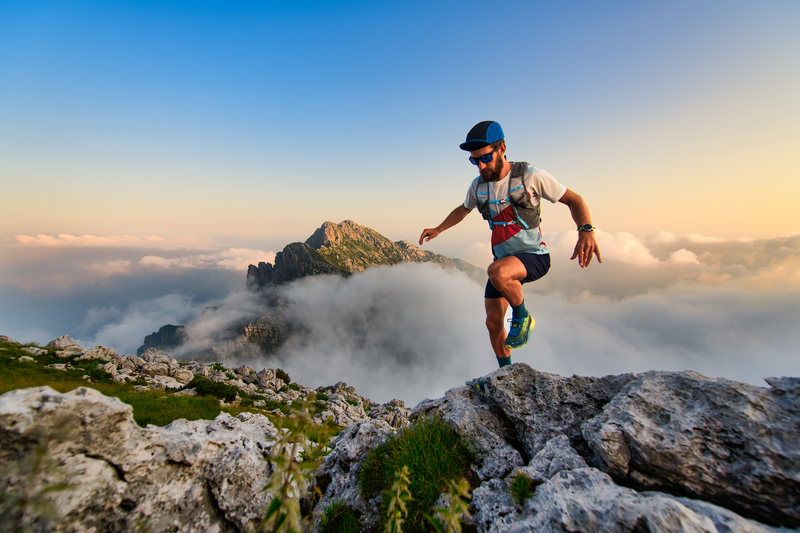
94% of researchers rate our articles as excellent or good
Learn more about the work of our research integrity team to safeguard the quality of each article we publish.
Find out more
ORIGINAL RESEARCH article
Front. Environ. Sci. , 14 September 2022
Sec. Environmental Economics and Management
Volume 10 - 2022 | https://doi.org/10.3389/fenvs.2022.978955
This article is part of the Research Topic ESG Investment and Its Societal Impacts View all 28 articles
Digital transformation, as an important way to optimize the existing resources of enterprises, is a driving force to achieve their high-quality development. Intangible capital is used to reflect the status of digital transformation of enterprises, so as to explore the relationship between the decision of enterprises on digital transformation and the ESG scoring effect of enterprises under financing constraints. The results show that when enterprises are faced with strong financing constraints, the management’s decision on digital transformation tends to be conventional. Although maintaining the traditional business model is more beneficial, it is not conducive to the improvement of ESG score. To further analyze the digital transformation mechanism of enterprises, this paper examines the decision-making behavior of enterprises under financing constraints from the perspective of intermediary mechanism. The results show that the decision of increasing intangible capital of enterprises promotes the improvement of enterprise performance, and then drives the promotion of the enterprise ESG score. This study enriches the research achievements in the field of digital transformation of enterprises, and provides reference for heterogeneous enterprises to select digital transformation decisions.
The input with information technology and data as key elements has become an important way for the transformation, upgrade and leapfrog development of enterprises. Since the Fifth Plenary Session of the 18th CPC Central Committee put forward the “implementation of the national big data strategy”, relevant departments have issued corresponding support policies to promote the digital transformation of enterprises. By the end of 2020, the digitalization rate of production equipment of industrial enterprises above designated size has reached 49.4%1. To realize digital transformation, enterprises need to invest more resources in IT, digital transformation and talents (Li et al., 2020). General Purpose Technologies input in digital transformation often need a lot of other complementary investments, such as business process redesign, new product and business model development and related human capital investment (Brynjolfsson et al., 2021). These complementary investments are often invisible and difficult to measure, and it is difficult to fully reflect their business performance and enterprise value. Accounting methods failing to reflect intangible capital significantly reduce the vitality of enterprises and markets (Farhi and Gourio, 2018; Autor et al., 2020; Crouzet and Eberly, 2021). However, according to the major ESG evaluation systems in the world, all ESG evaluation systems include information technology related options. Therefore, is there a correlation between the increase of intangible capital brought by digital transformation and the ESG score? The existing research rarely deals with this topic.
ESG involves a wide range of contents in Environmental, Social and Governance. In order to accurately obtain the evaluation of E, S, and G, corresponding digital transformation is also required, such as adding corresponding software and information systems, etc (Jun et al., 2022). Emerging technologies such as artificial intelligence have driven society into the era of digital economy, and its foothold lies in the transformation and application of enterprise digitalization. The disclosure of digitization-related information is a form of intellectual capital disclosure that can provide investors with potentially value-related information (Ricci et al., 2020), especially contributing to the improvement of ESG scores. In an era where society attaches great importance to ESG, poor performance of ESG means that companies do not pay enough attention to environmental and social responsibility, and corporate governance needs to be further improved at all stages (Thomas, 1995). The ESG score obviously represents its market prospects, and with the advancement of the digitalization process, the flow of internal resources within the enterprise is smoother and the information is more transparent (Wang Xiaohong et al., 2022), and the ESG score is correspondingly higher. From the perspective of the social responsibility of economic entities, digital transformation will also deeply empower enterprises to fulfill their social responsibilities, thereby generating social spillover effects through more and better corporate social responsibility. Digital transformation can not only enhance the willingness of enterprises to fulfill their social responsibilities, but also improve the ability of an enterprise to fulfill its social responsibilities and to enhance the fulfillment of corporate social responsibilities by improving the enterprise management mechanism, improving the production methods of the enterprise, and improving the external relations of the enterprise (Shen Minghao, 2022).
Will the large amount of unaccounted intangible capital invested in the process of digital transformation affect the enterprise ESG score? How to measure the intangible capital invested in the process of enterprise digital transformation? Whether intangible capital has synergy with tangible capital and its influence on the enterprise ESG score has become the focus of academic circles. To analyze the above problems, the academic circles have carried out relevant research, which mainly focuses on the measurement of intangible capital, the influence of intangible capital on enterprise performance and the choice of intangible capital.
First, the meaning and measurement of intangible capital. Intangible capital is usually defined as the intangible resources that enter the production process (Yang and Shi, 2018), mainly including software, intellectual property, brand and innovative business processes (Crouzet and Eberly, 2019). It is highly correlated with tangible capital (McGrattan and Prescott, 2014) and is one of the important driving forces of business activities. In the process of enterprise digital transformation, in addition to the equipment related to digital equipment, enterprises also need intangible resources such as management and training, business process transformation, information network and corporate culture, which have increased intangible capital after accounting treatment (Xiang and Tian, 2014). Therefore, the intangible capital discussed in this paper refers to the result of accounting treatment of intangible resources invested by enterprises in the period of digital transformation. It is used to depict the underestimation of the potential enterprise ESG score due to the failure to accurately reflect the value of intangible capital in financial statements.
According to the traditional accounting standards, the balance sheet cannot reflect the value brought by new business processes or other types of intangible capital. Intangible capital has been considered to be particularly elusive, and its depreciation rate is unknown, so it is difficult to adopt the traditional methods for evaluating capital stock (Tambe et al., 2020). How to measure intangible capital is one of the core issues concerned by academic circles. Throughout the existing research, the main methods to measure intangible capital are: adopting enterprise knowledge capital and organizational capital (Peters and Taylor, 2017), Tobin Q (Brynjolfsson et al., 2021), IT investment measurement (Tambe et al., 2020), and input-output (McGrattan, 2020). The above methods are mainly based on the sources of intangible capital, and measured from two dimensions including input and output (Xu et al., 2018). Although these two methods of measuring intangible capital complement each other and improve the accuracy of intangible capital assessment, it is difficult to reflect the various intangible complementary investments invested by enterprises in the process of digital transformation in the three major statements. For example, Peters and Taylor (2017) measured intangible capital in the fields of R&D, management, sales, etc. Although it can be found in financial statements, the measurement involving other intangible investments still needs further study.
Second, the influence of intangible capital on enterprise performance. Intangible capital, such as software and information systems, is becoming increasingly important to the profits of enterprises, and the expansibility of intangible capital makes it easier for enterprises to gain competitive advantage (Autor et al., 2020; Akcigit and Ates, 2021). The influence of intangible capital on enterprise performance can be summarized from two aspects. On the one hand, intangible capital can improve enterprise productivity by increasing industry concentration and market forces (Crouzet and Eberly, 2018; Crouzet and Eberly, 2019); on the other hand, enterprises adopt more intangible capital and scale-biased technology brought by the progress of information technology, which forms the “superstar” effect (Autor et al., 2020), and realizes the steady growth of profits. Intangible capital not only affects the business performance of enterprises, but also affects the macroeconomic quality. It is an important source of productivity and economic growth (Yang and Shi, 2018). According to the research of Crouzet and Eberly (2021), intangible capital affects the accuracy of total factor productivity in the United States, especially when the wrong measurement of intangible capital and the increase of bonus rate together cause a downward deviation of one-third to two-thirds of total factor productivity growth in the United States. Based on this, intangible capital investment affects enterprise performance, and more resources can be invested in the process of enterprise ESG construction when enterprise performance is improved, thus improving enterprise ESG score.
Third, the choice of intangible capital investment of enterprises. Although some studies have shown that the input of intangible capital can help enterprises improve their performance, not all types of intangible assets can achieve enterprise performance improvement (Chappell and Jaffe, 2018). The existing research on the choice of intangible capital of enterprises mainly focuses on two aspects. On the one hand, it is considered that the intangible assets of enterprises are puzzled by financing constraints, because under the financial constraints, enterprises can obtain additional cost bonus through intangible capital, thus distorting the decision of enterprises on investment in intangible capital (Altomonte et al., 2021). The availability of financing is the key factor for enterprises to invest in intangible capital; On the other hand, the choice of intangible capital is faced with the opportunity cost of balancing with tangible capital. As the intangible investment of enterprises is as important as the tangible investment (Corrado et al., 2009), the study of American companies by McGrattan & Prescott (2014) confirms that intangible investment is highly correlated with tangible investment. Therefore, in the face of uncertain external environment, the management of enterprises is faced with certain choices of the intangible capital investment required for digital transformation.
To sum up, the existing researches mainly focus on the problems of intangible capital and business performance. Although some researches begin to involve the choice of intangible capital investment, there is a lack of consideration on the choice of intangible capital investment, the synergy between intangible capital and tangible capital, and even less research on the choice of intangible capital investment from the level of business pressure. Therefore, compared with the existing literature, the marginal contribution of this paper may lie in: First, analyzing the influence of digital transformation and enterprise ESG score from the perspective of the choice of intangible capital investment of enterprises. Especially, considering the influence of the decision of enterprises on digital transformation on the enterprise ESG score under the background of financing constraints; Secondly, using the interaction between intangible capital and fixed assets to express the synergy between them after the enterprise makes decision on digital transformation, and measuring the influence of digital transformation on the enterprise ESG score from the perspective of capital synergy after the digital transformation of enterprises. Using the interaction between intangible capital and fixed assets to measure the effect of digital transformation of enterprises. Compared with that using a single intangible capital, it can better reflect the collaborative transportation result of intangible capital and fixed assets after decision-making of digital transformation made by enterprise management, and its conclusion may be more convincing. Thirdly, using the ratio of intangible capital to fixed assets to measure the decision-making behavior of enterprises in digital transformation. The ratio of intangible capital to fixed assets is divided into three quantile groups according to 25, 50, and 75%, which are multiplied by the financing constraints of enterprises, respectively, to depict the dynamic changes of intangible capital selection of heterogeneous enterprises, and to show the influence of the changing state of intangible capital on the ESG score of enterprise performance in the process of digital transformation.
To describe the decision of enterprises on digital transformation, this study adopts the general equilibrium model, integrates the operating pressure, financing constraints and choices of intangible capital investment into the model, and discusses the effects of operating pressure, financing constraints and intangible capital investment choices on enterprise performance. To analyze this problem, this paper studies the three-sector model, as follows:
Drawing lessons from Altamonte et al. (2021) to describe the consumer behavior pattern. It is assumed that the representative household consumption income is
Where,
As far as enterprise sector is concerned, its decisions on production are made as follows: 1) The enterprise keeps the traditional technology at the ratio of
In terms of the decision on maintaining traditional technology or making investment in new technology, the enterprise management needs to consider the business pressure. Under the pressure of operation, enterprises are more likely to choose leverage financing. Studies have shown that the management’s choice of leverage ratio is based on the judgment of the enterprise’s future development. If there are good projects, the enterprise needs to raise funds externally (Harris and Raviv, 1991). Generally speaking, the greater the business pressure faced by an enterprise, the greater the possibility that the management will consider investing in new technologies, and the greater the possibility of financing through financial institutions. Assuming that the cost function of an enterprise is expressed as:
In addition to business pressure, which will affect the management’s decision-making, corporate financing constraint itself is also an important factor affecting management’s choice of new technology investment. Generally speaking, the weaker the corporate financing constraint, the easier it is for the management to raise funds to invest in new technologies. Let
Faced with financing constraints, to increase investment in new technologies, enterprises can raise funds from the financial sector through mortgage. Assuming that the initial investment assets of an enterprise are
According to the principle of supply and demand of family sector, bank sector and enterprise sector, the conditions for profit maximization of enterprise sector can be expressed as follows:
According to the profit maximization function of enterprises,
On both sides of Eq. 8, the derivative of
Inference 1: the weaker the financing constraint
Deriving from both sides of Eq. 8 about
Inference 2: considering that other conditions remain unchanged, the greater the intangible capital investment ratio caused by the decision of enterprises on digital transformation, the more conducive it is to promote the ESG information disclosure of enterprises.
In a volatile, uncertain, complex and ambiguous digital environment, unplanned digital initiatives are infeasible, and the lack of clear strategic goals and practical paths will lead to unsatisfactory digital transformation of enterprises (Amit & Han, 2017; Lichtenthaler, 2020). However, considering the high frequency of unexpected events in the process of digital transformation (Nambisan et al., 2017), it is not feasible to strictly follow the strategic plan and achieve digital transformation by avoiding risks. Especially when the management plays the role of an agent, on the one hand, the management is hired and assessed by the principal, and has the motivation to improve the company’s ESG through digital means and achieve the improvement of the company’s performance; on the other hand, when the company faces financing constraints, The digital transformation of the management is faced with the “dilemma” of choosing, that is to “break the boat” to carry out digital transformation or maintain the original model? In summary, the digital decision-making process for management is shown as Figure 1.
The research objects of this paper are A-share listed companies. The data comes from Wind Information Financial Terminal Database, covering the period from 2017 to 2020. To avoid data interference to the accuracy of research conclusions, according to the usual practice of existing research, the data processing methods of this paper are as follows: 1) Delete ST, *ST and the stocks to be delisted; 2) Delete financial listed companies; 3) Delete listed companies with a listing period of less than 1 year. Due to the significant heterogeneity among listed companies, especially the quite different financial indicators of some listed companies, to avoid the interference of the outliers of individual indicators on the sample data, this paper adopts 1% truncated processing for all data.
To construct and depict the decision of enterprises on digital transformation, measure the digital transformation of enterprises with intangible capital, and consider the synergy between intangible capital and fixed assets (the interaction between intangible capital and fixed assets), the following measurement models are considered:
According to Eq. 9, the expected
ESG information disclosure of explained variables. ESG disclosure data of A shares began in 2017, and the collection range of equity research data is A shares non-ST shares from 2017 to 2020. ESG refers to the score of corporate social responsibility. This study mainly adopts the A-share Wind score standard and FTSE Russell score standard.
Explaining the interaction between the logarithm of variable intangible capital and the fixed asset logarithm. In the process of digital transformation, with the increase of intangible capital investment, the cooperation between intangible capital and fixed assets is helpful to improve enterprise performance. In this study, intangible capital investment mainly includes intellectual capital and organizational capital (Eisfeldt and Papanikolaou, 2014; Peters and Taylor, 2017). Intellectual capital is measured by R&D input and expenditure, and sales, general and administrative expenditures (SG&A for short) are accounted into intangible capital by using perpetual inventory method. This study draws on Peters and Taylor’s (2017) method for intangible capital accounting. To analyze the problem in a simple manner, see the choice of intangible capital investment below as the digital transformation decision-making of enterprises.
In this study, intangible capital is not used alone, but expressed by the interaction between intangible capital and fixed assets. There are two reasons: First, in the digital transformation of enterprises, with the input of intangible capital, intangible capital still needs to rely on fixed assets and other equipment to achieve mass production, and there is certain mutual synergy between intangible capital and fixed assets; secondly, to describe the decision of enterprises on digital transformation, it can be measured by the ratio of intangible capital to fixed assets, and the influence of the interaction between intangible capital and fixed assets on enterprise performance can be measured by the change of the ratio of intangible capital to fixed assets.
Business performance (income). The existing indicators of enterprise performance measurement mainly include return on assets (Brown and Kimbrough, 2011; Zhang et al., 2015; Dai and Li, 2021; Zheng and Huang, 2021), return on equity (Zhang et al., 2015; Dai and Li, 2021), sales profit rate (Zhang et al., 2015), operating income growth (Liu M. et al., 2021), per capita operating income (Zhang and Pan, 2019), and Tobin’s Q (Abernethy et al., 2019). To implement digital transformation, enterprises often need to invest more in digital resources and organizations (Wang and Wang, 2021), and realize product and service innovation to meet customer needs (Luo and Jiang, 2021). To catch the change of enterprise management caused by the choice of intangible capital investment in the digital transformation of enterprises, the method put forward by Zhang and Pan (2019) is used for reference, and the operating income indicator is used to measure enterprise performance. At this time, compared with other indicators, operating income can more directly reflect the change of output caused by the digital transformation of enterprises.
Financing constraints. The existing indicators for measuring the financing constraints faced by enterprises mainly include SA (Lu and Chen, 2017; Jiang et al., 2019; Zhou et al., 2021), KZ index (Jiang et al., 2019) and WW index. In this study, SA method is adopted for financing constraints.
Other control variables. To avoid the possible collinearity between the control variables and the explained variables, the control variables in this study mainly include ending cash flow logarithm (lncash), Tobin Q (tobin_q), asset-liability ratio logarithm (lndebt) and the top ten shareholders’ shareholding ratio (lnshare_holder) by referring to the design methods of Jiang et al. (2019) and Zhou et al. (2021). The logarithm of cash flow at the end of the period (lncash) is mainly measured by the ending balance of cash and cash equivalents in the financial report of the listed company; Tobin’s Q (tobin_q) is measured by the ratio of the company’s market value to replacement cost.
Logarithmic transformation is adopted for dependent variables, in which the related variables such as final cash flow, operating pressure, and financing constraint variables may have negative values and will be treated according to the digital-to-analog conversion equation. To reduce the interference to the accuracy of the model caused by the possible abnormal values of related variables, all variables are truncated by 1%.
See Table 1 for statistical description of main variables. As shown in Table 1, the minimum and maximum scores of ESG information disclosure scores of listed companies are 0.77 and 2.27, respectively, indicating that there is a big gap among listed companies. The logarithmic minimum and maximum values of operating income of listed companies are 0.30 and 7.27, respectively, which leads to such great differences among listed companies. On the one hand, it may be related to the status of the industry in which the enterprises are located, and on the other hand, it may be related to the ESG information disclosure decision of enterprise management. Furthermore, the minimum value and maximum value of the interaction term (lncap_fix) between variable intangible capital logarithm and fixed asset logarithm are −1.73 and 7.12, respectively, which indicates that there is a big difference in heterogeneity among enterprises. On the one hand, it is the heterogeneity of the company itself; on the other hand, it is mainly reflected in the difference caused by the choice of intangible assets of enterprises in the process of digital transformation.
Equation 9 reflects the impact of the interaction between intangible capital and fixed assets on enterprise performance, as shown in Table 2. According to Table 2, Column (1) indicates the cash flow logarithm (lncash), Tobin Q (tobin_q), asset-liability ratio logarithm (lndebt), the top ten shareholders’ shareholding ratio (lnshare_holder), business performance (lnincome), time and industry fixed effect at the end of the control period. Without considering the financing constraints, the interaction between the intangible capital logarithm and the fixed asset logarithm is helpful to improve the enterprise ESG score. The performance interaction item lncap_fix is increased by 1%, and the enterprise ESG score is increased by 0.014%; Column (2), on the basis of Column (1), considers the influence of the interactive item lncap_fix on the enterprise ESG score under the financing constraints faced by the enterprise. The results show that the influence of interactive item lncap_fix on enterprise ESG score is 0.004% higher than that of Column (1), indicating that the digital transformation of management is more helpful to improve enterprise ESG score under financing constraints.
Furthermore, to test the robustness of Columns (1) and (2) in Table 2, this paper intends to adopt FTSE Russell scoring standard. Column (3) of Table 2 considers the regression result of FTSE Russell score, and the result shows that the interaction between the intangible capital logarithm and the fixed asset logarithm can promote the improvement of enterprise ESG score, which is reflected by every 1% increase of interaction lncap_fix contributing to every 0.11%increase of enterprise ESG score. The effect of FTSE Russell score is obviously better than that of Wind ESG score; similarly, on the basis of Column (3), Column 4) considers the influence of the interactive item lncap_fix on the enterprise ESG score under the financing constraints faced by enterprises. The results show that the interactive item lncap_fix contributes to the improvement of enterprise ESG score.
The above results show that under the financing constraint, the interaction between the intangible asset logarithm and the fixed asset logarithm is helpful to promote the enterprise ESG score. To get rid of the financing constraint, the management is motivated to change the ratio of intangible capital to fixed assets, that is, to promote the structural transformation of enterprises, so as to achieve the purpose of improving business performance.
Column (2) of Table 2 shows that the financing constraint makes the interactive item lncap_fix promote the improvement of enterprise ESG score. How does financing constraint reduce the impact of interactive item lncap_fix on the enterprise ESG score? To analyze this problem, with other conditions unchanged, this paper reveals how the financing constraints faced by enterprises affect the decision of enterprises on digital transformation, and then depicts its impact on enterprise ESG score.
The financing constraint SA is divided into three dimensions according to the 25 and 75% quantiles, and the SA value with quantile less than 25% is defined as the group with strong financing constraint; the SA value with quantile between 25 and 75% is defined as the group with strong financing constraints; the SA value with quantile greater than 75% is defined as the group with weak financing constraints. Therefore, CF1, CF2 and CF3 are respectively used to represent three virtual variables: strong financing constraint, relatively strong financing constraint and weak financing constraint.
The three virtual variables showing the strength of financial constraints faced by enterprises are multiplied by the intangible capital logarithm, respectively, to describe the influence of digital transformation decision on enterprise performance under the financial constraints faced by enterprises. The results are shown in Table 3. According to Table 3, the Column (1) of Table 3 shows that when the interactive item lncap_fix is increased by 1%, the enterprise ESG score is increased by 0.021%, which is consistent with the conclusion of Column (2) of Table 2. The process of financing constraints affecting the influence of digital transformation decision-making on enterprise ESG score can be expressed as follows: under strong financing constraints, increasing intangible capital investment will increase enterprise ESG score by 0.007%; however, in the face of weak financing constraints, increasing intangible capital investment will not improve the enterprise ESG score; faced with weak financing constraints, increasing intangible capital investment will increase the enterprise ESG score by 0.008%. Compared with the enterprises not under financing constrains, increasing intangible capital investment helps to improve the enterprise ESG score under financing constraints.
The above results show that, when enterprises are faced with financing constraints, increasing intangible capital investment may not necessarily improve the enterprise ESG score, but will promote enterprises under strong financing constraints to continue to choose to invest in fixed assets, that is, to adopt the conventional decision. With the gradual relaxation of financing constraints on enterprises, the coefficients of interactive terms intan_cap✕CF2 and intan_cap✕CF3 increase, indicating that the decision on intangible capital investment is helpful to improve enterprise performance. That is, the management adopts the most dramatic decision on digital transformation, thus promoting the improvement of enterprise ESG score. To check whether the above conclusions are correct, GMM method is adopted, and the results are shown in Column (2) of Table 3, respectively. As shown in Column (2) of Table 3, the results are basically consistent with those in Column (1) of Table 3, indicating that financing constraints restrict the choice of intangible capital investment of enterprises.
Take Mobvista (01860.HK), a digital marketing company, as an example. Mobvista is a technology service company based on the global mobile Internet ecosystem. Its main business is to provide mobile app developers with marketing technology services and cloud computing technology services. Mobvista was listed on the Hong Kong Stock Exchange in 2018 and is known as “the first stock of intelligent mobile advertising in the new economy in the world”. Most of the company’s revenue comes from overseas, and it is one of the leading mobile Internet companies going overseas. Its most important product is programmatic advertising platform Mintegral. According to the statistics of AppsFlyer in March 2022, Mintegral ranks fourth in the global all-category retention strength list in both iOS and Android systems. In addition to Mintegral, Mobvista strives to build a SaaS tool ecosystem to empower B-end customers and its own development, and build a shared and win-win growth flywheel.
In the process of Mobvista’s investment in digitalization, it is faced with a large financing constraint. According to the company’s financial report, from 2017 to 2020, the company’s closing balance of cash and cash equivalents at the end of the period was about 300 million yuan on average. In 2017 and 2020, the initial balance of cash and cash equivalents was -200 million yuan. However, in order to survive in the industry, the company attaches great importance to customer privacy, and always regards data supervision as an important lifeline, which has been widely praised by the market. In 2021, the company won the ESG Award Titanium Award from Hong Kong’s “The Asset” magazine to Mobvista.
To test the relationship between intangible capital investment decision and enterprise ESG score, the intermediary mechanism analysis is introduced, and the enterprise performance is selected as the intermediary variable to study the path of enterprise ESG score. See Table 4 for the results of intermediary mechanism analysis. As shown in Table 4, Column (1) of Table 4 shows that the improvement of enterprise performance significantly improves enterprise ESG score; Column (2) of Table 4 shows that after the introduction of intermediary variables, the intermediary effect is 0.006. Although the intermediary effect is not large, the effect is remarkable.
The data description in Table 1 shows that there is great heterogeneity among enterprises. When faced with financing constraints and business pressure, are there differences in the choice of intangible capital investment among different types of enterprises? To analyze this problem, this paper attempts to consider the nature of enterprises (state-owned enterprises and private enterprises), the scientific and technological attributes of enterprises (traditional manufacturing and high-tech enterprises) and the proportion of intangible capital and fixed assets. Among them, enterprises engaged in the top 10 areas specified in “Made in China 2025″ are defined as high-tech enterprises, while others are regarded as traditional manufacturing industries.
The heterogeneity analysis is shown in Table 5. As shown in Table 5, Columns (1) and (2) in Table 5 correspond to the types of enterprises. For the types of state-owned enterprises and private enterprises, the lnintan_fix coefficient of the interaction between the intangible capital logarithm and the fixed asset logarithm is significantly positive after the selection of intangible capital. However, the effect of state-owned enterprises is better than that of private enterprises, which indicates that the lnintan_fix coefficient of state-owned enterprises is higher than that of private enterprises; for the heterogeneous enterprises with scientific and technological attributes, the intangible capital selection of traditional manufacturing enterprises is better than that of high-tech enterprises, which is reflected as their lnintan_fix coefficient of interaction term being higher than that of high-tech enterprises.
For the groups with intangible capital accounting for 25, 50, and 75%, the lower the proportion of intangible capital, the higher the interactive item lnintan_fix coefficient, and the stronger the willingness of enterprises to maintain fixed assets, as shown in Columns (5), (6) and (7) of Table 5.
Not all enterprises have the genes of digital transformation, and the management of heterogeneous enterprises is restricted by operating pressure and financing constraints. Through the investment of general-purpose technologies in enterprise digital transformation, this paper measures the enterprise digital transformation from the perspective of intangible capital, and measures the degree of enterprise digital transformation by the ratio of intangible capital to fixed assets. According to the mutual cooperation between intangible capital and fixed assets, it captures the influence and effect of digital transformation on enterprise ESG score. The results show that: 1) The digital transformation of enterprises is helpful to improve the enterprise ESG score. The product of the intangible capital logarithm and the fixed asset logarithm significantly improves the enterprise ESG score. However, under financing constraints, the product of the intangible capital logarithm and the fixed asset logarithm improves the enterprise ESG score. 2) When enterprises are faced with strong financing constraints, the management’s decision on digital transformation is more “radical”, which is more conducive to the improvement of enterprise ESG score, and may be more beneficial to increase the process of digital transformation.
Combined with the research conclusion, the suggestions for heterogeneous enterprises to carry out digital transformation mainly include:
First of all, revitalizing the assets, easing the financing constraints of enterprises, and providing financing guarantee for the digital transformation of enterprises. The conclusion of this study shows that, faced with the double constraints of business pressure and financing constraint, the best choice of the management will be conventional. That is, the power of digital transformation is insufficient, but it is beneficial to maintain or increase fixed assets, which is not conducive to the transformation and development of enterprises. Especially in the background of advocating high-quality development, the middle and lower reaches of the industry will face a big development dilemma in the future if enterprises fail to seize the opportunity to catch up. Especially for the traditional manufacturing industry, digital transformation is imminent. For the traditional manufacturing industry which mainly focuses on fixed assets, activating the fixed assets and increasing the value of the fixed assets will help ease the financing constraints and provide a good financing guarantee for digital transformation. For the traditional manufacturing industry, because the interaction item of the product of the intangible capital logarithm and the fixed asset logarithm has a good effect on enterprise performance, the digital transformation of the traditional manufacturing industry is helpful to improve enterprise performance. For the traditional manufacturing industry with operating pressure, it is not appropriate to adopt short-term assessment alone. By combining short-term assessment with long-term assessment, the management will have the courage to “cut off all means of retreat” to carry out digital transformation.
Secondly, launching special policies at the national level to encourage the digital transformation of private enterprises. Compared with state-owned enterprises, the digital transformation of private enterprises mainly revolves around marketization, without the support of special national policies. Compared with state-owned enterprises, the interaction item of the intangible capital logarithm multiplied by the fixed asset logarithm brought by the digital transformation of private enterprises has less effect on enterprise performance improvement than that of state-owned enterprises. From the marginal effect, there is more room for private enterprises to improve their performance through digital transformation. From the perspective of stimulating market vitality and building a new development pattern, the state encourages the digital transformation of private enterprises through relevant policies, which will help accelerate the development of economy to high quality and promote the pace of digital transformation of private enterprises.
Finally, supporting the digital transformation of enterprises having small proportion of intangible capital and facing financing constraints and operating pressure. The research shows that for enterprises with a small proportion of intangible capital, the management has the courage to “cut off all means of retreat” to carry out digital transformation under the condition of appropriately relaxing the corporate financing constraints. For this reason, enterprises with greater financial constraints can carry out digital transformation with financial support, and the specific measures can be taken from aspect such as expanding the mortgage financing rate and providing green financial support and personnel training support.
The raw data supporting the conclusions of this article will be made available by the authors, without undue reservation.
Conceptualization, JG and JL; methodology, JG and YL; vali-dation, JG, JL, and YL; formal analysis, JG, JL, and YL; investigation, JG, JL, and YL; resources, JG, JL, and YL; data curation, JG, JL, and YL; writing—original draft preparation, JG; writing—review and editing, JG, JL, and YL; supervision, YL; project administration, YL; funding acquisition. All authors have read and agreed to the published version of the manuscript.
The authors declare that the research was conducted in the absence of any commercial or financial relationships that could be construed as a potential conflict of interest.
All claims expressed in this article are solely those of the authors and do not necessarily represent those of their affiliated organizations, or those of the publisher, the editors and the reviewers. Any product that may be evaluated in this article, or claim that may be made by its manufacturer, is not guaranteed or endorsed by the publisher.
1This article is a general OLS measurement model. Of course, the core explanatory variable in the measurement model is the interaction term of the logarithm of intangible capital and the logarithm of fixed assets. The setting condition of this interaction variable is the effect of the explanatory variable intangible capital on the explained variable. When potentially affected by fixed assets, we need to consider using regression with interaction terms.
Abernethy, M. A., Kuang, Y. F., and Qin, B. (2019). The relation between strategy, CEO selection, and firm performance. Contemp. Acc. Res. 36 (3), 1575–1606. doi:10.1111/1911-3846.12463
Akcigit, U., and Ates, S. T. (2021). Ten facts on declining business dynamism and lessons from endogenous growth theory. Am. Econ. J. Macroecon. 13, 257–298. doi:10.1257/mac.20180449
Altomonte, C., Favoino, D., Morlacco, M., and Tommaso, S. (2021). Markups, intangible capital and heterogeneous financial frictions. Centre for Economic Performance, LSE Working Paper.
Autor, D., Dorn, D., Katz, L. F., Patterson, C., and Reenen, J. V. (2020). The fall of the labor share and the rise of superstar firms. Q. J. Econ. 135, 645–709. doi:10.1093/qje/qjaa004
Brown, N. C., and Kimbrough, M. D. (2011). Intangible investment and the importance of firm-specific factors in the determination of earnings. Rev. Acc. Stud. 16, 539–573. doi:10.1007/s11142-011-9151-x
Brynjolfsson, E., Rock, D., and Syverson, C. (2021). The productivity J-curve: How intangibles complement general purpose technologies. Am. Econ. J. Macroecon. 13, 333–372. doi:10.1257/mac.20180386
Chappell, N., and Jaffe, A. (2018). Intangible investment and firm performance. Rev. Ind. Organ. 52, 509–559. doi:10.1007/s11151-018-9629-9
Corrado, C. A., Hulten, C. R., and Sichel, D. E. (2009). Intangible capital and economic growth. Rev. Income Wealth 55, 661–685. doi:10.1111/j.1475-4991.2009.00343.x
Crouzet, N., and Eberly, J. C. (2018). Intangibles, investment, and efficiency. AEA Pap. Proc. 108, 426–431. doi:10.1257/pandp.20181007
Crouzet, N., and Eberly, J. C. (2021). Intangibles, markups, and the measurement of productivity growth. NBER Working Paper.
Crouzet, N., and Eberly, J. C. (2019). Understanding weak capital investment: The role of market concentration and intangibles. NBER Working Paper 25869.
Dai, H., and Li, Y. (2021). Does labor protection affect business performance? —— empirical evidence from the adjustment of China's minimum wage standard [J]. Theory Pract. Finance Econ. 2021 (6), 139–146.
Eisfeldt, A. L., and Papanikolaou, D. (2014). The value and ownership of intangible capital. Am. Econ. Rev. 104 (5), 189–194. doi:10.1257/aer.104.5.189
Farhi, E., and Gourio, F. (2018). Accounting for macro-finance trends: Market power, intangibles, and risk premia. NBER Working Paper 25282.
Harris, M., and Raviv, A. (1991). The theory of capital structure. J. Finance 46 (1), 297–355. doi:10.1111/j.1540-6261.1991.tb03753.x
Jiang, F., Cai, W., Cai, X., and Li, X. (2019). Micro-effect of bank competition: Empirical evidence from financing constraints [J]. Econ. Res. J. 2019 (6), 72–88.
Li, D., Ge, F., Yang, Z. G., Zhou, P. L., Wu, R. H., and Chen, H. (2020). Research report on digital transformation of Chinese enterprises: Great changes and reconstruction [R]. Beijing: Tsinghua University Global Industry Research Institute, 4–5.
Liu, M., Du, M., and Liu, X. (2021). Government subsidies and the performance of new energy enterprises: A perspective of heterogeneity and time lag [J/OL]. Sci. Res. Manag., 1–20. Available at: http://kns.cnki.net/kcms/detail/11.1567.G3.20211021.1144.004.html (Accessed 11 29, 2021).
Liu, Z., Shen, H., Welker, M., Zhang, N., and Zhao, Y. (2021). Gone with the Wind: An externality of earnings pressure. J. Account. Econ. 72, 101403. doi:10.1016/j.jacceco.2021.101403
Lu, S., and Chen, S. (2017). Does the government's favoritism ease the financing constraints of enterprises? Quasi-natural experiments from China [J]. J. Manag. World 2017 (5), 51–65.
Luo, J., and Jiang, Q. (2021). Analysis on the evolution of product and service innovation priority under digital transformation-based on Haier Zhijia case [J/OL]. Stud. Sci. Sci. 1-17, 11–29. doi:10.16192/j.cnki.1003-2053.20211118.006
McGrattan, E. R. (2020). Intangible capital and measured productivity. Rev. Econ. Dyn. 37, 147–166. doi:10.1016/j.red.2020.06.007
McGrattan, E. R., and Prescott, E. C. (2014). A reassessment of real business cycle theory. Am. Econ. Rev. 104, 177–182. doi:10.1257/aer.104.5.177
Peters, R. H., and Taylor, L. A. (2017). Intangible capital and the investment-q relation. J. Financial Econ. 123, 251–272. doi:10.1016/j.jfineco.2016.03.011
Tambe, P., Hitt, L. M., Rock, D., and Brynjolfsson, E. (2020). Digital capital and superstar firms. NBER Working Paper.
Wang, Y., and Wang, L. (2021). Research on the type identification and transformation mode selection of traditional enterprise digital transformation strategy [J/OL]. Manag. Rev. 2021, 1–10. doi:10.14120/j.cnki.cn11-5057/f.20211123.001
Xiang, X., and Tian, L. (2014). On off-balance-sheet intangible assets: Based on the perspective of the integration of finance and strategy-an analysis of intangible resources, intangible assets and intangible capital [J]. Account. Res. 2014 (4), 3–9+95.
Xu, X., Wang, G., and Shi, Y. (2018). Intangible capital investment, industry spillover effect and growth difference: A study based on transnational data [J]. World Econ. Stud. 2018 (10), 108–120.
Yang, S., and Shi, X. (2018). Intangible capital and sectoral energy intensity: Evidence from 40 economies between 1995 and 2007. Energy Policy 122, 118–128. doi:10.1016/j.enpol.2018.07.027
Zhang, T., and Pan, L. (2019). Can patents improve enterprise performance? —— empirical research based on patent data of listed companies in China [J]. Shanghai Finance 36 (5), 63–70.
Zhang, X., Jin, X., and Xu, L. (2015). Can the executive elite governance model improve enterprise performance? -Research on the moderating effect of social association [J]. Econ. Res. J. 2015 (3), 100–114.
Zheng, Y., and Huang, J. (2021). The impact of patent acquisition strategy on enterprise performance-an integrated perspective of application and maintenance decision [J/OL]. Sci. Res. Manag., 1–16. Available at: http://kns.cnki.net/kcms/dtail/11.1567.G3.20211021.1624.012.html (Accessed 11 29, 2021).
Keywords: intangible capital, ESG score, decision-making behavior, enterprise performance, digital transformation
Citation: Gao J, Li J and Luo Y (2022) Does the enterprise’s choice of intangible capital help to promote its ESG score?. Front. Environ. Sci. 10:978955. doi: 10.3389/fenvs.2022.978955
Received: 27 June 2022; Accepted: 25 July 2022;
Published: 14 September 2022.
Edited by:
Xiao-Guang Yue, European University Cyprus, CyprusReviewed by:
Wei Zhang, Anhui University of Finance and Economics, ChinaCopyright © 2022 Gao, Li and Luo. This is an open-access article distributed under the terms of the Creative Commons Attribution License (CC BY). The use, distribution or reproduction in other forums is permitted, provided the original author(s) and the copyright owner(s) are credited and that the original publication in this journal is cited, in accordance with accepted academic practice. No use, distribution or reproduction is permitted which does not comply with these terms.
*Correspondence: Yuwei Luo, MjA5NTk4Njg0NUBxcS5jb20=
Disclaimer: All claims expressed in this article are solely those of the authors and do not necessarily represent those of their affiliated organizations, or those of the publisher, the editors and the reviewers. Any product that may be evaluated in this article or claim that may be made by its manufacturer is not guaranteed or endorsed by the publisher.
Research integrity at Frontiers
Learn more about the work of our research integrity team to safeguard the quality of each article we publish.