- 1Department of Environmental Science and Geology, Wayne State University, Detroit, MI, United States
- 2Department of Civil and Environmental Engineering, Wayne State University, Detroit, MI, United States
Inhalation and/or ingestion of toxic heavy metals enriched in road dust have toxic effects on humans. From inhalation and ingestion of dust, release of readily bioavailable elements sorbed on to dust, could lead to health issues such as lung or skin cancer, kidney dysfunction, hypertension, dermal lesions, peripheral neuropathy, and vascular disease. On 49 road dust and soil samples collected in the Metro Detroit area, we analyzed a suite of trace metals in <63 μm and size-separated fractions to quantify the extent of metal enrichment and contamination status. We evaluated Enrichment Factor (EF), Pollution Index (PI), Geoaccumulation Index (GI), and Pollution Load Index (PLI). The average Chromium (Cr) concentration of 198 ppm is the highest reported for any global city in literature while in ∼80% samples, Barium (Ba), Cr, Lead (Pb), and Zinc (Zn) concentrations exceeded the USEPA limit. The EF for Barium (Ba) was found to be ≥9 in all samples, and for the rest of other elements was >1.5. The PI values for Cr, Ba, Zinc (Zn), Lead (Pb), and Selenium (Se) indicate elevated levels and for Ba, Cr, and Zn up to a moderate level. PLI values in >50% of road dusts were ≥3, indicating many sites are ‘heavily polluted’. The PLI value in Detroit road dust is higher than those reported for some other global cities including Shanghai. Observed strong correlations between Cr-Zn and Cadmium (Cd)-Silver (Ag) likely indicate a common metal source. Cluster analysis of data for Cd, Arsenic (As), Pb, and Zn appears to be distinct between different proximally related clusters, while Nickel (Ni) and Ba are potentially more distinct between property type clusters. In general, metal concentrations of industrial samples are more commonly distinct with over half of metals (≥6 of 11) differing from residential and/or soil samples. Three generalized “groups” were identified from the combination of hierarchical cluster and PCA analyses followed by varimax rotation: Group 1 characterized by Cr, Cd, Ni, Ag, Zn, and Copper (Cu); Group 2 characterized by Pb, As, Mercury (Hg), and minor Ba; and Group 3 characterized by Se. This is the first study to report all four metrics of metal pollution along with rigorous statistical analysis.
1 Introduction
Detroit, a sprawling post-industrial city interwoven with a network of roads and highways, has numerous possible sources of organic and inorganic pollutants. Heavy metals (defined as metals with densities >5 g cm−3 and include Pb, Cr, Cd, As, Hg, Ni, Zn, and Cu) are of particular concern. Detroit became a world-class industrial powerhouse during the early 1920s due to a booming auto industry, and grew to become the fourth-largest city in the United States. This growth was accompanied with large scale industrial pollution. One possible source of metal pollution that has garnered much attention in recent years is fugitive road dust. Fugitive dust is generally defined as “natural or human-associated dusts (particulate only) that become airborne due to the forces of wind, human activity, or both” (USEPA, 1975). Road dusts are defined in this study as solid particulate materials, <63 μm in size, that are deposited on road surfaces. These dusts are a complex mélange of natural and anthropogenic materials that are in a constant cycle of transport, deposition, and resuspension. Sources of heavy metals to road dust include traffic emission (e.g., vehicle exhaust aerosols, tire-wear particles, brake lining wear particles, weathered street surface particles), and industrial emission which includes chemical plants, metallurgical industry, coal combustion, oil refineries, petrochemical plants, and mining (Wei and Yang, 2010).
Heavy metals from urban road dusts and surface soils can reach the human body via ingestion, direct inhalation, and dermal contact. Exposure to heavy metals may cause various negative health outcomes at differing scales. For example, lead contamination is of particular concern with a recent global report estimating over 674,000 deaths attributable to lead exposure in 2010 (Lim et al., 2012). Excessive exposure to heavy metals can damage the nervous, skeletal, circulatory, enzymatic, endocrine, and immune systems (Li et al., 2013). The chronic effects of Cd-enriched soil dust through inhalation consist of lung cancer, chronic obstructive lung disease, and impaired pulmonary function (Nordberg et al., 2007).
Unlike many organic pollutants, heavy metals do not degrade over time but only change species and move between different reservoirs. There are two distinct sources contributing to the heavy metals in the environment: 1) from the natural weathering of the parent rocks and minerals, commonly referred as “background level” and 2) metals derived from human impacts (anthropogenic origin) which includes mining, smelting, and other industrial processes (De Miguel et al., 1997, 1999; Thorpe and Harrison, 2008; Charlesworth et al., 2011; Loganathan et al., 2013; Sappa et al., 2020). Because most of the heavy metals have higher distribution coefficients, Kd (ratio of concentrations in solid to that in the solution phase), with increasing concentrations in decreasing particle-sized solid particulate matter, the variations of metal concentration are a function of particle-size and concentrations of total organic matter (e.g., Ravichandran et al., 1995). Thus, it is helpful to normalize the metal concentration in dust samples to the average concentration of the same metal in the upper crust (Wedepohl, 1995) or to the background concentrations in the study area (i.e., pre-anthropogenic level, Murray et al., 2004).
Sources of heavy metals are grouped into point and non-point sources of pollution. In most of the road dust studies, the sources are diffused, arising from multiple sources. Point sources of heavy metal pollution such as mining, foundries, smelters, and other metal-based industrial operations, while still dangerous, are logistically less complicated to understand and remediate than non-point sources (Tchounwou et al., 2012). Prohibition of tetraethyl lead in gasoline and cessation of lead-based paint usage are examples of large-scale regulatory actions taken in the United States that have reduced two major non-point sources of lead pollution in the environment (Mielke and Reagan, 1998; Mielke et al., 2011). According to Mielke et al. (2011), the Pb contamination in Detroit was ranked 4th highest in the contiguous United States in terms of estimated Pb additives released during 1950–1982. While several measures have greatly reduced the introduction of lead into the environment, the impact of past usage still remains since the lead is stored in soil, sediment, and dusts (Wong et al., 2006; Mielke et al., 2011; Loganathan et al., 2013; Souto-Oliveira et al., 2019).
Road dust are potent media for transportation and distribution of heavy metals in urban environments (Mielke and Reagan, 1998; Denier van der Gon et al., 2013). Numerous authors around the globe have reported substantial enrichment of metals in urbanized areas with road dusts having the highest enrichment, greatest mobility of solid particulate media, and having impacts on storm water (De Miguel et al., 1997; Tong, 1998; Manta et al., 2002; Banerjee, 2003; Fakayode and Olu-Owolabi, 2003; Ferreira-Baptista and De Miguel, 2005; Wei and Yang, 2010; Apeagyei et al., 2011; Laidlaw et al., 2012; Sorenson, 2013). In this work, a suite of road dust samples from residential, commercial, and industrial sites in Detroit were collected, dried and sieved to different size fractions. From the measurements of a suite of trace metals, the present study is designed to address the following research questions:
i) How do the concentrations of As, Ba, Cd, Cr, Cu, Pb, Hg, Ni, Se, Ag, and Zn metals vary in road dust in Detroit, MI and how do these values compare with values reported for other cities in US as well as in other cities in the world? Are there any difference in the enrichment factors in bulk and size fractions for these metals?
ii) How does the pollution index, geoaccumulation index, and pollution load index vary among the samples? How do these values compare with the values reported in other cities in US and other cites globally?
iii) Are the concentrations of trace metals more distinct between proximally related or property type related clusters? and
iv) Using hierarchical cluster analysis and principal component analysis, can we identify potential contamination profiles (i.e., chemical “signatures” of contamination)?
This study provides detailed analysis of heavy metal data from a suite of sampling sites and provides insights on the contamination level and contamination profiles/patterns of road dusts. This is the first study that combines the use of four pollution metrics (enrichment factor, geoaccumulation factor, pollution index, and pollution load index), in conjunction with metal analysis in size-fractionated road dust, and utility of, descriptive statistics (correlation analysis and hypothesis testing), and multivariate statistics (PCA and cluster analysis) to provide a comprehensive analysis of heavy metal contamination of road dust in Detroit.
2 Materials and methods
2.1 Field sampling
Road dust samples were collected on 8th August 2019 and 13th September 2019 in Detroit and surrounding areas (42.2°−42.45°N, 83.0°−83.3°W). A total of 51 samples (47 dust and 4 soil) were collected with 34 samples from the first trip (coded as A, B, and C) and 17 from the second trip (coded as D and E, Figure 1, Supplementary Table S1). The sample collection sites were chosen to represent variations in current and historical land use, as estimated by property tax zoning and traffic density. Additional sites were selected based on likely major sources of aerosol particles such as trash incinerators, fuel refineries, coal fired power plants, and other industrial activities. Remaining sites were selected to provide additional spatial coverage in the region, localized sources of dust generation/transport (i.e., demolition sites and open fields), and areas that showed obvious dust collection.
Soil samples were also collected in open field areas. Four soil samples were collected approximately ∼10 m off of dust collection site and collected upper ∼10 cm of the soil. Road samples were collected using precleaned brooms and dustpans and transferred into 1-gallon LDPE plastic bags. Soil samples were collected using a shovel and stored in similar bags. Roughly 1–3 kg of dust/soil was collected for each sample, which was then labelled and double bagged. All tools were cleaned of any residual dust using distilled water, paper towel, and canned compressed air duster (1,1-difluoroethane) between successive samples. Due to rain days before the second sampling trip, many samples collected were found to be damp.
All samples were sieved with 2 mm and 63 μm sieves with only the portion <63 μm being kept for analysis (<63 μm fraction referred to as “bulk”). This size is chosen as it is the size boundary between silt and sand, with silt being more mobile with higher surface area per unit mass than sand. For example, when a surface area of 54 μm-size spherical grain undergoing weathering to several 16 μm-size spherical grains, the size of the grain decreases by a factor of 3.4 while the total surface area of the smaller grains becomes 11.4 times larger. Since sorption of anthropogenic and/or bioavailable heavy metal contaminants is surface-area dependent, a subset of 10 samples were chosen for further size-separation using 45 μm and 32 μm sieves to produce three size fractions (45–63 μm, 32–45 μm, and <32 μm).
2.2 Trace metal preparation and analysis
Trace metals were analyzed at the Activation Laboratories Ltd. using their “Ultratrace 6-Near Total Digestion-ICP and ICP-MS” package. Mercury concentrations were measured using “1G-Aqua Regia—Hg Cold Vapour FIMS” package [ALL (Activation Laboratories Ltd.,) 2020a,b]. Activations Laboratories is a SCC GLP (Standards Council of Canada, Good Laboratory Practice) compliant facility with 45 techniques certified by ISO/IEC 17025:2017 (International Organization for Standardization/International Electrotechnical Commission) certification and is FDA (Food and Drug Administration) registered and inspected. Trace metals were analyzed for all bulk and size fractionated samples from 2019 (bulk = 51; size fractionated = 30). The Ultratrace 6 method was used to determine the concentrations of 61 major and trace elements (ALL, 2020a, ALL, 2020b). Briefly, ∼0.25 g of the bulk (<63 um) road dust/soil sample was digested first by concentrated hydrofluoric acid and then a mixture of perchloric and nitric acid. Digestion was conducted in a microprocessor-controlled hotbox until incipient dryness was attained, at which time aqua regia was introduced to bring sample back into solution. Solution was then analyzed by ICP-OES for determination of major, minor and some of the trace elements (Al, Ca, Fe, K, Mg, Mn, Na, P, S, and Sc). Each batch also contained 14% quality control samples for digestion and 13% quality control for instrumental analysis which consists of 5 method reagent blanks, 10 in-house controls, 10 sample duplicates, and 8 certified reference materials. Remaining elements were determined by ICP-MS following dilution of sample solution. Quality control samples include one blank per 40 samples, one in-house control per 20 samples, digested standards per 80 samples, and one duplicate per 15 samples with the instrument being recalibrated every 80 samples.
A 0.5 g aliquot was taken from each sample and digested in aqua regia at 90°C to minimize Hg volatilization. In this solution, the Hg is considered stable as it has been oxidized to Hg2+. Stannous chloride is added to the solution to reduce the Hg2+ to elemental Hg which is preferable for its determination via the 253.7 nm absorption peak. Through the sample and reductant solution, argon is bubbled to transfer Hg atoms into the absorption cell which is then analyzed in a Perkin Elmer FIMS 100 cold vapor Hg analyzer.
Quality control measurements agreed within 20%, on average, for all elements studied with certified reference values for 14 individual reference standards (Supplementary Table S2). Additionally, all six sample and duplicate measurements agreed within an average of 20% for all elements.
2.3 Multivariate statistical analysis
Identification of possible relationships between concentrations of heavy metals and geochemical relationships in road dusts are complex due to many different factors influencing distribution of heavy metals, which hinder elucidation of geochemical and/or their origin relationships. Both descriptive and multivariate statistical analyses are commonly utilized to reveal underlying relationships between heavy metals and their geochemistry and/or their origin (Lee et al., 2006; Yongming et al., 2006; Zheng et al., 2008; Lu et al., 2010; Zhang et al., 2016). The methods used in this study are Pearson’s correlation, k-means clustering, principal component analysis (PCA), and hierarchical cluster analysis (CA). These are commonly used in environmental studies to identify potential relationships between samples and metals, potential contamination profiles, and relative strength of the relationships. Statistical analyses were performed using Matlab 2021 with the Statistics and Machine Learning Toolbox (Matlab, 2021), on As, Ba, Cd, Cr, Cu, Pb, Hg, Ni, Se, Ag, and Zn. Pearson’s correlation analysis was applied to form a correlation matrix to obtain correlation coefficient and p values between heavy metals. We consider the correlation coefficient to be significantly different from zero when p < 0.05. Geospatial clustering (k-means clustering) was performed on latitude and longitude data in MATLAB to identify 6 geographic clusters of samples. This clustering was performed due to high sampling density in several areas and in attempts to investigate relationships within and between areas of high sampling density. The data was analyzed on samples with metal concentrations >3σ which were then removed to prevent bias in PCA. Due to large ranges and variations in metal concentrations, the concentration data were normalized by Z-scores using the standard deviation before PCA and CA analysis. The Euclidean distance metric was calculated using standardized metal concentration and plotted following Ward’s method. Ward’s method utilizes the incremental summation of squares when forming clusters (Matlab, 2021). PCA was performed using a singular value decomposition algorithm of standardized metal data to produce principal components that are representative of the data. The loadings of these components describe the variance in a dataset with the first principal component accounting for the most variance and each successive component accounting for lesser amounts of variance. This description of variance by the components may be interpreted as a result of some underlying influence from some initial source. Interpretation of PC load is often difficult, therefore rotation such as Varimax rotation are applied which enhance the variances of loads, following removal of components with eigenvalues <1 (Harrison et al., 1996; Mostert et al., 2010).
3 Results
3.1 Heavy metal concentrations
The concentration of eleven trace metals (As, Ba, Cd, Cr, Cu, Pb, Hg, Ni, Se, Ag, and Zn) are summarized in Table 1 and raw data is given in Supplementary Table S3. In all samples, the Al variations is the lowest by factor 3 (factor = maximum concentration/minimum concentration) and such variations is attributed to variations in granulometric parameters (Supplementary Table S3 and Supplementary Table S4). Relatively large variations are found for Mn, Zn, Hg, Ag, Cr, Pb and Cd by factors of 27, 23, 21, 20, 19, 14 and 10, respectively (Supplementary Table S3). Relatively lower variations are found by factors for Ba (1.8), Se (4.4), Ni (4.3), As (4.6), and with Cu and Fe (7.4 and 7.8). The average concentrations of heavy metals in 49 road dust samples decreased in the order Zn (556 ± 460 ppm)>Ba (346 ± 53 ppm) > Cr (198 ± 132 ppm) > Pb (134 ± 78.8 ppm) > Cu (91.6 ± 39.9 ppm) > Ni (39.1 ± 14.8 ppm) > As (8.0 ± 2.3 ppm) > Se (1.4 ± 0.4 ppm) > Cd (1.09 ± 0.6 ppm) > Ag (0.43 ± 0.39 ppm) > Hg (0.09 ± 0.07 ppm). This is similar to findings in an earlier study on road dust samples in which select metal concentrations was reported in the decreasing order: Pb > Cr, Ni > As > Cd (Howard et al., 2019), except for Cr. The differences in metal concentrations are likely due to the greater number of road dust samples collected in this study (this study n = 49 vs. previous n = 23) and larger study area. The elevated metal concentrations compared to the background levels, which vary due to variations in mineralogy, are related to anthropogenic activities. A large variations in the concentrations of these metals from road dusts have been widely reported, with data distributions being reported as normal, lognormal, nonparametric, or highly variable (Charlesworth et al., 2003, Charlesworth et al., 2011; Wei and Yang, 2010; Apeagyei et al., 2011; Loganathan et al., 2013; Hanfi et al., 2020). In a subset of samples analyzed, the concentrations exceed the general soil screening level (SSL) of the EPA based on target hazard quotient (THQ) = 0.1 tables, with the THQ being target value for the hazard quotient defined as a unitless ratio of exposure concentration to reference concentration where a value greater than unity indicates an individual will likely experience adverse health effects (US EPA, 1998; USEPA, 2020). Arsenic is the only element that exceeds the EPA’s SSL level in all 49 samples, with a relative bioavailability factor of 0.6 (US-EPA, 1996; US EPA, 2007).
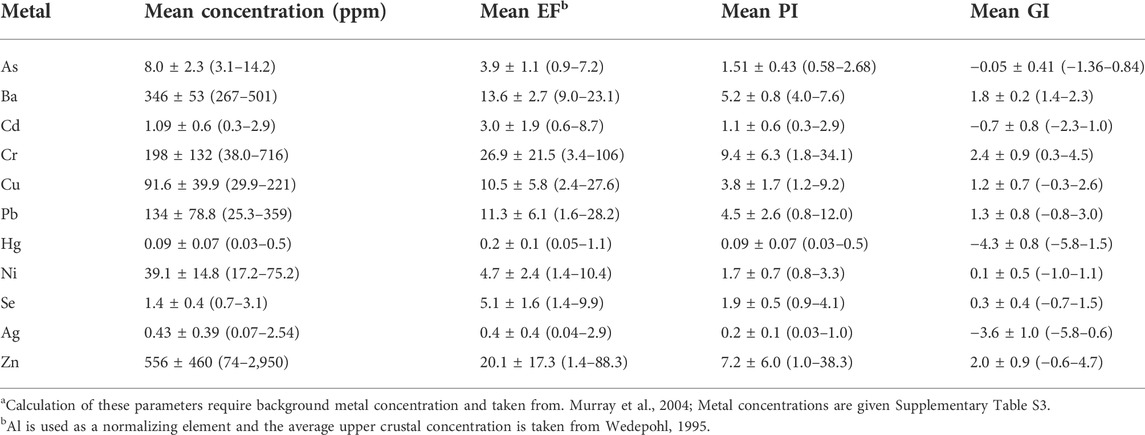
TABLE 1. Summary of concentration (ppm), enrichment factor (EF)*, pollution index (PI)*, and geoaccumulation. Index (GI)* of Heavy Metals (n = 49).
The heavy metals that most commonly reported in road dust is Pb followed closely by Cu, Cr, Zn, and Cd. The average Pb concentration of road dust in this study (134 ± 78.8 ppm) was less than the average values reported in 11 of the 14 studies (range: 31.8–2,583 ppm) conducted globally (Supplementary Table S5; Hopke and Natusch, 1980; Fergusson and Ryan, 1984; Stone and Marsalek, 1996; De Miguel et al., 1997; Tong, 1998; Ferreira-Baptista and De Miguel, 2005; Yongming et al., 2006; Shi et al., 2008; Zhang and Wang, 2009; Lu et al., 2010; Apeagyei et al., 2011; Quiñonez-Plaza et al., 2017; Howard et al., 2019). Studies reporting much higher levels of Pb are >20 years old data which represent studies in road dusts that may have been derived from the use of leaded gasoline. The average concentration of Cu reported in this study is less than the average concentration reported in 8 of the 12 studies compiled. The mean concentration of Zn in road dusts reported here was greater than the reported average concentration in 8 of the 11 studies compiled. Average Cd concentration in road dusts reported in this study is less than 6 of the 8 compiled studies.
Note that the average concentration of Cr reported in this study is higher than the reported average concentration of any of the 14 studies listed. The ranking of Cr contamination in Detroit road dusts in comparison to other urban areas is not definitive, mainly due to the relatively smaller sample size of data set (n = 45) and lack of speciated Cr data needed for risk assessment. The remaining heavy metals of interest for this study are less commonly reported and their discussion is given later. Bulk metal concentrations in road dusts and urban soils are reported in numerous studies and are compared to results in this study in Supplementary Table S5 and Supplementary Table S6 (Spittler and Feder, 1979; Carey et al., 1980; Czarnowska, 1980; Gibson and Farmer, 1986; Lux, 1986; Pfeiffer, 1988; Angelone et al., 1995; Paterson et al., 1996; Wong et al., 1996; De Miguel et al., 1998; Wilcke et al., 1998; Cal-Prieto et al., 2001; Murray et al., 2004; Mielke et al., 2011; Howard et al., 2019).
3.2 Metal pollution indicators
The pollution indicators calculated from the metal concentrations are useful in estimating the relative levels of pollution and anthropogenic impacts. The most commonly used four indices are: 1) enrichment factor (EF); 2) pollution index (PI); 3) Geoaccumulation index (GI); and 4) pollution load index (PLI). Calculation of these indices require local geochemical background metal concentrations and we have used the mean concentrations in subsurface silty clay from easternmost geologic units of Southeast Michigan (Murray et al., 2004).
3.2.1 Pollution index
Pollution index (PI) is useful for basic estimation of a sample’s relative degree of pollution of a single metal and calculated as follows:
where (Ci)s and (Ci)bkg are concentrations of metal i in sample (s) and background (bkg) (e.g., Chen et al., 2015). Commonly, PI values are used in the quantification of other pollution indicators such as Tomlinson’s pollution load index (PLI) (discussed later), the Nemerow integrated pollution index (NIPI), and the integrated pollution index (IPI) (Tomlinson et al., 1980; Nemerow, 1991; Chen et al., 2005). The pollution level is categorized as follows (Chen et al., 2005): PI ≤ 1 is Low, 1 < PI ≤ 3 is Mid-Level, and PI > 3 is High. This study did not adopt a classification system based on PI as it is less commonly utilized for pollution classification index than Geoaccumulation index (Müller, 1969; Wei and Yang, 2010; Loganathan et al., 2013; Chen et al., 2015; Hanfi et al., 2020).
A summary of mean PI values for all bulk samples is reported in Table 1 with PI values of individual samples presented in Supplementary Table S7. Chromium and Zn have the highest PI values, with 38 and 34, respectively. The average values of PI for Cr and Zn are 9.4 ± 6.3 and 7.2 ± 6.0, respectively (Table 1). The lowest range and mean values are found for Hg (range: 0.03–0.5; mean: 0.09 ± 0.07) and Ag (0.03–1.0; 0.20 ± 0.10). The mean PI values of 9 of 11 trace metals are >1, suggesting some elevated metal pollution relative to local background soil. The mean PI values of trace metals in samples decreases in the order Cr > Zn > Ba > Pb > Cu > Se > Ni > As > Cd > Ag > Hg. Note that Ba, Cr, Zn, and Cu have minimum PI ≥ 1, suggesting persistent elevation of these trace metals in Detroit road dusts. Likewise, the minimum PI of Pb and Se are >0.84. For two soil samples, (A05S and A03S) the PI < 1.0 for all metals.
3.2.2 Enrichment factor
Enrichment factor (EF) is a measure of the fraction of the total amount derived from anthropogenic activities. This can be calculated by normalizing metal concentrations to an element that varies minimally in concentration and does not vary due to anthropogenic or natural processes. We choose aluminum as the reference element in this study because it’s concentration remains unaffected by the soil redox processes and biogeochemical cycling (Buat-Menard and Chesselet, 1979; Ravichandran et al., 1995). Furthermore, its concentration does not vary greatly between upper continental crust (UCC Al = 8.04% by weight) and bulk continental crust (BCC Al = 8.41%) (Taylor and McLennan, 1995) while the other commonly used element, Ti, differs from the UCC (Ti = 0.3 wt%) to BCC (Ti = 0.54 wt%) and thus Al is used as a normalization index element. The EF is calculated using the Eq. 2:
where EFi is enrichment factor for metal ‘i’, Ci and Cref are concentrations (ppm) of metal i, and reference element and subscripts s and bkg denote sample and background sample, respectively (Buat-Menard and Chesselet, 1979; Lawson and Winchester, 1979; Sutherland, 2000). If the pre-anthropogenic metal concentration and metal/Al ratios at a given site differ from those of the average upper crust values, then, the site-specific pre-anthropogenic values need to be determined. In such a case, when calculated EF levels are >1.5 (in principle, >1.0), the samples are considered to be enriched. The metal enrichment level categories, based on EF, are as follows (Sutherland (2000)): EF < 2: depleted to minimally enriched; EF 2–5: moderate enrichment; EF 5–20: significant enrichment and EF 20–40: very highly enriched, and EF > 40: extremely enriched. Note that the samples with low EF (i.e., near 1) does not necessarily mean that the metal lacks any anthropogenic influence, but that the metal is not specifically enriched relative to the chosen background values.
The average enrichment factors in all samples suggest high enrichment, EFs as high as 106 for Cr, 88 for Zn, 28 for Cu and Pb, and 23 for Ba (Table 1, Supplementary Table S8). These are some of the highest values reported in global literature. Chromium occurs in two valence states, trivalent (Cr III) and hexavalent (Cr VI), of which Chromium VI is the most toxic. While Cr (III) is an essential element in humans, Cr (VI) targets the respiratory tract organ for toxicity, for acute (short-term) and chronic (long-term) inhalation exposures. Exposure to Cr (VI) via ingestion and inhalation of superfine soil dust that are enriched in Cr (VI) could result in shortness of breath, coughing, and wheezing as well bronchitis, decreased pulmonary function, pneumonia, and other respiratory effects (US. EPA United States Environmental Protection Agency, 1998; USEPA, 1998; Wilbur et al., 2012). For Ag and Hg, the average EF values are less than 1.0, indicating depletion and is likely due to the used background values which may not reflect the actual pre-anthropogenic concentrations. The average EFs decrease in the order: Cr > Zn > Ba > Pb > Cu > Se > Ni > As > Cd > Ag > Hg. The mean EF of Cr and Zn being >20 classify these metals as “very highly enriched” while Ba, Cu, Pb, and Se mean EF values of >5 as “significantly enriched”. The average EFs of soil samples are consistently lower than the averages from road dusts suggesting a greater degree of anthropogenic influence on road dusts.
3.2.3 Geoaccumulation index
Geoaccumulation index (GI) takes in to account the natural fluctuations in background concentrations. GI is calculated as follows:
where (Ci)s and (Ci)bkg are concentrations of metal i in sample (ppm) and background concentration, respectively. The background (BKG) concentration is multiplied by 1.5 to account for natural BKG variations. Categorization of pollution levels in GI index are as follows: practically unpolluted (PUP): GI ≤ 0; PUP to moderately polluted (MP): 0 < GI ≤ 1; MPP: 1 < GI ≤ 2; moderately to heavily polluted: 2 < GI ≤ 3; heavily polluted: 3 < GI ≤ 4; heavily to extremely polluted: 4 < GI ≤ 5; and extremely polluted: GI > 5; (Müller, 1969). A summary of mean GI values for all bulk samples (Table 1) and individual samples (Supplementary Table S9) indicate GI values of heavy metals decreases in the following order: Cr > Zn > Ba > Pb > Cu > Se > Ni > As > Cd > Ag > Hg. The heavily polluted metals are Zn (average: 2.0 and highest: 4.7), Cr (2.4, 4.5), and Pb falls in the moderately polluted category (average: 1.3, 3.0) (Table 1) along with Pb, Cu, and Ba.
3.2.4 Pollution Load Index
Pollution load index (PLI) is used as a metric to assess the overall pollutant loading in sediment, soil, and road dust (Tomlinson et al., 1980; Chen et al., 2015; Gloaguen et al., 2021). Since this indicator considers the level of metal contamination from several metals, it is a better index of the overall pollution status of a sampling site. PLI is calculated as follows:
where PI1, PI2, and PI3 are the PI of heavy metals 1, 2, and 3 respectively, and n is number of trace metals (Tomlinson et al., 1980). The PI values of Hg and Ag are <1.0 and hence were not included in the PLI calculation. Based on PLI, road dust samples can be categorized as follows: unpolluted: PLI ≤1; slightly polluted: 1 < PLI ≤2; moderately polluted: 2 < PLI ≤3; and heavily polluted: PLI ≥3 (Chen et al., 2015; Liu et al., 2016).
A summary of PLI and sample type are reported in Supplementary Table S10 with PLI and location type of individual bulk samples in Supplementary Table S11. The four location types initially identified for this study are residential, industrial, commercial, and soil which were designated based on property tax zoning. The average PLI of sample types decreases in the order: industrial > residential > commercial > and soil. In total, 92% of all samples had a PLI >2 including 100% of commercial and industrial samples.
3.3 Heavy metal concentration and pollution indicators in size-fractionated road dusts
The variation in mean heavy metal concentration within bulk (<63 μm) and size-fractionated samples are reported in Supplementary Table S12 and individual size fractionated samples in Supplementary Table S4. The average concentrations of heavy metals in the bulk and size-fractionated samples decreased in the order Zn > Ba > Cr > Pb > Cu > Ni > As > Se > Cd > Ag > Hg which is similar to the bulk samples. Metal concentration generally increases with decreasing grain-size for As, Cu, Pb, Hg, Ni, Ag, and Zn, although the variations between fractions are all within ±1σ. Cd and Se do not show clear change with grain-size, while Ba and Cr show a general increase with increasing grain-size, although variations remain within ±1σ.
The variations in mean pollution indicators for bulk (<63 μm) and size-fractionated samples are reported in Table 2, with individual size-fractionated samples in Supplementary Tables S13–S16. The mean PLI generally increases with decreasing grain size, with PLI of the <32 μm fraction is similar to that of the bulk (within ± 1σ). The average EF of all metals generally increases with decreasing grain size although the differences between fractions are not significant within ±1σ. The mean GI values for As, Cu, Hg, Ni, Se, and Ag generally increase with decreasing grain size while for other elements generally either there is a decreasing trend or lack of clear change with grain size. Overall, there appear to be no statistically significant variations in heavy metal content or contamination level between the different size fractions.
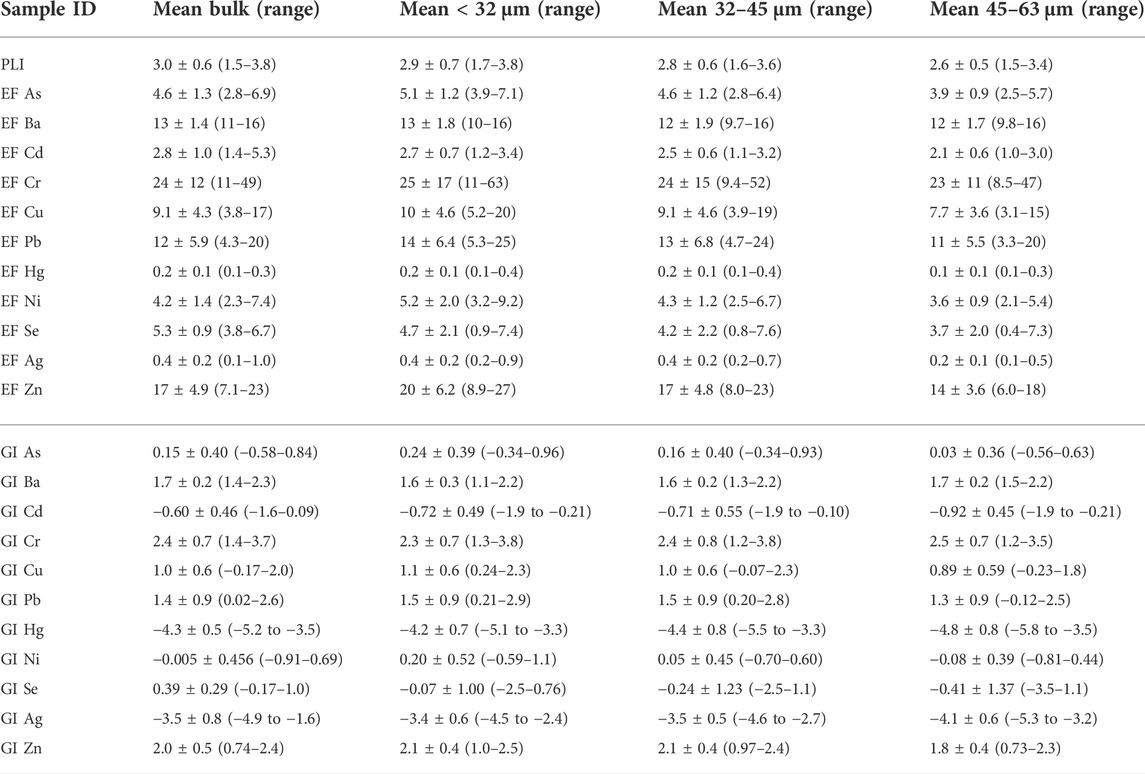
TABLE 2. Comparison of Pollution Load Index (PLI), geoaccumulation index (GI), and enrichment factor (EF) in bulk and size fractions.
3.4 Statistical analyses
Statistical analyses provide additional information allowing a more complete understanding of underlying patterns in heavy metal contamination. The relationships between concentrations of As, Ba, Cd, Cr, Cu, Pb, Hg, Ni, Se, Ag, and Zn and sample locations were investigated using descriptive statistics, k-means geographic clustering, hierarchical clustering, and principal component analysis followed by varimax rotation. Preceding statistical analysis, preliminary analysis revealed four samples (A07, A08, E04, and E09) are statistical outliers with concentration values >3σ of mean metal concentration. The large variations in geochemical data often result in outliers and non-normal distributions which will bias many statistical methods unless outliers are removed (Reimann et al., 2002) and therefore, they were eliminated from multivariate analysis. The remaining samples were then normalized by z-scores prior to analysis.
3.4.1 Geographic and location type categorization
The K-means clustering was performed on location datum (latitude and longitude) to identify geographic clusters of samples (circled clusters Figure 2). This analysis classifies points by their distance to k centroids (k = 6 chosen; Euclidean distance used as metric). Six cluster centers are determined that minimize the average distance between samples to a common center, resulting in sample clusters near each other. Cluster 6 contained only one point (E02) located nearly equidistant from cluster 3 and cluster 5, and thus it is ignored. Clustered samples are interpreted as proximally related, and the relationships within and between these groupings is compared to groups identified by location type (dots Figure 2).
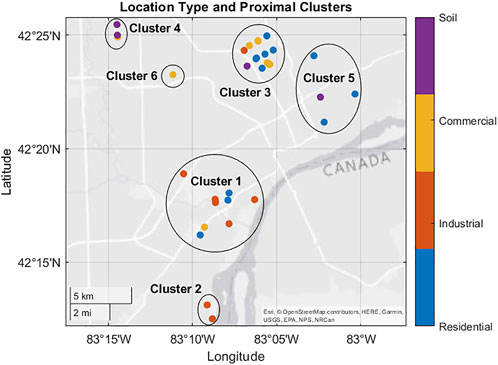
FIGURE 2. Clusters identified by k-means clustering and classified by location type (Identified by tax zoning code).
3.4.2 Correlation analysis
A Pearson’s correlation matrix was constructed using normalized metal data with the correlation coefficients (r coefficients) and p values reported in Table 3. All metals are moderately correlated (r ≥ 0.4) with p ≤ 0.05 with Cd and Zn each being correlated to six other metals.
3.4.3 Student’s t-test
To assess whether proximity or location type produces more distinctive groupings, the average values of metal data categorized by proximal cluster and location type were compared by Student’s two-sample t-test. The T-tests were performed assuming unequal variance with a significance threshold of α = 0.05. For this study, a comparison (e.g., one location type to another or one proximal group to another) is deemed distinct if the null hypothesis that the groups have the same mean may be rejected. This is done as a preliminary step in identifying methods of categorization that may be most useful for further study. The results for proximal clusters are reported in Table 4 and for location type in Supplementary Table S17. Zinc and As in Table 4 are the elements most commonly distinct between clusters with each being distinct in 6 of 10 cluster comparisons. Ba, Se, and Hg are least commonly distinct between proximal clusters with Hg and Se being distinct in 3 of 10 comparisons and Ba being distinct in 2 of 10. Additionally, the Hg in Cluster 5 is distinct from all other clusters besides cluster 2. The most geochemically distinct clusters are 1 and 4 with 9 of 11 elements being distinct. Overall, Cluster 4 is the most distinct from other clusters although is not as distinct from Cluster 2 with only 3 metals being distinct. Cluster 2 is the least geochemically distinct.
Location-type clusters (Supplementary Table S17) show fewer distinct patterns than the proximal clusters. The metals that are most distinct between location types are Ni and Ba which were distinct in 5 of 6 and 4 of 6 comparisons, respectively. As and Cd are notably not distinct in any of the location type comparisons. Strong distinctions exist between industrial locations and soil (7 of 11 elements) and residential locations (6 of 11 elements). In addition, residential and soil samples are the least geochemically distinct with only 2 of 11 elements having statistically significant differences in concentration. Commercial locations were only distinct from other location types in 3 of 11 metals in each comparison, although not the same elements for each.
3.4.4 Hierarchical cluster analysis
To better understand the relationships between trace metals, hierarchical cluster analysis was performed. We applied Ward’s method, which produces clusters by minimizing variance (or maximizing R2) within each successive cluster. Two main clusters are observed, with Cluster 1 containing Cd, Ag, Cr, Zn Ni, and Cu and Cluster 2 containing Pb, As, Hg, and Ba (Figure 3).
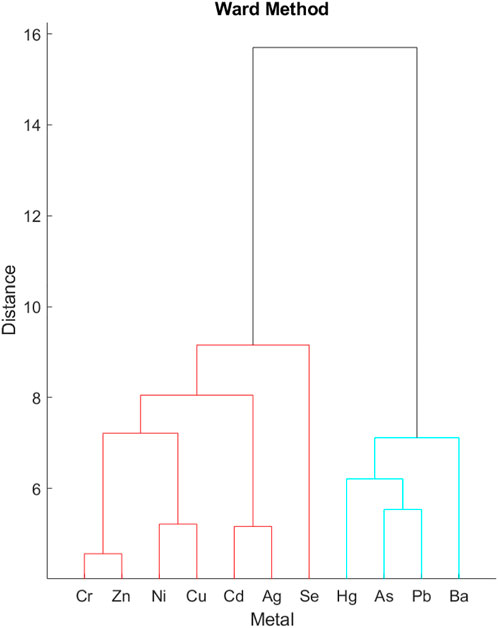
FIGURE 3. Dendrogram of trace metals. Colored groups indicate cluster separation at 70% maximum linkage distance (∼11).
3.4.5 Principal component analysis
Principal component analysis (PCA) was conducted on the normalized metal concentration data. Initial loadings and the variance explained by each component are reported in Supplementary Table S18. The first 3 principal components (PC) explain >70% of the variance in the data, with each individual PC explaining ≥9%. Varimax rotation was applied to aid in interpretation of PCs. The results of varimax rotation are reported in Table 5.
4 Discussion
The quantitative metrics, i.e., Pollution Index, Enrichment Factor, Pollutant Load Index and Geoaccumulation Index, to determine anthropogenic metals pollution critically depend on the pre-anthropogenic concentrations. Most studies have focused on a subset of these metrices in quantifying road dust metal pollution, but we have calculated all four metrics used to assess metal pollution in road dust, soil, and sediment matrices. It is anticipated that the EF, PI, and GI are expected to exhibit same order of decreasing values, based on their calculations given above. The PI values of Hg and Ag are <1.0 for all samples suggesting that there is no Hg contamination above background levels in Detroit road dusts or soils, although, it should be noted that the background value of Hg in subsurface silty clay reported in Murray et al. (2004) is relatively high with an average concentration of 0.98 ppm. Therefore, it is possible that PI values for Hg doesn’t represent actual pollution level. Overall, there is a persistent elevation of measured trace metals in Detroit road dusts.
4.1 Enrichment factor
It is widely known that the sorbed (or bioavailable and anthropogenic) concentration of metals are higher in finer grain-size fraction, due to increased surface per unit mass of soil compared to larger grain size fraction. A comparison of EFs for <32 μm, 32–45 μm and 45–63 μm (Table 3) indicates the enrichment factors generally follow the sequence: 32 μm > 32–45 μm > 45–63 μm. However, the differences in the EF between different size fractions in a sample is <10% which is much smaller than the range of values for all samples (Table 2). It was shown that on < 10 μm road dust samples from California, the range of EF reported values for Cr, Ni, Cu, Zn, and Pb are: 137–605, 1.2–87.6, 4.9–98.9, 6.0–735, and 5.0–18.9, respectively, with such high EF values attributed to very fine particle analyzed (McKenzie et al., 2008). Note that the surface area per unit mass in a 10 μm grain size is about 15 times higher than that of a 39 μm size. Earlier study of sediments from Clinton River in the southeast Michigan watershed located north of the study area reported maximum EF values of 8.7 (Cd), 7.3 (Pb), 3.4 (Zn), 2.6 (Cu), 1.6 (Ni), and <1 (As) using background concentrations of the local glacial lobe (van Hees et al., 2010). Another study in Baoji, China reported average EF values in road dust of 3.51 (Cu), 9.03 (Pb), 6.20 (Zn), and 1.20 (Ni) using background values in Chinese soil and Fe as a reference element (Lu et al., 2009). A study from Dhanbad and Bokaro regions of India reported EF in road dust ranging from 0.58–0.94 (Cu), 1.76–3.29 (Zn), 0.41–0.8 (Ni), 0.51–1.22 (Cr), and 9.1–36.6 (Pb) using average crustal composition and Sr as a reference element (Singh, 2011). Residential and industrial areas in Singapore reported median EF in street dusts of 5.4–7.2 (Cr), 2.3–7.2 (Ni), 30–46 (Cu), 19–25 (Pb), and 34–38 (Zn) using upper crustal average values and Al as a reference element (Yuen et al., 2012). Overall, this study reports relatively higher enrichment factors for several metals.
4.2 Geoaccumulation index
The calculated GI values for <32 μm fraction is slightly higher than that for bulk (<63 μm) fraction for As, Cu, Pb, Ni, and Zn (Table 2) and the values in <32 μm fraction is higher than that in 45–63 μm fraction (Table 3). The only metals observed with minimum GI values of >1.0 are Ba, Cr, Pb, and Zn, indicating moderate pollution in all Detroit road dusts. Two samples are classified as heavily polluted with GI of 4.7 (A08) and 4.5 for Cr (B07). These values can be compared to the values reported from other global cities: average GI of 0.18 (Cr), 1.48 (Cu), 1.81 (Pb), 1.48 (Zn), 0.34 (Ni), and 2.64 (Cd) in road dusts of several cities in China (Wei and Yang, 2010); In Dhanbad and Bokaro regions (India) Singh. (2011) reported GI values ranging from 1–3 (Pb) and <0 for all other metals (i.e., Cu, Zn, Ni, and Cr) (Singh, 2011). In New York, GI values of 0.45 (Cr), 3.39 (Cu), 6.05 (Pb), 3.59 (Zn), -4.41 (Ni), and 5.74 (Cd) in road dusts were reported in mid-1980s (Fergusson and Ryan, 1984). In Sault Ste Marie, Canada average GI values of road dusts were reported to be 0.01 (Cr), 1.36 (Cu), 1.21(Pb), 0.60 (Zn), −0.61 (Ni), and 2.5(Cd) (Stone and Marsalek, 1996). Road dusts of Luanda, Angola, Ferreira-Baptista and De Miguel (2005 reported GI values of −1.82 (Cr), 0.31 (Cu), 3.01 (Pb), 1.08 (Zn), −2.01 (Ni), and 2.87 (Cd) in road dusts (Ferreira-Baptista and De Miguel, 2005). In summary, some of GI values for Detroit is higher than those in many of these cities.
4.3 Pollution load index
The average Pollution Load index (PLI) in road dusts in this study are classified as “heavily polluted” according to the classification of Liu et al. (2016), with the majority of road dust samples classified as “highly polluted” (51% of dust samples; n = 45), suggesting ubiquitous high metal contamination in road dusts. No samples had PLI ≤1 suggesting that all road dusts and urban soils in Detroit are at minimum classified as “slightly polluted” (Liu et al., 2016). Direct comparison of PLI values from different regions of the world is difficult due to differences in geochemical background, land use, industrial history, population, number of vehicles driven per unit population, etc., between cities as well as differences in the metals used for PLI calculation. Earlier studies of road dusts reported average PLI values of 2.0–2.6 (averages of different areas in Shanghai, China), 1.5–6.5 (Loudi, China), 14.6 (residential area, Singapore) 20.6 (industrial area, Singapore), and 1.51 (Wuhan, China) (Yang et al., 2010; Yuen et al., 2012; Zhang et al., 2012; Wang et al., 2019). Previous studies also reported PLI values in urban soils of 2.38 (Kaifeng City, China), 1.1 (range = 0.2–13.4; various soils in China), 3.64 (Subaé River, Brazil), and 2.3–9.2 (Detroit, United States) (Chen et al., 2015; Liu et al., 2016; Howard et al., 2019; Gloaguen et al., 2021). Further risk and/or hazard-based research into Detroit road dusts is required to assess potential negative public health outcomes and/or negative ecological effects.
4.4 Student’s T-test
We performed T-tests to determine whether mean metal concentrations are more statistically distinct in clusters defined by geographic clustering or by land use zoning. A higher degree of distinction between the proximity clusters supports the hypothesis that variations in those elements is influenced by proximity to common sources. Additionally, we looked for individual elements or sets of elements that are distinct between clusters, which may support the possibility of local sources in or nearby a cluster. For example, if the Hg concentration in a cluster is distinctly elevated in a proximal cluster compared to other proximal clusters, it would support the hypothesis of a source of Hg contamination nearby that cluster. Conversely, under the assumption that tax zoning serves as a proxy for types of land use (e.g., industry, commercial business, or residence), greater distinction of elements between location types would support a hypothesis that variations are influenced by how that area is used. We interpret the results in Table 4 and Supplementary Table S17 with these assumptions.
Overall, we find mean metal concentrations at clusters defined by proximity are more often distinct at a significant level than those defined by land use type. In Table 4 the distinct means of Zn and As in 6 of 10 comparisons potentially suggests variations in these metals are partially controlled by proximal sources. In contrast, the relative similarity in averages of Ba, Se, and Hg (distinct in 2-3 of 10 comparisons) suggests that these metals may have a source not specific to one location. Notably, Hg in proximal cluster 5 is distinct from all other clusters except cluster 2, which has a small sample size (n = 2). This suggests a possible local source of Hg proximal to cluster 5. Note that cluster 4 also contains two soil samples which have low concentrations, thus some of the distinctions with this cluster may be somewhat biased if proximity is the only criteria for categorization.
The relatively fewer distinct patterns between location types in Supplementary Table S17 than proximal clusters potentially suggests that proximally related sources may control geochemical distinctions in contamination than location type. Although, the difference in number of groups compared in two datasets and relative number of samples in the datasets may bias direct comparisons between the datasets. The distinction of Ni and Ba in 4-5 of 6 comparisons suggests that differences in their contamination may be related to use characteristics such as traffic density or industrial activity. Control of Ba contamination by use characteristics is supported further from a lack of distinction between proximal clusters (i.e., only distinct in 2 of 10 comparisons). Absence of distinction between groups for As and Cd suggests these metals may not be controlled by use characteristics. In conjunction with observations in Table 4 where As and Cd are distinct in 6 of 10 and 5 of 10 clusters, respectively, variations of these metals may be controlled by location and/or local sources. The distinction of 6-7 of 11 elements between both residential and soil suggests industrial locations may be geochemically distinctive from soil or residential samples. Conversely, the distinction of 2 of 11 elements between residential and soil suggests they are relatively similar.
4.5 Hierarchical cluster analysis
The strong clustering of Hg, Pb, As, and Ba in Cluster 1 suggests a common source between these metals, with strong connection between As and Pb. A strong clustering of Cd, Ag, Cr, Zn, Ni, and Cu in Cluster 2 possibly indicate a common source for these metals with a relatively strong connection between Cr and Zn. The linkage between Cd and Ag at a higher level in Cluster 1 indicates a weaker association with the possible common source or a mixing of contributions from multiple sources. The high-level linkage of Se with the Cluster 1 indicates a weak association of Se with other elements in the cluster.
4.6 Principal component analysis
Following varimax rotation, the first principal component is dominated by Cd, Cr, Ni, Ag, and Cu, each with loadings ≥0.4, along with a lesser loading of Zn (Table 5). These metals were linked in cluster analysis further suggesting a common source (more details given in section 4.7). PC 2 is dominated by Pb, As, and Hg with a lesser loading from Ba. These metals were also linked in Cluster 1, which supports the suggestion of a common source. Finally, PC 3 is dominated almost solely by Se and a strong negative loading of Ba, with a minor loading of Zn. The negative loading of Ba may be interpreted as a negative correlation of Ba with the overall direction of this load.
4.7 Statistical analysis summary and interpretations
The application of multiple statistical methods allows for a more complete understanding of heavy metal distribution and possible sources. Previous studies have applied different methods of pollution source characterization and apportionment such as analysis of specific sources (brake pads, tire wear, exhaust, etc.), different pollution sources (smelters, coal fired power plants, e-waste recycling, etc.), and specific grain type analysis (slag glass, road paint, etc.) (Adachi and Tainosho, 2004; Thorpe and Harrison, 2008; Sepúlveda et al., 2010; Charlesworth et al., 2011; Zhang et al., 2011; Loganathan et al., 2013). Adachi and Tainosho (2004) characterized tire embedded particles based on composition and morphology resulting in the following groups: brake dust (Cu and Ba), yellow paint (Cr and Pb), “mixed” (Cr, Cu, Zn, Ba, and Pb), and tires (Zn). Another study using a combination of EF and multivariate statistics identified sources as Commercial/Domestic (Ag and Hg), Industrial/traffic (Cr, Cu, Pb, and Zn), and Soil (As) (Yongming et al., 2006). In an extensive literature review, Thorpe and Harrison (2008) summarized concentrations of different non-exhaust particle sources including brake wear, tires, road surface abrasion, and re-suspension of road dusts and concluded that there are no suitable elemental tracers that specifically discriminate non-exhaust particle contributions, other than the use of Cu:Sb ratios for brake dust. The study reported that Ba was commonly reported in road dusts and attributed part of its abundance to BaSO4 fillers used in brake linings. Barium-containing compounds are also extensively used as dispersants in various vehicular fluids (Thorpe and Harrison, 2008; Charlesworth et al., 2011).
Three contamination profiles identified in this study are classified based on the rough chemical classifications of the constituent elements, and represent the three general patterns that describe overall metal contamination in Detroit road dusts/soils. Group 1 is labelled “transition metal rich” and coincides with the profile identified in PC 1 and cluster 1 which contains Cr, Cd, Ni, Ag, Zn and Cu. Group 2 is labelled “post-transition and metalloid rich” and coincides with PC 2 and cluster 2 which contains Pb, As, Hg, and minor Ba. Group 3 is labelled “non-metal rich” and coincides with PC 3 and is partially indicated by the high-level linkage of Se in cluster analysis. Previous studies have identified several of the metals studied here as “natural” or geogenic elements (As, Cr), although the EF values of road dusts in this study indicate these metals are not enriched via natural processes (Manta et al., 2002; van Hees et al., 2010). Results from the hypothesis testing of location types and clusters both suggest certain metals such as Cr are distinctively elevated in industrial areas and proximal cluster 1 which is itself a heavily industrialized area. Several elements elevated in industrial areas (e.g., Cr, Zn, and Ni) coincide with those in the transition metal rich group suggesting that this group may represent the signature of contamination from industrial sources. Although, the results of location type hypothesis testing suggest that it is Ni and Ba that show greater distinction between location types which somewhat contradict this connection. Overall, the relationship between the transition metal rich group to industrial activity requires further investigation to test the validity of such association.
5 Conclusion
This study represents a multifaceted approach on the characterization of road dusts in the Detroit area with datasets consisting of trace metal data analyzed using pollution indicators, and univariate and multivariate statistical analysis. From the collection and analysis of a suite of bulk (<63 μm) and a sub-set of size-fractionated road dust and soil samples for a suite of heavy metals (Pb, Cr, Cd, As, Ag, Hg, Se, Ba, Ni, Zn, and Cu), from a post-industrial city in southeast Michigan, the following conclusions are drawn:
i) We report the highest concentration of Cr in Detroit compared to any other major global city, with average concentration of 198 ppm (range: 38–716), and mean pollutant metrics of: EF 27 (3–106), PI 9.4 (1.8–34), and GI 2.4 (0.3–4.5). While this study didn’t address the speciation of Cr, inhalation and ingestion of finer soil-laden Cr (VI) could cause major health problems;
ii) The mean enrichment factor for As, Cd, Cu, Ni and Pb are 3.9, 3.0, 10.5, 4.7, and 11.3, respectively; the corresponding PI values are 1.5, 1.1, 3.8, 1.7, and 4.5 respectively, showing highly polluted nature of road dust;
iii) Zinc and Ba have the highest enrichment factors after Cr, with values of 20 and 14, respectively and the corresponding PI values of 7.2 and 5.2, respectively, suggesting all road dusts and soils in Detroit are significantly enriched in Zn and Ba due to anthropogenic influences;
iv) The mean Pb concentration of 134 ppm is about 10 times higher than the crustal average concentration and thus lead pollution is prevalent;
v) The Geoaccumulation Index values of all road dusts are higher for Ba, Cr, Cu, Pb, and Zn, indicating high pollution. The Pollution Load Index values of road dusts and soils in Detroit indicate pollution levels of As, Ba, Cr, Cu, Pb, Ni, Se, and Zn are high suggesting all samples are at least slightly polluted with >50% of road dust values of ≥3 classifying them as “heavily polluted”. Average PLI is higher than reported in some other global cities such as Shanghai, although direct comparison is difficult due to a variety of factors;
vi) There are significant linear correlations between trace metals, with the stronger correlations being between the pairs Cr-Zn (r = 0.76, p < 0.01) and Cd-Ag (r = 0.70, p < 0.01) potentially suggesting a common source for these metal pairs;
vii) Concentrations of Cd, As, Pb, and Zn in road dust are potentially more distinct between different proximally related clusters, while Ni and Ba are between property type clusters. In general, metal concentrations of industrial samples are more commonly distinct for about half of metals (≥6 of 11) differing from residential and/or soil samples;
viii) Three generalized contamination profiles or “groups” were identified by the combination of hierarchical cluster and PCA analyses followed by varimax rotation. These groups are:
• Group 1 (“transition metal rich”): characterized by Cr, Cd, Ni, Ag, Zn, and Cu;
• Group 2 (“post-transition and metalloid rich”): characterized by Pb, As, Hg, and minor Ba; and
• Group 3 (“non-metal rich”): characterized by Se.
This study lays a foundation for tracking toxic trace metal clusters in road dust in Detroit and future study should focus on areas where children are likely to get higher levels of toxic metals exposure, which include children’s playground, preschool and other highly used public places. Given the potential distinction of Cd, As, Pb, and Zn being influenced by proximal sources, the areas with greatest contamination may warrant investigation into potential point sources in the study area. Additionally, the elevated concentration of Hg in proximal cluster 5 requires further investigation into possible nearby Hg sources.
Data availability statement
The original contributions presented in the study are included in the article/Supplementary Material, further inquiries can be directed to the corresponding author.
Author contributions
The idea of this research was conceived by MB and MD. The field sampling was conducted jointly by MD, MB, CT and TD. All the laboratory work was conducted by MD as part of his master’s thesis. Statistical analyses on the data were performed by SB and MD. All authors have contributed by reviewing multiple versions of the manuscript.
Conflict of interest
The authors declare that the research was conducted in the absence of any commercial or financial relationships that could be construed as a potential conflict of interest.
Publisher’s note
All claims expressed in this article are solely those of the authors and do not necessarily represent those of their affiliated organizations, or those of the publisher, the editors and the reviewers. Any product that may be evaluated in this article, or claim that may be made by its manufacturer, is not guaranteed or endorsed by the publisher.
Supplementary material
The Supplementary Material for this article can be found online at: https://www.frontiersin.org/articles/10.3389/fenvs.2022.974237/full#supplementary-material
References
Activation Laboratories Ltd (2020a). 1G - aqua regia - Hg cold Vapour FIMS. Actlabs.comAvailable at: https://actlabs.com/geochemistry/exploration-geochemistry/mercury/.
Activation Laboratories Ltd (2020b). Ultratrace 6 - near total digestion - ICP and ICP-ms. Actlabs.comAvailable at: https://actlabs.com/geochemistry/exploration-geochemistry/4-acid-near-total-digestion/.
Adachi, K., and Tainosho, Y. (2004). Characterization of heavy metal particles embedded in tire dust. Environ. Int. 30, 1009–1017. doi:10.1016/j.envint.2004.04.004
Angelone, M., Corrado, T., and Dowgiallo, G. (1995). Lead and cadmium distribution in urban soil and plants in the city of Rome: a preliminary study.” in Proceedings of the Third International Conference on the Biogeochemistry ofTrace Elements, 23–24. France: INRA Institut National de la Recherche Agronomique, Versailles France.
Apeagyei, E., Bank, M. S., and Spengler, J. D. (2011). Distribution of heavy metals in road dust along an urban-rural gradient in Massachusetts. Atmos. Environ. X. 45, 2310–2323. doi:10.1016/j.atmosenv.2010.11.015
Banerjee, A. D. K. (2003). Heavy metal levels and solid phase speciation in street dusts of Delhi, India. Environ. Pollut. 123, 95–105. doi:10.1016/S0269-7491(02)00337-8
Buat-Menard, P., and Chesselet, R. (1979). Variable influence of the atmospheric flux on the trace metal chemistry of oceanic suspended matter. Earth Planet. Sci. Lett. 42, 399–411. doi:10.1016/0012-821X(79)90049-9
Cal-Prieto, M. J., Carlosena, A., Andrade, J. M., Martínez, M. L., Muniategui, S., López-Mahía, P., et al. (2001). Antimony as a tracer of the anthropogenic influence on soils and estuarine sediments. Water. Air. Soil Pollut. 129, 333–348. doi:10.1023/A:1010360518054
Carey, A. E., Gowen, J. A., Forehand, T. J., Tai, H., and Wiersma, G. B. (1980). Heavy metal concentrations in soils of five United States cities, 1972 urban soils monitoring program. Pestic. Monit. J. 13, 150.
Charlesworth, S., de Miguel, E., and Ordóñez, A. (2011). A review of the distribution of particulate trace elements in urban terrestrial environments and its application to considerations of risk. Environ. Geochem. Health 33, 103–123. doi:10.1007/s10653-010-9325-7
Charlesworth, S., Everett, M., McCarthy, R., Ordóñez, A., and de Miguel, E. (2003). A comparative study of heavy metal concentration and distribution in deposited street dusts in a large and a small urban area: Birmingham and coventry, west midlands, UK. Environ. Int. 29, 563–573. doi:10.1016/S0160-4120(03)00015-1
Chen, H., Teng, Y., Lu, S., Wang, Y., and Wang, J. (2015). Contamination features and health risk of soil heavy metals in China. Sci. Total Environ. 512–513, 143–153. doi:10.1016/j.scitotenv.2015.01.025
Chen, T. Bin, Zheng, Y. M., Lei, M., Huang, Z. C., Wu, H. T., Chen, H., et al. (2005). Assessment of heavy metal pollution in surface soils of urban parks in Beijing, China. Chemosphere 60, 542–551. doi:10.1016/j.chemosphere.2004.12.072
Czarnowska, K. (1980). Akumulacja metali ciężkich w glebach, roślinach i niektórych zwierzętach na terenie Warszawy. Rocz. Glebozn. 31, 77.
De Miguel, E., Jiménez De Grado, M., Llamas, J. F., Martín-Dorado, A., and Mazadiego, L. F. (1998). The overlooked contribution of compost application to the trace element load in the urban soil of Madrid (Spain). Sci. Total Environ. 215, 113–122. doi:10.1016/S0048-9697(98)00112-0
De Miguel, E., Llamas, J. F., Chacón, E., Berg, T., Larssen, S., Røyset, O., et al. (1997). Origin and patterns of distribution of trace elements in street dust: Unleaded petrol and urban lead. Atmos. Environ. X. 31, 2733–2740. doi:10.1016/S1352-2310(97)00101-5
Denier van der Gon, H. A. C., Gerlofs-Nijland, M. E., Gehrig, R., Gustafsson, M., Janssen, N., Harrison, R. M., et al. (2013). The PolicyRelevance of WearEmissions fromRoad transport, nowand in the future-an InternationalWorkshop report and consensus statement. J. Air Waste Manag. Assoc. 63, 136–149. doi:10.1080/10962247.2012.741055
Fakayode, S. O., and Olu-Owolabi, B. I. (2003). Heavy metal contamination of roadside topsoil in Osogbo, Nigeria: Its relationship to traffic density and proximity to highways. Environ. Geol. 44, 150–157. doi:10.1007/s00254-002-0739-0
Fergusson, J., and Ryan, D. (1984). The elemental composition of street dust from large and small urban areas related to city type, source and particle size. Sci. Total Environ. 34, 101–116. doi:10.1016/0048-9697(84)90044-5
Ferreira-Baptista, L., and De Miguel, E. (2005). Geochemistry and risk assessment of street dust in Luanda, Angola: A tropical urban environment. Atmos. Environ. X. 39, 4501–4512. doi:10.1016/j.atmosenv.2005.03.026
Gibson, M. J., and Farmer, J. G. (1986). Multi-step sequential chemical extraction of heavy metals from urban soils. Environ. Pollut. Ser. B Chem. Phys. 11, 117–135. doi:10.1016/0143-148X(86)90039-X
Gloaguen, T. V., Motta, P. N. S. D., and Couto, C. F. (2021). A grain-size correction for metal pollution indexes in river sediments. Int. J. Sediment Res. 36, 362–372. doi:10.1016/j.ijsrc.2020.10.005
Hanfi, M. Y., Mostafa, M. Y. A., and Zhukovsky, M. V. (2020). Heavy metal contamination in urban surface sediments: Sources, distribution, contamination control, and remediation. Environ. Monit. Assess. 192, 32. doi:10.1007/s10661-019-7947-5
Harrison, R. M., Smith, D. I. T., and Luhana, L. (1996). Source apportionment of atmospheric polycyclic aromatic hydrocarbons collected from an urban location in Birmingham, U.K. Environ. Sci. Technol. 30, 825–832. doi:10.1021/es950252d
Hopke, P. K., and Natusch, D. F. S. (1980). Multielemental characterization of urban roadway dust. Environ. Sci. Technol. 14, 164–172. doi:10.1021/es60162a006
Howard, J., Weyhrauch, J., Loriaux, G., Schultz, B., and Baskaran, M. (2019). Contributions of artifactual materials to the toxicity of anthropogenic soils and street dusts in a highly urbanized terrain. Environ. Pollut. 255, 113350. doi:10.1016/j.envpol.2019.113350
Laidlaw, M. A. S., Zahran, S., Mielke, H. W., Taylor, M. P., and Filippelli, G. M. (2012). Re-suspension of lead contaminated urban soil as a dominant source of atmospheric lead in Birmingham, Chicago, Detroit and Pittsburgh, USA. Atmos. Environ. X. 49, 302–310. doi:10.1016/j.atmosenv.2011.11.030
Lawson, D. R., and Winchester, J. W. (1979). A standard crustal aerosol as a reference for elemental enrichment factors. Atmos. Environ. X. 13, 925–930. doi:10.1016/0004-6981(79)90003-9
Lee, C. S. L., Li, X., Shi, W., Cheung, S. C. N., and Thornton, I. (2006). Metal contamination in urban, suburban, and country park soils of Hong Kong: A study based on gis and multivariate statistics. Sci. Total Environ. 356, 45–61. doi:10.1016/j.scitotenv.2005.03.024
Li, H., Qian, X., Hu, W., Wang, Y., and Gao, H. (2013). Chemical speciation and human health risk of trace metals in urban street dusts from a metropolitan city, Nanjing, SE China. Sci. Total Environ. 456–457, 212–221. doi:10.1016/j.scitotenv.2013.03.094
Lim, S. S., Vos, T., Flaxman, A. D., Danaei, G., Shibuya, K., Adair-Rohani, H., et al. (2012). A comparative risk assessment of burden of disease and injury attributable to 67 risk factors and risk factor clusters in 21 regions, 1990-2010: A systematic analysis for the global burden of disease study 2010. Lancet 380, 2224–2260. doi:10.1016/S0140-6736(12)61766-8
Liu, D., Ma, J., Sun, Y., and Li, Y. (2016). Spatial distribution of soil magnetic susceptibility and correlation with heavy metal pollution in Kaifeng City, China. Catena 139, 53–60. doi:10.1016/j.catena.2015.11.004
Loganathan, P., Vigneswaran, S., and Kandasamy, J. (2013). Road-deposited sediment pollutants: A critical review of their characteristics, source apportionment, and management. Crit. Rev. Environ. Sci. Technol. 43, 1315–1348. doi:10.1080/10643389.2011.644222
Lu, X., Wang, L., Lei, K., Huang, J., and Zhai, Y. (2009). Contamination assessment of copper, lead, zinc, manganese and nickel in street dust of Baoji, NW China. J. Hazard. Mat. 161, 1058–1062. doi:10.1016/j.jhazmat.2008.04.052
Lu, X., Wang, L., Li, L. Y., Lei, K., Huang, L., and Kang, D. (2010). Multivariate statistical analysis of heavy metals in street dust of Baoji, NW China. J. Hazard. Mat. 173, 744–749. doi:10.1016/j.jhazmat.2009.09.001
Lux, W. (1986). Schwermetallgehalte und-isoplethen in Böden, subhydrischen Ablagerungen und Pflanzen im Südosten Hamburgs: Beurteilung eines Immissionsgebietes. Hamburg: Verein zur Förderung d. Bodenkunde.
Manta, D. S., Angelone, M., Bellanca, A., Neri, R., and Sprovieri, M. (2002). Heavy metals in urban soils: a case study from the city of palermo (sicily), Italy. Sci. Total Environ. 300, 229–243. doi:10.1016/S0048-9697(02)00273-5
Matlab, (2021). Statistics and machine learning Toolbox user’s guide, 9954. Available at: https://se.mathworks.com/help/stats/index.html.
McKenzie, E. R., Wong, C. M., Green, P. G., Kayhanian, M., and Young, T. M. (2008). Size dependent elemental composition of road-associated particles. Sci. Total Environ. 398, 145–153. doi:10.1016/j.scitotenv.2008.02.052
Mielke, H. W., Laidlaw, M. A. S., and Gonzales, C. R. (2011). Estimation of leaded (Pb) gasoline’s continuing material and health impacts on 90 US urbanized areas. Environ. Int. 37, 248–257. doi:10.1016/j.envint.2010.08.006
Mielke, H. W., and Reagan, P. L. (1998). Soil is an important pathway of human lead exposure. Environ. Health Perspect. 106, 217–229. doi:10.2307/3433922
Mostert, M. M. R., Ayoko, G. A., and Kokot, S. (2010). Application of chemometrics to analysis of soil pollutants. TrAC Trends Anal. Chem. 29, 430–445. doi:10.1016/j.trac.2010.02.009
Murray, K. S., Rogers, D. T., and Kaufman, M. M. (2004). Heavy metals in an urban watershed in southeastern Michigan. J. Environ. Qual. 33, 163–172. doi:10.2134/jeq2004.1630
Nemerow N. L. (Editor) (1991). Stream, lake, estuary, and ocean pollution. 2nd edition (United States: New York, NY (United States): Van Nostrand Reinhold Publishing Co). Available at: https://www.osti.gov/biblio/7030475.
Nordberg, G. F., Fowler, B. A., Nordberg, M., and Friberg, L. (2007). Handbook on the Toxicology of metals. Elsevier. doi:10.1016/B978-0-12-369413-3.X5052-6
Paterson, E., Sanka, M., and Clark, L. (1996). “Urban soils as pollutant sinks - a case study from Aberdeen, Scotland,” in Applied geochemistry (Elsevier), 129–131. doi:10.1016/0883-2927(95)00081-X
Pfeiffer, E.-M., (1988). Trace elements and heavy metals in soils and plants of the Southeast Asian metropolis Metro Manila and of some rice cultivation provinces in Luzon, Philippines. Verein zur Förderung der Bodenkunde in Hamburg.
Quiñonez-Plaza, A., Wakida, F. T., Temores-Peña, J., Rodriguez-Mendivil, D. D., Garcia-Flores, E., Pastrana-Corral, M. A., et al. (2017). Total petroleum hydrocarbons and heavy metals in road-deposited sediments in Tijuana, Mexico. J. Soils Sediments 17, 2873–2886. doi:10.1007/s11368-017-1778-1
Ravichandran, M., Baskaran, M., Santschi, P. H., and Bianchi, T. S. (1995). History of trace metal pollution in sabine-neches estuary, beaumont Texas. Environ. Sci. Technol. 29, 1495–1503. doi:10.1021/es00006a010
Reimann, C., Filzmoser, P., and Garrett, R. G. (2002). Factor analysis applied to regional geochemical data: Problems and possibilities. Appl. Geochem. 17, 185–206. doi:10.1016/s0883-2927(01)00066-x
Sepúlveda, A., Schluep, M., Renaud, F. G., Streicher, M., Kuehr, R., Hagelüken, C., et al. (2010). A review of the environmental fate and effects of hazardous substances released from electrical and electronic equipments during recycling: examples from China and India. Environ. Impact Assess. Rev. 30, 28–41. doi:10.1016/j.eiar.2009.04.001
Shi, G., Chen, Z., Xu, S., Zhang, J., Wang, L., Bi, C., et al. (2008). Potentially toxic metal contamination of urban soils and roadside dust in Shanghai, China. Environ. Pollut. 156, 251–260. doi:10.1016/j.envpol.2008.02.027
Singh, A. K. (2011). Elemental chemistry and geochemical partitioning of heavy metals in road dust from Dhanbad and Bokaro regions, India. Environ. Earth Sci. 62, 1447–1459. doi:10.1007/s12665-010-0630-3
Sorenson, J. R. (2013). Potential reductions of street solids and phosphorus in urban watersheds from street cleaning. Cambridge, MassachusettsReston: U.S. Geological Survey, 2009–2011. doi:10.3133/sir20125292
Souto-Oliveira, C. E., Babinski, M., Araújo, D. F., Weiss, D. J., and Ruiz, I. R. (2019). Multi-isotope approach of Pb, Cu and Zn in urban aerosols and anthropogenic sources improves tracing of the atmospheric pollutant sources in megacities. Atmos. Environ. X. 198, 427–437. doi:10.1016/j.atmosenv.2018.11.007
Spittler, T. M., and Feder, W. A. (1979). A study of soil contamination and plant lead uptake in Boston urban gardens. Commun. Soil Sci. Plant Anal. 10, 1195–1210. doi:10.1080/00103627909366973
Stone, M., and Marsalek, J. (1996). Trace metal composition and speciation in street sediment: Sault Ste. Marie, Canada. Water Air Soil Pollut. 87, 149–169. doi:10.1007/BF00696834
Sutherland, R. A. (2000). Bed sediment-associated trace metals in an urban stream, Oahu, Hawaii. Environ. Geol. 39, 611–627. doi:10.1007/s002540050473
Taylor, S. R., and McLennan, S. M. (1995). The geochemical evolution of the continental crust. Rev. Geophys. 33, 241–265. doi:10.1029/95rg00262
Tchounwou, P. B., Yedjou, C. G., Patlolla, A. K., and Sutton, D. J. (2012). Molecular, clinical and environmental toxicicology volume 3: Environmental Toxicology. Exp. Suppl. 101, 133–164. doi:10.1007/978-3-7643-8340-4_6
Thorpe, A., and Harrison, R. M. (2008). Sources and properties of non-exhaust particulate matter from road traffic: A review. Sci. Total Environ. 400, 270–282. doi:10.1016/j.scitotenv.2008.06.007
Tomlinson, D. L., Wilson, J. G., Harris, C. R., and Jeffrey, D. W. (1980). Problems in the assessment of heavy-metal levels in estuaries and the formation of a pollution index. Helgol. Meeresunters. 33, 566–575. doi:10.1007/BF02414780
Tong, S. T. Y. (1998). Indoor and outdoor household dust contamination in Cincinnati, Ohio, USA. Environ. Geochem. Health 20, 123–133. doi:10.1023/A:1006561832381
US EPA (1998). Ecological risk assessment guidance for superfund: Process for designing and conducting risk assessments.
US EPA (2007). Guidance for evaluating the oral bioavailability of metals in soils for use in human health risk assessment. Available at: https://semspub.epa.gov/work/03/2218794.pdf.
US-EPA (1996). Soil screening guidance : User ’s guide. Environ. Prot. Agency. Available at: https://rais.ornl.gov/documents/SSG_nonrad_user.pdf.
USEPA (1975). Fugitive emissions and fugitive dust emissions. U.S. Environmental Protection Agency, Office of Air and Water Management, Office of Air Quality Planning and Standards, Control Programs Development Division.
USEPA (1998). IRIS toxicological review of Chromium VI (1998 final). Washington, USEPA 1998: U.S. Environmental Protection Agency.
USEPA (2020). Regional screening level (RSL) summary table 2020 november corrected (TR=1E-06, THQ=1.0). 11. Available at: https://semspub.epa.gov/src/document/HQ/400431.
US. EPA United States Environmental Protection Agency (1998). Toxicological review of trivalent Chromium.” in Toxicol. Rev. Trivalent Chromium (CAS No. 16065-83-1) - support summ. Inf. Integr. Risk inf. Syst. Available at: https://iris.epa.gov/static/pdfs/0028tr.pdf.
van Hees, E. H. P., Chopin, E. I. B., Sebastian, T. M., Washington, G. D., Germer, L. A. M., Domanski, P., et al. (2010). Distribution, sources, and behavior of trace elements in the Clinton River Watershed, Michigan. J. Gt. Lakes. Res. 36, 606–617. doi:10.1016/j.jglr.2010.08.007
Wang, G., Chen, J., Zhang, W., Chen, Y., Ren, F., Fang, A., et al. (2019). Relationship between magnetic properties and heavy metal contamination of street dust samples from Shanghai, China. Environ. Sci. Pollut. Res. 26, 8958–8970. doi:10.1007/s11356-019-04338-4
Wedepohl, H. K. (1995). The composition of the continental crust. Geochim. Cosmochim. Acta 59, 1217–1232. doi:10.1016/0016-7037(95)00038-2
Wei, B., and Yang, L. (2010). A review of heavy metal contaminations in urban soils, urban road dusts and agricultural soils from China. Microchem. J. 94, 99–107. doi:10.1016/j.microc.2009.09.014
Wilbur, S., Abadin, H., Fay, M., Yu, D., Tencza, B., Ingerman, L., et al. (2012). Toxicological profile for Chromium. Atlanta (GA): Agency for Toxic Substances and Disease Registry US.
Wilcke, W., Müller, S., Kanchanakool, N., and Zech, W. (1998). Urban soil contamination in Bangkok: heavy metal and aluminium partitioning in topsoils. Geoderma 86, 211–228. doi:10.1016/S0016-7061(98)00045-7
Wong, C. S. C., Li, X., and Thornton, I. (2006). Urban environmental geochemistry of trace metals. Environ. Pollut. 142, 1–16. doi:10.1016/j.envpol.2005.09.004
Wong, M. H., Wong, J. W. C., and Chen, T. B. (1996). “Trace metal contamination of the Hong Kong soil environment: a review,” in Contaminants and the soil environment in the australasia-pacific region Kluwer Academic Publ, 501.
Yang, T., Liu, Q., Li, H., Zeng, Q., and Chan, L. (2010). Anthropogenic magnetic particles and heavy metals in the road dust: magnetic identification and its implications. Atmos. Environ. X. 44, 1175–1185. doi:10.1016/j.atmosenv.2009.12.028
Yongming, H., Peixuan, D., Junji, C., and Posmentier, E. S. (2006). Multivariate analysis of heavy metal contamination in urban dusts of Xi’an, Central China. Sci. Total Environ. 355, 176–186. doi:10.1016/j.scitotenv.2005.02.026
Yuen, J. Q., Olin, P. H., Lim, H. S., Benner, S. G., Sutherland, R. A., and Ziegler, A. D. (2012). Accumulation of potentially toxic elements in road deposited sediments in residential and light industrial neighborhoods of Singapore. J. Environ. Manage. 101, 151–163. doi:10.1016/j.jenvman.2011.11.017
Zhang, C., Qiao, Q., Appel, E., and Huang, B. (2012). Discriminating sources of anthropogenic heavy metals in urban street dusts using magnetic and chemical methods. J. Geochem. Explor. 119–120, 60–75. doi:10.1016/j.gexplo.2012.06.014
Zhang, C., Qiao, Q., Piper, J. D. A., and Huang, B. (2011). Assessment of heavy metal pollution from a Fe-smelting plant in urban river sediments using environmental magnetic and geochemical methods. Environ. Pollut. 159, 3057–3070. doi:10.1016/j.envpol.2011.04.006
Zhang, J., Wang, Y., Liu, J., Liu, Q., and Zhou, Q. (2016). Multivariate and geostatistical analyses of the sources and spatial distribution of heavy metals in agricultural soil in Gongzhuling, Northeast China. J. Soils Sediments 16, 634–644. doi:10.1007/s11368-015-1225-0
Zhang, M., and Wang, H. (2009). Concentrations and chemical forms of potentially toxic metals in road-deposited sediments from different zones of Hangzhou, China. J. Environ. Sci. 21, 625–631. doi:10.1016/S1001-0742(08)62317-7
Keywords: heavy metals, road dust, enrichment factor, geoaccumulation index, pollution load index, Hierarchical analysis, PCA analysis
Citation: Denny M, Baskaran M, Burdick S, Tummala C and Dittrich T (2022) Investigation of pollutant metals in road dust in a post-industrial city: Case study from Detroit, Michigan. Front. Environ. Sci. 10:974237. doi: 10.3389/fenvs.2022.974237
Received: 21 June 2022; Accepted: 29 July 2022;
Published: 09 September 2022.
Edited by:
Jun Zhou, University of Massachusetts Lowell, United StatesReviewed by:
Maurizio Barbieri, Sapienza University of Rome, ItalyTing Wang, University of Massachusetts Lowell, United States
Copyright © 2022 Denny, Baskaran, Burdick, Tummala and Dittrich. This is an open-access article distributed under the terms of the Creative Commons Attribution License (CC BY). The use, distribution or reproduction in other forums is permitted, provided the original author(s) and the copyright owner(s) are credited and that the original publication in this journal is cited, in accordance with accepted academic practice. No use, distribution or reproduction is permitted which does not comply with these terms.
*Correspondence: Mark Baskaran, Baskaran@wayne.edu