- School of Geographical Sciences, Liaoning Normal University, Dalian, China
As the most important ecological barrier in eastern China, the three northeast provinces have extraordinary ecological significance. Their geographical location and climatic conditions make the distribution pattern of vegetation unique. Among them, climate change and human activities are the main factors affecting the richness and complexity of vegetation, climate change dominates the overall pattern of vegetation distribution, and regional human intervention locally changes the growth state of vegetation. Therefore, based on NDVI data of vegetation, meteorological data and socio-economic data, statistical methods such as Sen’s slope estimator, Mann-Kendall trend test, center of gravity transfer model, Hurst index and coefficient of variation were adopted to analyze the spatio-temporal changes and future trends in vegetation coverage; The use of Geographic detector to understand the relationship between NDVI to climate change and human activities. The results suggested that: 1) The three northeast provinces of China experienced an overall increasing NDVI at a rate of 0.016%/year from 2001 to 2020. The NDVI shows intensive heterogeneity in space, exhibiting a landscape of high in the East, low in the west, high in the north and low in the south; 2) The results of geographic detectors show that the temporal and spatial distribution pattern of vegetation NDVI is mainly determined by ≥ 10°C accumulated temperature, ≥ 0°C accumulated temperature, annual average temperature and land use type. The interaction between factors presents a bi-variable enhancement and nonlinear enhancement, and human activity factors enhance the contribution of climate factors. 3) Future changes in NDVI indicated that the vegetation coverage showed a trend of “overall improvement and regional degradation” from 2001 to 2020, and showed reverse persistence. The degraded area of vegetation will be more than the improved area. The research results will help the three northeast provinces cope with global climate change, enrich the research on the vegetation in the three northeastern provinces, and provide a scientific basis for formulating appropriate regional ecological protection policies.
1 Introduction
Vegetation is the connecting link between atmospheric, soil and other ecological activities. Vegetation, as an important section of the terrestrial ecosystem, connects the material circulation and energy flow between the layers of the earth, and plays an indispensable role in regulating terrestrial carbon balance and climate system. Vegetation absorbs carbon dioxide and releases oxygen through photosynthesis to realize the value of carbon fixation and oxygen release (Zhang et al, 2017). In addition, the growth state of vegetation reflects the positive and negative feedback of human beings to natural vegetation ,(Liu et al, 2015) as well as whether environmental protection policies (Ma et al, 2021) and ecological construction (Jiang et al, 2015) have achieved initial results. Therefore, dynamic monitoring of the vegetation is used to study and analyze the relationship between vegetation change and climate change and human activities (Jiang et al, 2017). With the rapid development of remote sensing technology, many countries, organizations and institutions have formulated plans for global satellite data receiving, processing and generating remote sensing datasets (Kaku, 2019). Making it possible to obtain long-time series and multi-scale standard data integration, which provides convenience for vegetation dynamic monitoring. NDVI (normalized vegetation index) is the ratio between difference between near infrared band and visible band and the sum of the values (Roy et al, 2016). It is an important evaluation index reflecting vegetation growth status and vegetation coverage (Wang et al, 2020), which is widely used in vegetation dynamic monitoring because it can well reveal the evolution of regional ecological environment. The three northeast provinces in China are geographically in the humid and semi humid areas of China, with the largest natural forest areas in China - Northeast Daxing’an Mountains, Xiaoxing’an Mountains and Changbai Mountains (Dong et al, 2015). It is the most important ecological barrier in northeast China. Consequently, studying its vegetation change trend and driving factors has important practical significance for regional ecological protection, confronting with global climate change and realizing the goal of carbon neutralization.
Currently, the relevant research on NDVI is mainly carried out at the global scale (Fensholt and Proud, 2012; Eastman et al,2013), the regional scale (Chuai et al,2013; Zhang et al,2013), and the time scale includes the annual scale (Mo et al, 2015), the seasonal scale (Baniya et al, 2018) and the growth season scale (Wang et al, 2014), the research content mainly focuses on the spatial-temporal distribution characteristics and change trends of NDVI. (Pan et al, 2019; Meng et al, 2020), the driving factors affecting vegetation (Yang et al, 2019) and the future prediction of vegetation (Wang et al., 2020; Zhou et al, 2020). For Northeast China, many scholars have explored the temporal and spatial distribution pattern of NDVI in growing season (Guo et al, 2017; Chu et al, 2019) and interannual scale (Wang et al, 2009; Dehua et al, 2012). The research shows that NDVI shows an increasing trend both on an interannual scale and a seasonal scale. In terms of driving factors, most scholars conduct correlation analysis from the perspective of climate factors such as temperature and precipitation (Guo et al, 2007; Li et al., 2021) and the relationship between NDVI and spei (Li et al, 2020), PDSI (Zheng et al, 2015). The research shows that NDVI is positively correlated with temperature and precipitation and negatively correlated with drought, however, there is less comprehensive consideration of climate and human activities; In terms of future prediction of NDVI, there is a comparative lack of relevant research in Northeast China. Climate factors directly influence the trend of vegetation change, and human social and economic activities will also directly affect the temporal and spatial evolution of vegetation in the region. In view of this, it is necessary to conduct an overall study on the dynamic change monitoring, driving factor analysis and future change trend of vegetation NDVI in the three northeast provinces, so as to deepen the overall understanding of the coupling system of “vegetation–climate - human activities”. In terms of climate change, Pei et al. (2019) and Wang et al., 2020 respectively analyzed the impact of climate change on the wetlands in the Songnen Plain of Inner Mongolia and Northeast China. These studies showed that there was a certain correlation between temperature and precipitation and NDVI of vegetation. However, they only analyzed the impact of climate on NDVI. When analyzing the response of NDVI of vegetation to climate, they only considered such indicators as temperature and precipitation, and the selection of climatic factors affecting vegetation was not comprehensive. Therefore, I considered temperature, precipitation, accumulated temperature Dryness, humidity index and other indicators, which makes the relationship between climate and NDVI more comprehensive. In terms of research methods, many scholars choose correlation analysis (Qu et al, 2018; Liu et al, 2019; Gao et al, 2021), but this method is weak in the connection and interpretation of polygenes. In contrast, geographic detectors are more suitable for detecting the impact of multiple factors on vegetation NDVI (Huo and Sun, 2021; Wang et al, 2021; Zhang et al, 2022).
In terms of regional human activities, the advantages and disadvantages of human activities in the three northeastern provinces to the natural environment also need to be comprehensively considered (Cheng et al, 2021). Due to industrial and agricultural construction, the unreasonable development and utilization of resources has led to the depletion of forest resources, the reduction of grassland resources and the saturation of cultivated land development (Wang and Cheng, 2022), which to some extent has an adverse impact on the vegetation coverage. However, the ecological environment management in the three northeast provinces has achieved initial results in human activities. With the national attention to the ecological environment, the three northeast provinces have introduced the green transformation of traditional industries (Du et al, 2019), and the transformation of their development path has provided a positive driving force for the vegetation construction and ecological environment in the three northeast provinces.
In addition, many scholars choose the dual perspectives of climate change and human activities in studying the response of vegetation. For example, Zheng et al. (2019) analyzed the climatic factors and human activities affecting the Loess Plateau, and concluded that the slope repaired by the “conversion of farmland to forest project” is the main influencing factor of human activities; Li et al., 2021 explored the relationship between vegetation, climate change and human activities at the Chinese scale, and further concluded that the impact of human activities on vegetation can be divided into destruction and restoration; Xue et al. (2022) analyzed the contribution of climate change and human activities to the vegetation in Northeast China. In addition, the main driving force of vegetation improvement in this region is human activities.
Therefore, it is necessary to further study the driving mechanism of climate change and human activities on vegetation change. In the study, we can neither focus on the negative impact of human activities and ignore the favorable climate conditions, nor exaggerate the positive impact of human activities and ignore the ecological risk caused by industrialization. Unreasonable research and consideration of these two aspects may lead to wrong implementation of environmental protection policies and ecological construction in the study area, as well as misunderstanding of climate change and human activities on vegetation change. Referring to previous studies, combined with the current situation, we should focus on the relationship between NDVI and climate factors and human activities, and pay special attention to the future prediction of NDVI in the three northeast provinces. This will be of great significance to the ecological protection and sustainable development of the ecological environment in the three northeast provinces, coping with global change and implementing the goal of carbon neutralization.
To sum up, this study has some characteristics compared with the papers on NDVI at this stage. First, the study area is selected from the three northeastern provinces, and the complex and diverse vegetation types formed by its unique hydrothermal conditions provide good vegetation conditions for the study of NDVI; Secondly, the research angle is climate change and human activities, which perfectly combine the current hot issue—climate change and human’s master position in nature; In addition, compared with correlation analysis, selecting NDVI factors driven by geo detector research is more comprehensive and intuitive. Finally, compared with the same type of NDVI papers, the research aspects of this study are more comprehensive, including the distribution, development, driving factors and future prediction of NDVI, which enriches the related research on NDVI of vegetation in the three northeastern provinces.
2 Materials and methods
2.1 Study area
The three northeast provinces are situated in the high latitudes of China and at the edge of the temperate monsoon climate zone. Which are located at 48° N ∼ 55° N and 118° E ∼ 135° E (Figure 1). There are certain differences among the three provinces due to different natural conditions. Liaoning Province has the same rainy and hot season, rich sunshine, high accumulated temperature, long winter and warm summer, short spring and autumn, and four distinct seasons. The rainfall is uneven, wet in the East and dry in the West Jilin Province transits from humid climate to semi humid climate and then to semi-arid climate from southeast to northwest. The annual average precipitation is 400–600 mm. The climate of Heilongjiang Province is characterized by low temperature and drought in spring, warm and rainy in summer, easy waterlogging and early frost in autumn, cold and long winter, short frost free period, and large regional differences in climate. The three northeast provinces are vast and sparsely populated, with a high level of industrialization, mainly dry farming. Vegetation types are complex and diverse, including cold temperate deciduous coniferous forest, temperate coniferous and broad-leaved mixed forest, temperate forest grassland, meadow grassland and dry grassland (Qian et al, 2003). Because of the unique vegetation distribution pattern, the three northeast provinces have become one of the sensitive areas for global change research (Huang et al, 2019).
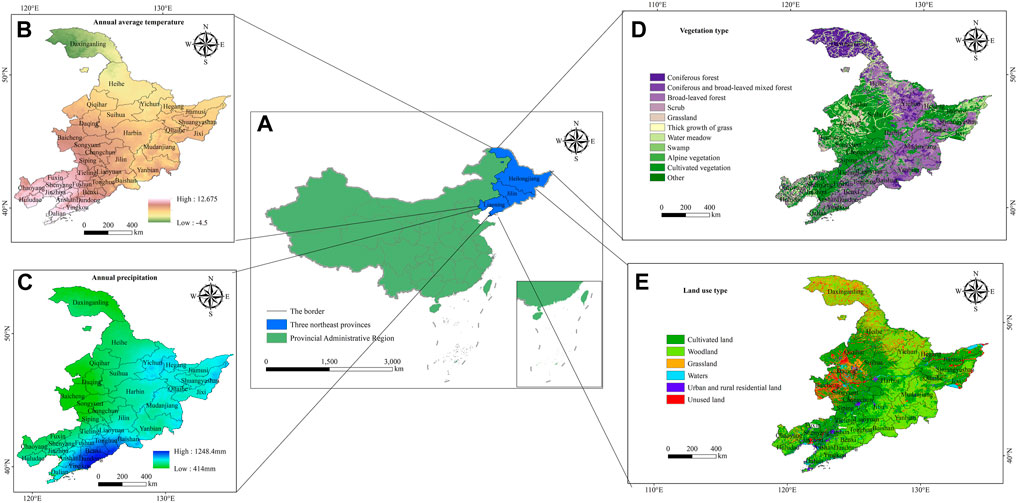
FIGURE 1. Map of the study area (A): Location map of the study area; (B) annual average temperature map of the study area; (C) annual precipitation map of the study area; (D) vegetation type map of the study area; (E) land use type map of the study area).
2.2 Data sources
MOD13A2 NDVI16d dataset is a MODIS vegetation index product provided by LPDAAC of the United States. MODIS-NDVI data improve the spatial resolution and chlorophyll sensitivity, and eliminate the interference of atmosphere and water vapor. The time span of NDVI data used in this study is 2001–2020, and the spatial and temporal resolution are 1 km and 16 d. The meteorological data are from the Resource and Environment Science and Data Center (http://www.resdc.cn). The meteorological data comes from China’s Meteorological background data set. The data of population, Gross Domestic Product (GDP) and sowing area of main crops are sorted into excel table by querying the statistical yearbook of the three northeast provinces.
Table 1, and then associated with the vector map of the three northeast provinces by ArcGIS; The data of land use types are derived from the remote sensing monitoring data of land use status in the three northeast provinces (Ning et al, 2018), and then generated by mosaic.
The preprocessing of NDVI data includes format conversion, coordinate conversion, data clipping, maximum synthesis and so on (Dai et al., 2021).Among them, the maximum value synthesis is used to process the monthly maximum value data to obtain the annual maximum value data of NDVI (Yang et al., 2019). Other data are processed through vector data clipping and projection transformation of the administrative boundary of the three northeast provinces in 2020. The statistical unit of the sowing area of main crops is set as the county-level administrative division, which is converted into a unified unit (hectare). Spatially associating statistical data with corresponding administrative unit using ArcGIS 10.2, at the same time, feature to raster were carried out to obtain the grid map of sowing area of main crops.
2.3 Methods
2.3.1 NDVI classification
To intuitively characterize dynamic changes of vegetation, the NDVI of vegetation in the three northeast provinces from 2001 to 2020 was reclassified into five vegetation coverage grades by equal interval algorithm (Peng et al, 2019) (Table 2).
2.3.2 Center of gravity transfer model
The shift of center of gravity represents the spatial evolution process of object elements, and can reflect the development and change trend of a certain phenomenon or activity in the region. In addition, the overall change trend and spatial distribution of NDVI can be described by the center of gravity transfer process of different levels of vegetation coverage (Cong et al, 2019). The Formula 1 for calculating the center of gravity transfer of vegetation cover in year t is as follows:
Where, the longitude coordinate of the distribution center of different levels of vegetation coverage is expressed as Xt, and the latitude coordinate is expressed as Yt; Cit represents the area of the ith vegetation cover patch in year t; Xit and Yit respectively represent the longitude and latitude coordinates of the center of gravity of the ith vegetation covered patch in the t year.
2.3.3 Index information extraction
In this study, comprehensively considering the principles of systematicness, typicality, dynamics, scientificity, quantifiability and availability, six climatic factors and four human activity factors were selected to explore their effects on vegetation NDVI in the three northeast provinces (Table 3). In order to judge whether there is a strong linear relationship between independent variables, it is necessary to select the appropriate independent variable factors and carry out collinearity diagnosis on the independent variable factors. After collinearity diagnosis by SPSS software, the Vif value is 1–7.9, of which the Vif values of ≥ 10°C accumulated temperature and ≥ 0°C accumulated temperature are greater than 10. Because the accumulated temperature is the main factor affecting the vegetation growth stage, it is necessary to retain the accumulated temperature when studying climate change.
The annual average temperature, annual precipitation, ≥ 0°C accumulated temperature, ≥ 10°C accumulated temperature, aridity, moisture index, population, GDP, land use type and sowing area of main crops are divided into six categories by the natural breakpoint method (Table 3 and Figure 2). Using the fishing net tools of ArcGIS 10.2, create 10 km × 10 km grids within the three northeast provinces, generate 16361 sampling points, extract the attribute values corresponding to X and Y in space to generate the attribute table, and then replace the attribute table into the geo detector software for processing.
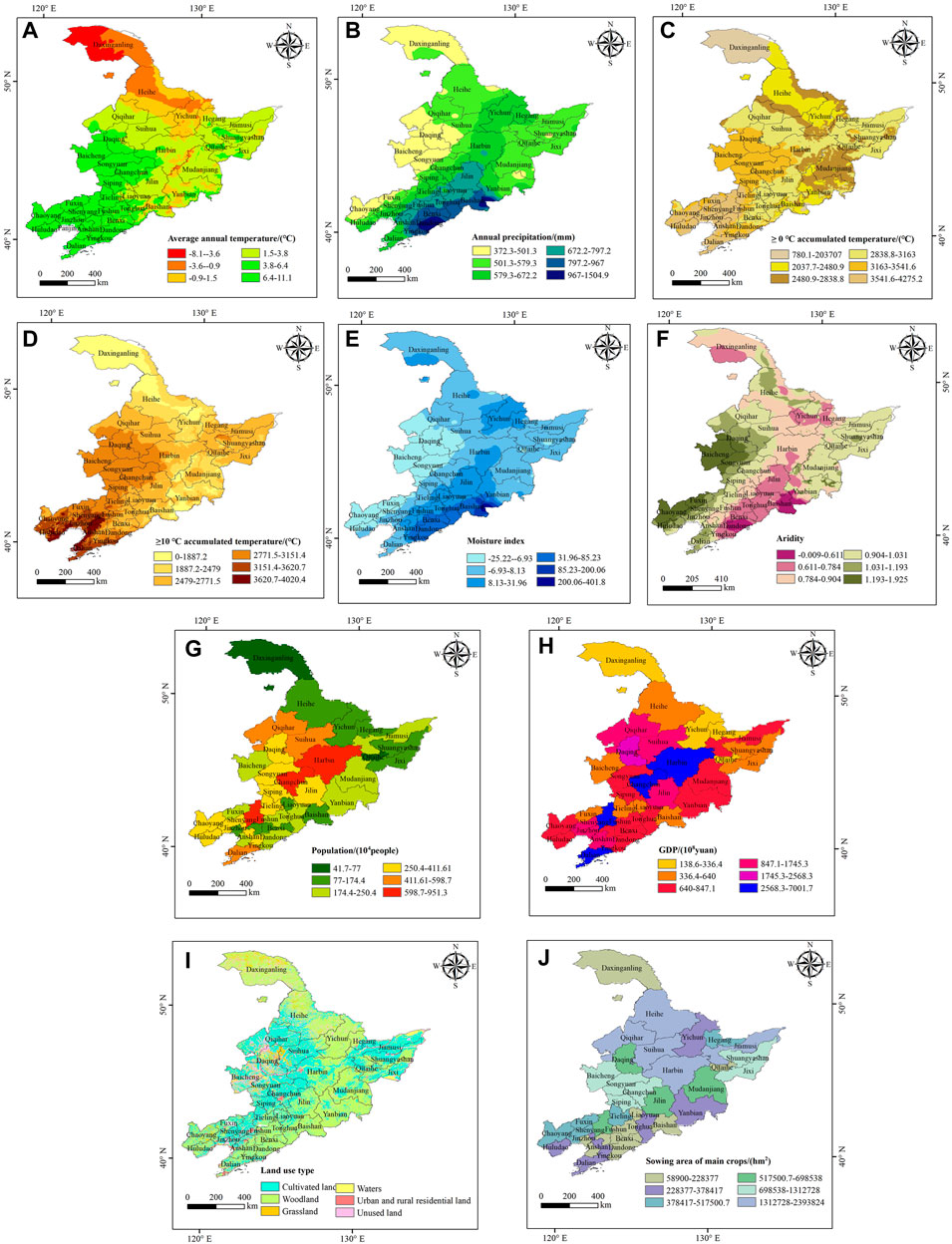
FIGURE 2. NDVI drivers and spatial distribution in the three northeast provinces. (A): Annual average temperature map; (B) Annual precipitation map; (C) ≥ 0°C accumulated temperature map; (D) ≥ 10°C accumulated temperature map; (E) Moisture index map; (F) Aridity map; (G) Population map; (H) GDP map; (I) Land use type map; (J) Sowing area of main crops map).
2.3.4 GeoDetector model
GeoDetector is a new spatial statistical method based on factors, ecology, interaction and risk to detect spatial differentiation and reveal driving factors (Ouyang et al, 2021).
1) The factor detector focuses on exploring the spatial differentiation of dependent variable y, that is, the interpretation of independent variable X to dependent variable Y. Measured by Q value, formula (2) and (3) are (Chen et al, 2020):
Where, the value of Q measures the interpretation of factor X to Y, and the value range of Q is (0, 1). The greater the value of Q, the stronger the interpretation of each factor, that is, the greater the impact on the spatial differentiation of NDVI. L is the category or division of X or Y; Nh and N represent the number of units in layer h and the entire area;
2) Ecological detector used to measure whether the influence of factor X on the spatial distribution of Y is significant, as shown in formula (4):
Where,
3) Interaction detector used to identify the interaction between factors, that is, to evaluate whether the interpretation of Y is enhanced, weakened or independent when the two factors interact together. The calculation result is obtained by comparing the Q value of each single factor with the Q value of interaction.
4) Risk detector was used to detect the appropriate range or type of impact of different factors on vegetation cover, and use the t-statistic as shown in Formula 5:
Where,
2.3.5 Theil-Sen median trend analysis and Mann-Kendall nonparametric test
The Theil-Sen median ananlysis, also known as Sen slope estimation, is a nonparametric statistical method. It is rarely affected by outliers and is suitable for analyzing the trend of long-time series data (Yuan et al, 2020). The calculation Formula 6 is:
Where,
Mann-Kendall is rated as a nonparametric statistical test method because its samples do not need to follow the normal distribution and are rarely disturbed by outliers. The statistical test method is shown in Formulas 7–11. For time series,
Where
2.3.6 Coefficient of variation
The coefficient of variation can measure the variation degree of observation sequence values, reflect the variation difference of spatial data in time series, and evaluate the stability of data. The calculation Formula 12 is (He et al, 2021):
Where
2.3.7 Hurst index
Hurst can predict the future development trend of a time or space-time series. Wherein the main principle is to construct a time series
The cumulative dispersion Formula 14 is:
The range is Formula 15:
Standard deviation is Formula 16
If
3 Results
3.1 Temporal and spatial distribution characteristics of NDVI
3.1.1 NDVI temporal distribution characteristics
Analyzing the interannual variation trend of average NDVI in the three northeast provinces from 2001 to 2020 by using linear regression (Figure 3). The results showed that the annual average value of NDVI remained between 0.79 and 0.84 during the whole study period, with the maximum NDVI of 0.837 recorded in 2017, and the minimum of 0.796 recorded in 2001. Overall, the annual average NDVI of vegetation in the three northeast provinces showed an increasing trend of fluctuation from 2001 to 2020, with a trend rate of 0.16 × 10−3/year, vegetation growth generally shows a good development trend.
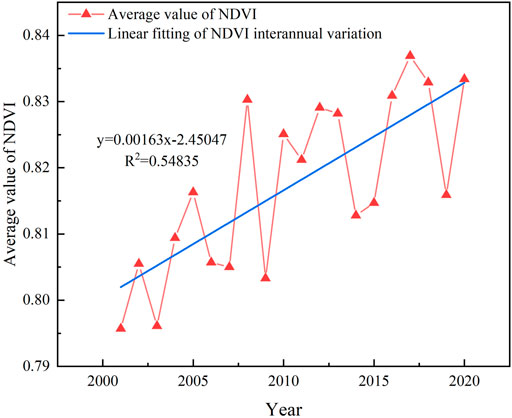
FIGURE 3. NDVI change trend of annual average vegetation in the three northeast provinces from 2001 to 2020.
3.1.2 NDVI spatial distribution characteristics
Calculating the average value of 20 years, and classifying into five levels using the equal spacing method in ArcGIS 10.2, (Figure 4). It can be seen from the Figure 4 that the spatial heterogeneity of vegetation coverage in the three northeast provinces from 2001 to 2020 is strong, mainly manifested in the distribution pattern of high vegetation coverage in the east, low in the west, high in the north and low in the south. From the spatial distribution of NDVI levels, the area of high vegetation coverage accounts for the largest, reaching 74.98%; followed by medium and high vegetation coverage, with an area ratio of 20.09%; medium vegetation coverage area, medium and low vegetation coverage area and low vegetation coverage area the proportion of regional area is small, which is 3.37, 0.9 and 0.66% respectively, where is mainly distributed in the south of Daqing, Baicheng, Songyuan, Jixi and the coast of Liaodong Bay. There are medium and high vegetation coverage areas and high vegetation coverage areas in the rest of the three northeast provinces, which shows that the vegetation growth in the three northeast provinces is good to a certain extent.
According to the classification of vegetation coverage in Table 1, introducing the center of gravity transfer model. The results (Figure 5) showed that the center of gravity points of different vegetation coverage levels have obviously moved, which better presents the spatial change orientation of vegetation NDVI in the three northeast provinces in the past 20 years. The center of gravity of low vegetation coverage area (0 < NDVI <0.2) is concentrated in Siping City of Jilin Province and shifted southward to Tieling City of Liaoning Province; The center of gravity of the middle and low vegetation coverage area migrated southeast from Songyuan to Siping City (0.2 < NDVI <0.4). The center of gravity of the vegetation coverage area is mainly concentrated at the junction of Baicheng city and Songyuan City, moving from southeast to Southeast, and moving to Siping City by 2020 (0.4 < NDVI <0.6). The main moving direction of the center of gravity in the medium and high vegetation coverage area is southwest-southwest, starting from Songyuan City to Siping City (0.6 < NDVI <0.8). The center of gravity of the high vegetation coverage area is mainly concentrated in Harbin, and the moving direction of the center of gravity is southwest-northeast (0.8 < NDVI <1).
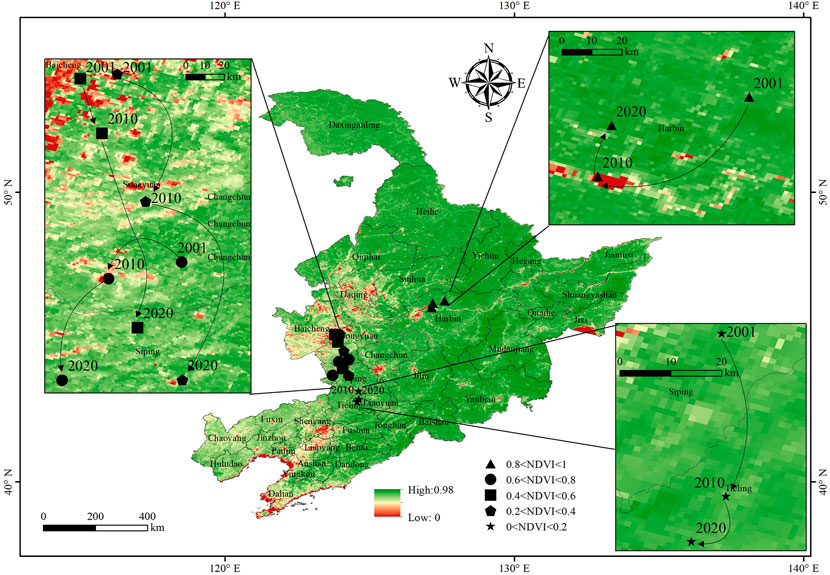
FIGURE 5. Center of gravity migration of different vegetation coverage in the three northeast provinces from 2001 to 2020.
3.2 Geographical detection model for NDVI drivers
3.2.1 Influence analysis of detection factors
The results of the factor detector reflect the influence of the magnitude of each. Driver on the NDVI in the three northeast provinces, as expressed by the q value of each driver. As shown in Figure 6, climate factors and human activity factors have an impact on NDVI in the three northeast provinces. The influence order of each factor is ≥10°C accumulated temperature, ≥ 0°C accumulated temperature, average annual temperature, land use type, aridity, moisture index, population, sowing area of main crops, annual precipitation > GDP.
3.2.2 Significance difference analysis of detection factors
Whether the impact of each driving factor on NDVI in the three northeast provinces is significant is reflected by ecological detection. The results with significant statistical difference between each two factors show that if there is significant difference between row factor and column factor, it is marked as “Y”, otherwise it is marked as “N”. It can be seen from Figure 7 that there is a significant difference between the annual average temperature and ≥0°C accumulated temperature and ≥10°C accumulated temperature, and there is no significant difference with other factors. There were significant differences between annual precipitation and ≥0°C accumulated temperature ≥10°C accumulated temperature, aridity and moisture index, but there was no significant difference with other factors. There was significant difference between land use types and annual precipitation, aridity, moisture index, population and GDP, but there was no significant difference with other factors. There was no significant difference between the sowing area of main crops and other factors.
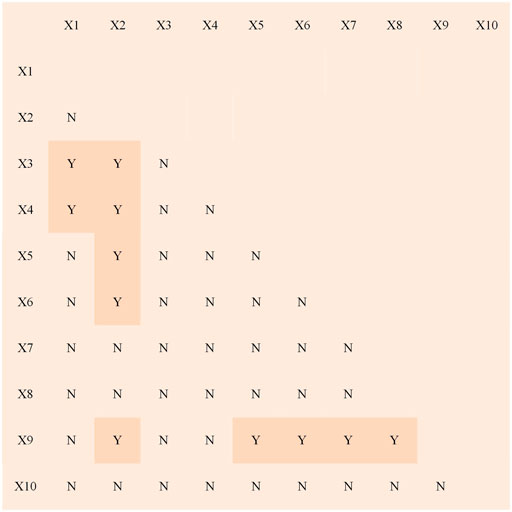
FIGURE 7. Statistical significance of detection factors (confidence level 95%). Note: Y indicates that there is significant difference (95% confidence) between the two factors on NDVI; N indicates no significant difference.
3.2.3 Interaction analysis of detection factors
The interaction detection is used to evaluate the impact of the interaction of different influencing factors on NDVI in the three northeast provinces, and to judge whether the interaction of the two factors will increase or weaken the interpretation of NDVI in the three northeast provinces. It can be seen from the Figure 8 that there is interaction between various factors on NDVI in the three northeast provinces. The interaction between factors presents the relationship of bi-variable enhancement and nonlinear enhancement, and there is no relationship of mutual independence and weakening. Moreover, the interpretation of all factors after interaction is stronger than that of a single factor. X1 ∩ X2, x1 ∩ X8, X2 ∩ X3, X2 ∩ X7, X2 ∩ X8, X2 ∩ x9, X2 ∩ X10, X5 ∩ X8, X5 ∩ X8, X7 ∩ X8, X7 ∩ x9, X7 ∩ X10, X8 ∩ x9, X8 ∩ X10, x9 ∩ X10 showed nonlinear enhancement, and other combinations showed bi-variable enhancement. When ≥0°C accumulated temperature and ≥10°C accumulated temperature with the strongest interpretation of single factor were combined with other factors, the interpretation of each factor was improved. Among them, the interpretation of the interaction between land use type and annual average temperature, ≥ 0°C accumulated temperature and ≥10°C accumulated temperature reached more than 40%, and the interpretation of ≥10°C accumulated temperature ∩ land use type [Q (x4 ∩ x9) = 0.447] on NDVI was the strongest. It can be seen that the interpretation of four human activity factors including population, GDP, land use type and sowing area of main crops increases significantly after combined with six climate factors. In the combination of climate factors and human activity factors, ≥ 10°C accumulated temperature ∩ land use type has the strongest interpretation for NDVI in the three northeast provinces. Within the climatic factors, the combination of ≥0°C accumulated temperature and annual precipitation has the strongest interpretation, reaching 0.335. Within the human activities factors, the land use type ∩ sowing area of main crops [Q (x9 ∩ X10) = 0.35] has the strongest interpretation for NDVI in the three northeast provinces, and the interpretation of GDP with the weakest interpretation of single factor increases significantly after combined with other factors.
3.2.4 Analysis of suitable range of detection factors
Based on the geographic detector method, the suitable types or ranges of climatic factors and human activity factors on vegetation growth were analyzed (Figure 9), and the results of each factor passed the statistical test at 95% confidence. Risk detection can study the appropriate range for various factors to promote vegetation growth. The greater the NDVI value of vegetation, the more suitable the characteristics of this factor for vegetation growth. As shown in the Figure 9, the NDVI value is the highest when the annual average temperature is—0.9–1.5°C and the lowest when the annual average temperature is 6.4–11.1°C; The mean value of the NDVI reached a maximum when the annual precipitation was 967–1,504.9 mm. It can be seen that good hydrothermal combination is a necessary condition for vegetation NDVI growth. Compared with the high accumulated temperature, the vegetation grows better when the accumulated temperature is low. The possible explanation is that the increase of temperature will reduce the soil water content, which has an adverse impact on the growth of vegetation. When the aridity is the lowest and the moisture index is high, it is conducive to vegetation growth; Among the land use types, the NDVI value of woodland is the highest, which is mainly distributed in the north end of Daxing’an Mountains, Xiaoxing’an Mountains and Changbai Mountain. These areas have a wide forest area and high vegetation coverage; In addition, when the population is small (417000–77000 people) and the GDP level is the lowest (138.6–33.64 billion yuan km−2), the NDVI value is higher. This is because there is little human intervention and the surface vegetation maintains the original natural state.
3.3 NDVI change trend and future trend
3.3.1 Analysis of NDVI change trend
Combined with Theil-Sen median trend analysis and Mann-Kendall test, this study monitors the change trend of NDVI in the three northeast provinces. We reclassify the Sen trend into three regions, they are stable region (−0.0005 < SNDVI < 0.0005), improved region (SNDVI ≥ 0.0005) and degraded region (SNDVI < 0.0005). The significance of Mann-Kendall test results at the 0.05 confidence level is reclassified as significant change (z > 1.96 or Z <−1.96) and insignificant change (−1.96 ≤ Z ≤ 1.96) (Figure 9). Finally, the final trend division diagram is obtained through the superposition calculation of the ArcGIS grid calculator (Figure 10). On the whole, NDVI in the three northeast provinces shows a good trend, and the vegetation improvement area is much larger than the vegetation degradation area. The area of obvious NDVI improvement is the largest, accounting for 42.9% of the total area, followed by slight improvement area, accounting for 35.7% of the total area, and the stable area, slight degradation area and serious degradation area are small, accounting for 11.7%, 8%, 1.7% of the total area respectively.
The vegetation improvement area occupies most of the three northeast provinces, of which the areas with obvious vegetation improvement are mainly distributed in the north and west of Heilongjiang Province, the west of Suihua City, Yanbian Korean Autonomous Prefecture and Baishan City in the West and east of Jilin Province. Southeast of Liaoning Province. Areas with slight vegetation improvement are distributed all over the three northeast provinces, with the significantly improved areas as the boundary and distributed inward. The stable areas mostly occur in the junction of Heihe City, Qiqihar city and Suihua City in Heilongjiang Province and in Jilin Province and the central part of Liaoning Province. The degraded area accounts for a small proportion of the overall area, of which the slightly degraded areas are scattered in the three northeast provinces, and are partially concentrated in Shenyang City, Jinzhou City, Panjin City, Liaoyang City and the west of Anshan City in Liaoning Province. The severely degraded areas are mainly located in the west of Harbin, the northwest of Changchun, the south of Shenyang and the coastal area of Dalian.
3.3.2 NDVI stability analysis
The interannual variation of NDVI in the three northeast provinces from 2001 to 2020 is generally stable, with CV value of 0.01–4.47 and average value of 0.057. The interannual NDVI of the three northeast provinces was reclassified into five grades by using the geometric interval segmentation method (Table 4). It can be seen from Figure 11 that there are obvious spatial differences in NDVI of vegetation in the three northeast provinces. The fluctuation pattern is low in the middle east and high in the west. The extremely low fluctuation area and the low fluctuation area mostly coincide, which are roughly distributed in most areas of Heilongjiang Province, Southeast Jilin Province and northeast Liaoning Province. The moderate fluctuation area is roughly distributed on both sides of the low fluctuation area. The high volatility areas are located in the Midwest and northeast of Heilongjiang Province, the northwest of Jilin Province, the northwest of Liaoning Province, Panjin City, Dalian City, Daqing City, Harbin City, the northwest of Jilin Province and the coast of Liaodong Bay, and sporadically distributed with areas with high volatility.
3.3.3 Future trend analysis
This study uses MATLAB to calculate the Hurst index of the three northeast provinces. The Hurst index of NDVI in the three northeast provinces is low, with maximum, minimum, and mean values of 0.88, 0.1 and 0.44, respectively. The proportion of areas with a Hurst index less than 0.5 was 76.21%, while that of areas greater than 0.5 was 23.79%, indicating that the reversal of the vegetation trend in the future is likely in most areas of the three northeast provinces. This shows that the change trend of NDVI in most areas of the three northeast provinces in the future is opposite to that in the past 20 years, and the degraded area is greater than the improved area. In order to further study the future trend of NDVI in the three northeast provinces, the NDVI trends were coupled with the Hurst index classification results to obtain the spatial distribution of future changes of vegetation cover in the three northeast provinces (Figure 12), and the future change trends were classified into the following six levels: continuous improvement accounting for 19.73% of the total area and are distributed evenly in the three northeast provinces. The area from increase to decrease accounting for 64.91% of the total area, are concentrated in other areas except Jinzhou City, Shenyang City, Fuxin City and Liaoyang City. The area of continuous degradation accounts for 3.73% of the total area, scattered in the three northeast provinces, it is concentrated in Jinzhou City, Shenyang City, Fuxin City, Liaoyang City and Dalian coastal area. Meanwhile, the area from decreasing to increasing accounts for 10.24% of the total area, which is mainly distributed in the west of Heihe City, the border area between Harbin and Changchun, the middle of Mudanjiang, Shenyang, Liaoyang, Anshan, Panjin and the coastal area of Dalian. The random change area accounts for 0.05% of the total area, which is scattered in the three northeast provinces; Furthermore, there are few areas with no significant change, accounting for 1.34% of the total area.
4 Discussion
4.1 Discussion on the spatiotemporal dynamics of NDVI in the three northeast provinces
The NDVI values in the three northeast provinces showed a fluctuating increase trend from 2001 to 2020, with strong spatial heterogeneity. Meanwhile, most areas of the three northeast provinces are affected by the southeast monsoon and the precipitation on the windward slope of Daxing’an Mountains and Changbai Mountains, which are conducive to the growth of vegetation. This is in good agreement with the research results of Lin et al. (2020) and Yuan et al. (2015). Additionally, the overall good situation of vegetation is enough to prove that the fourth and fifth phases of the three North Shelterbelt plans proposed by China have achieved good results (Figure 3). However, the increased frequency and intensity of global extreme climate events, can lead to significant downward trend in vegetation cover, especially for the vegetation NDVI in the high latitudes of the northern hemisphere (Wang et al, 2016). It can be seen that NDVI decreased significantly in 2009, which is related to the severe snow disaster in 2008, and the response of vegetation growth to climate change has a lag effect (Na et al, 2018). Therefore, NDVI of vegetation showed a downward trend in 2009. The breakpoint appeared again in 2013, which is related to the flood of northeast China in 2013. The flood caused serious damage to vegetation and significantly reduced vegetation coverage. The obvious decline in 2019 is considered to be due to the impact of El Nino, alternating drought and flood disasters in 2019, and the growth capacity of vegetation is reduced due to the impact of extreme climate events (Xu et al, 2019).
4.2 Discussion on the results of geographical detectors in the three northeast provinces
The results of factor detection show that climate factors and human activity factors jointly affect vegetation differentiation. Among the climate factors, temperature has stronger interpretation than precipitation, which is closely related to the high latitude of the three northeast provinces and their climate characteristics. This research conclusion is consistent with the research results of Mao et al. (2012) and Mao et al., 2012. The interpretation of human activities is lower than that of climate factors, but the interpretation of land use types is higher, which indicates that the impact of human activities on NDVI is mainly reflected in the change of land use mode, which is consistent with the results of Nie et al. (2021). This may be attributed to the economic development, the improvement of human consciousness, the enhancement of human ability to transform the natural environment, and the land use distribution pattern changes with human activities. Interactive detection shows that the interpretation after interaction is more significant than that of single factor, which further proves that the NDVI change of vegetation in the three northeast provinces is mainly affected by climate factors and human activity factors (Ma et al, 2019; Zheng et al., 2019; Sun et al, 2020). The risk detection indicates that the vegetation grows well under good hydrothermal conditions and small human intervention, consistent with findings of Li et al. (2018).
4.3 Discussion on NDVI of future vegetation in three northeast provinces
Vegetation trends in the future present reverse persistence in the three northeast provinces. This indicates that the degraded area is greater than the improved area, which is consistent with the relevant research results of Yuan et al., 2020, With global warming and the sudden and frequent occurrence of extreme climate events, the temperature increases, the precipitation decreases (Peng et al, 2012), the evaporation increases, and the groundwater level decreases, which creates adverse conditions for vegetation growth. At the same time, it will inevitably have a significant impact on the three northeast provinces where the temperature dominates the distribution of vegetation. In addition, human transformation of the natural environment has gradually transformed cultivated land and grassland into construction land, and vegetation coverage has been reduced (Yin et al, 2020).
4.4 Advantages and limitations of the study
Based on the above, this study has certain advantages and limitations. MODIS data with large amount of data, many bands, wide application range and high precision are selected for the research data, which ensures the quality of the data to a certain extent; In terms of time scale, the selected NDVI data from 2001 to 2020 are of great practical significance; On the spatial scale, the three northeast provinces are selected as the research area, which enriches the research results of the research theme in the region. Previously, the research on the three northeast provinces mostly focused on Liaoning Province, Heilongjiang province or Jilin Province, and there are few studies on the three northeast provinces as a whole; In addition, from the perspective of multiple factors, this study uses geographic detectors to explore the factors affecting NDVI. Compared with correlation analysis (Nanzad et al, 2019) geographic detectors have certain advantages, which can analyze the influence of multiple factors on NDVI, as well as the influence and suitability range after factor interaction. The influencing factors are from two aspects, comprehensively considering meteorological factors and human activities (Guo et al, 2021), which are of great practical significance for regional ecological protection and vegetation restoration. However, the altitude and geomorphic factors in the natural factors are not considered, which can be emphasized in the later research, so as to make the analysis of the influencing factors of NDVI in the three northeast provinces more comprehensive. In addition, in view of the frequent occurrence of extreme climate events and the strengthening of human intervention in the natural environment, the development of efficient prediction models will be the focus of future work (Li et al, 2021).
5 Conclusion
Considering the three northeast provinces as the study area, based on Meteorological background data, socio-economic data, farmland management data and MODIS-NDVI, with the help of geographic detector model, MATLAB, ArcGIS, and using the methods of center of gravity transfer, geographic detector, coefficient of variation, Sen + MK trend and Hurst index, this study explored the temporal and spatial evolution law, driving factors and future development trend of NDVI. The main conclusions are as follows:
1) Considering the temporal trends in NDVI, the NDVI values in 2001–2020 in the three northeast provinces show an overall increasing trend in the range of 0.79–0.84, with small fluctuations and a growth rate of 0.16 × 10−3/year. Spatially, the annual average NDVI has great spatial heterogeneity, showing a pattern of high in the east, low in the west, high in the north and low in the south. Among them, the areas with high vegetation coverage and medium and high vegetation coverage are large, and the vegetation NDVI in the three northeast provinces is growing well. The center of gravity of each vegetation coverage grade has obviously moved in all directions from 2001 to 2020.
2) The influence of climate factors is greater than that of human activities, and human activities increase the interpretation of climate factors. The influence of each factor is ranked as: ≥10°C accumulated temperature, ≥ 0°C accumulated temperature, average annual temperature, land use type, aridity, moisture index, population, sowing area of main crops, annual precipitation, GDP, and temperature dominated the distribution of vegetation NDVI in the three northeast provinces. Nevertheless, the interactions between factors exhibited mostly bi-variable enhancement and nonlinear enhancement. The interaction of the two factors enhances the influence of a single factor. The combination with the strongest interpretation is ≥10°C accumulated temperature ∩ land use type, and the interpretation is 0.447.
3) From 2001 to 2020, the overall fluctuation of NDVI in the three northeast provinces is small and has favorable stability, and the vegetation improvement area is much larger than the vegetation degradation area. According to Hurst’s prediction results, the future change trend of NDVI in the three northeast provinces has the characteristics of reverse persistence, that is, the degraded area will be greater than the improved area.
Data availability statement
The original contributions presented in the study are included in the article/supplementary material, further inquiries can be directed to the corresponding author.
Author contributions
RW led the conception and design of the paper. YW led the literature review and data collection. YW and BL are responsible for method research and data processing. XL provided funding for this article. RW and XL led the review and editing of the paper.
Funding
This research is supported by the National Natural Science Foundation of China (NSFC) project (41671158); in part by the National Natural Science Foundation of China international (Regional) Cooperation and Exchange Program (41961144019); in part by the Natural Science Youth Project of Liaoning Education Department (LQ2020026); in part by Dalian Science and Technology Star Project in 2021 (2021RQ101).
Acknowledgments
The authors gratefully thank the School of Geographical Sciences, Liaoning Normal University, for providing the facilities during this research work and for supporting the related research.
Conflict of interest
The authors declare that the research was conducted in the absence of any commercial or financial relationships that could be construed as a potential conflict of interest.
Publisher’s note
All claims expressed in this article are solely those of the authors and do not necessarily represent those of their affiliated organizations, or those of the publisher, the editors and the reviewers. Any product that may be evaluated in this article, or claim that may be made by its manufacturer, is not guaranteed or endorsed by the publisher.
References
Baniya, B., Tang, Q., Huang, Z., Sun, S., and Techato, K. A. (2018). Spatial and temporal variation of NDVI in response to climate change and the implication for carbon dynamics in Nepal. Forests 9 (6), 329. doi:10.3390/f9060329
Chen, T., Xia, J., Zou, L., and Hong, S. (2020). Quantifying the influences of natural factors and human activities on NDVI changes in the Hanjiang river basin, China. Remote Sens. 12 (22), 3780. doi:10.3390/rs12223780
Cheng, Q., Zhong, F., and Wang, P. (2021). Potential linkages of extreme climate events with vegetation and large-scale circulation indices in an endorheic river basin in northwest China. Atmos. Res. 247, 105256. doi:10.1016/j.atmosres.2020.105256
Chu, H., Venevsky, S., Wu, C., and Wang, M. (2019). NDVI-based vegetation dynamics and its response to climate changes at Amur-Heilongjiang River Basin from 1982 to 2015. Sci. Total Environ. 650, 2051–2062. doi:10.1016/j.scitotenv.2018.09.115
Chuai, X. W., Huang, X. J., Wang, W. J., and Bao, G. (2013). NDVI, temperature and precipitation changes and their relationships with different vegetation types during 1998–2007 in Inner Mongolia, China. Int. J. Climatol. 33 (7), 1696–1706. doi:10.1002/joc.3543
Cong, P., Chen, K., Qu, L., and Han, J. (2019). “Temporal and spatial changes of the yellow river delta wetland based on multi-source data during 30 years,” in Proceedings of the tiangong-2 remote sensing application conference (Singapore: Springer), 286–299. doi:10.1007/978-981-13-3501-3-27
Dai, X., Yao, L., Yuan, Z., and Sun, K. (2021). Characteristics of NDVI temporal and spatial variation in the source area of the yangtze river and its response to hydrothermal conditions IOP conference series: Earth and environmental science. IOP Conf. Ser. Earth Environ. Sci. 768, 012013. doi:10.1088/1755-1315/768/1/012013
Dehua, M., Zongming, W., Ling, L., and Guang, Y. (2012). Correlation analysis between NDVI and climate in northeast China based on AVHRR and GIMMS data sources. Remote Sens. Technol. Appl. 27 (1), 77–85. doi:10.11873/j.issn.1004-0323.2012.1.77
Dong, L., Zhang, L., and Li, F. (2015). Developing additive systems of biomass equations for nine hardwood species in Northeast China. Trees 29 (4), 1149–1163. doi:10.1007/s00468-015-1196-1
Du, J. L., Liu, Y., and Diao, W. X. (2019). Assessing regional differences in green innovation efficiency of industrial enterprises in China. Int. J. Environ. Res. Public Health 16 (6), 940. doi:10.3390/ijerph16060940
Eastman, J. R., Sangermano, F., Machado, E. A., Rogan, J., and Anyamba, A. (2013). Global trends in seasonality of normalized difference vegetation index (NDVI), 1982–2011. Remote Sens. 5 (10), 4799–4818. doi:10.3390/rs5104799
Fensholt, R., and Proud, S. R. (2012). Evaluation of earth observation based global long term vegetation trends—comparing GIMMS and MODIS global NDVI time series. Remote Sens. Environ. 119, 131–147. doi:10.1016/j.rse.2011.12.015
Gao, X., Huang, X., Lo, K., Dang, Q., and Wen, R. (2021). Vegetation responses to climate change in the qilian mountain nature reserve, northwest China. Glob. Ecol. Conservation 28, e01698. doi:10.1016/j.gecco.2021.e01698
Guo, E., Wang, Y., Wang, C., Sun, Z., Bao, Y., Mandula, N., et al. (2021). NDVI indicates long-term dynamics of vegetation and its driving forces from climatic and anthropogenic factors in Mongolian plateau. Remote Sens. 13 (4), 688. doi:10.3390/RS13040688
Guo, J., Hu, Y., Xiong, Z., Yan, X., Li, C., and Bu, R. (2017). Variations in growing-season NDVI and its response to permafrost degradation in Northeast China. Sustainability 9 (4), 551. doi:10.3390/su9040551
Guo, Z. X., Wang, Z. M., Song, K. S., Zhang, B., Li, F., and Liu, D. W. (2007). Correlations between forest vegetation NDVI and water/thermal condition in Northeast China forest regions in 1982–2003. Chin. J. Ecol. 26 (12), 1930–1936. doi:10.13292/j.1000-4890.2007.0349
Han, X., Zuo, D., Xu, Z., Cai, S., and Gao, X. (2018). Analysis of vegetation condition and its relationship with meteorological variables in the Yarlung Zangbo River Basin of China. Proc. IAHS. 379, 105–112. doi:10.5194/piahs-379-105-2018
He, P., Xu, L., Liu, Z., Jing, Y., and Zhu, W. (2021). Dynamics of NDVI and its influencing factors in the Chinese Loess Plateau during 2002–2018. Reg. Sustain. 2 (1), 36–46. doi:10.1016/J.REGSUS.2021.01.002
Huang, X., Zhang, T., Yi, G., He, D., Zhou, X., Li, J., et al. (2019). Dynamic changes of NDVI in the growing season of the Tibetan plateau during the past 17 years and its response to climate change. Int. J. Environ. Res. Public Health 16 (18), 3452. doi:10.3390/ijerph16183452
Huo, H., and Sun, C. (2021). Spatiotemporal variation and influencing factors of vegetation dynamics based on geodetector: A case study of the northwestern yunnan plateau, China. Ecol. Indic. 130, 108005. doi:10.1016/j.ecolind.2021.108005
Jiang, L., Bao, A., Guo, H., and Ndayisaba, F. (2017). Vegetation dynamics and responses to climate change and human activities in Central Asia. Sci. Total Environ. 599, 967–980. doi:10.1016/j.scitotenv.2017.05.012
Jiang, W., Yuan, L., Wang, W., Cao, R., Zhang, Y., and Shen, W. (2015). Spatio-temporal analysis of vegetation variation in the yellow river basin. Ecol. Indic. 51, 117–126. doi:10.1016/j.ecolind.2014.07.031
Kaku, K. (2019). Satellite remote sensing for disaster management support: A holistic and staged approach based on case studies in sentinel asia. Int. J. Disaster Risk Reduct. 33, 417–432. doi:10.1016/j.ijdrr.2018.09.015
Li, J., Wang, J., Zhang, J., Zhang, J., and Kong, H. (2021). Dynamic changes of vegetation coverage in China-Myanmar economic corridor over the past 20 years. Int. J. Appl. Earth Observation Geoinformation 102, 102378. doi:10.1016/J.JAG.2021.102378
Li, K., Tong, Z., Liu, X., Zhang, J., and Tong, S. (2020). Quantitative assessment and driving force analysis of vegetation drought risk to climate change: Methodology and application in Northeast China. Agric. For. Meteorology 282, 107865. doi:10.1016/j.agrformet.2019.107865
Li, W., Li, C., Liu, X., He, D., Bao, A., Yi, Q., et al. (2018). Analysis of spatial-temporal variation in NPP based on hydrothermal conditions in the Lancang-Mekong River Basin from 2000 to 2014. Environ. Monit. Assess. 190 (6), 321–415. doi:10.1007/s10661-018-6690-7
Li, Y., Zhao, Z., Wang, L., Li, G., Chang, L., and Li, Y. (2021). Vegetation changes in response to climatic factors and human activities in Jilin province, China, 2000–2019. Sustainability 13 (16), 8956. doi:10.3390/SU13168956
Lin, X., Niu, J., Berndtsson, R., Yu, X., Zhang, L., and Chen, X. (2020). NDVI dynamics and its response to climate change and reforestation in Northern China. Remote Sens. 12 (24), 4138. doi:10.3390/rs12244138
Liu, X., Tian, Z., Zhang, A., Zhao, A., and Liu, H. (2019). Impacts of climate on spatiotemporal variations in vegetation NDVI from 1982–2015 in Inner Mongolia, China. Sustainability 11 (3), 768. doi:10.3390/su11030768
Liu, Y., Li, Y., Li, S., and Motesharrei, S. (2015). Spatial and temporal patterns of global NDVI trends: Correlations with climate and human factors. Remote Sens. 7 (10), 13233–13250. doi:10.3390/rs71013233
Liu, Y., Tian, J., Liu, R., and Ding, L. (2021). Influences of climate change and human activities on NDVI changes in China. Remote Sens. 13 (21), 4326. doi:10.3390/rs13214326
Ma, Q., Long, Y., Jia, X., Wang, H., and Li, Y. (2019). Vegetation response to climatic variation and human activities on the Ordos Plateau from 2000 to 2016. Environ. Earth Sci. 78 (24), 709–715. doi:10.1007/s12665-019-8732-z
Ma, Z., Guo, J., Li, W., Cai, Z., and Cao, S. (2021). Regional differences in the factors that affect vegetation cover in China. Land Degrad. Dev. 32 (5), 1961–1969. doi:10.1002/LDR.3847
Mao, D., Wang, Z., Liu, D., and Song, K. (2012). “Responses of forest NDVI in Northeast China to precipitation change,” in Advanced technology in teaching-proceedings of the 2009 3rd international conference on teaching and computational science (WTCS 2009) (Berlin, Heidelberg: Springer), 849–856. doi:10.1007/978-3-642-25437-6-114
Mao, D., Wang, Z., Luo, L., and Ren, C. (2012). Integrating AVHRR and MODIS data to monitor NDVI changes and their relationships with climatic parameters in Northeast China. Int. J. Appl. Earth Observation Geoinformation 18, 528–536. doi:10.1016/j.jag.2011.10.007
Meng, X., Gao, X., Li, S., and Lei, J. (2020). Spatial and temporal characteristics of vegetation NDVI changes and the driving forces in Mongolia during 1982–2015. Remote Sens. 12 (4), 603. doi:10.3390/rs12040603
Mo, X., Liu, S., Lin, Z., Wang, S., and Hu, S. (2015). Trends in land surface evapotranspiration across China with remotely sensed NDVI and climatological data for 1981–2010. Hydrological Sci. J. 60 (12), 2163–2177. doi:10.1080/02626667.2014.950579
Na, L., Na, R., Zhang, J., Tong, S., Shan, Y., Ying, H., et al. (2018). Vegetation dynamics and diverse responses to extreme climate events in different vegetation types of inner Mongolia. Atmosphere 9 (10), 394. doi:10.3390/atmos9100394
Nanzad, L., Zhang, J., Tuvdendorj, B., Nabil, M., Zhang, S., and Bai, Y. (2019). NDVI anomaly for drought monitoring and its correlation with climate factors over Mongolia from 2000 to 2016. J. arid Environ. 164, 69–77. doi:10.1016/j.jaridenv.2019.01.019
Nie, T., Dong, G., Jiang, X., and Lei, Y. (2021). Spatio-temporal changes and driving forces of vegetation coverage on the loess plateau of Northern Shaanxi. Remote Sens. 13 (4), 613. doi:10.3390/RS13040613
Ning, J., Liu, J., Kuang, W., Xu, X., Zhang, S., Yan, C., et al. (2018). Spatiotemporal patterns and characteristics of land-use change in China during 2010–2015. J. Geogr. Sci. 28 (5), 547–562. doi:10.1007/s11442-018-1490-0
Ouyang, D., Zhu, X., Liu, X., He, R., and Wan, Q. (2021). Spatial differentiation and driving factor Analysis of urban construction land change in county-level city of guangxi, China. Land 10 (7), 691. doi:10.3390/LAND10070691
Pan, S., Zhao, X., and Yue, Y. (2019). Spatiotemporal changes of NDVI and correlation with meteorological factors in northern China from 1985-2015. E3S Web of Conferences, 131. France: EDP Sciences, 01040. doi:10.1051/e3sconf/201913101040
Pei, Z., Fang, S., Yang, W., Wang, L., Wu, M., Zhang, Q., et al. (2019). The relationship between NDVI and climate factors at different monthly time scales: A case study of grasslands in inner Mongolia, China (1982–2015). Sustainability 11 (24), 7243. doi:10.3390/su11247243
Peng, J., Dong, W., Yuan, W., and Zhang, Y. (2012). Responses of grassland and forest to temperature and precipitation changes in Northeast China. Adv. Atmos. Sci. 29 (5), 1063–1077. doi:10.1007/s00376-012-1172-2
Peng, W., Kuang, T., and Tao, S. (2019). Quantifying influences of natural factors on vegetation NDVI changes based on geographical detector in Sichuan, western China. J. Clean. Prod. 233, 353–367. doi:10.1016/j.jclepro.2019.05.355
Qian, H., Yuan, X. Y., and Chou, Y. L. (2003). “Forest vegetation of northeast China,” in Forest vegetation of northeast Asia (Dordrecht: Springer), 181–230. doi:10.1007/978-94-017-0143-3_6
Qu, S., Wang, L., Lin, A., Zhu, H., and Yuan, M. (2018). What drives the vegetation restoration in yangtze river basin, China: Climate change or anthropogenic factors? Ecol. Indic. 90, 438–450. doi:10.1016/j.ecolind.2018.03.029
Roy, D. P., Kovalskyy, V., Zhang, H. K., Vermote, E. F., Yan, L., Kumar, S. S., et al. (2016). Characterization of Landsat-7 to Landsat-8 reflective wavelength and normalized difference vegetation index continuity. Remote Sens. Environ. 185, 57–70. doi:10.1016/j.rse.2015.12.024
Sun, Y. L., Shan, M., Pei, X. R., Zhang, X. K., and Yang, Y. L. (2020). Assessment of the impacts of climate change and human activities on vegetation cover change in the Haihe River basin, China. Phys. Chem. Earth, Parts A/B/C 115, 102834. doi:10.1016/j.pce.2019.102834
Wang, B., and Cheng, W. (2022). Effects of land use/cover on regional habitat quality under different geomorphic types based on InVEST model. Remote Sens. 14 (5), 1279. doi:10.3390/RS14051279
Wang, B., Xu, G., Li, P., Li, Z., Zhang, Y., Cheng, Y., et al. (2020). Vegetation dynamics and their relationships with climatic factors in the Qinling Mountains of China. Ecol. Indic. 108, 105719. doi:10.1016/j.ecolind.2019.105719
Wang, F., Wang, X., Zhao, Y., and Yang, Z. (2014). Correlation analysis of NDVI dynamics and hydro-meteorological variables in growth period for four land use types of a water scarce area. Earth Sci. Inf. 7 (3), 187–196. doi:10.1007/s12145-013-0139-x
Wang, M., Fu, J. E., Wu, Z., and Pang, Z. (2020). Spatiotemporal variation of NDVI in the vegetation growing season in the source region of the Yellow River, China. ISPRS Int. J. Geoinf. 9 (4), 282. doi:10.3390/ijgi9040282
Wang, S., Yang, B., Yang, Q., Lu, L., Wang, X., and Peng, Y. (2016). Temporal trends and spatial variability of vegetation phenology over the Northern Hemisphere during 1982-2012. PloS one 11 (6), e0157134. doi:10.1371/journal.pone.0157134
Wang, Y., Shen, X., Jiang, M., and Lu, X. (2020). Vegetation change and its response to climate change between 2000 and 2016 in marshes of the Songnen Plain, Northeast China. Sustainability 12 (9), 3569. doi:10.3390/su12093569
Wang, Y., Zhang, Z., and Chen, X. (2021). Quantifying influences of natural and anthropogenic factors on vegetation changes based on geodetector: A case study in the poyang lake basin, China. Remote Sens. 13 (24), 5081. doi:10.3390/rs13245081
Wang, Y., Zhang, Z., and Chen, X. (2021). Quantifying influences of natural and anthropogenic factors on vegetation changes based on geodetector: A case study in the poyang lake basin, China. Remote Sens. 13 (24), 5081. doi:10.3390/RS13245081
Wang, Z. M., Guo, Z. X., Song, K. S., Luo, L., Zhang, B., Liu, D. W., et al. (2009). Responses of vegetation NDVI in northeast China to climate change. Chin. J. Ecol. 28 (6), 1041–1048. doi:10.13292/j.1000-4890.2009.0166
Xu, C., McDowell, N. G., Fisher, R. A., Wei, L., Sevanto, S., Christoffersen, B. O., et al. (2019). Increasing impacts of extreme droughts on vegetation productivity under climate change. Nat. Clim. Chang. 9 (12), 948–953. doi:10.1038/s41558-019-0630-6
Xue, L., Kappas, M., Wyss, D., Wang, C., Putzenlechner, B., Thi, N. P., et al. (2022). Assessment of climate change and human activities on vegetation development in Northeast China. Sensors 22 (7), 2509. doi:10.3390/s22072509
Yang, J., Wan, Z., Borjigin, S., Zhang, D., Yan, Y., Chen, Y., et al. (2019). Changing trends of NDVI and their responses to climatic variation in different types of grassland in inner Mongolia from 1982 to 2011. Sustainability 11 (12), 3256. doi:10.3390/su11123256
Yang, Y., Wang, S., Bai, X., Tan, Q., Li, Q., Wu, L., et al. (2019). Factors affecting long-term trends in global NDVI. Forests 10 (5), 372. doi:10.3390/f10050372
Yin, D., Li, X., Li, G., Zhang, J., and Yu, H. (2020). Spatio-temporal evolution of land use transition and its eco-environmental effects: A case study of the yellow river basin, China. Land 9 (12), 514. doi:10.3390/LAND9120514
Yuan, J., Bian, Z., Yan, Q., Gu, Z., and Yu, H. (2020). An approach to the temporal and spatial characteristics of vegetation in the growing season in western China. Remote Sens. 12 (6), 945. doi:10.3390/rs12060945
Yuan, W., Wu, S. Y., and Hou, S. (2020). “Projecting future vegetation change for northeast China using CMIP6,” in EGU general assembly conference abstracts, 9639. doi:10.5194/egusphere-egu2020-9639
Yuan, X., Li, L., Chen, X., and Shi, H. (2015). Effects of precipitation intensity and temperature on NDVI-based grass change over Northern China during the period from 1982 to 2011. Remote Sens. 7 (8), 10164–10183. doi:10.3390/rs70810164
Zhang, T., Cao, G., Cao, S., Zhang, X., Zhang, J., and Han, G. (2017). Dynamic assessment of the value of vegetation carbon fixation and oxygen release services in Qinghai Lake basin. Acta Ecol. Sin. 37 (2), 79–84. doi:10.1016/j.chnaes.2016.12.005
Zhang, Y., Gao, J., Liu, L., Wang, Z., Ding, M., and Yang, X. (2013). NDVI-based vegetation changes and their responses to climate change from 1982 to 2011: A case study in the koshi river basin in the middle himalayas. Glob. Planet. Change 108, 139–148. doi:10.1016/j.gloplacha.2013.06.012
Zhang, Y., He, Y., Li, Y., and Jia, L. (2022). Spatiotemporal variation and driving forces of NDVI from 1982 to 2015 in the Qinba Mountains, China. Environ. Sci. Pollut. Res. 29, 52277–52288. doi:10.1007/s11356-022-19502-6
Zhang, Y., He, Y., Li, Y., and Jia, L. (2021). China. doi:10.21203/rs.3.rs-415051/v1Temporal and spatial changes and driving forces of NDVI from 1982-2015 in qinba Mountains
Zheng, H., Shen, G., He, X., Yu, X., Ren, Z., and Zhang, D. (2015). Spatial assessment of vegetation vulnerability to accumulated drought in Northeast China. Reg. Environ. Change 15 (8), 1639–1650. doi:10.1007/s10113-014-0719-4
Zheng, K., Wei, J. Z., Pei, J. Y., Cheng, H., Zhang, X. L., Huang, F. Q., et al. (2019). Impacts of climate change and human activities on grassland vegetation variation in the Chinese Loess Plateau. Sci. Total Environ. 660, 236–244. doi:10.1016/j.scitotenv.2019.01.022
Zheng, K., Wei, J. Z., Pei, J. Y., Cheng, H., Zhang, X. L., Huang, F. Q., et al. (2019). Impacts of climate change and human activities on grassland vegetation variation in the Chinese Loess Plateau. Sci. Total Environ. 660, 236–244. doi:10.1016/j.scitotenv.2019.01.022
Zhou, Z., Ding, Y., Shi, H., Cai, H., Fu, Q., Liu, S., et al. (2020). Analysis and prediction of vegetation dynamic changes in China: Past, present and future. Ecol. Indic. 117, 106642. doi:10.1016/j.ecolind.2020.106642
Keywords: the three northeast provinces, normalized vegetation index, geographic detectors, future trends, climate change
Citation: Wu R, Wang Y, Liu B and Li X (2022) Spatial-temporal changes of NDVI in the three northeast provinces and its dual response to climate change and human activities. Front. Environ. Sci. 10:974988. doi: 10.3389/fenvs.2022.974988
Received: 21 June 2022; Accepted: 10 August 2022;
Published: 30 August 2022.
Edited by:
Peng Liu, Institute of Remote Sensing and Digital Earth (CAS), ChinaReviewed by:
Enliang Guo, Inner Mongolia Normal University, ChinaPir Mohammad, Hong Kong Polytechnic University, Hong Kong SAR, China
Copyright © 2022 Wu, Wang, Liu and Li. This is an open-access article distributed under the terms of the Creative Commons Attribution License (CC BY). The use, distribution or reproduction in other forums is permitted, provided the original author(s) and the copyright owner(s) are credited and that the original publication in this journal is cited, in accordance with accepted academic practice. No use, distribution or reproduction is permitted which does not comply with these terms.
*Correspondence: Xueming Li, bGl4dWVtaW5nOTk5QDE2My5jb20=