- 1Academy of Guangzhou Development, Guangzhou, China
- 2School of Management, Guangzhou University, Guangzhou, China
- 3Nanyang Technological University, Singapore, Singapore
Existing studies have focused on the impact of innovation on carbon emission performance but ignore the importance of government support for innovation. To overcome this challenge, this paper adopts a spatial difference-in-difference (DID) model to assess the impact of government support for innovation on urban carbon emission performance based on a quasi-natural experiment of innovative city pilots (ICP) in China. Using the high-resolution carbon emission data of 1 km × 1 km for 238 cities from 2008 to 2019 in China, this paper employees an extended stochastic frontier analysis (SFA) model to measure urban carbon emission performance. Our findings indicate that ICP implementation leads to a 1.3% improvement in local carbon emission performance. Meanwhile, there is a significant spatial spillover effect of ICP implementation, with a 3.3% improvement in the carbon performance of the surrounding areas. The results of the mechanism analysis suggest that government innovation support affects carbon emission performance by promoting total factor productivity improvement, green innovation, and industrial upgrading. Further analysis shows that ICP has the strongest impact on carbon performance in the eastern region, and the impact is stronger for large cities and resource-dependent cities. Finally, the paper carries out a series of robustness tests to ensure the reliability of the analytical results, including parallel trend tests, placebo tests and re-estimation of different methods. Based on the findings, this paper proposes feasible policy recommendations in terms of continuous promotion of government innovation support, regional cooperation and differentiated innovation support formulation.
1 Introduction
In recent years, the rapid increase in greenhouse gas emissions has exacerbated global warming and extreme climate phenomena, which have seriously threatened the sustainable development of human society (Magazzino, 2017a; Bai et al., 2019; Du and Li, 2019; Chen and Lin, 2020; Statistical Review of World Energy, 2021; He et al., 2021; Li Y et al., 2022, Li Z et al., 2022; Wang K-L et al., 2022; Wu C et al., 2022). As the country with the highest carbon emission in the world, China’s total carbon emission has increased from 1.419 billion tons in 1978 to 9.899 billion tons in 2020, an increase of 6.98 times (BP, 2021). This means that China faces enormous pressure to reduce emissions. In 2020, the Chinese government also proposed the dual carbon goals of “2030 carbon peak” and “2060 carbon neutrality”, aiming to alleviate the climate problems caused by greenhouse gas emissions. Improving carbon emission performance, i.e., the output per unit of carbon emission, is regarded as the most powerful policy instrument to achieve the dual carbon goals. How to improve carbon emission performance has attracted a large number of scholars to study and discuss.
However, the existing literature has not reached a consistent conclusion on the impact of government support for innovation on carbon emission performance. One view is that government support for innovation can effectively improve carbon emission performance. Pan A et al. (2022) selected enterprise-level panel data from 2010 to 2018 to investigate the effect of the pilot carbon emission trading scheme (CETS). He found a significant positive effect of government support on carbon emission performance and total factor productivity based on the PSM-DID model. Doğan et al. (2022) used data from the G7 countries from 1994 to 2004 to study the effects of environmental taxes on carbon emissions, natural resource rents, and renewable and non-renewable resources. He found environmental tax policies can significantly reduce carbon emissions and improve carbon emission performance in these countries. The opposite view is that government support for innovation has a very limited effect on improving carbon emission performance. Fu et al. (2022) adopted a game-theoretical framework to examine firms’ operational strategies under a carbon tax policy. They concluded that carbon taxes do not necessarily lead to the adoption of green technologies and the improvement of carbon emission performance. Yıldırım et al. (2022) empirically investigated the impact of environmental innovation on CO2 emissions in the energy sector based on a large dataset of 32 OECD countries from 1997 to 2018. Using a panel smooth transition regression (PSTR) model, they found that the impact of government innovation on carbon emission performance is unstable at different stages due to rebound effects.
Improving carbon emission performance through technological innovation is an important measure for countries to mitigate climate problems in the future (Adedoyin et al., 2022; Pan X et al., 2022). The motivation of this paper is to comprehensively assess the impact of government innovation support on carbon emission performance. This paper argues that existing research faces three challenges, ignoring these challenges may lead to conflicting views. The first challenge is to select a more effective model to assess carbon emission performance. The most widely used methods are data envelopment analysis (DEA) and stochastic frontier analysis (SFA) (Kumbhakar et al., 2014; Filippini and Hunt, 2015; Kang et al., 2022). DEA based on linear programming ignores unobserved city heterogeneity in carbon emission performance (Filippini and Hunt, 2015). Meanwhile, the traditional SFA method cannot remove individual effects, time effects and unobserved heterogeneity at the same time (Kumbhakar et al., 2014). This can lead to over- or underestimation of city carbon emission performance and interfere with the impact of government support for innovation. The second challenge is to circumvent the endogenous interference of government innovation support. Existing literature generally uses indicators such as government subsidies and tax incentives to measure the government’s support for innovative behavior, but such indicators have a strong correlation with urban economic development (Rawte, 2017; Fu et al., 2022; Tang C et al., 2022). Carbon emission performance is also strongly related to economic development, and the resulting endogenous interference will reduce the reliability of the estimated results. The third challenge is to overcome the effect of spatial factors on the results. There are many industrial clusters in China, which makes the economic development of neighboring cities and carbon emissions have obvious spatial correlation (Liu et al., 2022; Zhang Y et al., 2022). In addition, the talents and technologies attracted by the local government through innovation support also accumulate innovation elements for the surrounding areas, thereby affecting the carbon emission performance of the surrounding areas (Peng H et al., 2021; Gao and Yuan, 2022; Zhao and Sun, 2022). Ignoring the potential impact of spatial factors on carbon emission performance in the evaluation model reduces the reliability of the results. To overcome the above challenges, this paper adopts a spatial difference-in-difference (DID) model and uses a quasi-natural experiment in China to assess the impact of government innovation support on urban carbon emission performance.
The contribution of this paper is mainly in the following three points. First, based on the prefecture-level panel data from 2008 to 2019, this paper adopts the extended SFA model proposed by Kumbhakar et al. (2014) to evaluate carbon emission performance. This approach considers all the time-varying, time-invariant, and city characteristics, which help obtain more reliable calculation of carbon emission performance. Second, this paper assesses the impact of government innovation support on carbon emission performance through a quasi-natural experiment. To explore the role of the government in urban innovation, China has implemented the policy of innovative city pilots (ICP), which is committed to improving the agglomeration of urban innovation elements through government participation. ICP identified Shenzhen as the first pilot city for innovation, and in 2009, 14 cities including Dalian and Qingdao were identified as pilot cities for innovation. From 2010 to 2013, more than 40 innovative city pilots were successively approved. In April 2018, another 17 cities were approved to build national innovative cities, and the number of innovative city pilots increased to 78. This policy has strong exogenous nature and can avoid the interference of endogeneity on the evaluation results to a certain extent. The ICP from China is an incremental reform that provides important lessons for other countries and regions committed to improving carbon emission performance through government innovation support policies. Finally, this paper incorporates spatial factors in the traditional DID model. The results estimated through spatial DID model reduce the interference of spatial factors, which can more reliably assess the impact of government innovation support on carbon emission performance.
The reminder of this study is organized as follows: Section 2 provides the literature review. Section 3 provides the policy background and research hypothesis, Section 4 provides the methods and data, Section 5 provides the results, and Section 6 provides the conclusions, recommendations, and limitations.
2 Literature review
The existing literature focuses on two aspects of carbon emission performance, including the measurement of carbon emission performance and the impact of technological innovation on carbon emission performance. First, in the measurement of carbon emission performance, the previous studies used the indicator of economic output per unit of carbon emissions (Lee et al., 2002; Filippini and Hunt, 2015; Chen et al., 2022; Kang et al., 2022). The higher the value of this indicator, the higher the level of carbon emission performance. However, this indicator is limited in that it ignores the potential influence of other factors on carbon emission performance, such as population and industry level (Kang et al., 2022). To overcome this limitation, recent studies widely use DEA and SFA to measure carbon emission performance (Hua et al., 2007; Filippini and Hunt, 2015; Cao and Wu, 2022; Kang et al., 2022). It solves for the optimal combination of input and output factors, and measures carbon emission performance through the gap between actual carbon emissions and expected carbon emissions of optimal combination (Hua et al., 2007; Choi et al., 2012; Molinos-Senante et al., 2014; Liu et al., 2021; Zhang et al., 2021). However, this approach does not consider the unobserved heterogeneity among cities. The bias is acceptable in small samples. However, for large samples, the overestimation or underestimation due to unobserved heterogeneity must be considered (Filippini and Hunt, 2015; Kang et al., 2022). SFA measures carbon emission performance through extracting the residuals from the stochastic frontier function estimates (Aigner et al., 1977). The closer the regression residuals are to zero, the higher the carbon emission performance. However, the traditional SFA model still cannot separate the unobserved heterogeneity in the residuals. Kumbhakar et al. (2014) proposed an extended SFA model that can separate the time-varying characteristics, time-invariant characteristics, and urban heterogeneity in the residuals simultaneously. Therefore, this extended SFA model will be applied in this paper to evaluate urban carbon emission performance more reliably. As an important tool for climate mitigation, how to improve carbon emission performance is a key academic concern. According to previous studies, economic development, industrial structure, government intervention, the level of financial development and the level of foreign investment are the key factors influencing carbon emission performance (Magazzino, 2016; Ashraf et al., 2020; Song et al., 2021; Li L et al., 2022; Pan A et al., 2022; Wang L et al., 2022).
Second, with the rise of emerging technologies such as industrial robots, big data, cloud computing and artificial intelligence, whether technological innovation can provide new impetus for energy conservation and emission reduction has become a hot research topic (Su et al., 2020; Prasath Kumar et al., 2021; Wang K-L et al., 2021; Li N et al., 2022; Saheb et al., 2022). The improvement of cleaner production technology can reduce carbon emissions in the process of production of enterprises and reduce carbon emissions per unit of output (Zhou and Zhao, 2016; Zhou et al., 2021). Zhang and Liu (2022) and studied the impact of digital finance and green technology innovation on carbon emissions in China and found that technology innovation enhances carbon emission performance. Also, Kuang et al. (2022) explored the impact of green technology innovation and renewable energy investments on reducing carbon emissions and found that in the long-term technology innovation can enhance carbon performance. However, studies have also shown that technology innovations increase the risk of enterprises, and the returns to enterprises are particularly limited (Li W et al., 2022; Su and Fan, 2022). Shaikh and Randhawa (2022) found that open technological innovation can also create risks within the organization that can jeopardize the company’s operations. Wang S et al. (2022) conducted an in-depth study on the behavioral decisions of executive teams and corporate green technology innovation. He suggested that technological innovation is characterized by long cycles, high investments and high risks for companies.
Previous studies show that energy saving, and emission reduction cannot be achieved solely by enterprises themselves through technological innovation (Qiu, 2022; Zhang R et al., 2022). One important reason is that technological innovations that focus on energy efficiency and emission reduction do not bring higher excess returns to companies (Li W et al., 2021). Companies will devote limited resources to more profitable projects (Salmani and Partovi, 2021; Gabdullina et al., 2022). At this point, the government must subsidize and support the innovative behavior of enterprises to reduce the R&D risks of enterprises (Ma and Li, 2021; Fan et al., 2022). Especially for green production technology, government incentives can stimulate the innovation of enterprises to a certain extent. Therefore, it is necessary to pay attention to the role of government innovation support in the improvement of urban carbon emission performance.
3 Policy background and research hypothesis
3.1 Policy background of innovative city pilots
ICP is an important policy proposed by China based on the increasingly competitive international situation (Yang J et al., 2022). This is to enhance the innovation capacity and realize the national development plan. In the future, China hopes to use independent innovation as a driving force to promote the restructuring of the industrial economy and the construction of a sustainable society. In 2008, Shenzhen became the first innovative pilot city. The country leverages Shenzhen’s good innovation capability base to radiate neighboring cities. It is hoped that the leading role of science and technology will be brought into play to achieve an overall improvement in the level of innovation in the region. At the beginning of 2010, 14 more cities, including Dalian and Qingdao, joined the list of pilot innovative cities. With the accelerated expansion of the pilot scale of innovative cities, the number of innovative cities nationwide has reached 78 as of 2018 (see Figure 1). From the lo-cation of the pilot innovative cities, there are a certain number of pilots distributed in the east, middle and west of the country. The relatively economically developed coastal provinces in the east have more pilot innovative cities (Yang Z et al., 2022). In terms of the development of innovative cities, the goal of building innovative cities is gradually evolving from enhancing innovation to restructuring urban industries and building sustainable societies. The country is leveraging the policy advantages of these innovative cities and promoting the synergistic development of innovation levels in the surrounding areas (Gao and Yuan, 2022). Such a trend is important to China’s early entry into the forefront of innovative countries.
3.2 Research hypothesis
In this study, we suppose that government innovation support will enhance local carbon emission performance through three main channels. First, government innovation support will improve local carbon performance by enhancing total factor productivity. It has been documented that government innovation support significantly increases total factor productivity (Pan A et al., 2022). This implies that the output from the given total carbon emissions also increases significantly, leading to an increase in carbon emission performance. Second, government innovation support drives the level of local green innovation and thus enhances carbon performance. Government innovation support can effectively reduce the risk of enterprise innovation and greatly stimulate enterprises’ innovation behavior in energy saving and emission reduction and other green technologies (Lin and Ma, 2022). Thus, green innovation can significantly reduce carbon emissions per unit of output, i.e., lead to the improvement of carbon emission performance. Finally, government innovation support will also promote industrial upgrading, thus improving the urban carbon emission performance. Government innovation support can accelerate the transformation of local enterprises from production and processing to R&D, i.e., industrial upgrading (Su and Fan, 2022). Industrial upgrading leads to a decrease in the share of energy inputs in enterprise production and an increase in the value added of products (You and Zhang, 2022). Therefore, enterprises can achieve higher output with lower resource inputs, which leads to the improvement of urban carbon emission performance. Based on the above analysis, this paper proposes the first research hypothesis.
H1: Government innovation support can improve local carbon emission performance through promoting total factor productivity, green innovation and industrial upgrading.
In addition to influencing local carbon performance, local government innovation support may also affect the carbon emission performance of neighboring regions through spillover effects. Government innovation can effectively attract various innovation factors to cluster locally, such as R&D personnel and R&D funds (Li X et al., 2021). Has mentioned in his study that government environmental support has a significant innovation agglomeration effect. Similarly, this idea was also supported by the study of (Peng W et al., 2021). Neighboring regions can then share the benefits of local innovation agglomeration through technological cooperation. Thus, they can improve their own carbon emission performance. In addition, neighboring regions can provide a broad market for the output of local innovation factors and match technical talents. The resulting industrial upgrading will improve the overall carbon emission performance of the region (Yang and Liu, 2020; Kuang et al., 2022; Yang Z et al., 2022). Therefore, the second hypothesis is proposed in this paper.
H2: There is a significant positive spillover effect of government innovation on the carbon emission performance of neighboring regions.
4 Methods and data
4.1 Spatial difference-in-difference model
This paper employees the ICP as a quasi-natural experiment and adopts the DID model to assess the impact of government support for innovation on urban natural carbon emissions. Selecting the implementation of ICP as dependent variables can reduce the potential interference caused by endogenous problems to a certain extent. In addition, considering the spatial spillover effect of urban carbon emissions (Gao and Yuan, 2022; Zhao and Sun, 2022), this paper further incorporates spatial factors into the traditional DID model, and uses the spatial DID to evaluate the impact of the implementation of ICP on urban carbon emission performance.
In the inclusion of spatial factors, the most widely used methods are Spatial Lag Model (SLM), Spatial Error Model (SEM) and spatial Durbin Model (SDM) (Zhao and Sun, 2022). SLM includes the spatial lag term of the dependent variable in the model. SEM incorporates the spatial lag term of the error term into the model. SDM incorporates both the spatial lag terms of the independent variable and the dependent variable into the model. Considering the robustness, this paper will report the estimated results of these three models in the benchmark analysis. First, the spatial DID model based on SLM is constructed as follows:
where
where
where
Taking the k-th independent variable as the example, the result can be expressed as a partial differential matrix according to the above formula:
The above matrix reflects that the average values of diagonal elements and off-diagonal elements are respectively displayed in the partial differential matrix, and the changes of the independent variables in this region and other regions denote the direct and indirect effects.
4.2 Variable
4.2.1 Dependent variable
Based on the review of existing carbon emission performance assessment approaches, the extended SFA model proposed by Kumbhakar et al. (2014) is adopted in this paper. This model can separate the time-varying inefficiency, time-invariant inefficiency, and urban heterogeneity in the residuals at the same time. The model is specified as follows:
where
4.2.2 Independent variable
ICP is an incremental reform, with six batches of cities implementing ICP. Specifically, 77% of the pilot cities establishment concentrated between 2010 and 2013, including 41, 6, 3 and 10 cities in 2010, 2011, 2012 and 2013, respectively. Only Shenzhen was established in 2008, and the remaining 17 pilot cities were established in 2018. This study adopts the implementation of ICP as the independent variable to assess the effect of government support for innovation on the improvement of urban carbon emission performance. The value is 1 if city i has implemented ICP in year t and 0 if it has not implemented ICP.
4.2.3 Control variable
To assess the impact of ICP on urban carbon emission performance more reliably, this paper incorporates a series of control variables in the model, including the level of economic development (lnrgdp), industrial structure (is), government intervention (gov), the level of financial development (fin) and the level of foreign investment (fdi) (Magazzino, 2017b; Ashraf et al., 2020; Song et al., 2021; Wang B et al., 2021; Pan X et al., 2022; Wang and Huang, 2022; Wang S et al., 2022; Wang W et al., 2022; Wu D et al., 2022). The specific measures of each variable are shown in Table 1. Table 2 further reports the descriptive statistics for each variable.
4.3 Data
This paper is based on the open-source spatial grid monthly dataset of anthropogenic carbon emissions (ODIAC) deduced by the team of Oda et al. (2018). This dataset reports high-resolution carbon emission data of 1 km × 1 km, which is aggregated to form a prefecture-level city panel carbon emission dataset. The period of the sample is from 2008 to 2019. The control variables selected in this paper come from the Chinese City Statistics Database (CCSD) in Chinese Research Data Services (CNRDS) Platform (https://www.cnrds.com/Home/Index#/FinanceDatabase/DB/CCSD) and the China Urban Statistical Yearbook. Since the spatial DID model requires the data structure to be a balanced panel, this paper excludes city samples with missing values in any year. The balanced panel dataset contains 238 cities per year with a total of 2856 samples.
5 Results
5.1 Measurement of carbon emission performance
Figure 2 shows the carbon emission performance maps of cities based on SFA model in 2010, 2015 and 2019. In the same year, the darker color denotes the higher carbon emission performance. In terms of the national carbon emission performance distribution, the average carbon emission performance of northern cities is relatively high in these 3 years. While the carbon emission performance of southern cities is relatively low on average. Meanwhile, the carbon emission performance of coastal cities is on average higher than that of inland cities at similar latitudes. We also found that the pattern of carbon emission performance in China remains roughly the same from 2010 to 2019, but there is an overall increase in carbon emission performance.
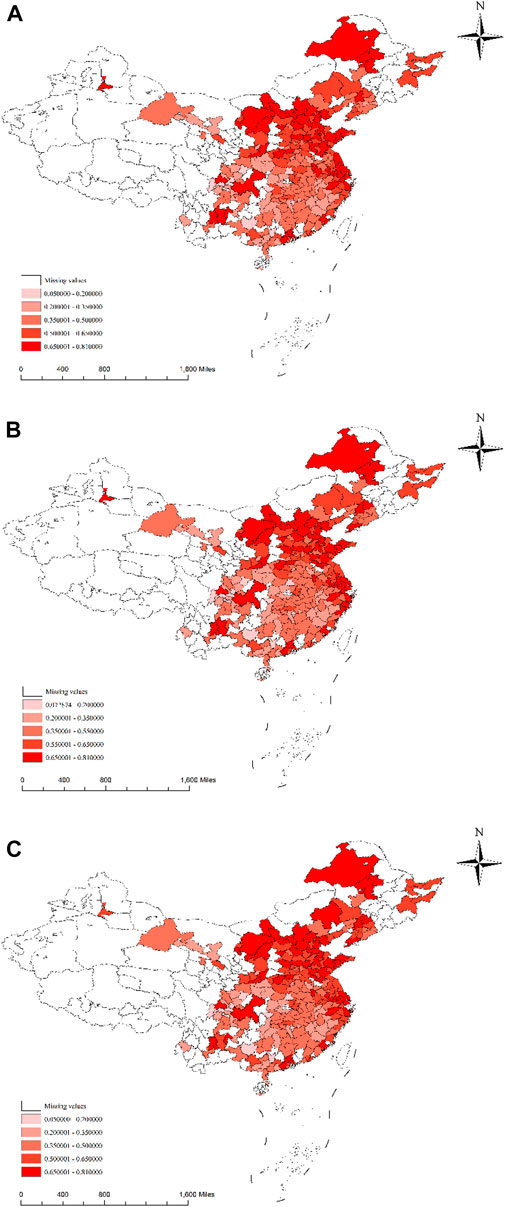
FIGURE 2. Carbon emission performance maps of cities. (A) denotes the maps in 2010, (B) denotes the maps in 2015, and (C) denotes the maps in 2019.
5.2 Spatial autocorrelation test
This paper tested the spatial correlation of carbon emission performance of cities. Scatter plots of Moran index can reflect the spatial correlation of carbon emission performance more visually. Figure 3 shows the scatter plots of carbon emission performance of cities in 2010, 2015 and 2019. In these three plots, the horizontal axis represents the standardized carbon emission performance, and the vertical axis represents the spatial lagged values. The coefficients of the primary fit line according to the scatterplot are significantly smaller than zero, which indicates that there is a spatial negative correlation between the urban carbon emission performance. Table 3 shows the specific results of Moran index of carbon emission performance. The Moran index is significantly negative at the 1% level for the period 2008 to 2019. The values of the indexes are between -1 and 0. This shows that the carbon emission performance of cities in China has a strong spatial correlation. Therefore, spatial factors should be considered in the estimation model.
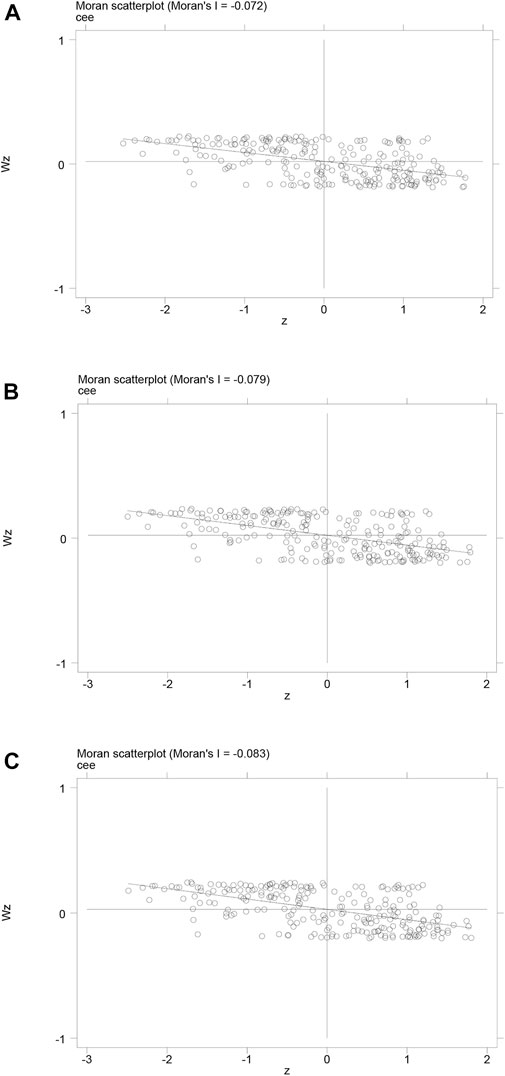
FIGURE 3. Moran scatterplot of urban carbon emission performance. (A) denotes the Moran scatterplot in 2010, (B) denotes the Moran scatterplot in 2010 in 2015, and (C) denotes the Moran scatterplot in 2010 in 2019.
5.3 Impact of innovative city pilots on carbon emission performance
Table 3 reports the baseline regression results. For comparison and to ensure the robustness of the results, here we report the regression results including the fixed effects model, the SLM model, the SEM model, and the SDM model. According to the results, there is a significant positive contribution of ICP policy on carbon emission performance. The coefficients of ICP on carbon emission performance calculated by the four models are 0.9% (p < 0.01), 1.7% (p < 0.01), 1.2% (p < 0.01) and 1.3% (p < 0.01), respectively. Since SDM considers both spatial lag effects and spatial error effects, its assessment of ICP effects is more reliable. Thus, the implementation of ICP leads to a final improvement of urban carbon emission performance by 1.3% after excluding the spatial factor interference.
The results indicates that ICP policy can improve carbon emission performance. Meanwhile, the pilot of innovative cities helps to respond to cities for green development and economic improvement. In terms of other control variables, the effect of GDP per capita on carbon emission performance is significantly negative at the 1% level in all four models. The effect of industrial structure on carbon emission performance is also negative at the 1% level. The effect of government expenditure on carbon emission performance is still significantly negative at the 1% level in all four models. On the contrary, the effect of deposit and loan balances of financial institutions on carbon emission performance is significantly positive at the 1% level in all four models. The effect of actual utilization of foreign finance on carbon emission performance is insignificant.
The estimation results of SDM model show that ICP policy has an important enhancement effect on carbon emission performance. Since ICP pilot cities are distributed across the country and carbon emission performance is also spatially correlated, it is necessary to discuss the spatial spillover effects. Table 5 further reports the spatial spillover effects of ICP policies on carbon emission performance. Specifically, the direct, indirect, and total effects of ICP policy on carbon emission performance improvement are significantly positive at the 1% level. This indicates that ICP policies in the region can significantly contribute to the carbon emission performance of the region firstly, and significantly contribute to the carbon emission performance of other regions. Thus, the average effect of ICP on carbon emission performance is all elevated, which is consistent with the study of Xu et al. (2021). However, the study of Xu et al. (2021) ignored the spatial spillover effect of ICP. This paper holds that the contribution of ICP policy to the carbon emission performance of the region is greater than that for other regions. In addition, some studies use fiscal innovation spending or carbon taxes to measure government innovation support and find that the improvement in carbon performance is not significant (Fu et al., 2022; Yıldırım et al., 2022). This paper argues that the assessment results based on the above indicators may be subject to endogenous interference. In contrast, this paper uses ICP to measure government innovation support, which can reduce the potential interference from endogenous. In conclusion, the government should also pay attention to the demonstration role of pilot cities, which can improve the radiation efficiency by enacting policies such as inter-regional collaboration (De Noni et al., 2017; Tang D et al., 2022). Such policies can promote the overall improvement of carbon emission performance in a larger scale.
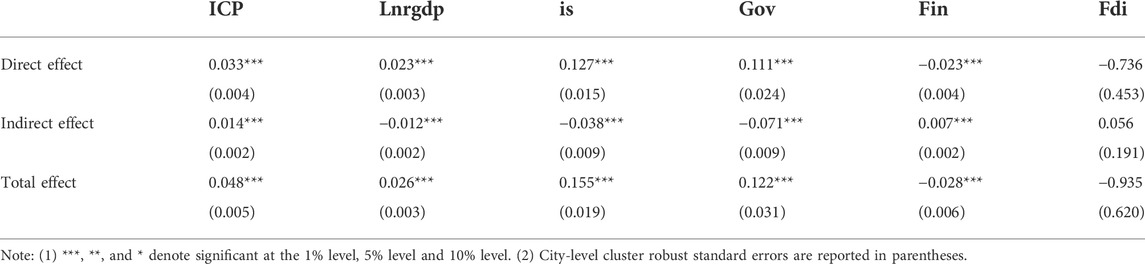
TABLE 5. Direct effect, indirect effect, and total effect of SDM in Table 4.
5.4 Parallel trend test
Figure 4 reports the results of the parallel trend test. None of the regression coefficients passed the significance test before the implementation of the ICP policy. This shows that there is no significant difference between the control and experimental groups before the implementation of the policy. The hypothesis of parallel trend was satisfied. In addition, after the implementation of ICP policy, the regression coefficients showed a trend of in-creasing and then decreasing. This shows that the innovative city pilot policy has the strongest effect in the first 2 years of implementation. And as time passes, the effect of the policy on carbon emission performance starts to decline. This means that in the short term, the pilot innovative cities can bring about an improvement in carbon emission performance, but the effect will gradually diminish. The government should improve the effectiveness of the ICP policy more in the long term while ensuring the short-term performance of the policy.
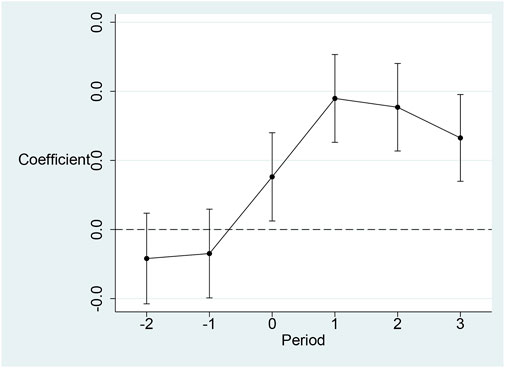
FIGURE 4. Results of Parallel Trend Test. The X-axis denotes the window period for ICP implementation. The Y axis represents the regression coefficient of ICP implementation. The year before ICP is implemented as the base period.
5.5 Further analysis
5.5.1 Mechanism of innovative city pilots affecting urban carbon emission performance
To test hypothesis 1, this paper constructs the following mediating effect model to explore the mechanism of the innovative city pilots affecting urban carbon emission performance:
where Mit denotes the mediating variable, including total factor productivity, green innovation, and industrial upgrading. Total factor productivity is measured through the extended SFA model, where total GDP is the dependent variable and population, government expenditure and foreign investment are the independent variables. Green innovation is measured by the logarithm of the total number of green invention patents and green applicable patents in city i in year t. This paper measures industrial upgrading through the following equation according to the study of Jie and Qian (2016):
where a denotes the share of industry m of city i in the GDP at time t. This indicator denotes the evolution of the proportional relationship between the three major industries in China from the dominance of the primary industry to the dominance of the secondary and tertiary industries. Higher value of this indicator means the higher level of industrial upgrading. If the coefficients of ICP in Eqs. 11 and M in Eq. 12 pass the significance test, it indicates that ICP affects urban carbon emission performance through promoting labor productivity, green innovation, and industrial upgrading. Table 6 reports the regression results for this model.
According to the results in Table 6, the impact of ICP on tfp, green_inn and iu are 0.252 (p < 0.01), 1.376 (p < 0.01) and 0.191 (p < 0.01), which all pass the 1% significance test. This shows that government innovation support can significantly improve the urban total factor productivity, green innovation, and industrial upgrading, which are consistent with the study of Xu et al. (2021) and Zheng and Ge (2022). In addition, the results hold that the coefficients of tfp, green_inn and iu are 0.018 (p < 0.01), 0.008 (p < 0.01) and 0.033 (p < 0.01). This means that there are significant mechanisms for ICP to improve urban carbon emission performance through the promotion of urban total factor productivity, green innovation, and industrial upgrading, that is, the results support hypothesis 1. Therefore, the government should not only strengthen its support for technological innovation, but also further improve the market allocation of production factors and strengthen the positive impact of the above three mechanisms (Shen et al., 2021; Xi and Mei, 2022).
5.5.2 Heterogeneity analysis
There are huge differences in development between different regions in China. In terms of economic development level, the eastern region is higher than the western region in the central region (Dai and Mischke, 2014). As a government-led financial support policy, there may be differences in the intensity and effectiveness of ICP implementation in different economic development regions. Therefore, it is necessary to analyze the differences in the impact of ICP on different regions. Second, the impact effect of ICP is also related to the size of cities. The larger the city has a more complex and well-developed industrial system, the higher the scale effect of ICP implementation will be (Pan A et al., 2022). Therefore, the differences in the impact of ICP on the carbon emission performance of cities of different sizes should be further explored. Finally, the resource-dependent cities of cities are also factors to be considered. Compared with resource-based cities, non-resource-based cities consume less energy and have lower upside of carbon emission performance from ICP (Sun et al., 2022). Therefore, this paper further evaluates the differences in the impact of ICP on cities of different resource types.
Table 7 reports the results of the heterogeneity analysis of ICP policies on carbon emission efficiency. The first row of Table 7 is the baseline row, and the effects of ICP policies on carbon emission performance are reported from left to right for eastern regional cities, small cities, and non-resource cities, respectively. In terms of geographic location, the impact of ICP policy on carbon emission performance for eastern cities is 1.9%. The effect of ICP policy on carbon emission performance in central cities is significantly lower compared to eastern cities, which is only 0.1%. And the improvement effect of ICP policy on carbon emission performance in western cities is not significantly different from that in eastern cities. This indicates that the government should pay attention to the efficiency of the role of ICP policies in central cities, while ensuring the continued improvement of carbon emission performance in the east and west. The synergistic green development of the country’s eastern, central, and western cities should be advocated. The size of cities al-so makes a difference in the impact of ICP policies on carbon emission performance. For small cities, ICP policies can significantly improve carbon emission performance by 0.9%. This improvement is more pronounced in large cities. The level of improvement in carbon emission performance for ICP policies in large cities is 1.7%, which is nearly twice as high as in small cities.
The results indicate that the country should pay attention to the radiative effect brought by large cities while paying attention to the development of green innovation in large cities. The large cities should be the center of a more efficient synergy of policy implementation in the surrounding small cities (Li X et al., 2022). Which will promote the synergistic enhancement of technological innovation and green development in a wider range of cities through the extensive layout of ICP policies. The difference between resource-based cities and non-resource-based cities is obvious. The specific setting of resource-based cities is based on the total amount of carbon emissions. Here we set the cities with higher total carbon emissions as resource-based cities using the data of total carbon emissions of different cities. Conversely, the remaining ones are non-resource-based cities. The study of Kang et al. (2022) points out that the focus of energy efficiency and emission reduction is on less efficient regions, but does not further assess the differences in the impact of government innovation support on cities with different resource types. The effect of ICP policy for non-resource-based cities on carbon emission performance is insignificant. In contrast, the ICP policy of resource-based cities has a 2% enhancement effect on carbon emission performance. On the one hand, this indicates that resource-based cities are uniquely positioned to improve their carbon emission performance based on improved technological innovation, which is consistent with the findings of Zheng and Ge (2022). On the other hand, this paper suggests that government needs to improve the effectiveness of ICP policies in non-resource-based cities. According to the study of Kang et al. (2022), better policy guidance and implementation are needed to promote the carbon emission performance of all types of cities.
5.6 Robustness test
5.6.1 Placebo test
Considering ICP policies may also affect the carbon emission performance of non-pilot cities, this would lead to unreliable estimation results. In this paper, a placebo test is conducted using Monte Carlo simulation. Firstly, we randomly selected samples from the control group multiple times as the treatment group. Then, based on this we then perform PSM-DID regression analysis and estimate the parameters. If the estimated parameters are normally distributed with a mean value of 0, then the results of the analysis in this paper are reliable. Figure 5 gives the estimated coefficient distributions and kernel density curves after 500 randomly drawn samples. As expected from the placebo test, the estimated coefficients show a normal distribution, and the mean value is around 0. This shows that the change in carbon emission performance of the real treated group originates from the implementation of the ICP policy.
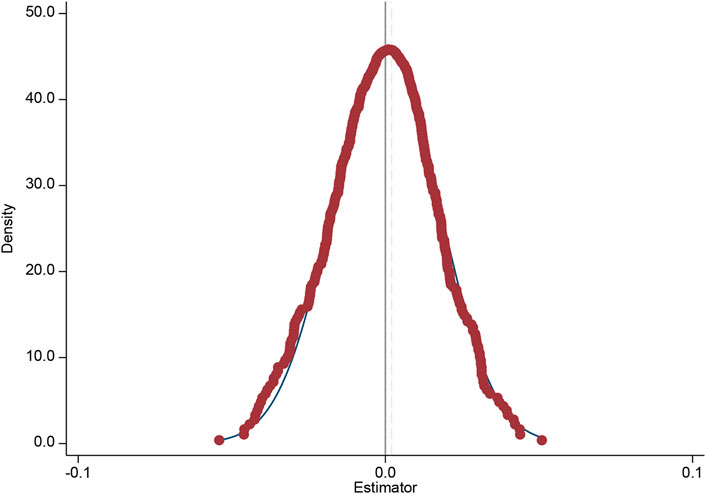
FIGURE 5. Results of placebo test. Treatment groups were randomly drawn 500 times in the control group by Monte Carlo simulation and DID regression was performed. Plot the obtained regression coefficients as a distribution graph. This figure reports the results of carbon emission performance of non-pilot cities as a dependent variable, presenting a normal distribution with an average value of 0.
5.6.2 Re-estimation using PSM-DID
In our previous study, we used a spatial panel regression. Based on this paper we obtained a positive result and concluded that the contribution of ICP policy on carbon emission performance is significant. To strengthen the robustness of the study, here we modify the methodology. Instead of using a spatial panel, we use the PSM method for post-matching regressions. In applying the PSM method, we used two conventional matching methods, namely 1:1 nearest neighbor matching and kernel density matching. Table 8 reports the regression results after matching using these two methods. The results show that the ICP policy can significantly improve the carbon emission performance of cities by either using nearest neighbor matching or kernel density matching. This is consistent with the results of the previous study using spatial panels. The results of this paper are robust.
5.6.3 Re-estimation of different dependent variable
To avoid the potential influence of variable settings on the estimation results, this paper also chooses to measure the carbon emission performance of cities by taking the logarithm of GDP per unit of carbon emissions. Table 9 reports the re-estimation results for the replaced dependent variables. Table 9 shows that the effects of ICP policy on urban carbon emission performance under the fixed effects model, SLM model, SEM model and SDM model are 4.9%, 3.7%, 3.8%, and 3.4%, respectively. And all the coefficients passed the 1% significance test. This implies the regression results of replacing individual explanatory variables remain consistent with those of the previous paper. The conclusions of this paper are relatively re-liable.
5.6.4 Re-estimation excluding contemporaneous policy disturbances
To exclude the interference of other contemporaneous policies on the analysis results of this paper, this paper further controls for low carbon city pilot (LCCP) and carbon emission trading pilot (CETP) policy shocks in the model (Chen et al., 2021; Cui et al., 2021). After adding the above two policy shocks, the results of the impact of ICP on urban carbon emission performance are shown in Table 10. According to the results in Table 10, the coefficients of ICP and LCCP are 0.009 (p < 0.01) and 0.001 (p > 0.1) in column (1). The coefficients of ICP and CETP are 0.009 (p < 0.01) and 0.011 (p < 0.01) in column (2). The above results show that controlling for LCCP and CETP separately, ICP still has a significant positive effect on carbon emission performance. This shows that the conclusion that government innovation support enhances carbon emission performance is robust.
5.6.5 Re-estimation based on an expanded SDID approach
Chagas et al. (2016) proposed a spatial DID method that can decompose the treatment effects of the spatial weight matrix. We used this method for re-estimation to ensure the reliability of the analytical results in this paper. The results are shown in the following Table 11.
According to the results in Table 11, the coefficients of ICP and wICP in restricted model are 0.016 (p < 0.01) and 0.067 (p < 0.01), which both passes the 1% significance test. In addition, the coefficients of WT,TICP and WNT,TICP in restricted model are 0.028 (p < 0.01) and 0.081 (p < 0.01), which also passed the 1% significance test. This means that the implementation of ICP policy not only significantly improves the local carbon emission performance, but also enhance the carbon emission performance of the surrounding areas. Consistent conclusions are obtained based on the extended SDID model estimation proposed by Chagas et al. (2016), indicating the robustness of the analytical results in this paper.
6 Conclusion and recommendations
6.1 Conclusion
This paper uses a spatial DID model to assess the effect of government innovation support on urban carbon emission performance based on a quasi-natural experiment of ICP from China. The main findings of this paper can be summarized in the following three points.
First, this paper measures urban carbon emission performance through the extended SFA model proposed by (Kumbhakar et al., 2014). The measurement results indicate that the average urban carbon emission in China from 2008 to 2019 is 49.7%, and there is still much room for improvement. In addition, our findings indicate that there is a significant spatial correlation in urban carbon emission performance, and that the carbon emission performance in northern and coastal regions is much higher than that in central and western regions.
Second, the estimation results of the spatial DID indicate that the implementation of ICP leads to a 1.3% improvement in the urban carbon emission performance. Meanwhile, the implementation of ICP also leads to a 3.3% improvement in the urban carbon emission performance of the surrounding areas. The total effects of carbon emission performance improvement from ICP implementation are 4.8%. The results shows that government innovation support not only significantly improves local carbon emission performance, but also has a positive spatial spillover effect.
Third, the results of mechanism analysis show that government innovation support enhances urban carbon performance mainly through three mechanisms, namely total factor productivity improvement, green innovation, and industrial upgrading. This paper also conducts a heterogeneity analysis for cities of different regions, sizes, and resource dependencies. The results show that there is no significant difference in the contribution of ICP to carbon performance between eastern and western cities, while the effect of ICP in central cities is relatively low. Meanwhile, the increase of ICP on carbon performance in large cities reaches almost twice that of small cities. In addition, we also observe that ICP in resource-based cities have a significant increase on carbon performance, while ICP in non-resource-based cities have no significant effect on carbon performance.
Finally, a series of robustness tests were conducted to ensure the reliability of the analysis results. The parallel trend test showed that there was no significant difference between the carbon emission performance of the treatment group and the control group before the implementation of ICP, while the carbon emission performance of the treatment group was significantly higher than that of the control group after the implementation of ICP. Therefore, the assessment results of spatial DID are relatively reliable. Meanwhile, the placebo test, re-estimation based on PSM-DID and re-estimation by replacing the dependent variable all yielded more consistent conclusions. This paper further controls for two policies, low-carbon pilot cities and carbon emissions trading pilot, respectively. The results show that after controlling for the above two policies ICP still has a significant positive impact on urban carbon emission performance.
This paper highlights the important role of government innovation support in improving urban carbon performance. Future research can further explore whether the effect of government innovation support differs across firms with different characteristics through micro data of firms. In addition, there is necessary to provide more assessments of the emission reduction effects of different types of government innovation support.
6.2 Recommendations
Based on the findings of the study, this paper puts forward the following recommendations.
First, government innovation support should be increased to improve carbon emission performance. China has become the country with the highest total carbon emissions in the world. The key to achieving peak and neutral carbon targets lies in the control of total emissions from high carbon sectors and the control of overall sectoral emissions performance. The findings of this paper suggest that the implementation of ICP not only significantly improves local carbon emission performance, but also has significant spillover effects on neighboring regions. Therefore, the role of innovation support in pollution control should be better utilized. On the one hand, government innovation support should focus on traditional sectors such as oil, steel and construction. Promote the improvement of carbon emission performance of traditional sectors through financial subsidies and tax incentives. On the other hand, government innovation support also needs to foster frontier industries such as carbon capture and storage. These industries can absorb carbon emissions from traditional sectors, thus effectively improving the overall carbon performance of the region.
Then, for economies with differences in regional development, such as China, differentiated innovation support policies should be developed for different regions. China’s economic development is characterized by a more developed eastern coastal region and a more backward central and western region. As a result, the eastern region has been the first to complete industrial upgrading and transformation and has higher carbon performance. While the central and western regions have taken over part of the industrial transfer from the eastern regions, and their carbon emission performance is low. If the similar innovation support policy is adopted nationwide, it will inhibit the willingness of the central and western regions to improve their carbon emission performance through green innovation. Therefore, it is necessary for the government to give stronger incentives to the central and western regions to gather innovation factors to improve their carbon emission performance. The findings of this paper show that ICP has no significant enhancing effect on carbon emission performance in the western region. There are also differences in the effects of ICP with different city sizes and resource dependence. Therefore, the government should consider its own geographic environment, city size and resource dependencies when providing innovation support. For example, for western cities such as Xining and Lanzhou, the implementation of ICP may not be effective in improving carbon emission performance. In contrast, for cities such as Shanghai, Nanjing, or Hangzhou, ICP can significantly improve carbon emission performance. In addition, government can balance such regional differences through the setting of carbon emission trading allowances. For heavy industries in resource-based cities, such as mining and smelting, allow them to obtain higher carbon quotas through green technology innovation. This would further amplify the effect of government innovation support on the carbon performance of such regions.
6.3 Limitations
The study in this paper also has limitations, and further research can be extended in the following ways. First, due to the lack of firm-level carbon emission data, this paper only assesses the impact of government innovation support on carbon emission performance at the city level. Further research can explore the impact of government innovation from a more microscopic perspective by quantifying firm-level carbon emissions. Second, this paper focuses on the impact of government innovation support in a sample of developing countries represented by China. Further research can compare the differences in the impact of government innovation support on carbon performance across countries at different stages of development.
Data availability statement
Publicly available datasets were analyzed in this study. This data can be found here: https://www.nies.go.jp/doi/10.17595/20170411.001-e.html.
Author contributions
CY: Conceptualization; Data curation; Methodology; Writing—original draft. CT: Funding acquisition; Supervision; Validation; Project administration. HL: Writing—review and editing; Software; Resources. YT: Writing—review and editing. YZ: Writing—review and editing. CZ: Writing—review and editing.
Funding
This work was supported by National Social Science Fund of China (Grant No. 19CGL008).
Conflict of interest
The authors declare that the research was conducted in the absence of any commercial or financial relationships that could be construed as a potential conflict of interest.
Publisher’s note
All claims expressed in this article are solely those of the authors and do not necessarily represent those of their affiliated organizations, or those of the publisher, the editors and the reviewers. Any product that may be evaluated in this article, or claim that may be made by its manufacturer, is not guaranteed or endorsed by the publisher.
Appendix A
Table A1 reports the regression results for the stochastic frontier function. The regression coefficients of economic aggregate (
References
Adedoyin, F. F., Erum, N., and Ozturk, I. (2022). Does higher innovation intensity matter for abating the climate crisis in the presence of economic complexities? Evidence from a global panel data. Technol. Forecast. Soc. Change 181, 121762. doi:10.1016/j.techfore.2022.121762
Aigner, D., Lovell, C. A. K., and Schmidt, P. (1977). Formulation and estimation of stochastic frontier production function models. J. Econ. 6, 21–37. doi:10.1016/0304-4076(77)90052-5
Ashraf, N., Comyns, B., Tariq, S., and Chaudhry, H. R. (2020). Carbon performance of firms in developing countries: The role of financial slack, carbon prices and dense network. J. Clean. Prod. 253, 119846. doi:10.1016/j.jclepro.2019.119846
Bai, C., Du, K., Yu, Y., and Feng, C. (2019). Understanding the trend of total factor carbon productivity in the world: Insights from convergence analysis. Energy Econ. 81, 698–708. doi:10.1016/j.eneco.2019.05.004
Cao, L., and Wu, Y. (2022). Inequality of pollutant discharge in an urban agglomeration and nonurban agglomeration-evidence from a new Theil-DEA model. Environ. Sci. Pollut. Res. 29, 21876–21890. doi:10.1007/s11356-021-17405-6
Chagas, A. L. S., Azzoni, C. R., and Almeida, A. N. (2016). A spatial difference-in-differences analysis of the impact of sugarcane production on respiratory diseases. Regional Sci. Urban Econ. 59, 24–36. doi:10.1016/j.regsciurbeco.2016.04.002
Chen, H., Guo, W., Feng, X., Wei, W., Liu, H., Feng, Y., et al. (2021). The impact of low-carbon city pilot policy on the total factor productivity of listed enterprises in China. Resour. Conservation Recycl. 169, 105457. doi:10.1016/j.resconrec.2021.105457
Chen, J., Hu, X., Huang, J., and Lin, R. (2022). Market integration and green economic growth-recent evidence of China's city-level data from 2004-2018. Environ. Sci. Pollut. Res. 29, 44461–44478. doi:10.1007/s11356-022-19070-9
Chen, Y., and Lin, B. (2020). Slow diffusion of renewable energy technologies in China: An empirical analysis from the perspective of innovation system. J. Clean. Prod. 261, 121186. doi:10.1016/j.jclepro.2020.121186
Choi, Y., Zhang, N., and Zhou, P. (2012). Efficiency and abatement costs of energy-related CO2 emissions in China: A slacks-based efficiency measure. Appl. Energy 98, 198–208. doi:10.1016/j.apenergy.2012.03.024
Cui, J., Wang, C., Zhang, J., and Zheng, Y. (2021). The effectiveness of China's regional carbon market pilots in reducing firm emissions. Proc. Natl. Acad. Sci. U.S.A. 118, e2109912118. doi:10.1073/pnas.2109912118
Dai, H., and Mischke, P. (2014). Future energy consumption and emissions in east-, central- and west-China: Insights from soft-linking two global models. Energy Procedia 61, 2584–2587. doi:10.1016/j.egypro.2014.12.253
De Noni, I., Ganzaroli, A., and Orsi, L. (2017). The impact of intra- and inter-regional knowledge collaboration and technological variety on the knowledge productivity of European regions. Technol. Forecast. Soc. Change 117, 108–118. doi:10.1016/j.techfore.2017.01.003
Doğan, B., Chu, L. K., Ghosh, S., Diep Truong, H. H., and Balsalobre-Lorente, D. (2022). How environmental taxes and carbon emissions are related in the G7 economies? Renew. Energy 187, 645–656. doi:10.1016/j.renene.2022.01.077
Du, K., and Li, J. (2019). Towards a green world: How do green technology innovations affect total-factor carbon productivity. Energy Policy 131, 240–250. doi:10.1016/j.enpol.2019.04.033
Fan, R., Wang, Y., Chen, F., Du, K., and Wang, Y. (2022). How do government policies affect the diffusion of green innovation among peer enterprises? - an evolutionary-game model in complex networks. J. Clean. Prod. 364, 132711. doi:10.1016/j.jclepro.2022.132711
Filippini, M., and Hunt, L. C. (2015). Measurement of energy efficiency based on economic foundations. Energy Econ. 52, S5–S16. doi:10.1016/j.eneco.2015.08.023
Fu, K., Li, Y., Mao, H., and Miao, Z. (2022). Firms' production and green technology strategies: The role of emission asymmetry and carbon taxes. Eur. J. Operational Res. doi:10.1016/j.ejor.2022.06.024
Gabdullina, G., Gilmanov, M., Akhmetgareeva, A., Khusainova, S., Khamidullin, M., and Gareeva, G. (2022). Assessment of the resource utilization efficiency of transport companies. Transp. Res Procedia 63, 1055–1060. doi:10.1016/j.trpro.2022.06.106
Gao, K., and Yuan, Y. (2022). Government intervention, spillover effect and urban innovation performance: Empirical evidence from national innovative city pilot policy in China. Technol. Soc. 70, 102035. doi:10.1016/j.techsoc.2022.102035
He, K., Ramzan, M., Awosusi, A. A., Ahmed, Z., Ahmad, M., and Altuntaş, M. (2021). Does globalization moderate the effect of economic complexity on CO2 emissions? Evidence from the top 10 energy transition economies. Front. Environ. Sci. 9, 778088. doi:10.3389/fenvs.2021.778088
Hua, Z., Bian, Y., and Liang, L. (2007). Eco-efficiency analysis of paper mills along the Huai River: An extended DEA approach. Omega 35, 578–587. doi:10.1016/j.omega.2005.11.001
Jie, C., and Qian, Z. (2016). The synergy effects of city size and industrial structure on urban labor productivity in China. J. Finance Econ. 42, 75–86. doi:10.16538/j.cnki.jfe.2016.09.007
Kang, J., Yu, C., Xue, R., Yang, D., and Shan, Y. (2022). Can regional integration narrow city-level energy efficiency gap in China? Energy Policy 163, 112820. doi:10.1016/j.enpol.2022.112820
Kuang, H., Akmal, Z., and Li, F. (2022). Measuring the effects of green technology innovations and renewable energy investment for reducing carbon emissions in China. Renew. Energy 197, 1–10. doi:10.1016/j.renene.2022.06.091
Kumbhakar, S. C., Lien, G., and Hardaker, J. B. (2014). Technical efficiency in competing panel data models: A study of Norwegian grain farming. J. Prod. Anal. 41, 321–337. doi:10.1007/s11123-012-0303-1
Lee, J.-D., Park, J.-B., and Kim, T.-Y. (2002). Estimation of the shadow prices of pollutants with production/environment inefficiency taken into account: A nonparametric directional distance function approach. J. Environ. Manag. 64, 365–375. doi:10.1006/jema.2001.0480
LeSage, J., and Pace, R. K. (2009). Introduction to spatial econometrics. New York: Chapman and Hall/CRC. doi:10.1201/9781420064254
Li, L., Li, M., Ma, S., Zheng, Y., and Pan, C. (2022). Does the construction of innovative cities promote urban green innovation? J. Environ. Manag. 318, 115605. doi:10.1016/j.jenvman.2022.115605
Li, N., Feng, C., Shi, B., Kang, R., and Wei, W. (2022). Does the change of official promotion assessment standards contribute to the improvement of urban environmental quality? J. Clean. Prod. 348, 131254. doi:10.1016/j.jclepro.2022.131254
Li, W., Xu, J., Ostic, D., Yang, J., Guan, R., and Zhu, L. (2021). Why low-carbon technological innovation hardly promote energy efficiency of China? - based on spatial econometric method and machine learning. Comput. Industrial Eng. 160, 107566. doi:10.1016/j.cie.2021.107566
Li, X., Lai, X., and Zhang, F. (2021). Research on green innovation effect of industrial agglomeration from perspective of environmental regulation: Evidence in China. J. Clean. Prod. 288, 125583. doi:10.1016/j.jclepro.2020.125583
Li, Y., Zhang, Y., Pan, A., Han, M., and Veglianti, E. (2022). Carbon emission reduction effects of industrial robot applications: Heterogeneity characteristics and influencing mechanisms. Technol. Soc. 70, 102034. doi:10.1016/j.techsoc.2022.102034
Li, Z., Wu, B., Wang, D., and Tang, M. (2022). Government mandatory energy-biased technological progress and enterprises' environmental performance: Evidence from a quasi-natural experiment of cleaner production standards in China. Energy Policy 162, 112779. doi:10.1016/j.enpol.2022.112779
Lin, B., and Ma, R. (2022). Green technology innovations, urban innovation environment and CO2 emission reduction in China: Fresh evidence from a partially linear functional-coefficient panel model. Technol. Forecast. Soc. Change 176, 121434. doi:10.1016/j.techfore.2021.121434
Liu, J., Li, X., and Zhong, S. (2022). Does innovation efficiency promote energy consumption intensity? New evidence from China. Energy Rep. 8, 426–436. doi:10.1016/j.egyr.2022.05.096
Liu, Z., Xu, J., Wei, Y., Hatab, A. A., and Lan, J. (2021). Nexus between green financing, renewable energy generation, and energy efficiency: Empirical insights through DEA technique. Environ. Sci. Pollut. Res., 1–14. doi:10.1007/s11356-021-17092-3
Ma, H., and Li, L. (2021). Could environmental regulation promote the technological innovation of China's emerging marine enterprises? Based on the moderating effect of government grants. Environ. Res. 202, 111682. doi:10.1016/j.envres.2021.111682
Magazzino, C. (2017a). Economic growth, CO2 emissions and energy use in the south caucasus and Turkey: A PVAR analyses. Int. Energy J. 16.
Magazzino, C. (2017b). The relationship among economic growth, CO2 emissions, and energy use in the APEC countries: A panel VAR approach. Environ. Syst. Decis. 37, 353–366. doi:10.1007/s10669-017-9626-9
Magazzino, C. (2016). The relationship among real GDP, CO2 emissions, and energy use in south caucasus and Turkey. Int. J. Energy Econ. Policy 6, 672–683.
Mele, M., and Magazzino, C. (2020). A Machine Learning analysis of the relationship among iron and steel industries, air pollution, and economic growth in China. J. Clean. Prod. 277, 123293. doi:10.1016/j.jclepro.2020.123293
Molinos-Senante, M., Hernández-Sancho, F., Mocholí-Arce, M., and Sala-Garrido, R. (2014). Economic and environmental performance of wastewater treatment plants: Potential reductions in greenhouse gases emissions. Resour. Energy Econ. 38, 125–140. doi:10.1016/j.reseneeco.2014.07.001
Pan, A., Zhang, W., Shi, X., and Dai, L. (2022). Climate policy and low-carbon innovation: Evidence from low-carbon city pilots in China. Energy Econ. 112, 106129. doi:10.1016/j.eneco.2022.106129
Pan, X., Pu, C., Yuan, S., and Xu, H. (2022). Effect of Chinese pilots carbon emission trading scheme on enterprises' total factor productivity: The moderating role of government participation and carbon trading market efficiency. J. Environ. Manag. 316, 115228. doi:10.1016/j.jenvman.2022.115228
Peng, H., Shen, N., Ying, H., and Wang, Q. (2021). Can environmental regulation directly promote green innovation behavior?-- based on situation of industrial agglomeration. J. Clean. Prod. 314, 128044. doi:10.1016/j.jclepro.2021.128044
Peng, W., Yin, Y., Kuang, C., Wen, Z., and Kuang, J. (2021). Spatial spillover effect of green innovation on economic development quality in China: Evidence from a panel data of 270 prefecture-level and above cities. Sustain. Cities Soc. 69, 102863. doi:10.1016/j.scs.2021.102863
Prasath Kumar, S., Ravindiran, A., Meganathan, S., Oral Roberts, N., and Anbarasi, N. (2021). Swarm robot materials handling paradigm for solar energy conservation. Mater. Today Proc. 46, 3924–3928. doi:10.1016/j.matpr.2021.02.402
Qiu, R. (2022). Carbon tax policy-induced air travel carbon emission reduction and biofuel usage in China. J. Air Transp. Manag. 10. doi:10.1016/j.jairtraman.2022.102241
Rawte, R. (2017). The role of ICT in creating intelligent, energy efficient buildings. Energy Procedia 143, 150–153. doi:10.1016/j.egypro.2017.12.663
Saheb, T., Dehghani, M., and Saheb, T. (2022). Artificial intelligence for sustainable energy: A contextual topic modeling and content analysis. Sustain. Comput. Inf. Syst. 35, 100699. doi:10.1016/j.suscom.2022.100699
Salmani, Y., and Partovi, F. Y. (2021). Channel-level resource allocation decision in multichannel retailing: A U.S. Multichannel company application. J. Retail. Consumer Serv. 63, 102679. doi:10.1016/j.jretconser.2021.102679
Shaikh, I., and Randhawa, K. (2022). Managing the risks and motivations of technology managers in open innovation: Bringing stakeholder-centric corporate governance into focus. Technovation 114, 102437. doi:10.1016/j.technovation.2021.102437
Shen, F., Liu, B., Luo, F., Wu, C., Chen, H., and Wei, W. (2021). The effect of economic growth target constraints on green technology innovation. J. Environ. Manag. 292, 112765. doi:10.1016/j.jenvman.2021.112765
Song, W., Mao, H., and Han, X. (2021). The two-sided effects of foreign direct investment on carbon emissions performance in China. Sci. Total Environ. 791, 148331. doi:10.1016/j.scitotenv.2021.148331
Statistical Review of World Energy (2021). Energy economics | home [WWW document]. Bp Glob. URL https://www.bp.com/en/global/corporate/energy-economics/statistical-review-of-world-energy.html (accessed 624, 22).
Su, Y., and Fan, Q. (2022). Renewable energy technology innovation, industrial structure upgrading and green development from the perspective of China's provinces. Technol. Forecast. Soc. Change 180, 121727. doi:10.1016/j.techfore.2022.121727
Su, Y., Yu, Y., and Zhang, N. (2020). Carbon emissions and environmental management based on big data and streaming data: A bibliometric analysis. Sci. Total Environ. 733, 138984. doi:10.1016/j.scitotenv.2020.138984
Sun, J., Xue, J., and Qiu, X. (2022). Has the sustainable energy transition in China's resource-based cities promoted green technology innovation in firms? Socio-Economic Plan. Sci. 101330, 101330. doi:10.1016/j.seps.2022.101330
Tang C, C., Qiu, P., and Dou, J. (2022). The impact of borders and distance on knowledge spillovers - evidence from cross-regional scientific and technological collaboration. Technol. Soc. 70, 102014. doi:10.1016/j.techsoc.2022.102014
Tang D, D., Li, Y., Zheng, H., and Yuan, X. (2022). Government R&D spending, fiscal instruments and corporate technological innovation. China J. Account. Res. 15, 100250. doi:10.1016/j.cjar.2022.100250
Wang B, B., Liu, F., Lin, W., Ma, Z., and Xu, D. (2021). Energy-efficient collaborative optimization for VM scheduling in cloud computing. Comput. Netw. 201, 108565. doi:10.1016/j.comnet.2021.108565
Wang K-L, K.-L., Xu, R.-Y., Zhang, F.-Q., and Cheng, Y.-H. (2022). Reinvestigating the spatiotemporal differences and driving factors of urban carbon emission in China. Front. Environ. Sci. 10. doi:10.3389/fenvs.2022.880527
Wang, K.-L., Zhao, B., Ding, L.-L., and Miao, Z. (2021). Government intervention, market development, and pollution emission efficiency: Evidence from China. Sci. Total Environ. 757, 143738. doi:10.1016/j.scitotenv.2020.143738
Wang L, L., Zeng, T., and Li, C. (2022). Behavior decision of top management team and enterprise green technology innovation. J. Clean. Prod. 367, 133120. doi:10.1016/j.jclepro.2022.133120
Wang S, S., Ren, H., Liang, L., Li, J., and Wang, Z. (2022). The effect of economic development on carbon intensity of human well-being: Evidence from spatial econometric analyses. J. Clean. Prod. 364, 132632. doi:10.1016/j.jclepro.2022.132632
Wang W, W., Xiao, W., and Bai, C. (2022). Can renewable energy technology innovation alleviate energy poverty? Perspective from the marketization level. Technol. Soc. 68, 101933. doi:10.1016/j.techsoc.2022.101933
Wang, Y., and Huang, Y. (2022). Impact of foreign direct investment on the carbon dioxide emissions of east asian countries based on a panel ARDL method. Front. Environ. Sci. 10. doi:10.3389/fenvs.2022.937837
Wu C, C., Su, N., Guo, W., and Wei, W. (2022). Import competition and the improvement in pollutant discharge from heterogeneous enterprises: Evidence from China. J. Environ. Manag. 310, 114809. doi:10.1016/j.jenvman.2022.114809
Wu D, D., Geng, Y., Zhang, Y., and Wei, W. (2022). Features and drivers of China's urban-rural household electricity consumption: Evidence from residential survey. J. Clean. Prod. 365, 132837. doi:10.1016/j.jclepro.2022.132837
Xi, Q., and Mei, L. (2022). How did development zones affect China's land transfers? The scale, marketization, and resource allocation effect. Land Use Policy 119, 106181. doi:10.1016/j.landusepol.2022.106181
Xu, L., Fan, M., Yang, L., and Shao, S. (2021). Heterogeneous green innovations and carbon emission performance: Evidence at China's city level. Energy Econ. 99, 105269. doi:10.1016/j.eneco.2021.105269
Yang, C., and Liu, S. (2020). Spatial correlation analysis of low-carbon innovation: A case study of manufacturing patents in China. J. Clean. Prod. 273, 122893. doi:10.1016/j.jclepro.2020.122893
Yang J, J., Xiong, G., and Shi, D. (2022). Innovation and sustainable: Can innovative city improve energy efficiency? Sustain. Cities Soc. 80, 103761. doi:10.1016/j.scs.2022.103761
Yang Z, Z., Yuan, Y., and Zhang, Q. (2022). Carbon emission trading scheme, carbon emissions reduction and spatial spillover effects: Quasi-experimental evidence from China. Front. Environ. Sci. 9, 824298. doi:10.3389/fenvs.2021.824298
Yıldırım, D. Ç., Esen, Ö., and Yıldırım, S. (2022). The nonlinear effects of environmental innovation on energy sector-based carbon dioxide emissions in OECD countries. Technol. Forecast. Soc. Change 182, 121800. doi:10.1016/j.techfore.2022.121800
You, J., and Zhang, W. (2022). How heterogeneous technological progress promotes industrial structure upgrading and industrial carbon efficiency? Evidence from China's industries. Energy 247, 123386. doi:10.1016/j.energy.2022.123386
Zhang, M., Li, L., and Cheng, Z. (2021). Research on carbon emission efficiency in the Chinese construction industry based on a three-stage DEA-Tobit model. Environ. Sci. Pollut. Res. 28, 51120–51136. doi:10.1007/s11356-021-14298-3
Zhang, M., and Liu, Y. (2022). Influence of digital finance and green technology innovation on China's carbon emission efficiency: Empirical analysis based on spatial metrology. Sci. Total Environ. 838, 156463. doi:10.1016/j.scitotenv.2022.156463
Zhang, R., Tai, H., Cheng, K., Zhu, Y., and Hou, J. (2022). Carbon emission efficiency network formation mechanism and spatial correlation complexity analysis: Taking the Yangtze River Economic Belt as an example. Sci. Total Environ. 841, 156719. doi:10.1016/j.scitotenv.2022.156719
Zhang, Y., Zhao, X., and Fu, B. (2022). Impact of energy saving on the financial performance of industrial enterprises in China: An empirical analysis based on propensity score matching. J. Environ. Manag. 317, 115377. doi:10.1016/j.jenvman.2022.115377
Zhao, M., and Sun, T. (2022). Dynamic spatial spillover effect of new energy vehicle industry policies on carbon emission of transportation sector in China. Energy Policy 165, 112991. doi:10.1016/j.enpol.2022.112991
Zheng, H., and Ge, L. (2022). Carbon emissions reduction effects of sustainable development policy in resource-based cities from the perspective of resource dependence: Theory and Chinese experience. Resour. Policy 78, 102799. doi:10.1016/j.resourpol.2022.102799
Zhou, W., Pian, R., Yang, F., Chen, X., and Zhang, Q. (2021). The sustainable mitigation of ruminal methane and carbon dioxide emissions by co-ensiling corn stalk with Neolamarckia cadamba leaves for cleaner livestock production. J. Clean. Prod. 311, 127680. doi:10.1016/j.jclepro.2021.127680
Keywords: carbon emission performance, innovative city pilots, spital difference-in-difference model, stochastic frontier analysis, spatial spillover effect
Citation: Yu C, Long H, Tu C, Tan Y, Zang C and Zhou Y (2022) Assessing the effectiveness of innovative city pilots in improving urban carbon emission performance: A spatial difference-in-difference approach. Front. Environ. Sci. 10:983711. doi: 10.3389/fenvs.2022.983711
Received: 01 July 2022; Accepted: 05 September 2022;
Published: 04 October 2022.
Edited by:
Wendong Wei, Shanghai Jiao Tong University, ChinaReviewed by:
Xue Qihang, Shandong University, ChinaYantuan Yu, Guangdong University of Foreign Studies, China
Copyright © 2022 Yu, Long, Tu, Tan, Zang and Zhou. This is an open-access article distributed under the terms of the Creative Commons Attribution License (CC BY). The use, distribution or reproduction in other forums is permitted, provided the original author(s) and the copyright owner(s) are credited and that the original publication in this journal is cited, in accordance with accepted academic practice. No use, distribution or reproduction is permitted which does not comply with these terms.
*Correspondence: Yu Zhou, eXVfemhvdUBnemh1LmVkdS5jbg==
†These authors have contributed equally to this work