/1520-0469(1989)046<3077:nsocod>2.0.co;2
Gong, S., Liu, Y., He, J., Zhang, L., Lu, S., and Zhang, X. (2022a). Multi-scale analysis of the impacts of meteorology and emissions on PM2. 5 and O3 trends at various regions in China from 2013 to 2020 1: Synoptic circulation patterns and pollution[J]. Sci. Total Environ. 815, 152770. doi:10.1016/j.scitotenv.2021.152770
Gong, S., Zhang, L., Liu, C., Lu, S., Pan, W., and Zhang, Y. (2022b). Multi-scale analysis of the impacts of meteorology and emissions on PM2.5 and O3 trends at various regions in China from 2013 to 2020 2. Key weather elements and emissions. Sci. Total Environ. 824, 153847. doi:10.1016/j.scitotenv.2022.153847
Hong, S. Y., Dudhia, J., and Chen, S. H. (2004). A revised approach to ice microphysical processes for the bulk parameterization of clouds and precipitation. Mon. Weather Rev. 132, 103–120. doi:10.1175/1520-0493(2004)132<0103:aratim>2.0.co;2
Huth, R., Beck, C., Philipp, A., Demuzere, M., Ustrnul, Z., Cahynova, M., et al. (2008). Classifications of atmospheric circulation patterns. Ann. N. Y. Acad. Sci. 1146, 105–152. doi:10.1196/annals.1446.019
Janjic, Z. I. (2001). Nonsingular implementation of the mellor-yamada level 2.5 scheme in the NCEP meso model, 437. Maryland: National Centers for Environmental Prediction. Office Note.
Kain, J. S. (2004). The Kain fritsch convective parameterization: an update. J. Appl. Meteor. 43, 170–181. doi:10.1175/1520-0450(2004)043<0170:tkcpau>2.0.co;2
Kalnay, E., Kanamitsu, M., Kistler, R., Collins, W., Deaven, D., Gandin, L., et al. (1996). The NCEP/NCAR 40-year reanalysis project. Bull. Am. Meteorol. Soc. 77, 437–471. doi:10.1175/1520-0477(1996)077<0437:tnyrp>2.0.co;2
Levy, I., Dayan, U., and Mahrer, Y. (2008a). Studying coastal recirculation with a simplified analytical land-sea breeze model. J. Geophys. Res. 113, D03104. doi:10.1029/2007jd008628
Levy, I., Dayan, U., and Mahrer, Y. (2008b). A five-year study of coastal recirculation and its effect on air pollutants over the East Mediterranean region. J. Geophys. Res. 113, D16121. doi:10.1029/2007jd009529
Levy, I., Dayan, U., and Mahrer, Y. (2010). Differing atmospheric scales of motion and their impact on air pollutants. Int. J. Climatol. 30, 612–619. doi:10.1002/joc.1905
Li, Y., Liu, J., Han, H., Zhao, T., Zhang, X., Zhuang, B., et al. (2019). Collective impacts of biomass burning and synoptic weather on surface PM2.5 and CO in Northeast China. Atmos. Environ. 213, 64–80. doi:10.1016/j.atmosenv.2019.05.062
Li, X., Miao, Y., Ma, Y., Wang, Y., and Zhang, Y. (2021). Impacts of synoptic forcing and topography on aerosol pollution during winter in Shenyang, Northeast China. Atmos. Res. 2021, 262105764. doi:10.1016/j.atmosres.2021.105764
Li, Y., Miao, Y., Che, H., and Liu, S. (2021). On the heavy aerosol pollution and its meteorological dependence in Shandong province, China. Atmos. Res. 256, 105572. doi:10.1016/j.atmosres.2021.105572
Liu, N., Zhou, S., Liu, C., and Guo, J. (2019). Synoptic circulation pattern and boundary layer structure associated with PM2.5 during wintertime haze pollution episodes in Shanghai. Atmos. Res. 228, 186–195. doi:10.1016/j.atmosres.2019.06.001
Miao, Y., Hu, X. M., Liu, S., Qian, T., Xue, M., Zheng, Y., et al. (2015). Seasonal variation of local atmospheric circulations and boundary layer structure in the Beijing-Tianjin-Hebei region and implications for air quality. J. Adv. Model. Earth Syst. 7 (4), 1602–1626. doi:10.1002/2015ms000522
Miao, Y., Guo, J., Liu, S., Liu, H., Li, Z., Zhang, W., et al. (2017). Classification of summertime synoptic patterns in Beijing and their associations with boundary layer structure affecting aerosol pollution. Atmos. Chem. Phys. 17, 3097–3110. doi:10.5194/acp-17-3097-2017
Miao, Y., Che, H., Zhang, X., and Liu, S. (2020). Integrated impacts of synoptic forcing and aerosol radiative effect on boundary layer and pollution in the Beijing–Tianjin–Hebei region, China. Atmos. Chem. Phys. 20 (10), 5899–5909. doi:10.5194/acp-20-5899-2020
Miao, Y., Che, H., Zhang, X., and Liu, S. (2021). Relationship between summertime concurring PM2.5 and O3 pollution and boundary layer height differs between Beijing and Shanghai, China. Environ. Pollut. 268, 115775. doi:10.1016/j.envpol.2020.115775
Mlawer, E. J., Taubman, S. J., Brown, P. D., Iacono, M. J., and Clough, S. A. (1997). Radiative transfer for inhomogeneous atmospheres: RRTM, a validated correlated-k model for the longwave. J. Geophys. Res. 102, 16663–16682. doi:10.1029/97jd00237
Nakanishi, M., and Niino, H. (2006). An improved mellor–yamada level-3 model: Its numerical stability and application to a regional prediction of advection fog. Bound. Layer. Meteorol. 119 (2), 397–407. doi:10.1007/s10546-005-9030-8
Richman, M. B. (1981). Obliquely rotated principal components: an improved meteorological map typing technique. J. Appl. Meteor. 20, 1145–1159. doi:10.1175/1520-0450(1981)020<1145:orpcai>2.0.co;2
Russo, A., Trigo, R. M., Martins, H., and Mendes, M. T. (2014). NO2, PM10 and O3 urban concentrations and its association with circulation weather types in Portugal. Atmos. Environ. 89, 768–785. doi:10.1016/j.atmosenv.2014.02.010
Russo, A., Gouveia, C., Levy, I., Dayan, U., Jerez, S., Mendes, M., et al. (2016). Coastal recirculation potential affecting air pollutants in Portugal: The role of circulation weather types. Atmos. Environ. 135, 9–19. doi:10.1016/j.atmosenv.2016.03.039
Russo, A., Gouveia, C. M., Soares, P. M., Cardoso, R. M., Mendes, M. T., and Trigo, R. M. (2018). The unprecedented 2014 legionnaires’ disease outbreak in Portugal: atmospheric driving mechanisms. Int. J. Biometeorol. 62 (7), 1167–1179. doi:10.1007/s00484-018-1520-8
Su, S., Song, M., Wu, C., Wu, X., Guangyuan, L., Xingyu, C., et al. (2015). The potential impact of temperature and wind speed in North China on the formation of winter haze [J]. Tech. Equip. Environ. Pollut. Control 9 (08), 3928–3936.
Sun, Z., Zhao, X., Li, Z., Tang, G., and Miao, S. (2021). Boundary layer structure characteristics under objective classification of persistent pollution weather types in the Beijing area. Atmos. Chem. Phys. 21, 8863–8882. doi:10.5194/acp-21-8863-2021
Wang, T., Du, H., Zhao, Z., Russo, A., Zhang, J., and Zhou, C. (2022a). The impact of potential recirculation on the air quality of Bohai Bay in China. Atmos. Pollut. Res. 13 (1), 101268. doi:10.1016/j.apr.2021.101268
Wang, T., Du, H., Zhao, Z., Zhou, Z., Russo, A., Xi, H., et al. (2022b). Prediction of the impact of meteorological conditions on air quality during the 2022 Beijing winter Olympics. Sustainability 14 (8), 4574. doi:10.3390/su14084574
Wang, T., Du, H., Zhao, Z., Zhang, J., and Zhou, C. (2022c). Impact of meteorological conditions and human activities on air quality during the COVID-19 lockdown in Northeast China[J]. Front. Environ. Sci. 454, 877268. doi:10.3389/fenvs.2022.877268
Wang, T., Du, H., Zhao, Z., Zhang, J., and Zhou, C. (2022d). Collective influences of boundary layer process and synoptic circulation on particulate pollution: A new study in changsha-zhuzhou-xiangtan urban agglomeration of central china. Front. Environ. Sci. 10, 939147. doi:10.3389/fenvs.2022.939147
Xu, T., Song, Y., Liu, M., Cai, X., Zhang, H., Guo, J., et al. (2019). Temperature inversions in severe polluted days derived from radiosonde data in North China from 2011 to 2016. Sci. Total Environ. 647, 1011–1020. doi:10.1016/j.scitotenv.2018.08.088
Xu, Y., Xue, W., Lei, Y., Huang, Q., Zhao, Y., Cheng, S., et al. (2020). Spatiotemporal variation in the impact of meteorological conditions on PM2.5 pollution in China from 2000 to 2017. Atmos. Environ. 223, 117215. doi:10.1016/j.atmosenv.2019.117215
Yang, Y., Zheng, X., Gao, Z., Wang, H., Wang, T., Li, Y., et al. (2018). Long-term trends of persistent synoptic circulation events in planetary boundary layer and their relationships with haze pollution in winter half-year over Eastern China. J. Geophys. Res. Atmos. 123, 10, 991–11, 007. doi:10.1029/2018JD028982
Ye, X., Song, Y., Cai, X., and Zhang, H. (2016). Study on the synoptic flow patterns and boundary layer process of the severe haze events over the North China Plain in January 2013. Atmos. Environ. 124, 129–145. doi:10.1016/j.atmosenv.2015.06.011
Zhai, L., Sun, Z., Li, Z., Yin, X., Xiong, Y., Wu, J., et al. (2019). Dynamic effects of topography on dust particles in the Beijing region of China. Atmos. Environ. 213, 413–423. doi:10.1016/j.atmosenv.2019.06.029
Zhang, Y. T., Pan, X., Tian, Y., Liu, H., Chen, X., Ge, B., et al. (2022). Transport patterns and potential sources of atmospheric pollution during the XXIV Olympic Winter Games period. Adv. Atmos. Sci. 39, 1608–1622. doi:10.1007/s00376-022-1463-1
Zhang, J. P., Zhu, T., Zhang, Q. H., Li, C. C., Shu, H. L., Ying, Y., et al. (2012). The impact of circulation patterns on regional transport pathways and air quality over Beijing and its surroundings. Atmos. Chem. Phys. 12, 5031–5053. doi:10.5194/acp-12-5031-2012
Zhang, Y., Guo, Y., Li, G., Zhou, J., Jin, X., Wang, W., et al. (2012). The spatial characteristics of ambient particulate matter and daily mortality in the urban area of Beijing, China. Sci. Total Environ. 435, 14–20. doi:10.1016/j.scitotenv.2012.06.092
Zhao, Z., Xi, H., Russo, A., Du, H., Gong, Y., Xiang, J., et al. (2019). The influence of multi-scale atmospheric circulation on severe haze events in autumn and winter in Shanghai, China. Sustainability 11 (21), 5979. doi:10.3390/su11215979
Zhao, Z., Zhou, Z., Russo, A., Xi, H., Zhang, J., Du, H., et al. (2021a). Comparative analysis of the impact of weather conditions and human activities on air quality in the Dongting and Poyang Lake Region during the COVID-19 pandemic. Atmos. Pollut. Res. 12 (5), 101054. doi:10.1016/j.apr.2021.101054
Zhao, Z., Zhou, Z., Russo, A., Du, H., Xiang, J., Zhang, J., et al. (2021b). Impact of meteorological conditions at multiple scales on ozone concentration in the Yangtze River Delta. Environ. Sci. Pollut. Res. 28, 62991–63007. doi:10.1007/s11356-021-15160-2
Zhou, C. J., Wei, G., Xiang, J., Zhang, K., Li, C., and Zhang, J. (2018). Effects of synoptic circulation patterns on air quality in Nanjing and its surrounding areas during 2013–2015. Atmos. Pollut. Res. 9, 723–734. doi:10.1016/j.apr.2018.01.015
Zhou, C., Wei, G., Zheng, H., Russo, A., Li, C., Du, H., et al. (2019). Effects of potential recirculation on air quality in coastal cities in the Yangtze River Delta. Sci. Total Environ. 651, 12–23. doi:10.1016/j.scitotenv.2018.08.423
Keywords: 2022 Beijing Winter Olympics, T-mode PCA, air quality, FLEXPART-WRF model, meteorological conditions
Citation: Wang T, Du H, Cheng W, Zhao Z, Zhang J and Zhou C (2022) Influence of meteorological conditions on the air quality during the 2022 Winter Olympics in Beijing. Front. Environ. Sci. 10:987272. doi: 10.3389/fenvs.2022.987272
Received: 06 July 2022; Accepted: 15 September 2022;
Published: 03 October 2022.
Edited by:
Tirthankar Banerjee, Banaras Hindu University, IndiaReviewed by:
Swagata Payra, Birla Institute of Technology, IndiaCopyright © 2022 Wang, Du, Cheng, Zhao, Zhang and Zhou. This is an open-access article distributed under the terms of the Creative Commons Attribution License (CC BY). The use, distribution or reproduction in other forums is permitted, provided the original author(s) and the copyright owner(s) are credited and that the original publication in this journal is cited, in accordance with accepted academic practice. No use, distribution or reproduction is permitted which does not comply with these terms.
*Correspondence: Jiping Zhang, empwcGt1QDE2My5jb20=; Chengjun Zhou, bnVkdF96Y2pAZm94bWFpbC5jb20=
Disclaimer: All claims expressed in this article are solely those of the authors and do not necessarily represent those of their affiliated organizations, or those of the publisher, the editors and the reviewers. Any product that may be evaluated in this article or claim that may be made by its manufacturer is not guaranteed or endorsed by the publisher.
Research integrity at Frontiers
Learn more about the work of our research integrity team to safeguard the quality of each article we publish.