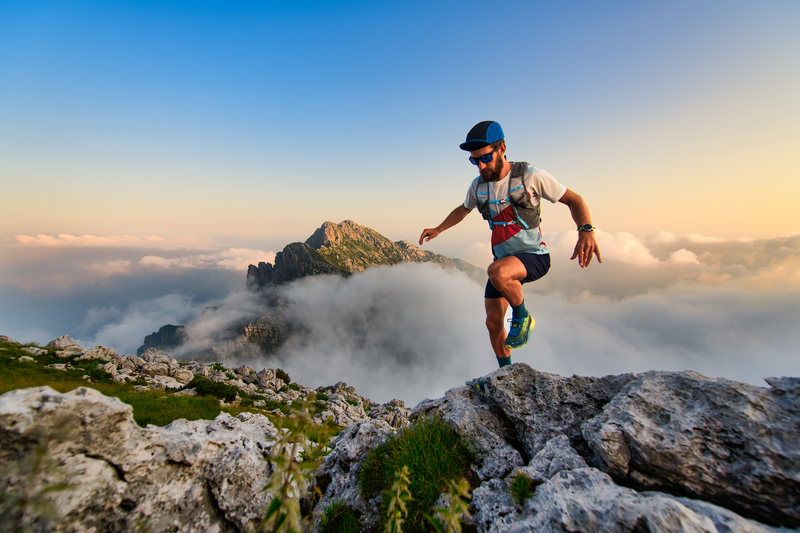
95% of researchers rate our articles as excellent or good
Learn more about the work of our research integrity team to safeguard the quality of each article we publish.
Find out more
ORIGINAL RESEARCH article
Front. Environ. Sci. , 14 September 2022
Sec. Interdisciplinary Climate Studies
Volume 10 - 2022 | https://doi.org/10.3389/fenvs.2022.984591
This article is part of the Research Topic Energy, Economy, and Climate Interactions: Challenges and Opportunities View all 12 articles
As global urbanization continues to accelerate, so does the amount of harm to the natural ecological environment caused by excessive resource extraction. In several Chinese cities, haze and excessive greenhouse gas emissions have become crucial to the development of an ecological society. To break the previous energy-intensive development model, the data element has emerged as a key driver of the new cycle of high-quality development. Therefore, additional investigation into the synergistic influence of the digital economy as a new factor on air pollution and CO2 emissions is warranted. This study examines the effects, spatial effects, and transmission mechanisms of the digital economy on the synergistic control of carbon and haze using panel data from 30 provinces in China from 2013 to 2019. The study concludes that the digital economy has an overall significant inhibiting effect on carbon dioxide emissions and haze pollution levels (the regression coefficients are -1.090 and -0.714 respectively), a significant driving effect on the efficiency of synergistic carbon and haze management, a spatial spillover effect, and a positive effect on neighboring regions (the regression coefficient is more than 0.239). By region, the digital economy in the eastern region has a greater impact on the effectiveness of carbon and haze management, whereas the digital economy in the western region has a greater impact on CO2 emission reduction, and the digital economy in the central region has an effect that is more consistent with the overall situation. Moreover, the digital economy may successfully encourage technical innovation, which in turn supports synergistic carbon and haze governance, and technological innovation plays a very important mediating role in this transmission mechanism, a finding that is resilient to geographical interaction effects. Consequently, relevant policy recommendations are presented.
China has started to pay attention to energy conservation and environmental preservation since the reform and opening up, particularly in the 1990s, but for a long time, economic growth still followed a primitive development model with high energy consumption and high emissions. Environmental pollution levels and CO2 emissions peaked in the first decade of the twenty-first century (Figure 1), and in 2007, China surpassed the United States as the world’s top carbon emitter (Liu, Z. et al., 2022). This was the result of a decade of strong economic expansion and increased industrialization. However, since 2013, different parts of the nation have faced differing levels of haze pollution (Figure 2), with PM2.5 concentrations in some places surpassing 1,000 micrograms per cubic meter, more than 40 times the permissible limit1. The Chinese government, at all levels, has implemented a number of initiatives to “tackle the haze with an iron fist,” including the adoption of the world’s strictest ultralow emission limits for flue gases and the widespread use of “coal-to-gas” (Xu and Ge, 2020) and “coal-to-electricity” (Xu et al., 2020). Particulate matter (PM) and sulfur dioxide (SO2) emissions, two of the three common air pollutants currently under control, have decreased by more than 80% from their pre-2014 peaks, while nitrogen oxide (NOx) emissions have decreased by 30% from their peaks. Indicators show that conventional pollutants have been reduced, but actual haze control is far less successful than anticipated. To maintain a particular level of air quality in the autumn and winter, the northern regions had to rely on extensive work stops and output limitations. Haze still appeared frequently, though. Widespread and protracted severe haze pollution was nevertheless inevitable in some locations, especially during the new coronavirus epidemic’s first emergence in early 2020, when the Chinese economy was nearly at a stop, seriously harming public health and the quality of economic growth (Huang et al., 2020), and even endangering lives (Han, C. et al., 2022).
FIGURE 1. The total carbon emissions and average annual haze concentration of China2
At this stage, the concept of green, low-carbon and sustainable development has become a mainstream trend in the world’s economic development, and the digital economy is an economic activity that uses data as a factor of production, using data computing, data sharing and other means3. It can efficiently increase social production efficiency and optimize the economic and industrial structure of the economy, acting as a major force behind the transformation of the traditional model of economic development and the advancement of the creation of an ecological civilization. Information components can now play an innovative role in the value chain because of the digital economy’s use of information technology to integrate and deploy resources to increase production efficiency (Miao, 2021). It is specifically suggested to encourage the integration of digitalization, intelligence, and greening in the industrial sector in the State Council’s “Action Plan to Reach the Carbon Peak by 2030.” A crucial step towards attaining carbon peaking and carbon neutrality (Liu, Z. et al., 2022) and fostering high-quality economic development is the deep integration of the digital economy and green development. However, few studies have been conducted on the digital economy and environmental pollution management, with most of the previous studies ranging from economic growth (Xiong and Xu, 2021), foreign investment (Xu et al., 2019), industrial structure (Su et al., 2021; Liu Y et al., 2022), environmental regulation (Wang et al., 2022) He, 2015), industrial agglomeration (Zeng and Zhao, 2009; Dong et al., 2020), technological innovation (Wang and Luo, 2020; Ding et al., 2022; Li et al., 2022), and other perspectives on the causes of environmental pollution and the paths of pollution abatement.
Therefore, this study constructs a spatial panel regression model using interprovincial panel data in China from 2013–2019 to measure the impact of the digital economy on the efficiency of synergistic carbon and haze management. It also examines whether technological innovation plays a mediating role between the two and better identifies the impact effect of the digital economy on atmospheric environmental management. The main innovation points are as follows. 1) Based on the perspective of atmospheric pollution, this study provides empirical evidence from China to study the pathway of synergistic management of the digital economy and carbon-haze, and enriches the research on the relationship between the digital economy and environmental pollution. 2) An input–output model is used to evaluate the efficiency of regional carbon and haze management, taking into account the differences in economic development between the eastern, central and western regions, to examine the effects of the digital economy on the management of two major pollutants, carbon dioxide and haze pollution, and to identify the similarities and differences in the effects of the digital economy on both, providing a feasible method for measuring the efficiency of environmental management, especially air pollution management. 3) To comprehensively reflect the true development of China’s digital economy at the provincial level, this paper constructs a comprehensive digital economy index evaluation system for Chinese cities from three aspects: the level of information infrastructure construction, the level of digital industrialization and the level of industrial digitization. 4) To improve the study of the transmission mechanism of the digital economy on the synergistic management of carbon-haze and to verify the mediating effect of the mechanism of technological innovation between the two in consideration of spatial interaction.
The rest of this paper is arranged as follows: Section 2 details the literature review. Section 3 presents the theoretical analysis and research hypothesis. Section 4 introduces the model construction, election and setting of variables, and data sources. Section 5 presents the spatial agglomeration characteristics of air pollution, the benchmark regression results, and the transmission mechanism. A robustness test is presented in Section 6. Section 7 summarizes the main research conclusions and discusses policy enlightenment. Figure 3 illustrates the digital economy the graphical abstract of this research. The abbreviations and terms used in the text are in Supplementary Appendix SA, while the models and equations involved are in Supplementary Appendix SB.
In terms of sectors related to the digital economy, Chen and Yan (2020) explored the impact and working mechanism of e-commerce development on SO2 air pollution prevention and control in Chinese cities and found that compared to e-commerce services, e-commerce development can significantly reduce the level of SO2 air pollution in Chinese cities and contribute more significantly to the reduction of SO2 emissions per unit of GDP. Cao et al. (2021) used the Multiperiod Difference-in-Difference method to examine the impact of the National E-commerce Demonstration Cities (NEDC) pilot on green total factor productivity (GTFP) and found that the cities’ GTFP increased by an average of 1.24% after the NEDC policy shock. The average increase in GTFP after the NEDC policy shock was 1.24%, which was more effective for western and resource-based cities, providing new evidence on the relationship between e-commerce and green development. Yang et al. (2021b) verified that there is an inverted U-shaped curve relationship between the development of the Internet industry and haze pollution in China and that haze pollution can be curbed through technological innovation in communication.
In fact, the ICT (information and communication technology) industry, the predecessor of the digital economy, has been studied in relation to the effects of environmental governance for much longer. Toffel and Horvath (2004) make a distinction between the two-way effects of ICT development on energy consumption. On the one hand, ICT development makes it possible to replace the capital-driven model with a drive to replace the costs of traditional trading, production and distribution processes, increasing the efficiency of energy and reducing the intensity of energy consumption. On the other hand, the increased efficiency of distribution stimulates market consumption demand, which in turn can lead to a sudden increase in energy consumption and exacerbate environmental pollution. There has been a great deal of debate around this idea, with some scholars arguing that ICT can improve the efficiency of companies in organizing production management, making production planning better and thus avoiding wasted energy. In addition, ICT itself is characterized by technological advances that can effectively eliminate redundancies in the production process and increase output efficiency (Moyer and Hughes, 2012). ICT at scale can drive changes in manufacturers’ production automation, reducing production costs and improving energy efficiency. In contrast, other scholars have argued that in addition to the increased energy consumption associated with the expansion of consumption, the development of the ICT sector requires a large amount of energy to keep it functioning and that the electricity consumption-driven nature of China’s economic development has become more pronounced as the ICT sector continues to grow (Peng, 2013), a finding that also holds true in emerging market economies and developed regions such as the United Kingdom, United States and Korea (Sadorsky, 2012; Kim and Heo, 2014). This also suggests that while the ICT sector can drive technological advances in production through factor substitution, it can also trigger new consumption growth and generate significant energy rebound effects.
At the same time, the issue of global warming caused by greenhouse gases has received widespread and sustained attention. The factors contributing to excessive CO2 emissions are complex and involve urbanization (Zhao and Wang, 2022), industrial production, human life and even transportation, with the burning of fossil fuels for electricity generation being the most important influence (Xu et al., 2017). It is generally accepted that the main way to control carbon emissions from industrial electricity use is to reduce the use of fossil fuels or to seek new alternative energy sources (Minx et al., 2011). Thus, increasing the efficiency of existing energy use or improving production technologies can, to some extent, mitigate or curb the increase in the greenhouse effect (Lorenzoni et al., 2007). However, there is a certain path dependency for the production technology factor, and the tendency of manufacturers to make technological progress is implicit (Acemoglu et al., 2012). In the absence of external constraints, technological progress can in turn increase CO2 emissions (Jaffe et al., 2002). Thus, the digital economy, as a development model with innovative technologies, has a similar dual effect on CO2 emissions.
Finally, a large number of studies have been conducted on the synergistic reduction of air pollutants and carbon dioxide. Qian et al. (2021) constructed a data set of over 170,000 observations and used a scenario simulation approach to reveal the huge potential for synergistic air pollutant and CO2 emission reduction in China’s industrial sector from the perspective of microenterprises and suggested that continuous improvement of energy efficiency, rationalization and restructuring of production in the existing industrial sector, accelerated electrification and vigorous development of non-fossil energy generation are important ways to achieve synergistic emission reduction benefits. Yang et al. (2022) used a pilot policy-oriented approach to empirically analyse the impact of haze pollution in the pilot provinces of the Emissions Trading System (ETS) and its surrounding provinces by using the difference-in-difference (DID) and propensity score matching-DID (PSM-DID) methods based on data from 2000 to 2017 from 31 provinces in mainland China. They found that the ETS in the pilot areas could alleviate regional haze pollution and achieve a win–win situation of controlling haze pollution and promoting CO2 emission reduction. In provinces adjacent to the pilot areas, it played a spillover effect, but the impact on PM2.5 concentrations was weaker than in the pilot areas, and there was a lag period. Zhang Q-Y. et al. (2022) argue that most studies have focused on the best measures or pathways for co-control, but there is a lack of studies that have assessed multiple cities comparatively. Only Li et al. (2020) analysed the co-control performance of Chinese cities in terms of the spatial distribution of CO2 and PM2.5 concentrations.
In conclusion, most studies have only explored the digital economy and environmental governance from one aspect or discussed the path of synergistic management of CO2 emissions and haze, without exploring the synergistic management of CO2 and haze from the perspective of the digital economy. This paper will focus on the construction of an evaluation index system for the digital economy, study the spatial effects of the digital economy and synergistic carbon-haze governance, and propose pathways for the synergistic governance of carbon and haze.
A review of the above literature reveals that the effect of the digital economy on environmental pollution is uncertain, and the relationship between economic development and the governance of haze and carbon emissions is inconclusive. However, some studies suggest that the development of the digital economy can play a role in the synergistic management of CO2 and haze. Unfortunately, relatively little literature has been published on the transmission pathways of this effect, and the underlying mechanisms of the digital economy’s influence on the synergistic management of CO2 and haze have not been systematically clarified. This paper therefore discusses this mechanism based on the questions raised above and proposes the following research hypothesis.
First, the digital economy runs on Internet technology, and data, as its main production factor, have fewer negative effects on the ecological environment in the process of circulation, exchange and storage, and the output side does not cause direct pollution to the environment. Compared to traditional industrial manufacturing, it has strong technological attributes and a green production model (Zhou et al., 2021). Again, the relatively high threshold of the digital economy industry means that digital enterprises need to meet the conditions of diversified business capabilities, mature structure and management, and focus on sustainable development. To enhance the visibility of enterprises and form positive social demonstration effects, policy makers will pay more attention to public opinion monitoring, to ecological and environmental benefits, and to optimize the industrial structure of enterprises when developing enterprises (Li, X. et al., 2021). The digital economy will bring a new round of production innovation driven by technological innovation. To improve factor productivity, enterprises will strengthen technological information cross-collaboration and cooperation through industrial digitization and mobile internet, ensure that the production behavior of enterprises is consistent with the objectives of digital governance, use digital technology to combine enterprise resources to achieve technological innovation and improve the digital level of enterprises, thus improving the efficiency of factor use and reducing unnecessary. This will improve the efficiency of factor use and reduce unnecessary resource loss, thus laying the consciousness and material foundation for the transformation of production methods to scale and green in the future (Liu, Y. et al., 2022). To break the dilemma of private data and fragmented information between departments, cutting-edge technologies such as big data, blockchain and cloud computing will be further promoted and applied in the dynamic environmental monitoring system, accelerating the transparency of data resources with the help of the Internet platform, enhancing the data collection capacity of government departments, improving the accuracy of data analysis and prediction, and improving the environmental monitoring system. This will enable the government to introduce reasonable control measures to regulate the green production of manufacturers (Su et al., 2021). Based on the above analysis, Hypothesis 1 is proposed.
H1:. Digital economy development has a positive spatial effect on the efficiency of synergistic CO2 and haze management.Second, urban agglomerations, as the main strategic form in the current evolution of China’s urbanization, have led to economic exchanges between localities and neighboring cities, promoting cooperation and coordinated development between various types of cities. However, there are still problems with the uneven distribution of resources and regional economic differentiation among cities. In addition, waste pollutants from factories and enterprises located in the upper reaches of cities may cause additional pollution in the downstream areas along rivers, while air pollution caused by factories and enterprises located at the edges of cities may easily lead to cross-border cross-pollution along with atmospheric dispersion, resulting in the spatial aggregation effect of environmental pollution and leading to an increase in government pollution. The spatial agglomeration effect of environmental pollution has led to increased costs and pressure on governments to control pollution (Li, Z. et al., 2021; Sun et al., 2021). The rise of the digital economy, with its fast, convenient and highly permeable characteristics, has broken the traditional geographical boundaries and transcended spatial and temporal limitations, with the Internet as its main information transmission tool, linking regions together and making the exchange, collection and processing of information more convenient, open and transparent (Li and Liu, 2021). Furthermore, because the production activities of enterprises are closely related to the level of their own production technology, for example, resource-based enterprises, which mainly consume natural resources, cause far more damage to the environment than green enterprises, and thanks to the convenience of the economic model, the digital economy can play a role in promoting the exchange and promotion of green manufacturing technology among enterprises, enhancing the efficiency of enterprise learning, and urging enterprises to monitor each other. It also helps to reduce the average amount of emissions and the cost of managing polluting waste (Han C et al., 2022). Once again, the reusability and non-zero sum nature of the data itself lead to a minimal marginal cost of natural resources consumed in the process of acquisition and exchange, and its low-input, high-yield nature perfectly suits the current government’s requirements for green development. This will accelerate cross-regional cooperation and the outreach of the digital economy, which is conducive to improving regional collaborative governance policies and enhancing environmental quality (Wang et al., 2021). Based on the above analysis, hypothesis 2 is proposed.
H2:. The digital economy can promote the efficiency of synergistic carbon-haze management in neighboring regions through spatial spillover effects.Third, the literatures have pointed out that the development of the digital economy can significantly promote technological innovation, leading to changes in production models. In the context of the digital economy era, the emergence of new technologies such as big data, blockchain, cloud computing and artificial intelligence has accelerated the high-speed flow of technological information between enterprises, stimulating them to constantly learn to imitate new technologies and actively use the latest advanced intelligent equipment to optimize their production processes to maximize the use of natural resources, reduce waste per unit of production, provide a competitive advantage in the marketplace, and help reduce the amount of polluting waste and final emissions generated during the production process (Li et al., 2022). For example, enterprises will combine new digital technologies with traditional industrial and manufacturing industries to analyse whether there is avoidable waste of resources or excessive emissions in the last round of production by looking at the various types of data collected during the production process, seeking the most rational allocation of resources and optimizing the efficiency of the combination of production factors (Meng and Wang, 2021; Ding et al., 2022). In addition, the digitalization of industries can contribute to the change of traditional production models and the optimization of industrial structures, gradually transforming resource-intensive enterprises into knowledge- and technology-intensive ones while fostering new industries, new business models and new economic growth points. The digital economy, as a new development model, can provide a driving force for economic development and industrial upgrading. The establishment of an information technology sharing mechanism through blockchain attracts corporate investment and accelerates the integration of enterprises with the ecosystem, with economic benefits as the core and environmental benefits as a supplement, thus achieving innovative management of the ecological environment and promoting environmental awareness in related fields (Pan et al., 2022). Based on the above analysis, Hypothesis 3 is proposed.
H3:. The digital economy influences the synergistic management of carbon-haze through technological innovation.
To test whether Hypothesis 1 holds, a two-way fixed effects panel data model is constructed (Ding et al., 2022).
where i is individual, t is time, Y is the explanatory variable, and the three variables selected in this paper are carbon haze co-governance efficiency (lncsce), carbon dioxide emissions (lnCO2), and haze concentration (lnsmog); lnde is the level of development of the digital economy; C is other control variables; u and v denote individual and time effects, respectively, and
Furthermore, a spatial Durbin model is constructed on the basis of (1) considering spatial interaction effects to test whether hypothesis 2 holds.
where
Finally, to examine whether technological innovation acts as a mediating variable in this process, the paper constructs a mediating effects model to test hypothesis 3.
where
To comprehensively examine the effect of the digital economy (lncsce) on carbon dioxide emissions as well as haze governance, a super-efficiency SBM model (Supplementary Appendix SC) is used to construct an input–output system (Tone, 2001; Li et al., 2019). Among the input indicators, the total investment in environmental pollution control in each province is selected for measurement in this paper, and this indicator can reflect the overall level of environmental pollution control in the region in that year. Among the expected outputs, the air quality index is chosen for measurement, and there are two kinds of indicators for evaluating air quality, API and AQI, with the latter being more commonly used by meteorological observation stations in China, so the AQI index is chosen in this paper.
As the AQI is daily data, it is necessary to first find the annual average air quality index of each city, and then weight the sum according to the share of the area of the city and the sum of the cities as weights, and then obtain the annual average air quality index of the province for the year, and finally inverse the data after dimensionless processing. For the undesirable output, the provincial CO2 emissions and the provincial haze averages were selected, and the calculation used for provincial CO2 emissions was consistent with the national emission inventory accounting method (Shan et al., 2017). To further refine the impact of the main pollutant PM2.5, the annual average PM2.5 values for the 30 Chinese provinces assigned by the Atmospheric Composition Analysis Group (ACAG) at Dalhousie University on the basis of NASA raster data were chosen for this paper, while the statistical years for the main pollutants of the haze are limited. These data are relatively more current than the publicly available data from Columbia University and provide a more refined count of the major pollutants (Southerland et al., 2022). At the same time, a nonnegative treatment is applied to the efficiency of the carbon and haze co-governance. In addition, carbon dioxide emissions (lnCO2) and mean haze (lnsmog) are also considered as explanatory variables (Yang. et al., 2022a; Yang et al., 2022b).
The Digital Economy Index (lnde), to measure the level of development of the digital economy, is divided into “level of information infrastructure”, “level of digital industrialization” and “level of industrial digitization” (Supplementary Appendix SD).
Information infrastructure reflects the level of demand for information services and the capacity of information services in the region, mainly in terms of the construction of communication hardware facilities, so the length of fiber-optic cable lines and the number of mobile internet users and 4G users are taken into account (Han D et al., 2022).
The level of digital industrialization refers to the scale of ICT-related industries involving e-commerce and software business; therefore, the number of e-commerce transaction enterprises, e-commerce sales, software business revenue, software product revenue, and electronic information manufacturing assets are included in this section (Wang et al., 2021).
The digitalization of industry is mainly reflected in the process management and automated production of enterprises through digital technology, and this series of digitalization processes are all part of technological change. Therefore, expenditures on introducing technology, expenditures on technological improvement, the number of websites per 100 enterprises, and the number of computers per 100 people are taken as variables to be investigated in this section (Li et al., 2022). The weighting of each indicator is based on the entropy method (Supplementary Appendix SE).
The level of economic development (lnpgdp), measured as the natural logarithm of regional GDP per capita, is a variable that reflects the level of regional economic development and the overall level of consumption of the population. In general, regions with a high level of economic development will have cleaner production technologies by manufacturers, which can, to some extent, reduce the pollution of the regional atmosphere from production (Meng and Wang, 2021). However, an increase in consumer demand can also contribute to energy consumption by inducing an increase in the scale of production, which in turn exacerbates pollutant emissions, a factor that potentially affects the efficiency of carbon haze control; therefore, this variable should also be taken into account in the model (Ding et al., 2022).
Population density (lnpd), measured by the natural logarithm of the ratio of the number of permanent residents at the end of the year to the area of the built-up area in that year, is a variable that affects the efficiency of CO2 and haze control through scale and agglomeration effects (Pan et al., 2022). Agglomeration effects can lead to pollution reduction through cost savings and technology spillovers (Zeng and Zhao, 2009; Dong et al., 2020). However, as the size of the economy continues to increase, the scale effect may diminish when production activities exceed the carrying capacity of the environment, thereby exacerbating pollutant emissions and inhibiting the efficiency of carbon haze management. Therefore, the effect of population density on the efficiency of carbon haze management depends on the relative size of the scale and aggregation effects.
The level of external openness (lnfdi) is measured here as the natural logarithm of actual foreign investment utilized in the year. There are two classical opposing hypotheses on the relationship between foreign investment and environmental pollution control: the ‘pollution haven’ hypothesis (Copeland and Taylor, 1994) and the ‘pollution halo’ hypothesis (Dean, 2002). The pollution haven hypothesis suggests that foreign investors prefer to invest in countries or regions with low environmental access standards to avoid the costs of pollution control, thereby increasing environmental pollution in the host country. The halo hypothesis, on the other hand, suggests that green technologies have a spatial spillover effect and can improve environmental governance in host countries. Given these two contrasting accounts, the direction of the impact of foreign direct investment (FDI) on the level of carbon-haze governance is to be determined (Zhao and Wang, 2022).
The level of finance (lnfl), measured here by the natural logarithm of the Digital Inclusive Finance Index from the Digital Finance Research Centre of Peking University, is chosen to show that financial capital has an expansionary effect on energy consumption by stimulating market consumption and manufacturers’ production (Ge L. et al., 2022). However, given the externalities of environmental pollution control, the environmental standards set by regions for manufacturers are becoming more stringent, and therefore, manufacturers need to invest more in R&D in the technology factor, which in turn increases desired output (Mesagan et al., 2022). Therefore, the direction of the impact of financial development on the efficiency of carbon-haze governance is also undetermined.
Environmental regulation intensity (lner), at this stage, is usually assessed through government pollution control costs and environmental taxes, which has the advantage of integrating informal and formal environmental regulation (Shan et al., 2017; Wang et al., 2022). Thus, by using the logarithm of the total investment in environmental pollution control in the current year as a proxy variable, manufacturers’ willingness to emit pollutants will be discouraged when the total investment in environmental control tends to be stringent, thus improving the efficiency of environmental control.
Technological innovation (lntec) is measured by the total number of patent applications granted in the year, as the digital economy itself has a certain technological threshold and is highly knowledge intensive, it is more likely to produce some innovations than other industries, and these achievements can play a catalytic role in the production process, thus improving the overall production efficiency. In addition, the development of the digital economy can lead to the development of digital finance, the promotion of financial technology can also effectively improve the efficiency of enterprise financing, and enterprises can invest more capital in technology factors, while technological innovation is also an important path to reduce pollution emissions, so this indicator is used as a mediating variable (Ma et al., 2022).
Among the explanatory variables, data on carbon dioxide emissions are obtained from the provincial energy inventory of the CEADs database, air quality index and total investment in environmental pollution control are obtained from the global warming database and carbon neutral database of the CSMAR platform, and total investment in pollution control is obtained from the environmental research database of the EPSDATA platform. Among the explanatory variables, the data related to the digital economy index were obtained from the digital economy database of the CSMAR platform. For the control variables and mechanism variables, the data are taken from the regional research database of the EPSDATA platform, and some missing values in the database are filled in from the public data in the official website of the National Bureau of Statistics, with no missing values. Descriptive statistics for the data areshown in Table 1.
First, a baseline regression was conducted based on Model 1) to examine the effects of digital economy development on CO2 emissions, haze pollution and synergistic governance efficiency under individual and temporal two-way fixed effects. Theregression results are shown in Table 2.
TABLE 2. Baseline regression coefficients of the digital economy on the synergistic management of carbon and haze
The regression coefficient of the core explanatory variable of this paper, digital economy (lnde), is negative for both carbon dioxide emissions (lnCO2) and haze averages (lnsmog), indicating that the digital economy has a significant inhibitory effect on both, while the regression coefficient of the digital economy is positive for the efficiency of synergistic carbon-haze management (lncsce), indicating that the development of the digital economy has a significant driving effect, and hypothesis 1 was verified. Further analysis of the controlled variables shows that three indicators, namely, the level of economic development, the level of FDI and the level of finance, are able to suppress carbon dioxide emissions and haze pollution while improving the efficiency of the region’s collaborative carbon-haze management. Specifically, with the development of the economic level, the effect of industrial agglomeration becomes more obvious, and manufacturers produce cleaner technology and cause less damage to the environment. In the case of carbon dioxide and haze pollution, an increase in the level of external openness does not produce a pollution haven effect, and the results are more in favor of the halo hypothesis, which is able to improve the level of environmental governance in the host country through the introduction of foreign investment. In addition, the level of finance can likewise have a dampening effect on carbon-haze management.
The variables different from the digital economy are population density and environmental regulation intensity. Population density has a significant positive effect on CO2 emissions, which indicates that the scale effect of industry formation at this stage exceeds the environmental carrying capacity, and the innovation compensation brought by the agglomeration effect has not significantly changed this situation. The intensity of environmental regulation has a negative effect on the efficiency of synergistic carbon-haze management, a significant positive effect on carbon dioxide emissions, and an insignificant inhibitory effect on haze pollution, which may be due to the large coverage of investment in environmental regulation and the small amount of investment involving carbon dioxide and haze pollution. In addition, pollution control has a lagging effect, and the effect of environmental regulations on the current year’s control may not be obvious. The rising investment in environmental management is also related to the rising indicators of carbon dioxide, respirable particulate matter and three wastes in China, which laterally indicates that there is still room for improving the strength of environmental management at this stage.
To study the spatial structure characteristics of carbon-haze pollution and considering that the research scope of this paper is provincial panel data and the administrative area of each province varies in size, the use of a simple neighboring spatial weight matrix may increase the error. A geographical distance matrix is constructed by combining the diffusion transfer effect of atmospheric particulate matter as well as carbon compounds in the atmosphere. The geographic distance weight matrix is a matrix constructed from spatial distances. The use of a geographic distance matrix helps to distinguish the strength of the interaction between different regions, and the influence between regions tends to gradually decrease as the geographic distance increases. The inverse of the geographical distance between the administrative centers of two provinces (urban areas) is used here. In addition, China’s economic development shows a spatially distributed characteristic of high in the east and low in the west, the level of economic development between provinces may also have a certain correlation in space, and the level of economic development of one region is most likely to be influenced by the spatial dependence of related regions. Therefore, this paper starts from the regional GDP per capita and simultaneously constructs an economic distance weight matrix. The economic distance weight matrix can better describe the economic development differences between regions; the greater the development differences between different geographical units are, the less relevant they are, and the smaller the development differences are, the greater the correlation. The inverse of the average value of regional GDP per capita of each province during the period 2013–2019 is used here. In addition, to take into account both the economic development level and geographical distance indicators, an economic-geographic nested spatial weight matrix is constructed with the coefficient set at 0.5. Meanwhile, all three matrices involved in this paper (the adjacency matrix in the robustness test does not need to be normalized) are normalized.
Accordingly, the main variables were further tested for spatial correlation, and the indices were selected from the classical Moran index. The results are shown in Table 3.
According to Table 3, it can be found that during the period 2013–2019, each of the main variables passed the significance test at the 10% level conditional on the three spatial weight adjacency matrices, and the global Moran index was positive, which indicates that there is a positive spatial correlation between digital economic development, CO2 emissions and the mean values of haze. Therefore, spatial spillover needs to be taken into account in the model, and a spatial econometric regression model is used for analysis.
The SDM can be transformed into a spatial error model (SEM) or a spatial lag model (SLM) under certain conditions. To determine the specific form of the spatial econometric model, the LM test and the Wald test were carried out in turn, and the results showed that both the spatial error model and the spatial lag model passed the LM test, so the spatial Durbin model could be chosen. Further Wald tests were conducted, and the results showed that the original hypothesis was also rejected at the 1% level, indicating that the SDM cannot be reduced to a spatial error model or a spatial lag model. Therefore, the SDM is the optimal choice for this study.
In addition, a Hausman test of the model was needed, and the results showed that both significantly rejected the original hypothesis at the 1% confidence level; therefore, a fixed effects model was selected. Finally, an LR test was carried out, and again, the original hypothesis was rejected, and the estimation results and significance of the time and individual two-way fixed effects were selected. In this paper, the individual and temporal two-way fixed spatial Durbin model with fixed benefits was selected for estimation. Accordingly, regressions were conducted in the case of the geographical distance matrix, the economic distance matrix and the economic-geographical nested matrix, and the results are shown in Table 4.
TABLE 4. Spatial regression coefficients of the digital economy on the synergistic management of carbon and haze
From Table 4, it can be found that the regression coefficients of the digital economy on the synergistic management of carbon-haze are positive and pass the significance test at the 10% level under the three spatial weight matrix settings, while the digital economy has a significant negative effect on both carbon dioxide emissions and haze, which is not different from the baseline regression results. The comprehensive results show that the digital economy can effectively promote the improvement of the efficiency of the synergistic management of carbon-haze, which again supports hypothesis 1. Meanwhile, the lag term coefficients of the digital economy on synergistic carbon haze management have a positive effect of 10% under the geographic distance matrix and the economic-geographic nested matrix and a significant positive effect at the 1% level under the economic distance matrix, indicating that the development of the digital economy has a significant effect on improving the efficiency of carbon-haze management in the surrounding areas, with a significant positive spatial spillover effect. The spatial spillover effect of carbon dioxide emissions and haze averages on neighboring regions is obvious, and the spatial spillover coefficients of carbon and haze collaborative management on neighboring regions are both positive at the 5%, indicating that carbon dioxide and haze pollutants emitted in the region will cause diffuse pollution to neighboring regions with atmospheric flow. The digital economy will not only benefit the management of the local ecological environment but also have a positive effect on other areas within the agglomeration, thus improving the efficiency of the synergistic management of carbon and haze in multiple regions, and there is a spatial spillover effect.
As the spatial Durbin model incorporates the explanatory variables in different geographical units as well as the explanatory variables, where spatial lags can produce some bias in the regression results, the regression coefficients of the explanatory variables do not explain the true situation well, and it is necessary to decompose the coefficients into direct effects, indirect effects and total effects. The direct effect indicates the influence of the explanatory variables in the region on the explanatory variables in the region, the total effect indicates the influence of the explanatory variables in the region and the surrounding regions on the explanatory variables in the region, and the indirect effect indicates the influence of the explanatory variables in the surrounding regions on the explanatory variables in the region. Accordingly, the effects of the model were decomposed, and Table 5 demonstrates the results of the effects of the core variable lnde on each of the explanatory variables.
Analysis of Table 5 shows that the direct, indirect and total effects of the digital economy on carbon-haze management are all significantly positive at the 10% level under the three spatial weight matrices, and the coefficient of the indirect effect is higher than that of the direct effect, indicating the existence of a spatial spillover effect. The coefficient of the indirect effect is higher than the coefficient of the direct effect, indicating the existence of a spatial spillover effect. In contrast, the development of the digital economy in the surrounding areas will also lead to the improvement of the efficiency of the region’s collaborative carbon-haze control.
Considering China’s vast territory, the level of economic development varies greatly from region to region, and the industrial structure varies. This may lead to a certain characteristic of digital economy development in spatial distribution. This paper adopts the kriging interpolation method, logarithmically processing the digital economy index of each province in 2013, 2016 and 2019 after interpolation prediction analysis, considering that the pankriging interpolation method can be well applied to the prediction between discontinuous geographical units, so in the figure, the non-study area of this paper is also taken into account, and the overall still distribution can be seen in Figure 4.
FIGURE 4. Digital economy development in 30 provinces and regions in China, 2013 (A), 2016 (B) and 2019 (C).
According to Figure 4, there are significant spatial differences in the regional distribution of the core explanatory variables in this paper, consistent with the development characteristics of a high east and low west. It is therefore necessary to run subsample regressions for different regions, which are divided into three regions: eastern, central and western4. This section focuses on examining the heterogeneity in regional distribution, whereby a matrix of geographical distance weights is constructed for the eastern, central and western regions, and the two-way fixed spatial Durbin model under all controlled variables is still followed except for matrix changes, the results of which are shown in Table 6.
TABLE 6. The effects coefficients of the digital economy on synergistic management of carbon-haze in different regions.
According to the results in Table 6, the effect of the digital economy on the synergistic management of carbon haze is spatially heterogeneous, with the indirect effect in the eastern region being significantly positive at the 5% level and the coefficient being higher than that in the central and western regions, and the coefficient in the central region passing the significance test at the 10% level and the regression coefficient being higher than that in the western region. The effect of the digital economy on the synergistic governance of carbon-haze is the strongest in the eastern region, with a gradual decrease from east to the west in a stepped pattern. This may be due to the high level of development of the digital economy in the eastern region, where the cost of imitation is low and learning efficiency is high. Different enterprises can promote innovation in their products and production models by imitating the practices of competing enterprises and at the same time sharing the production methods and innovative ideas of high-efficiency enterprises with low-efficiency enterprises through blockchain technology, thereby improving the allocation of market factors, optimizing the efficiency of resource use, and driving the transformation of industrial production intensification. As the level of digital economy development in the eastern region far exceeds that of other regions, there are also differences in digital economy development between the central and western regions, leading to a gradual weakening of the synergistic effect of the digital economy on carbon haze management from east to west, confirming the existence of spatial spillover effects. In addition, the mean coefficients of the digital economy on CO2 emissions and haze in the three regions are positive, while the indirect effects are mostly significantly negative at the 1% level, inferring that the digital economy can suppress air pollutant emissions through spatial spillover effects across the region. In summary, it was possible to confirm hypothesis 2.
Hypotheses 1 and Hypotheses 2 were tested above, namely, that the digital economy has a positive impact on the efficiency of carbon haze governance and has a spatial spillover effect. However, the transmission mechanism is still unclear. Based on the previous theoretical analysis, the regression analysis of Eqs 3–5 in turn is used to examine whether technological innovation acts as a mediating variable in the transmission mechanism, and since this part is mainly about the mechanism of the variable of technological innovation. Therefore, to facilitate the analysis, only the results of the analysis under the economic distance weight matrix are considered. The consideration for this is that the level of technological innovation tends to be closely linked to the level of regional economic development, which is more appropriate compared to the other two matrices, still using the two-way fixed spatial Durbin model. Table 7 shows the regression results for each of the three models.
Without considering the coefficients of spatial spillover effects, the results of model 3) indicate that the regression coefficients of the digital economy and the mean values of CO2 emissions and haze are significantly correlated, and the results of model 4) show that the digital economy can significantly contribute to the development of technological innovation. The results of model 5) show that the sign and significance between the digital economy and the explanatory variables remain unchanged, and technological innovation also has a significant effect on the explanatory variables, so it can be inferred that technological innovation plays the role of a mediating variable in this process. Specifically, the total effect of the digital economy on the efficiency of synergistic carbon and haze management, CO2 emissions and haze mean are 0.444, -0.171 and -0.227, respectively, while the direct effects are 0.328, -0.131 and -0.194, respectively, and the indirect effects are 0.116, -0.040 and -0.033, respectively.
In terms of coefficients that take spatial spillover effects into account, the above findings still hold true, with the sign direction remaining consistent across the board. The development of the digital economy in the surrounding areas (in this case based on a matrix of economic distances) can drive technological innovation in the region, and the effect is more pronounced, probably due to the dividends of regional competition. This may be because the diffusion of carbon haze does not follow an economic distribution spatially, and the effect of distant but similar economic regions on each other’s carbon-haze pollution is limited. Similarly, in terms of specific coefficients, all results are guaranteed to be significant, so it can be inferred that technological innovation also has a mediating effect in space.
Specifically, the total effect of the surrounding region’s digital economy on the efficiency of the region’s synergistic carbon-haze governance, CO2 emissions and haze averages are 0.534, -0.129 and -0.308, respectively, while the direct effects are 0.502, -0.102 and -0.251, respectively, and the indirect effects are 0.032, -0.027 and -0.057, respectively. Simple multiplication of coefficients may result in coefficients that are significant but not actually significant, so a test for mediating effects was conducted using the widely used bootstrap method, with a set sample of 500, and the results were still robust and significant at the 1% level. In summary, hypothesis 3 was tested.
In spatial econometric regression, robustness analysis is generally carried out by replacing the spatial weight matrix. Three common matrices are involved in the study of this paper: the geographical distance weight matrix, the economic distance weight matrix and the economic-geographical nested weight matrix, so the regression analysis of Eq. 2 is carried out using the classical adjacency matrix with the Queen adjacency. Again, all control variables were included in the model. The regression results in Table 8 show that the effects of the digital economy on the efficiency of carbon and haze co-governance, CO2 emissions and haze averages are unchanged in sign. Although the coefficients and significance have changed compared to the other three metrices, they remain convergent overall.
TABLE 8. Coefficients of the impact of the digital economy on the synergistic carbon and haze management efficiency, CO2 emissions and haze mean values with adjacency matrix
This paper is about the relationship between the digital economy and atmospheric respirable particles and CO2, but environmental pollution management involves three aspects: water pollution, air pollution, and soil pollution. Despite the fact that excessive emissions of carbon dioxide have caused a serious greenhouse effect, this indicator is still not classified as an air pollutant. However, the production of industrial waste gas or wastewater requires a certain amount of energy, which in turn increases the combustion of fossil fuels, resulting in the formation of large amounts of CO2 or CO, and some of the soot and respirable particulate matter are also emitted into the atmosphere. Therefore, this paper considers that CO2, respirable particulate matter and industrial emissions are correlated, so the explanatory variables are replaced by the logarithm of industrial emissions, and the parameters are estimated using a two-way fixed spatial Durbin model by choosing an economic-geographic nested matrix with no change in the controlled variables. The regression results are shown in Table 9.
A phased return to different periods of digital economy development, taking the “G20 Digital Economy Development and Cooperation Initiative” proposed at the G20 Summit in Hangzhou in 2016 as the node, classifies the digital economy development before 2016 as the starting stage (2013–2016). At the same time, 2016 was used as a transition year, after which the full development phase of the digital economy (2016–2019) was entered. Considering that the panel is changed to a short panel, only individual effects are fixed, while all control variables are included and parameter estimation is carried out in an economic-geographic nested matrix. The results show that the sign of the coefficients of the digital economy on each of the explanatory variables does not change, among which the efficiency of the synergistic management of carbon-haze in the start-up period fails to pass the test at the 10% level, which may be caused by the small sample size due to the shortened panel. This may be due to the small sample size caused by the shortened panel, but overall, the model is still robust.
Using interprovincial panel data in China during 2013–2019 as a sample, this paper explores the impact of the digital economy on the efficiency of collaborative carbon-haze governance based on theoretical analysis and empirical research by constructing a spatial panel regression model, using a two-way fixed effects model, a spatial Durbin model and a mediating effects model for empirical analysis, and further examining whether technological innovation plays an intermediary role in the spatial transmission process of the digital economy.
The results of the study show that (1) the development of the digital economy can effectively reduce carbon-haze emissions in the production process, which has a positive effect on improving the efficiency of carbon-haze synergistic management. Specifically, for every 1% growth in the digital economy, the efficiency of synergistic carbon and haze management also increases by 0.231%. At the same time, the suppression effects on carbon dioxide and haze are stronger, with their regression coefficients of -1.090 and -0.714 respectively. At the same time, whatever the spatial matrix used, the digital economy can improve the efficiency of carbon-haze co-governance in neighbouring regions through spatial spillover effects. This means that every 1% growth in the local digital economy increases the efficiency of the surrounding area by at least 0.239%. 2) For the region itself, the regression coefficient for technological innovation is 0.295 (p < 0.05). For the surrounding area, this result is 0.212 (p < 0.01). This suggests that technological innovation acts as a mediating variable in the effect of the digital economy on the efficiency of synergistic carbon haze management, taking into account spatial effects. 3) Digital economy development has a significant spatial spillover effect only in the eastern and central regions (the regression coefficients are 0.346 and 0.217 respectively), with the eastern region having the greatest effect on improving the efficiency of synergistic carbon and haze management.
Based on the above findings, this paper makes the following policy recommendations.
First, build an inclusive digital economy and increase the penetration rate of data elements. The digital economy has become a new driving force in reducing air pollutant emissions and curbing the haze phenomenon. Governments at all levels should increase investment in the internet industry and effectively promote the construction of digital China, especially by accelerating the construction of 5G services, big data technology, artificial intelligence and other related infrastructure, to further consolidate the advantages of the information technology dividend and thus achieve the development of a high-quality green and low-carbon economy (Yang et al., 2021a).
Second, reduce the digital divide between regions and breakdown the barriers to digital technology transfer (Ge W. et al., 2022). At present, the level of development of the digital economy in China’s various regions is unbalanced, and geographically, it shows an overall spatial distribution characteristic of high in the east and low in the west. From a global perspective, the spatial spillover effect of the digital economy should be reasonably utilized, and efforts should be made to narrow the gap in the development of the digital economy within the region. At the same time, each local government should introduce relevant policies to actively guide large high-tech enterprises in the region to help small and medium-sized enterprises, play a leading role, and urge leading digital enterprises in the province to provide technical support to less developed areas of the digital economy.
Third, create a digital city system and realize information technology to assist city operations. Transform the government’s concept of digital governance, attach importance to the value of digital assets, improve the efficiency of digital operations, and reasonably revitalize digital dividends. Regional information sharing systems and emergency management mechanisms should be established, atmospheric carbon-haze detection and early warning services should be increased, and highly polluting enterprises should be digitally controlled through monitoring means, thereby scientifically bringing into play the scale and agglomeration effects and improving the efficiency of green urban development (Yang et al., 2022b).
The raw data supporting the conclusion of this article will be made available by the authors, without undue reservation.
BZ: Conceptualization, Methodology, Writing–original draft, Supervision, Funding acquisition. HZ: Methodology, Project administration, Writing–original draft, Writing–review and editing, Data curation. JY: Formal analysis, Writing–original draft. TH: Validation, Investigation, Writing–review and editing. JL: Methodology, Writing–original draft, Writing–review and editing.
This research is funded by the National Social Science Foundation of China (Grant No. 21BJY114) and the Major Project of Basic Research on Philosophy and Social Science in Henan Higher Education (Grant No. 2022-JCZD-35).
The authors would like to acknowledge the reviewers and colleagues for their valuable guidance and helpful comments.
The authors declare that the research was conducted in the absence of any commercial or financial relationships that could be construed as a potential conflict of interest.
All claims expressed in this article are solely those of the authors and do not necessarily represent those of their affiliated organizations, or those of the publisher, the editors and the reviewers. Any product that may be evaluated in this article, or claim that may be made by its manufacturer, is not guaranteed or endorsed by the publisher.
The Supplementary Material for this article can be found online at: https://www.frontiersin.org/articles/10.3389/fenvs.2022.984591/full#supplementary-material
1In 2005, the WHO air quality guidelines set a safe PM2.5 concentration of 10 micrograms per cubic meter, with a reduction to 5 micrograms per cubic meter by 2021.
2Annual average haze concentrations in China only became publicly available after 2013.
3There is still disagreement in the current academic community on the definition of the digital economy, and this study builds on the existing foundation by referring to China’s National Bureau of Statistics, “Statistical Classification of the Digital Economy and its Core Industries (2021)" (China’s National Bureau of Statistics, 2021. (China’s National Bureau of Statistics, 2021. http://www.stats.gov.cn/tjgz/tzgb/202106/t20210603_1818129.html. Accessed 21 June 2022), OECD, 2014. Measuring the Digital Economy: A New Perspective, Pan et al., 2022. Digital economy: An innovation driver for total factor productivity. Journal of Business Research 139, 303–311. and Zhang W et al., 2022. Digital economy and carbon emission performance: Evidence at China’s city level. Energy Policy 165, 112,927. The digital economy refers to a series of economic activities that use data resources as a key production factor, modern information networks as an important carrier, and the effective use of information and communication technology as an important driving force for efficiency improvement and economic structure optimization, mainly including digital development, digital innovation and digital application.
4Eastern region includes: Beijing, Tianjin, Hebei, Shanghai, Zhejiang, Fujian, Shandong, Guangdong, Hainan; Central region includes: Shanxi, Anhui, Jiangxi, Henan, Hubei, Hunan; Western region includes: Inner Mongolia, Guangxi, Chongqing, Sichuan, Guizhou, Yunnan, Tibet, Shaanxi, Gansu, Ningxia, Xinjiang, Liaoning, Jilin, Heilongjiang (Supplementary Figure S5).
Acemoglu, D., Aghion, P., Bursztyn, L., and Hemous, D. (2012). The environment and directed technical change. Am. Econ. Rev. 102 (1), 131–166. doi:10.1257/aer.102.1.131
Baron, R., and Kenny, D. (1986). The moderator-mediator variable distinction in social psychological research: Conceptual, strategic, and statistical considerations. J. Personality Soc. Psychol. 51, 1173–1182. doi:10.1037/0022-3514.51.6.1173
Cao, X., Deng, M., and Li, H. (2021). How does e-commerce city pilot improve green total factor productivity? Evidence from 230 cities in China. J. Environ. Manag. 289, 112520. doi:10.1016/j.jenvman.2021.112520
Chen, W., and Yan, W. (2020). Impact of internet electronic commerce on SO2 pollution: Evidence from China. Environ. Sci. Pollut. Res. 27 (20), 25801–25812. doi:10.1007/s11356-020-09027-1
Copeland, B. R., and Taylor, M. S. (1994). North-south trade and the environment. Q. J. Econ. 109 (3), 755–787. doi:10.2307/2118421
Dean, J. M. (2002). Does trade liberalization harm the environment? A new test. Can. J. Econ. 35 (4), 819–842. doi:10.1111/0008-4085.00155
Ding, C., Liu, C., Zheng, C., and Li, F. (2022). Digital economy, technological innovation and high-quality economic development: Based on spatial effect and mediation effect. Sustainability 14 (1), 216. doi:10.3390/su14010216
Ding, Y., Zhang, M., Chen, S., Wang, W., and Nie, R. (2019). The environmental kuznets curve for PM2.5 pollution in beijing-tianjin-hebei region of China: A spatial panel data approach. J. Clean. Prod. 220, 984–994. doi:10.1016/j.jclepro.2019.02.229
Dong, F., Wang, Y., Zheng, L., Li, J., and Xie, S. (2020). Can industrial agglomeration promote pollution agglomeration? Evidence from China. J. Clean. Prod. 246, 118960. doi:10.1016/j.jclepro.2019.118960
Du, G., Liu, S., Lei, N., and Huang, Y. (2018). A test of environmental Kuznets curve for haze pollution in China: Evidence from the penal data of 27 capital cities. J. Clean. Prod. 205, 821–827. doi:10.1016/j.jclepro.2018.08.330
Ge, L., Zhao, H., Yang, J., Yu, J., and He, T. (2022). Green finance, technological progress, and ecological performance—Evidence from 30 provinces in China. Environ. Sci. Pollut. Res. Int. doi:10.1007/s11356-022-20501-w
Ge, W., Xu, Y., Liu, G., Shen, B., Su, X., Liu, L., et al. (2022). Exploring the impact of the digital economy on carbon emission efficiency under factor misallocation constraints: New insights from China. Front. Environ. Sci. 10. doi:10.3389/fenvs.2022.953070
Han, C., Xu, R., Ye, T., Xie, Y., Zhao, Y., Liu, H., et al. (2022). Mortality burden due to long-term exposure to ambient PM2.5 above the new WHO air quality guideline based on 296 cities in China. Environ. Int. 166, 107331. doi:10.1016/j.envint.2022.107331
Han, D., Ding, Y., Shi, Z., and He, Y. (2022). The impact of digital economy on total factor carbon productivity: The threshold effect of technology accumulation. Environ. Sci. Pollut. Res. 29, 55691–55706. doi:10.1007/s11356-022-19721-x
He, Q. (2015). Fiscal decentralization and environmental pollution: Evidence from Chinese panel data. China Econ. Rev. 36, 86–100. doi:10.1016/j.chieco.2015.08.010
Huang, X., Ding, A., Gao, J., Zheng, B., Zhou, D., Qi, X., et al. (2020). Enhanced secondary pollution offset reduction of primary emissions during COVID-19 lockdown in China.
Jaffe, A. B., Newell, R. G., and Stavins, R. N. (2002). Environmental policy and technological change. Environ. Resour. Econ. 22 (1), 41–70. doi:10.1023/a:1015519401088
Kim, J., and Heo, E. (2014). Effect of ICT capital on the demands for labor and energy in major industries of Korea, US, and UK. Environ. Resour. Econ. Rev. 23, 91–132. doi:10.15266/kerea.2014.23.1.091
Li, J., Chen, L., Chen, Y., and He, J. (2022). Digital economy, technological innovation, and green economic efficiency—empirical evidence from 277 cities in China. MDE. Manage. Decis. Econ. 43 (3), 616–629. doi:10.1002/mde.3406
Li, X., Liu, J., and Ni, P. (2021). The impact of the digital economy on CO2 emissions: A theoretical and empirical analysis. Sustainability 13 (13), 7267. doi:10.3390/su13137267
Li, Y., Chiu, Y.-H., and Lu, L. C. (2019). Energy, CO2, AQI and economic performance in 31 cities in China: A slacks-based dynamic data envelopment analysis. Carbon Manag. 10 (3), 269–286. doi:10.1080/17583004.2019.1589841
Li, Y., Cui, Y., Cai, B., Guo, J., Cheng, T., and Zheng, F. (2020). Spatial characteristics of CO2 emissions and PM2.5 concentrations in China based on gridded data. Appl. Energy 266, 114852. doi:10.1016/j.apenergy.2020.114852
Li Z, Z., Li, N., and Wen, H. (2021). Digital economy and environmental quality: Evidence from 217 cities in China. Sustainability 13 (14), 8058. doi:10.3390/su13148058
Li, Z., and Liu, Y. (2021). Research on the spatial distribution pattern and influencing factors of digital economy development in China. IEEE Access 9, 63094–63106. doi:10.1109/access.2021.3075249
Liu Y, Y., Yang, Y., Li, H., and Zhong, K. (2022). DIgital economy development, industrial structure upgrading and green total factor productivity: Empirical evidence from China’s cities. Int. J. Environ. Res. Public Health 19 (4).2414. doi:10.3390/ijerph19042414
Liu Z, Z., Deng, Z., He, G., Wang, H., Zhang, X., Lin, J., et al. (2022). Challenges and opportunities for carbon neutrality in China. Nat. Rev. Earth Environ. 3 (2), 141–155. doi:10.1038/s43017-021-00244-x
Lorenzoni, I., Nicholson-Cole, S., and Whitmarsh, L. (2007). Barriers perceived to engaging with climate change among the UK public and their policy implications. Glob. Environ. Change 17 (3), 445–459. doi:10.1016/j.gloenvcha.2007.01.004
Ma, Q., Tariq, M., Mahmood, H., and Khan, Z. (2022). The nexus between digital economy and carbon dioxide emissions in China: The moderating role of investments in research and development. Technol. Soc. 68, 101910. doi:10.1016/j.techsoc.2022.101910
Meng, F., and Wang, W. (2021). The influence of factor-biased technological progress on the share of labour income in the digital economy. Technol. Anal. Strateg. Manag., 1–16. doi:10.1080/09537325.2021.1998431
Mesagan, E. P., Akinsola, F., Akinsola, M., and Emmanuel, P. M. (2022). Pollution control in africa: The interplay between financial integration and industrialization. Environ. Sci. Pollut. Res. 29 (20), 29938–29948. doi:10.1007/s11356-021-18489-w
Miao, Z. (2021). Digital economy value chain: Concept, model structure, and mechanism. Appl. Econ. 53 (37), 4342–4357. doi:10.1080/00036846.2021.1899121
Minx, J. C., Baiocchi, G., Peters, G. P., Weber, C. L., Guan, D., and Hubacek, K. (2011). A “carbonizing dragon”: China’s fast growing CO2 emissions revisited. Environ. Sci. Technol. 45 (21), 9144–9153. doi:10.1021/es201497m
Moyer, J. D., and Hughes, B. B. (2012). ICTs: Do they contribute to increased carbon emissions? Technol. Forecast. Soc. Change 79 (5), 919–931. doi:10.1016/j.techfore.2011.12.005
Ozcan, B., and Apergis, N. (2018). The impact of internet use on air pollution: Evidence from emerging countries. Environ. Sci. Pollut. Res. 25 (5), 4174–4189. doi:10.1007/s11356-017-0825-1
Pan, W., Xie, T., Wang, Z., and Ma, L. (2022). Digital economy: An innovation driver for total factor productivity. J. Bus. Res. 139, 303–311. doi:10.1016/j.jbusres.2021.09.061
Peng, G. (2013). Green ICT: A strategy for sustainable development of China’s electronic information industry. China. 11, 68–86. doi:10.1353/chn.2013.0031
Qian, H., Xu, S., Cao, J., Ren, F., Wei, W., Meng, J., et al. (2021). Air pollution reduction and climate co-benefits in China’s industries. Nat. Sustain. 4 (5), 417–425. doi:10.1038/s41893-020-00669-0
Sadorsky, P. (2012). Information communication technology and electricity consumption in emerging economies. Energy Policy 48, 130–136. doi:10.1016/j.enpol.2012.04.064
Shan, Y., Guan, D., Liu, J., Mi, Z., Liu, Z., Liu, J., et al. (2017). Methodology and applications of city level CO2 emission accounts in China. J. Clean. Prod. 161, 1215–1225. doi:10.1016/j.jclepro.2017.06.075
Southerland, V. A., Brauer, M., Mohegh, A., Hammer, M. S., van Donkelaar, A., Martin, R. V., et al. (2022). Global urban temporal trends in fine particulate matter (PM2·5) and attributable health burdens: Estimates from global datasets. Lancet Planet. Health 6 (2), e139–e146. doi:10.1016/s2542-5196(21)00350-8
Su, J., Su, K., and Wang, S. (2021). Does the digital economy promote industrial structural upgrading? a test of mediating effects based on heterogeneous technological innovation. Sustainability 13 (18), 10105. doi:10.3390/su131810105
Sun, X., Chen, Z., Shi, T., Yang, G., and Yang, X. (2021). Influence of digital economy on industrial wastewater discharge: Evidence from 281 Chinese prefecture-level cities. J. Water Clim. Change 13 (2), 593–606. doi:10.2166/wcc.2021.447
Toffel, M. W., and Horvath, A. (2004). Environmental implications of wireless technologies: News delivery and business meetings. Environ. Sci. Technol. 38 (11), 2961–2970. doi:10.1021/es035035o
Tone, K. (2001). A slacks-based measure of efficiency in data envelopment analysis. Eur. J. Operational Res. 130 (3), 498–509. doi:10.1016/s0377-2217(99)00407-5
Wang, D., Zhou, T., Lan, F., and Wang, M. (2021). ICT and socio-economic development: Evidence from a spatial panel data analysis in China. Telecommun. Policy 45 (7), 102173. doi:10.1016/j.telpol.2021.102173
Wang, Q., Du, Z., Wang, B., Chiu, Y.-h., and Chang, T.-H. (2022). Environmental regulation and foreign direct investment attractiveness: Evidence from China provinces. Rev. Dev. Econ. 26 (2), 899–917. doi:10.1111/rode.12871
Wang, X., and Luo, Y. (2020). Has technological innovation capability addressed environmental pollution from the dual perspective of FDI quantity and quality? Evidence from China. J. Clean. Prod. 258, 120941. doi:10.1016/j.jclepro.2020.120941
Xiong, J., and Xu, D. (2021). Relationship between energy consumption, economic growth and environmental pollution in China. Environ. Res. 194, 110718. doi:10.1016/j.envres.2021.110718
Xu, S.-C., Li, Y.-W., Miao, Y.-M., Gao, C., He, Z.-X., Shen, W.-X., et al. (2019). Regional differences in nonlinear impacts of economic growth, export and FDI on air pollutants in China based on provincial panel data. J. Clean. Prod. 228, 455–466. doi:10.1016/j.jclepro.2019.04.327
Xu, S., and Ge, J. (2020). Sustainable shifting from coal to gas in North China: An analysis of resident satisfaction. Energy Policy 138, 111296. doi:10.1016/j.enpol.2020.111296
Xu, S., Wang, Y., Niu, J., and Ma, G. (2020). ‘Coal-to-electricity’ project is ongoing in north China. Energy 191, 116525. doi:10.1016/j.energy.2019.116525
Xu, X., Yang, G., Tan, Y., Zhuang, Q., Tang, X., Zhao, K., et al. (2017). Factors influencing industrial carbon emissions and strategies for carbon mitigation in the Yangtze River Delta of China. J. Clean. Prod. 142, 3607–3616. doi:10.1016/j.jclepro.2016.10.107
Yang, H., Gan, T., Liang, W., and Liao, X. (2022). Can policies aimed at reducing carbon dioxide emissions help mitigate haze pollution? An empirical analysis of the emissions trading system. Environ. Dev. Sustain. 24 (2), 1959–1980. doi:10.1007/s10668-021-01515-9
Yang, X., Su, X., Ran, Q., Ren, S., Chen, B., Wang, W., et al. (2022a). Assessing the impact of energy internet and energy misallocation on carbon emissions: New insights from China. Environ. Sci. Pollut. Res. 29 (16), 23436–23460. doi:10.1007/s11356-021-17217-8
Yang, X., Wang, W., Su, X., Ren, S., Ran, Q., Wang, J., et al. (2022b). Analysis of the influence of land finance on haze pollution: An empirical study based on 269 prefecture-level cities in China. Growth and Change. n/a(n/a). doi:10.1111/grow.12638
Yang, X., Wang, W., Wu, H., Wang, J., Ran, Q., and Ren, S. (2021a). The impact of the new energy demonstration city policy on the green total factor productivity of resource-based cities: Empirical evidence from a quasi-natural experiment in China. J. Environ. Plan. Manag., 1–34. doi:10.1080/09640568.2021.1988529
Yang, X., Wu, H., Ren, S., Ran, Q., and Zhang, J. (2021b). Does the development of the internet contribute to air pollution control in China? Mechanism discussion and empirical test. Struct. Change Econ. Dyn. 56, 207–224. doi:10.1016/j.strueco.2020.12.001
Zeng, D.-Z., and Zhao, L. (2009). Pollution havens and industrial agglomeration. J. Environ. Econ. Manag. 58 (2), 141–153. doi:10.1016/j.jeem.2008.09.003
Zhang, Q.-Y., Cai, B.-F., Wang, M.-D., Wang, J.-X., Xing, Y.-K., Dong, G.-X., et al. (2022). City level CO2 and local air pollutants co-control performance evaluation: A case study of 113 key environmental protection cities in China. Adv. Clim. Change Res. 13 (1), 118–130. doi:10.1016/j.accre.2021.10.002
Zhang W, W., Liu, X., Wang, D., and Zhou, J. (2022). Digital economy and carbon emission performance: Evidence at China's city level. Energy Policy 165, 112927. doi:10.1016/j.enpol.2022.112927
Zhao, C., and Wang, B. (2022). How does new-type urbanization affect air pollution? Empirical evidence based on spatial spillover effect and spatial durbin model. Environ. Int. 165, 107304. doi:10.1016/j.envint.2022.107304
Keywords: digital economy, synergistic carbon-haze governance, spatial spillover effect, mediating effect, CO2
Citation: Zhou B, Zhao H, Yu J, He T and Liu J (2022) Does the growth of the digital economy boost the efficiency of synergistic carbon-haze governance? evidence from China. Front. Environ. Sci. 10:984591. doi: 10.3389/fenvs.2022.984591
Received: 02 July 2022; Accepted: 18 August 2022;
Published: 14 September 2022.
Edited by:
Kevin Cheung, E3-Complexity Consultant, AustraliaReviewed by:
Xiaodong Yang, Xinjiang University, ChinaCopyright © 2022 Zhou, Zhao, Yu, He and Liu. This is an open-access article distributed under the terms of the Creative Commons Attribution License (CC BY). The use, distribution or reproduction in other forums is permitted, provided the original author(s) and the copyright owner(s) are credited and that the original publication in this journal is cited, in accordance with accepted academic practice. No use, distribution or reproduction is permitted which does not comply with these terms.
*Correspondence: Jiawei Liu, MTgzNjExMzY2NzVAMTYzLmNvbQ==
†These authors have contributed equally to this work and share first authorship
Disclaimer: All claims expressed in this article are solely those of the authors and do not necessarily represent those of their affiliated organizations, or those of the publisher, the editors and the reviewers. Any product that may be evaluated in this article or claim that may be made by its manufacturer is not guaranteed or endorsed by the publisher.
Research integrity at Frontiers
Learn more about the work of our research integrity team to safeguard the quality of each article we publish.