- College of economics and management, Northeast Agricultural University, Harbin, China
There are differences in resource endowments, environmental regulations, and production functional areas among different regions in China, which makes dairy farming have certain heterogeneity and imbalance in pollution control, which is mainly reflected in the differences in the environmental efficiency of dairy farming among regions. By analyzing the convergence trend of the dairy farming environment in different regions and scales, it is helpful to predict the potential of improving environmental efficiency, and promote the high-quality development of dairy farming. Based on the dairy farming data of 27 provinces (cities and districts) in China from 2004 to 2019, the SBM model of unexpected output was used to measure the environmental efficiency of dairy farming on different scales. It was found that there were certain differences in the environmental efficiency of dairy farming in China. The overall environmental efficiency of dairy farming showed a “U" evolution trend, including small-scale, medium-scale, and large-scale. The average values of their environmental efficiency were 0.6859, 0.6930, and 0.6855 respectively, and the environmental efficiency of medium-scale dairy farming was the best. The convergence analysis of environmental efficiency showed that there was a conflict between the traditional σ convergence and β convergence results. However, the club convergence showed that the environmental efficiency of small-scale dairy farming converged in general, which could be further divided into two club convergence. The environmental efficiency of medium-scale and large-scale dairy farming did not converge in general but did converge in four different clubs, respectively. Taking the highest environmental efficiency value in the “club” as the reference, the potential to improve the environmental efficiency of dairy farming in different sizes was calculated. The prediction results showed that the gap between the actual value and the potential value was narrowing. It is expected that the potential to improve the environmental efficiency of dairy farming in China will remain above 10% in the next 10–20 years. Based on the above studies, this paper put forward some policy suggestions, such as changing the development direction of dairy farming, changing scale expansion to structural upgrading, implementing differentiated environmental efficiency improvement strategies, narrowing the efficiency gap between regions, paying attention to the input-output structure of factors, and improving the efficiency of breeding environment from the perspective of “eating” and " emission ".
1 Introduction
The economy has shifted from a stage of high-speed growth to a stage of high-quality development in China1. It is necessary to promote a comprehensive green transformation of the economy and achieve new progress in the construction of ecological civilization. The main direction of high-quality development is to improve the quality of supply, the main problem is to solve the problem of unbalanced and insufficient development, and the main idea is to change the extensive consumption of resources to refined energy conservation. The concept of high-quality development not only points out the direction for solving the main contradictions of Chinese society in the new era but also puts forward new requirements for industrial development. To promote the high-quality development of animal husbandry, it is necessary to continue to promote the green and circular development of animal husbandry, vigorously promote the resource utilization of livestock and poultry breeding waste, promote the circulation of agriculture and animal husbandry, and comprehensively improve the level of green breeding, so as to reduce the environmental impacts associated with livestock production while addressing the increased demand for meat products (European Commission 2020)2. Therefore, during the “14th Five-Year Plan” period, the animal husbandry industry urgently needs to improve its quality and competitiveness and form a new pattern of high-quality development that is resource-saving and environmentally friendly in China.
As an important part of animal husbandry, the dairy farming industry is transforming and upgrading to high-quality development in China. With the increasing demand for dairy products, the scale and intensification of dairy farming have been continuously improved (Rehman et al., 2019). According to the statistics in “Review of China’s Dairy Industry Economic Situation in 2020 and Outlook for 2021″2, dairy consumption demand in 2020 has achieved the fastest growth in the past 15 years. If the total domestic milk production and the total import of dairy products equivalent to raw milk are calculated, the total demand for dairy products in 2020 has reached 54.31 million tons, with an increase of 8.0% compared with 2019. It is also the fastest-growing year for consumer demand for products. From the consumption demand for dairy products forcing the development of the dairy farming industry, it can be found that the level of dairy farming in China is constantly improving, the dairy industry pattern is gradually approaching large-scale and intensive, and the feeding conditions, industrial quality and production level of large-scale pastures are gradually improving. Driven by both demand growth and rising costs, the price of raw milk continued to climb in the second half of 2020, with the annual average price increasing by 3.8% year-on-year. The profitability of dairy farming has been significantly improved, with an average annual gross profit of 13.4%. According to the tracking and monitoring data of raw milk output of certified raw milk purchasing stations in China3, small scattered dairy cow farmers will speed up their withdrawal in 2020, and the number will continue to decrease. The process of large-scale farming is gradually accelerating. As of the end of the fourth quarter of 2020, the number of farms (households) involved in the nationwide licensed raw milk purchase stations decreased by 7.4% month-on-month and 11.3% year-on-year. The average number of farms (households) involved in raw milk purchasing stations increased by 10.4% month-on-month and 22.3% year on year.
Large-scale farming has obvious comparative advantages in saving costs, increasing production, and ensuring supply. Concentration of livestock production leads to other non-nutrients that can contribute to environmental pollutants (Rehman et al., 2017). Although reduction of livestock production in China may not be enough to reduce CO2 emissions in the short-run (Rehman et al., 2021), environmental pollution caused by dairy farming has become increasingly prominent. Dairy cows are the third-largest manure producing animal species after pigs and beef cattle, and milk have positive and constructive association with CO2 emission (Hussain and Rehman, 2022). Their manure emissions are rich in the chemical oxygen demand (COD), total nitrogen (TN), and total phosphorus (TP). During the breeding of dairy cows, the emissions will not only cause eutrophication of water but also pollute the air and soil (Baker, 2002). Compared with the United States, an adult cow consumes about 5 mu of manure, and a cow in China has less than 2 mu of land4, while large-scale dairy farms produce more manure, and the daily manure discharge of pastures with 1000 cows can reach about 40 tons, and the lack of sufficient land to absorb manure will lead to unsustainable dairy farming5. In the “First National Pollution Source Census Bulletin"6, the COD and AN emissions of the livestock and poultry breeding industry were 12.6826 million tons and 717,300 tons respectively, accounting for 95.8% and 78.1% of the COD and AN emissions from agricultural sources, accounting for 41.9% and 41.5% of national COD and AN emissions. The daily production of manure from dairy cows is significantly higher than that of other livestock and poultry. The amount of manure produced by an adult cow in one day is 3.6 times that of live pigs, 13.5 times that of mutton sheep, 219.0 times that of broiler chickens, and 117.0 times that of laying hens. A large number of manure and waste emissions cause the deterioration of the rural ecological environment increasingly serious. To effectively solve the problem of environmental pollution caused by dairy cattle breeding, we can start by improving the environmental efficiency of dairy cattle breeding.
Environmental efficiency reflects the degree of pollutant reduction under the condition of constant output and input, which can accurately measure the environmental pollution problem of dairy farming. In addition, there are differences in resource endowments, environmental regulations, and production functional areas in different regions, resulting in a certain heterogeneity and imbalance in the pollution control of dairy cattle breeding. It is mainly reflected in the differences in the environmental efficiency of dairy cattle breeding between regions. What is the current status of the environmental efficiency of dairy farming in China? What are the differences in the environmental efficiency of dairy farming between different scales in China? What is the evolution trend of dairy farming environmental efficiency in China in the long run? What is the potential for improving the environmental efficiency of dairy farming in China in the future? Answers to the above questions have important theoretical guiding significance and practical decision-making significance for the realization of environment-friendly development of dairy farming in China. This paper incorporates pollutant emissions into the analysis of the dairy farming efficiency model. Through the measurement and convergence analysis of dairy farming environmental efficiency, it is expected to clarify the evolutionary characteristics of dairy farming environmental efficiency in China and promote dairy cattle between different regions and different scales. The coordinated development of the breeding industry will improve the overall level of the dairy farming industry in China.
The following contents of this paper are arranged as follows: The second part is a literature review, including measurement methods of environmental efficiency, environmental efficiency measurement of dairy farming, and convergence test of environmental efficiency. Based on this, we will explore the shortcomings of existing studies and possible innovations in this paper. The third part is the environmental efficiency measurement of dairy farming in China. By constructing the SBM model of unexpected output, the environmental efficiency of dairy farming was measured, the differences of environmental efficiency of dairy farming in different regions and different scales were observed, and the reasons were analyzed. The fourth part is the environmental efficiency convergence trend of dairy farming in China. Based on the traditional σ convergence and β convergence, the environmental efficiency convergence of different scales of dairy farming is preliminarily identified, and the club convergence method is used as the main tool to test and analyze the environmental efficiency convergence of dairy farming at different scales. The fifth part further analyzes the potential of improving the environmental efficiency of dairy farming in China. Based on the calculation results of the environmental efficiency of dairy farming and the convergence analysis of the club, from the optimal improvement plan of input factors and pollutant emissions and the convergence of all members of the club. The two dimensions of growth space further analyze the potential of improving the environmental efficiency of dairy farming at different scales; the sixth part is discussion, focusing on the limitations of this study and future research directions. The seventh part is the research conclusion and policy implications.
2 Literature review
Environmental efficiency (EE) simultaneously targets economic benefits and environmental protection, requiring producers to provide higher levels of value under the premise of lower material input and lower emissions (Yan et al., 2020). Reinhard (1999) simplified the definition of environmental efficiency and believed that environmental efficiency is the ratio of the minimum quantity of pollutants that can be achieved to the actual quantity under the condition of maintaining output and conventional factor inputs unchanged. Combined with the needs of the research problem, this paper sorted out the environmental efficiency measurement method, the environmental efficiency measurement of the aquaculture industry, and the environmental efficiency convergence test in detail, so as to find the breakthrough and marginal contribution.
There are mainly two kinds of methods to calculate environmental efficiency, namely the parametric method and the nonparametric method. The former is represented by stochastic frontier analysis (SFA), and the latter is represented by DEA. DEA can not only solve the problems of multiple input and multiple output agricultural economies, but also effectively avoid the controversy of subjective data empowerment (Charnes et al., 1978). However, the traditional DEA model is based on the ideas of “input minimization” and “output maximization”, ignoring the negative output after incorporating environmental factors.
With the continuous maturity of technology, more and more studies have regarded pollution emissions on agricultural production efficiency. First of all, many studies have introduced pollutants as input variables into the DEA model, mainly including shadow price (Li et al., 2016) and the input variable method (Reinhard et al., 2000; Hailu and Veeman, 2001). Secondly, some scholars regard environmental pollution as a negative output. The methods used mainly include the reciprocal transformation method, the transformation vector method, the directional distance function method, and the SBM model (Scheel, 2001; Färe and Grosskopf, 2004). Fare proposed the directional distance function based on weak-disposal best-practice production frontiers, which further improved the DEA calculation method of environmental efficiency and realized the minimization of environmental pollution (Färe et al., 1989). Among them, the SBM-Undesirable model can deal with complex undesired output problems, and better solve the calculation deviation caused by the selection of angles and radial directions of the traditional DEA model (Tone and Tsutsui, 2010; Le et al., 2020).
In animal husbandry, DEA has been extensively used to measure the environmental efficiency of pig breeding (Galanopoulos et al., 2006). Especially in China, with the transformation of the hog industry, environmental externalities have become a very serious problem (Kuhn et al., 2020), and some studies have added negative output into the evaluation system of pig breeding efficiency (Kuhn et al., 2020; Zhong et al., 2021). Furthermore, many studies have used the SBM-DEA model with negative output to investigate environmental efficiency (Yao et al., 2017). In the dairy industry, technical and environmental efficiency were significantly and positively correlated (Soteriades et al., 2015), and variation in dairy management systems contributes to differences in environmental efficiency (Adenuga et al., 2018). There are differences in the environmental efficiency of dairy enterprises, and large dairy enterprises are environmentally inefficient compared with small dairy enterprises (Njuki et al., 2016).
For the calculation of the environmental efficiency of dairy cattle in the above studies, the amount of concentrated feed, green forage cost, labor number, fixed asset depreciation, and others are generally selected as input variables, and the output of main products and by-products are the expected output, and the amount of pollutant discharge is regarded as the undesired output.
The economic growth convergence theory is derived from the Solow model (Solow, 1956). The key assumption of the Solow model is the diminishing marginal returns of capital. The existence of this assumption enables the economy to converge to a stable state. Given the same exogenous factors, all economies eventually converge to the same stable state, that is, “unconditional convergence”. The concept of unconditional convergence is too harsh. Different economies should have different balanced growth paths and converge to different steady states, that is, “conditional convergence”. Subsequently, the academic community has begun to enrich the content of convergence theory and has formed σ convergence, β convergence, and club convergence (Phillips and Sul, 2007; Kounetas et al., 2021). With the deepening of research, many scholars have begun to test the dynamic evolution trend of environmental efficiency convergence (Guo et al., 2019). Countries with high and low environmental efficiency values tend to form club convergence (Camarero et al., 2013), environmental efficiency convergence is conditional on industrial structure, globalization, and consumer price index (Sun et al., 2020).
To sum up, the existing studies have extensively and deeply studied the measurement methods of environmental efficiency, the measurement of environmental efficiency of aquaculture and efficiency convergence, which lays a good theoretical foundation for this paper and provides useful experience for reference, but there is still room for further expansion: First of all, for the choice of efficiency measurement methods, most studies still use the traditional DEA model to measure the efficiency. However, the traditional DEA model can not fully consider the impact of “relaxation variables” on the efficiency value, resulting in deviation in the calculation of efficiency. Secondly, the existing measurement of environmental efficiency of breeding industry pays more attention to the environmental efficiency of pig breeding, while the in-depth research on the environmental efficiency of cow breeding is still insufficient. Cows are the third largest fecal livestock after beef cattle and pigs, so it is necessary to further study its impact on the ecological environment. Thirdly, based on the calculation of the environmental efficiency of dairy farming, it is necessary to carefully analyze its convergence mechanism. Therefore, based on the data on dairy farming in China from 2004 to 2019, this paper uses the SBM-Undesirable model to measure the environmental efficiency of dairy farming in China, and further uses the club convergence test method to test the convergence of the environmental efficiency of dairy farming in China, and improve the potential of environmental efficiency of dairy farming in China. At the same time, this paper explores how to improve the environmental efficiency of dairy farming from input and output.
Compared with previous studies, the possible marginal contributions of this paper are as follows: First of all, there are a lot of controversies on which scale of dairy farming environment is the best. Based on available data, this paper calculates and compares the environmental efficiency of dairy farming with different scales in China as comprehensively, systematically, and scientifically as possible, explores the evolution trend of environmental efficiency of dairy farming with different scales, gives practical reasons and explanations and provides decision-making for the direction of moderate scale farming in the future. Secondly, previous studies have further explored which factors restrict environmental efficiency, including individual characteristics of dairy farming, technical level, and farming scale. However, these studies ignore the most critical elements, which are the improvement of the input and output of dairy farming. How much room for improvement in the environmental efficiency of dairy farming is more concerned about and intends to solve in this paper.
3 Measurement of environmental efficiency of dairy breeding in China
3.1 Data sources
Because of the availability of data, this paper selects the statistical data of dairy farms with different scales from “Compilation of National Agricultural Product Cost and Benefit Information” (2005–2020) and " Pollution Coefficient Manual " in 27 provinces in China, and calculate and analyze the environmental efficiency of dairy farming. This paper discusses small-scale, medium-scale, and large-scale dairy farming. Since the premise of convergence research is to ensure the continuity of the data, this paper organizes dairy farming data of different scales into balanced panel data, and 208 small-scale, 304 medium-scale, and 224 large-scale dairy farming samples are obtained, and the samples are evenly distributed in 27 provinces of China, which can better reflect the actual situation of dairy farming in China. In addition, all the indicators involving price in the statistical data are deflated correspondingly by the price index of the corresponding product in the “China Statistical Yearbook” of the current year to avoid the impact of large price fluctuations on the stability of the data. To analyze the differences and convergence of dairy farming environmental efficiency between different provinces and different scales to the greatest extent, this paper uses the interpolation method or weighted average method to supplement the provinces with missing data in some years.
3.2 Model design
In addition to the dairy products (desired output), pollutants such as feces and urine (undesired output) are also obtained in the process of dairy farming. These pollutants include substances such as chemical oxygen demand (COD), total nitrogen (TN), total phosphorus (TP), copper (Cu), and zinc (Zn). The objective function of the SBM model includes the slack variables of input and output, which can effectively solve the slackness of input and output and the deviation caused by radial and angle selection. While obtaining the required efficiency, the SBM model can also obtain the input elements of the decision-making unit, the improvement goal and the degree of the undesired output. Therefore, this paper uses the SBM model of the undesired output to measure the environmental efficiency of dairy farming in China.
The model building process is as follows:
Assuming that there are n decision-making units in a production system, and each decision-making unit contains m inputs, s1 desirable outputs, and s2 undesired outputs, it can be expressed in vector form as:
Define matrices X、Yg、Yb as:
X > 0, Yg > 0, Yb > 0, the above production set can be transformed into:
λ ≥ 0 represents constant returns to scale (CRS), and if the equation satisfies both λ ≥ 0 and λ=1, it represents variable returns to scale (VRS). If the results obtained under the CRS and VRS are different, the results obtained under the assumption of VRS should be considered (Zheng et al., 1998). Therefore, this paper is based on the research hypothesis of VRS when measuring the environmental efficiency of dairy farming in China. x≥Xλ means the actual input is greater than the production frontier input; yg≤Ygλ means the actual expected output is less than the production frontier expected output; yb≥Ybλ means the actual undesired output is greater than the production frontier undesired output.
The traditional DEA model can neither fully consider the impact of the “slack variable” on the efficiency value, nor take into account the technical changes of how much the expected output increases and how much the undesired output decreases. To make up for this deficiency, Tone proposed an environmental efficiency model based on input and output slack variables, namely the SBM model, and further expanded the SBM model in 2004, so as to obtain an evaluation of environmental efficiency under the condition of undesired output (Tone, 2001). The SBM efficiency model of a specific decision unit (x0, y0g, y0b) can be expressed as:
s-, sg, sb represent the slack variables of the input variable, desired output, and undesired output, respectively; The objective function p* is strictly decreasing concerning s-, sg, sb. When s-=sg=sb=0, the function has an optimal solution, that is, p*=1 means the decision-making unit is sufficiently effective; if 0≤p*≤1, it indicates that there is a loss of efficiency in the falsely measured unit (environmental inefficiency). In this case, the input and output can be improved, and the magnitude of improvement is determined by the proportion of the slack variable to the respective input and output. Environmental inefficiency can be decomposed into input inefficiency and output inefficiency:
3.3 Explanation and descriptive statistics of variables
Input variables in this paper include the fee of concentrated feed, green roughage input, labor input, and fixed assets (Njuki et al., 2016). Among them, the input of concentrated feed refers to the total input of grain, beans, compound feed, mixed feed, and others (Yu and Associate, 2012; Bai et al., 2022); the input of green roughage refers to the total input of green feed, silage, hay, straw, and others; the labor input refers to the sum of the quantity and the number of employees, and the fixed asset input is represented by the fixed asset depreciation expense. On the whole, large-scale dairy farms have the highest input in concentrate feed, green roughage, and fixed assets, followed by medium-scale and small-scale dairy farms, while small-scale dairy farms have the largest labor input, followed by medium-scale and large-scale dairy farms. Desirable output variables include two types: main product output and by-product output value, where main product output refers to raw milk output, by-product output value refers to the total output value of other products except raw milk and the main product output of large-scale dairy farms and the highest value of by-products. The undesired output is measured by the total amount of pollutant discharge. The pollutant discharge coefficient is given in the “Pollution Coefficient Manual”, including chemical oxygen demand (COD), total nitrogen (TN), total phosphorus (TP), copper (Cu) and zinc (Zn), the above pollutant emissions (g/head-d) are used, to sum up as the undesired output. From the perspective of pollutant emissions, the small scale is the largest, the large scale ranks the second, and the medium scale is the smallest. The explanation and descriptive statistics of variables are shown in Table 1.
3.4 Calculation results
DEA-SOLVER Pro5.0 was used to measure the environmental efficiency of dairy farming with different scales in 27 provinces in China from 2004 to 2019 (Table 2). It was found that from 2004 to 2019, there was little difference in the average environmental efficiency of dairy farming with different scales, and the medium-scale efficiency value was the highest. In theory, compared with small-scale and medium-scale, the environmental efficiency of large-scale dairy farming should be the highest, but on the whole, the environment of large-scale dairy farming has not been effectively improved, which might result from the fact that the possible reason was that the construction of large-scale dairy farming had not yet reached the ideal state, and there was still a lot of pressure on waste disposal due to its large scale. For the small-scale dairy farming, Ningxia had the highest efficiency value of 0.8588, Yunnan, Hebei, Shandong, Jilin, and Hunan efficiency values are between 0.7–0.8, and Liaoning, Inner Mongolia, Heilongjiang and Shanxi are between 0.6–0.6 0.7, while the efficiency values of Henan, Fujian, and Guangxi were all less than 0.6. For medium-scale dairy farming, the efficiency values of Beijing, Xinjiang, Gansu, Ningxia, and Hunan were all higher than 0.8, and the efficiency values of Shaanxi, Jilin, Tianjin, Guangxi, Inner Mongolia, and Shanxi were between 0.7 and 0.8, the efficiency values of Liaoning, Heilongjiang, and Anhui were between 0.6 and 0.7, while the efficiency values of Henan, Shanghai, Chongqing, Fujian, and Sichuan were all less than 0.6. For the large-scale dairy farming, Gansu had the highest efficiency value at 0.9392, Beijing and Xinjiang were both above 0.8, 0.8886, and 0.8180 respectively, Qinghai and Fujian ranged from 0.7 to 0.8; Hubei, Shanxi, Shandong, Heilongjiang, Henan, Anhui, and Zhejiang ranged from 0.6 to 0.7, while the efficiency values of Guangdong, Liaoning, and Jiangsu were all below 0.6.
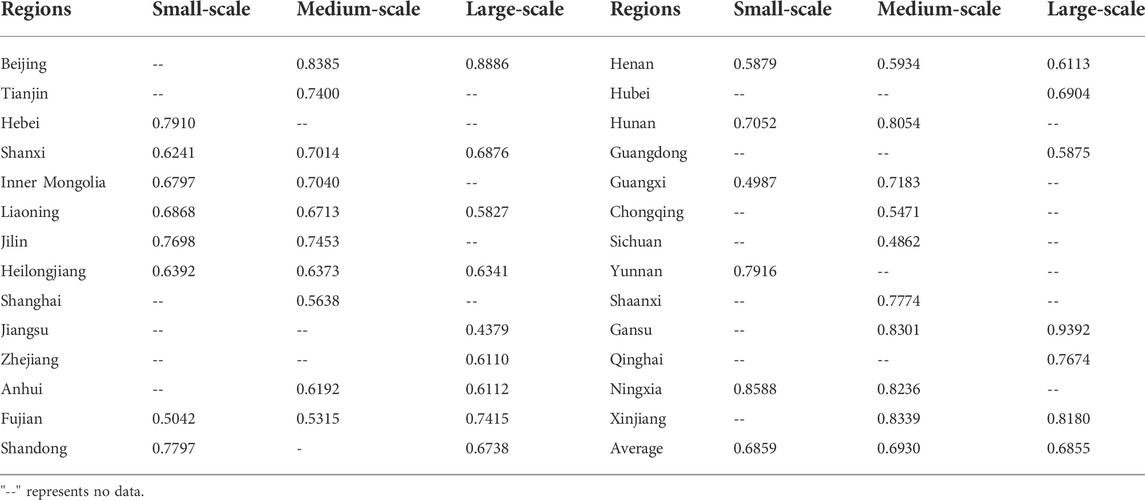
TABLE 2. Calculation results of environmental efficiency of dairy farming in different scales among provinces in China from 2004 to 2019.
To further observe the evolution trend of the environmental efficiency of dairy farming with different scales, this paper drew a trend map of the environmental efficiency of dairy farming with different scales in China from 2004 to 2019 (Figure 1), including changes in the average environmental efficiency of dairy farming with different scales, as well as changes in linear trends. On the whole, the environmental efficiency of dairy farming showed a “U"-shaped evolution trend. From 2004 to 2016, the environmental efficiency of dairy farming with different scales showed signs of continuous decline. After 2016, the environmental efficiency of medium-scale and large-scale dairy farming showed a steady increase and stabilized. The environmental efficiency of small-scale dairy farming increased significantly from 2016 to 2018 and then dropped rapidly in 2019. From the linear curve, it could be found that from 2004 to 2019, the environmental efficiency of small-scale dairy farming was in a sharp decline, and the environmental efficiency of medium-scale and large-scale dairy farming was relatively flat. The environmental efficiency of dairy farming was directly related to the national environmental pollution control policy, and the slowdown in economic growth provided more possible space for environmental improvement.
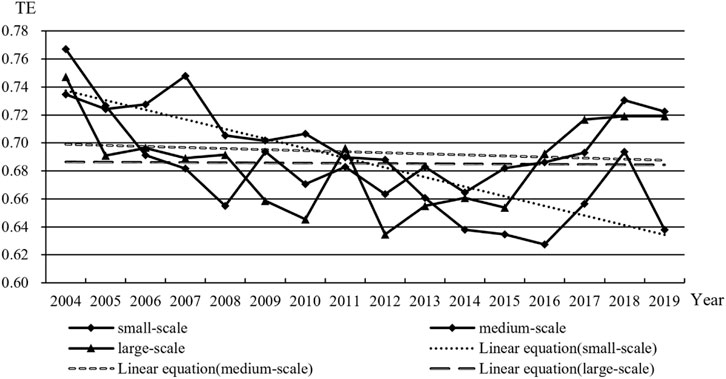
FIGURE 1. Variation trend of environmental efficiency of dairy farming on different scales in China from 2004 to 2019.
In 2016, the 14th meeting of the central financial and economic leading group discussed the resource utilization of livestock and poultry breeding waste for the first time, which provides basic guidelines and important guidelines for solving the problem of livestock and poultry breeding pollution. Since then, the central ministries and commissions have issued a series of action plans around the resource utilization of livestock and poultry breeding waste, which have made detailed deployment and top-level design for the treatment of livestock and poultry breeding environmental pollution, thus laying a solid foundation for the revitalization of rural ecology and the green transformation of industry. The introduction of these policies provides a good explanation for the improvement of the environmental efficiency of dairy farming after 2016 and the continuous decline of the environmental efficiency of dairy farming before 2016. The continued decline in the environmental efficiency of small-scale dairy farming is not inconsistent with current policies. After the outbreak of the “melamine” incident in 2008, the quality control of raw milk has become more and more strict (Yu and Associate, 2012), and the national policy has begun to support moderate-scale breeding, and guide small-scale farms to continuously withdraw from the breeding industry. There is a big gap between small-scale dairy farms and other scale dairy farms in terms of green production technology adoption and environmental governance investment. It is also an indisputable fact that their environmental efficiency is low. Relevant policies still need to be promoted to continue to guide the exit of small-scale dairy farms.
4 Convergence trend of environmental efficiency of dairy farming in china
4.1 Convergence theory analysis and model design
Convergence has been a topic of concern in economics for a long time, and the mechanisms of convergence can be divided into σ convergence, β convergence, and club convergence. β convergence means that the economic growth rate of backward regions is faster than that of developed regions, while σ convergence means that the gap between regions gradually narrows over time. These two convergence methods are closely related, and β convergence is a necessary but not sufficient condition for the σ convergence. Since σ convergence describes the trend of a certain index difference between regions, the coefficient of variation (CV) index can be used to measure the trend of the difference in dairy farming environmental efficiency between provinces. The specific expression is as follows:
σ represents the standard deviation of the environmental efficiency of dairy farming, Y represents the environmental efficiency of dairy farming,
Since β convergence is a necessary but not sufficient condition for σ convergence, it is difficult to deny the existence of β convergence from the above-mentioned σ convergence, so β convergence test is required. The β convergence model can be expressed as:
CPit and CPi0 represent the environmental efficiency of dairy farming in the t period and the initial period, respectively, T represents the spanned period, α and β represent parameters to be estimated, and εit represents the error term. If β < 0 and there is significance, it means that the environmental efficiency of dairy farming in this period has a β convergence trend. Correspondingly, the convergence rate can be calculated as:
If the growth rate of environmental efficiency in the lagging regions is much higher than that in the leading regions, resulting in the lagging regions surpassing the leading regions in the late stage, and the gap between the two is higher than that in the initial stage, the β convergence result obtained by the traditional Barrro equation is wrong (Hadri, 2000). At the same time, if there is a lot of noise in the data generation process, the traditional t-test is also inappropriate. To this end, Phillips proposed the club convergence test method (Phillips and Sul, 2007). The club convergence test method allows data to have various time trends and individual heterogeneity. The convergence of individuals is clustered, and individuals that converge to the same stable level will be divided into a convergence club group so that all local convergence conditions can be screened out using statistical methods to form different convergence clubs. This paper mainly analyzes the convergence of the environmental efficiency of dairy farming in China. Since there is no comparability between the environmental efficiency values measured by the SBM model in different years, only the differences between provinces within the same year can be analyzed. Therefore, this paper will use the club convergence test method to further analyze the convergence of environmental efficiency of dairy farming in China to understand the dynamic evolution characteristics. The analysis process is as follows:
For any panel data Xit, it can be decomposed into two parts:
μi represents a common factor for all individuals, and δit represents the loading matrix of time-varying factors, which can be further expressed as semiparametric equations:
δi represents a fixed component, which does not change with time; ξit obeys standard normal distribution and independent and identical distribution, but is weakly dependent and stable in time.
L(t) is a growth change equation used to eliminate the natural increase of variance with time. If the panel data is stationary, L(t) can be ignored, which ensures that for any a greater than or equal to 0, when time t tends to infinity, ξit will converge to δi. Therefore, if all individuals have the same common factor δi=δ, when a is greater than or equal to 0, all individuals will converge to the same equilibrium state, so the convergence or not can be judged by checking whether a is greater than or equal to 0. In the empirical analysis, convergence can be tested by the following model:
H0: δi=δ, a≥0, the null hypothesis can be transformed into the following logt test:
It represents that only part of the data is used in the regression. Therefore, the null hypothesis of convergence can be judged by a one-sided t-test robust to autoregression and heteroscedasticity to the coefficients of logt.logt test can not only judge the convergence of the sample population but also further judge whether the individual converges with different clubs when the population does not converge, that is, the club converges.
4.2 Traditional convergence test empirical results
4.2.1 σ Convergence test results
The coefficient of variation of the environmental efficiency of dairy farming with different scales is shown in Figure 2. The coefficient of variation of the environmental efficiency of small-scale, medium-scale and large-scale dairy farming all showed fluctuations of different magnitudes. The coefficient of variation of the environmental efficiency of small-scale dairy farming was basically in a state of continuous decline from 2004 to 2016. However, after 2016, there had been a trend of continuous fluctuations, indicating that the gap in the environmental efficiency of small-scale dairy cattle breeding in various provinces first narrowed and then expanded. The coefficient of variation of the environmental efficiency of medium-scale and large-scale dairy farming had been in a continuous state of oscillation from 2004 to 2019. Compared with medium-scale, large-scale fluctuations were relatively flat, indicating that the gap in the environmental efficiency of medium-scale and large-scale dairy farming in various provinces had not significantly narrowed or expanded. To sum up, it could be preliminarily determined that there was no sign of σ convergence in the overall environmental efficiency of small-scale, medium-scale, and large-scale dairy farming.
4.2.2 β Convergence test results
The absolute β convergence of the environmental efficiency of dairy farming with different scales is shown in Table 3. The small-scale, medium-scale, and large-scale regression results show that the estimated coefficients of ln (CPi0) are all negative, and they pass the significance test at the 1% level, which shows that there is an absolute β convergence phenomenon in the environmental efficiency of small-scale, medium-scale and large-scale dairy farming. Within the small-scale, medium-scale, and large-scale dairy farming, provinces with low environmental efficiency will move toward provinces with higher environmental efficiency are approaching. From the comparison of the regression coefficients, the large scale is more obvious, followed by the medium scale, and the small scale will be relatively slow, which is related to the current policy of promoting the moderate scale breeding of dairy cows.
4.3 Club convergence test empirical results
In the process of constructing the convergence model, it is pointed out that both the σ convergence test and the β convergence test ignore the convergence club. Therefore, it is necessary to use club convergence to test the convergence of environmental efficiency of dairy farming with different scales. Before performing the club convergence test operation, it is necessary to use logt test to explore the convergence of the environmental efficiency of dairy cows of different scales as a whole. The results showed that the T-value of the environmental efficiency of small-scale dairy farming was greater than -1.65, and the T-values f the environmental efficiency of medium-scale and large-scale dairy farming were both less than -1.65, indicating that the environmental efficiency of small-scale dairy farming has generally converged, while medium-scale and large-scale dairy farming. There is no overall convergence trend in environmental efficiency (Table 4).

TABLE 4. Overall convergence test (logt test) of environmental efficiency of dairy farming in different scales.
The overall lack of convergence does not mean that there is no convergence trend. There may also be club convergence in the environmental efficiency of dairy farming with different scales. It is necessary to use the logt test to explore the convergence combination of dairy farming environmental efficiencies of different scales. According to Table 5, it can be found that there is club convergence in the environmental efficiency of dairy farming with three scales, in which the small-scale converges in two clubs, and the medium-scale and large-scale converge in four clubs respectively.
Firstly, the environmental efficiency of small-scale dairy farming has 11 provinces that converge to the first club, including Hebei, Shanxi, Inner Mongolia, Liaoning, Heilongjiang, Fujian, Henan, Hunan, Guangxi, Yunnan, and Ningxia, while Jilin and Shandong converge to the second. club. Due to the pressure of market competition and policy evolution, small-scale dairy farms are gradually withdrawing from the ranks of dairy farming. The support for small-scale dairy farms in various regions is not high. Therefore, the development trend of environmental efficiency of small-scale dairy farming is the same. Shandong and Shandong are both major dairy farming provinces and major dairy farming areas. The exit rate of small-scale dairy farms is relatively slow, and the environmental efficiency is relatively low.
Secondly, the environmental efficiency of medium-scale dairy farming has 6 provinces that converge to the first club, including Beijing, Jilin, Anhui, Guangxi, Ningxia, and Xinjiang, 2 provinces converge to the second club, including Shanxi and Gansu, and 6 provinces converge to the third club, 6 provinces converge on the third club, including Tianjin, Heilongjiang, Shanghai, Fujian, Henan, Hunan, 4 provinces converged to the fourth club, including Liaoning, Chongqing, Sichuan, Shaanxi, while Inner Mongolia showed a divergent trend. The number of medium-scale dairy farms in operation is between 50 and 500. Based on the basic fact of “more people and less land” in China, the expansion of the dairy farming scale should be a gradual process. When the competitiveness of the industry is low, it is emphasized that excessive scale and unreasonable scale structure have accelerated the rapid growth of breeding costs. According to the field investigation, it is also found that whether it is based on land bearing constraints, or based on labor costs, fixed asset investment, and other constraints, medium-scale dairy farms have the most development potential. However, due to the differences in resource endowments in various regions, the gap between the environmental efficiency of medium-scale dairy farming will gradually emerge. Therefore, the environmental efficiency of each province will converge to different groups.
Thirdly, the environmental efficiency of large-scale dairy farming has 3 provinces that converge to the first club, namely Shanxi, Shandong, and Gansu, and 5 provinces that converge to the second club, namely Beijing, Heilongjiang, Fujian, Hubei, and Xinjiang, and 2 provinces converge to the second club. The third club is Qinghai and Guangdong, and the four provinces converge to the fourth club, namely Jiangsu, Zhejiang, Anhui, and Henan, while Liaoning shows a divergent trend. Compared with medium-scale dairy farms, the number of large-scale dairy farms in operation is larger, with more than 500 heads. Large-scale dairy farms are more common in northeast China, Inner Mongolia, and Xinjiang, mainly because these areas are relatively rich in land resources and can be consumed. The wasteland is relatively large, and at the same time, because of its huge scale, it has obtained a lot of subsidies from the state for manure pollution control. However, subsidies have not changed the status quo of huge pollution emissions, and they are still the main body of pollution emissions. This situation has not fully fitted the original purpose of the policy of supporting large-scale farming entities, nor has it narrowed the gap in the environmental efficiency of dairy farming between regions and improved the environmental efficiency of dairy farming, so the development trend has not converged within the same club.
5 Further analysis
Based on the above calculation results of dairy farming environmental efficiency and the club convergence analysis, the potential of dairy farming environmental efficiency improvement on different scales is further analyzed, which is of great significance for formulating effective environmental efficiency improvement policies. The pollution caused by dairy farming to the environment is the result of the combined action of a variety of pollutants. The environmental efficiency of dairy farming is a comprehensive measure of the sustainable development of dairy farming. Taking into account the “amount” of pollutants emitted in the dairy farming process, dairy farming can be characterized in the form of specific values between 0 and 1. The comprehensive level of environmental pollution can also further reflect the growth space of dairy farming environmental efficiency from the following two dimensions.
On the one hand, the SBM model of undesired output can not only give the environmental efficiency value of each decision-making unit but also provide each decision-making unit with the best improvement plan for input factors and pollutant emissions when the efficiency is optimal. From the perspective of the improvement of input factors, the improvement of green roughage and labor force of large-scale dairy farms is lower than that of medium-scale and small-scale dairy farms, which fully shows the advantages of large-scale development, which can reduce necessary waste (Table 6). For concentrate feed and fixed asset input, the improvement is larger, which results from the fact that large-scale farms have relatively sound machinery and equipment, but they have problems such as low profit on fixed assets, poor maintenance, and poor processing technology, which requires the most investment in fixed assets to be reduced. As a production factor with a high proportion of investment in dairy farms, fixed assets also indicate that in the process of further large-scale development in the future, we should pay more attention to the phenomenon of waste of investment in fixed assets. In terms of the degree of improvement of undesired output, contrary to the degree of improvement of most input factors, large-scale dairy farms require the highest degree of improvement, reaching 16.22%, indicating that although large-scale dairy farming has broken through the elements and technologies of traditional breeding models. However, due to the increase in the level of agglomeration, the pressure on the environment during this period is too high, the land resource endowment is weak, and it is difficult to absorb the discharge of a large number of pollutants. Therefore, it is necessary to recycle the waste of large-scale dairy farms and take advantage of questions to focus on. In general, dairy farms of different scales have a large space for investment saving and airborne pollutant emission reduction. The improvement of the environmental efficiency of dairy farming should not only focus on external factors, but also on the improvement of input and output.
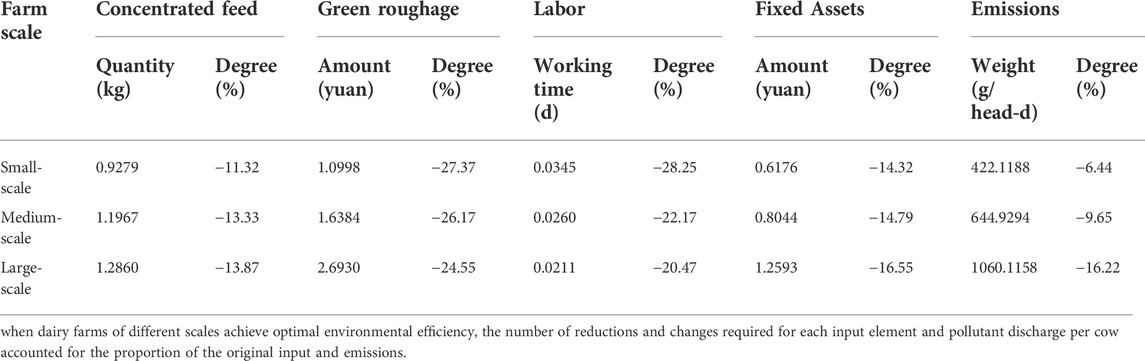
TABLE 6. Quantity and degree of improvement of input factors and pollutant emission of dairy farms of different sizes.
On the other hand, club convergence can judge the growth space of all members. In this paper, the highest environmental efficiency of each convergent club in a year was taken as the potential environmental efficiency level of the club members in that year, and the actual value, potential value, and growth space of dairy farming environmental efficiency of different scales are observed (Table 7). The results show that: First of all, the environmental efficiency of small-scale, medium-scale, and large-scale dairy farming has a large room for growth, among which the environmental efficiency of small-scale dairy farming has the greatest growth potential. However, the withdrawal of the industry should follow the principle of “step by step”, and long-term attention should be paid to the environmental pollution of small-scale dairy farming in the future. Secondly, the growth potential of the environmental efficiency of small-scale, medium-scale, and large-scale dairy farming shows a decreasing trend with time, namely the gap between the actual value and the potential value is constantly narrowing, which further verifies that the difference in environmental efficiency within the club is decreasing. and also shows that the current livestock and poultry environmental pollution control policies have played a significant role.
To predict the improvement potential of dairy farming environmental efficiency on different scales in the future, this paper uses the growth space to perform a simple linear regression on the time trend term (Figure 3). The results showed that the growth space of environmental efficiency of small-scale, medium-scale, and large-scale dairy farming will decrease by 0.24%, increase by 0.008%, and decrease by 1.4%, respectively. and the growth space will reach 15.07%, 17.44%, and 0 by 2035 (large-scale dairy farms have 0 growth space around 2030). Specifically, although the environmental efficiency of medium-scale dairy farming is currently the highest, its potential for improving environmental efficiency has not been significantly reduced, or the environmental efficiency of medium-scale dairy farming will not be the same as the potential growth value of large-scale dairy farming. The possible reason is that in the future, the national demand for “rations” will be further reduced based on ensuring the effective implementation of the national food security strategy, but the trend of high consumer demand for meat, eggs, and milk will continue to increase, especially for dairy products. According to statistics, since 1978, the dietary structure of Chinese residents has undergone significant changes, and the per capita grain consumption has gradually decreased, from 227.0 kg in 1978 to 132.7 kg in 2019, a decrease of 41.5%. In terms of milk consumption by urban and rural residents, the per capita milk consumption of urban residents has increased from 4.6 kg in 1995 to 16.7 kg in 2019, while the per capita milk consumption of rural residents has increased from 0.6 kg to 7.3 kg. With the continuous improvement of the per capita income of rural residents, the per capita milk consumption of rural residents has been on the rise, indicating that in the future, more land will be released, more labor will be released, and more feed grains will be provided for the development of dairy farming, the scale of dairy farming will continue to increase, and the favorable institutional environment and market The environment will promote the transformation of medium-scale dairy farms to large-scale dairy farms, and the environmental governance capacity of dairy farming will also be significantly improved. In short, according to the forecast results, it can be predicted that the potential for improving the environmental efficiency of dairy farming in China will remain above 10% in the next 10–20 years.
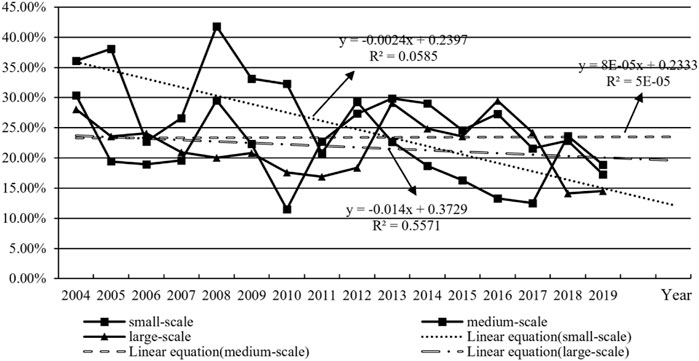
FIGURE 3. Prediction of environmental efficiency improvement potential of dairy farming on different scales.
6 Discussion
With the goal realization of building a moderately prosperous society in an all-round way, people’s material living standards have been significantly improved. According to Maslow’s Hierarchy of Needs Theory, when the most basic food needs (ie, ration needs) are met, the demand for high-protein meat, eggs, milk and other high-protein will further increase. The main supply of milk comes from dairy farming, so the sustainable development of dairy farming is a key concern today and in the future, and this problem is essentially to solve the problem of dairy cows” The problem of “eating” and “emission” has proved that solving “emission” is much more difficult than solving “eating”. In this paper, the environmental efficiency of dairy cattle breeding is used as a key indicator to measure the problem of “emission” in dairy cattle breeding industry, and the SBM model of undesired output is used to measure the environmental efficiency of dairy cattle breeding of different scales in China from 2004 to 2019, analyze its differences. The club convergence test method was used to test the convergence of environmental efficiency of dairy farming, and the potential to improve environmental efficiency was further calculated.
On the whole, this paper solves the four problems raised in the introduction, including the development status of the environmental efficiency of dairy farming in China, the differences in the environmental efficiency of dairy farming between different scales in China, the evolution trend of the environmental efficiency of dairy farming in China, and the potential for improving the environmental efficiency of dairy farming in China in the future. It is found that the larger the scale of dairy farming is, the higher the environmental efficiency is. On the contrary, medium-scale dairy farming has the best environmental efficiency, which further responds to and verifies the scientific connotation of why China proposes “moderate scale farming" (Wang et al., 2016). At the same time, the fluctuation of dairy farming environmental efficiency is not stable in China. This irregular fluctuation not only shows the value of this study, but also suggests that we should always pay attention to the changing trend of dairy farming environmental efficiency in the future. In addition, this paper further explores the improvement space of dairy farming environmental efficiency from the improvement of input and output of dairy farming.
However, this paper also has certain research limitations, as follows: First of all, due to the availability of data, the calculation of the environmental efficiency of dairy cattle breeding in this paper is only updated to 2019, and the impact of the COVID-19 epidemic on dairy cattle breeding has not been considered. At the end of 2019 and the beginning of 2020, the COVID-19 epidemic swept across China, which had a serious impact on the development of the dairy farming industry. The cost of production factors such as feed and labor increased significantly, but the consumption demand for dairy products continued to decline for a long time. It is inevitable that it will change the development pattern of the dairy farming industry, and it will inevitably have a certain impact on its environmental efficiency. However, due to the lack of data, it is impossible to analyze this impact, which is also the biggest regret of this paper. Secondly, only using simple linear regression to predict the improvement potential of dairy farming environmental efficiency may ignore the impact of internal and external factors on dairy farming environmental efficiency. According to the linear regression theory, linear regression can roughly simulate the future development direction and trend based on the previous data trend. Based on the club convergence method, this paper analyzes the evolution trend and improvement potential of dairy farming environmental efficiency. As a supplementary expansion, linear prediction only analyzes the general trend of dairy farming environmental efficiency growth space, and does not take into account the internal and external factors that affect dairy farming environmental efficiency. Considered carefully, this is also the main research flaw in this paper.
Although there are still some limitations in this paper, it also provides a clear direction for future research: First of all, after the data is updated, further in-depth research can be carried out on the impact of the COVID-19 epidemic on the development of dairy farming, especially on environmental efficiency, so as to make up for the lack of existing research in this paper. Secondly, it is necessary to explore more scientific forecasting methods to carry out special forecasting research on the improvement potential of dairy farming environment. At the same time, we can continue to explore the key factors that improve or hinder the dairy farming environment from both theoretical and empirical perspectives.
7 Conclusion and suggestions
This paper draws the following conclusions through research:
Firstly, the environmental efficiency of dairy farming in China was different on different scales. The overall environmental efficiency of dairy farming showed a u-shaped evolution trend, and the average environmental efficiency of small-, medium-, and large-scale dairy farming was 0.6859, 0.6930, and 0.6855, respectively. The environmental efficiency of medium-scale dairy farming was the best.
Secondly, the environmental efficiency of small-scale dairy farming converges in general but can be further divided into two clubs. The environmental efficiency of medium and large-scale dairy farming does not converge in general, and converges in four different clubs, respectively.
Thirdly, further measuring the improvement potential of environmental efficiency of dairy farming in China, it is found that there is a large room for growth of environmental efficiency of dairy farming with different scales, but the growth potential shows a declining trend over time, and the improvement potential of environmental efficiency of dairy farming in China will remain above 10% in the next 10–20 years. To improve the environmental efficiency of dairy farming, attention should be paid to the improvement of input-output factors.
To promote the coordinated development of the dairy farming industry between different regions and different scales and improve the overall level of the dairy farming industry in China. The following policy suggestions are put forward in this paper based on the above discussion:
First of all, change the development direction of the dairy farming industry from scale expansion to structural upgrading. From the measurement results of the environmental efficiency of dairy farming, it can be found that the larger the scale is, the higher the environmental efficiency is. On the contrary, the medium-scale dairy farms have more environmental advantages, which is not only in line with the policy instructions of moderate-scale dairy farming but also in line with the limited capacity of resources and the environment. Therefore, we should continue to support the development of moderate scale dairy cattle farms, encourage exogenous capital to inject into the dairy cattle industry, and form a resource-saving and environment-friendly development pattern of “common development, the joint opening of the new bureau and joint creation of the future”; meanwhile, it is necessary to strengthen the integration of industries, transform and upgrade from “flat” development to “structured” development, support the construction of interest connection mechanism between breeding subjects of different scales and different regions, and try to explore a variety of new ideas of “mutualism".
Secondly, it is necessary to implement differentiated environmental efficiency improvement strategies to narrow the efficiency gap between regions. Judging from the current development reality, there is a big gap in the environmental efficiency of dairy farming among provinces, which is mainly due to the great differences in resource endowments among provinces. Through the club convergence test, “ally regions” with the same development trend and the possibility of cooperating and fighting in the future have been explored. Therefore, differentiated environmental efficiency improvement strategies should be implemented in combination with regional resource endowments. Taking the large-scale dairy farming area converging to the second club as an example, Beijing and Heilongjiang are both advantageous dairy farming areas with high industrial concentration and strong typical demonstration roles. Therefore, advanced and mature environmental governance experience can be transmitted to Fujian and Gansu, and Gansu, as an important production base of alfalfa and oats, can continuously deliver necessary production factors to other regions. Effective cooperation between regions can shorten the gap and form a pattern of resource complementarity.
Thirdly, it is necessary to pay attention to the input-output structure of factors, and improve the environmental efficiency of breeding from “eating” and “discharging”. Through ascension of potential efficiency in different scale dairy farming environments further, it is found that the scale dairy farming has significant room to improve environmental efficiency, and the degree of improvement factor input and output is bigger, so the structure of input and output factors of farming should be adjusted by itself, so as to avoid waste of resources and production pressure. On the one hand, it is necessary to formulate an efficient and reasonable factor input plan to ensure that every kilogram of feed input, every working hour of labor input, and every unit of fixed assets input are meticulous; on the other hand, in the treatment of waste, especially manure, it is necessary to have scientific methods, proper operation, and simplified process, and realize the importance of solving the problem of “emission”.
Data availability statement
The raw data supporting the conclusion of this article will be made available by the authors, without undue reservation.
Author contributions
Conceptualization, JX; Methodology, JX; Software, JW; Validation, JX; Formal Analysis, JX and HW; Resources, HW; Data Curation, JW; Writing—Original Draft Preparation, JX; Writing—Review and Editing, JW and CL; Funding Acquisition, CL. All authors have read and agreed to the published version of the manuscript.
Funding
This work was funded by the National Natural Science Foundation of China (The effect of infant milk powder safety trust index on product competitiveness—Index measurement, Correlation model construction and market simulation, Project number 71673042).
Conflict of interest
The authors declare that the research was conducted in the absence of any commercial or financial relationships that could be construed as a potential conflict of interest.
Publisher’s note
All claims expressed in this article are solely those of the authors and do not necessarily represent those of their affiliated organizations, or those of the publisher, the editors and the reviewers. Any product that may be evaluated in this article, or claim that may be made by its manufacturer, is not guaranteed or endorsed by the publisher.
Footnotes
1From http://www.gov.cn/zhengce/2020-11/03/content_5556991.htm which is reported by the Chinese government.
2From http://www.cnfia.cn/archives/16712 which is reported by the China National Food Industry Association.
3From http://www.moa.gov.cn/which is reported by the Ministry of Agriculture and Rural Affairs of China.
4From https://www.sohu.com/a/154658984_648170.
5From https://www.sohu.com/a/397670382_120608855?_trans_=000014_bdss_dkmwzacjP3p:CP=
6From http://www.stats.gov.cn/tjsj/tjgb/qttjgb/qgqttjgb/201002/t20100211_30641.html which is reported by the National Bureau of Statistics of China.
References
Adenuga, A. H., Davis, J., Hutchinson, G., Donnellan, T., and Patton, M. (2018). Modelling regional environmental efficiency differentials of dairy farms on the island of Ireland. Ecol. Indic. 95, 851–861. doi:10.1016/j.ecolind.2018.08.040
Bai, Y., Li, L., Wang, F., Zhang, L., and Xiong, L. (2022). Impact of dairy imports on raw milk production technology progress in China. Int. J. Environ. Res. Public Health 19 (5), 2911. doi:10.3390/ijerph19052911
Baker, A. (2002). Fluorescence properties of some farm wastes: Implications for water quality monitoring. Water Res. 36 (1), 189–195. doi:10.1016/s0043-1354(01)00210-x
Camarero, M., Castillo, J., Picazo-Tadeo, A.J., and Tamarit, C. (2013). Ecoefficiency and convergence in OECD countries Economics. Environ. Resour. Econ. 55 (1), 87–106. doi:10.1007/s10640-012-9616-9
Charnes, A., Cooper, W. W., and Rhodes, E. (1978). Measuring the efficiency of decision making units. Eur. J. operational Res. 2 (6), 429–444. doi:10.1016/0377-2217(78)90138-8
European Commission (2020). Transitional Common Agricultural Policy 2021–2022. Available at: hhttps://ec.europa.eu/commission/presscorner/detail/en/ip_20_2236.
Färe, R., Grosskopf, S., Lovell, C. K., and Pasurka, C. (1989). Multilateral productivity comparisons when some outputs are undesirable: A nonparametric approach. Rev. Econ. statistics 71, 90–98. doi:10.2307/1928055
Färe, R., and Grosskopf, S. (2004). Modeling undesirable factors in efficiency evaluation: Comment. Eur. J. Operational Res. 157 (1), 242–245. doi:10.1016/s0377-2217(03)00191-7
Galanopoulos, K., Aggelopoulos, S., Kamenidou, I., and Mattas, K. (2006). Assessing the effects of managerial and production practices on the efficiency of commercial pig farming. Agric. Syst. 88 (2-3), 125–141. doi:10.1016/j.agsy.2005.03.002
Guo, S., Qian, Y., Nie, R., and Shi, H. (2019). Environmental efficiency evaluation and convergence analysis of provinces along “the belt and road” based on SBM-undesirable model. Ekoloji 28 (107), 2405–2415.
Hadri, K. (2000). Testing for stationarity in heterogeneous panel data. Econ. J. 3 (2), 148–161. doi:10.1111/1368-423x.00043
Hailu, A., and Veeman, T. S. (2001). Non-parametric productivity analysis with undesirable outputs: An application to the Canadian pulp and paper industry. Am. J. Agric. Econ. 83 (3), 605–616. doi:10.1111/0002-9092.00181
Kounetas, K. E., Polemis, M. L., and Tzeremes, N. G. (2021). Measurement of eco-efficiency and convergence: Evidence from a non-parametric frontier analysis. Eur. J. Operational Res. 291 (1), 365–378. doi:10.1016/j.ejor.2020.09.024
Kuhn, L., Balezentis, T., Hou, L., and Wang, D. (2020). Technical and environmental efficiency of livestock farms in China: A slacks-based DEA approach. China Econ. Rev. 62, 101213. doi:10.1016/j.chieco.2018.08.009
Le, T.-L., Luu, T.-T.-H., Huynh, N., and Chung, R. H. (2020). Environmental efficiency of rice production in Vietnam: An application of SBM-DEA with undesirable output. Biodiversitas 21 (6). doi:10.13057/biodiv/d210645
Li, T., Baležentis, T., Makutėnienė, D., Streimikiene, D., and Kriščiukaitienė, I. (2016). Energy-related CO2 emission in European Union agriculture: Driving forces and possibilities for reduction. Appl. Energy 180, 682–694. doi:10.1016/j.apenergy.2016.08.031
Njuki, E., Bravo-Ureta, B. E., and Mukherjee, D. (2016). The good and the bad: Environmental efficiency in northeastern US dairy farming. Agric. Resour. Econ. Rev. 45 (1), 22–43. doi:10.1017/age.2016.1
Phillips, P. C., and Sul, D. (2007). Transition modeling and econometric convergence tests. Econometrica 75 (6), 1771–1855. doi:10.1111/j.1468-0262.2007.00811.x
Rehman, A., Deyuan, Z., and Chandio, A. A. (2019). Contribution of beef, mutton, and poultry meat production to the agricultural gross domestic product of Pakistan using an autoregressive distributed lag bounds testing approach. Sage Open 9 (3), 215824401987719. doi:10.1177/2158244019877196
Rehman, A., Jingdong, L., Chandio, A. A., and Hussain, I. (2017). Livestock production and population census in Pakistan: Determining their relationship with agricultural GDP using econometric analysis. Inf. Process. Agric. 4 (2), 168–177. doi:10.1016/j.inpa.2017.03.002
Rehman, A., Ulucak, R., Murshed, M., Ma, H., and Işık, C. (2021). Carbonization and atmospheric pollution in China: The asymmetric impacts of forests, livestock production, and economic progress on CO2 emissions. J. Environ. Manag. 294, 113059. doi:10.1016/j.jenvman.2021.113059
Reinhard, S., Lovell, C. K., and Thijssen, G. J. (1999). Econometric estimation of technical and environmental efficiency: an application to Dutch dairy farms. Am. J. Agric. Econ. 81 (1), 44–60. doi:10.2307/1244449
Reinhard, S., Lovell, C. K., and Thijssen, G. J. (2000). Environmental efficiency with multiple environmentally detrimental variables; estimated with SFA and DEA. Eur. J. Operational Res. 121 (2), 287–303. doi:10.1016/s0377-2217(99)00218-0
Scheel, H. (2001). Undesirable outputs in efficiency valuations. Eur. J. operational Res. 132 (2), 400–410. doi:10.1016/s0377-2217(00)00160-0
Solow, R. M. (1956). A contribution to the theory of economic growth. Q. J. Econ. 70 (1), 65–94. doi:10.2307/1884513
Soteriades, A. D., Faverdin, P., March, M., and Stott, A. W. (2015). Improving efficiency assessments using additive data envelopment analysis models: An application to contrasting dairy farming systems. AFSci. 24 (3), 235–248. doi:10.23986/afsci.49446
Sun, H., Kporsu, A. K., Taghizadeh-Hesary, F., and Edziah, B. K. (2020). Estimating environmental efficiency and convergence: 1980 to 2016. Energy 208. doi:10.1016/j.energy.2020.118224
Tone, K. (2001). A slacks-based measure of efficiency in data envelopment analysis. Eur. J. operational Res. 130 (3), 498–509. doi:10.1016/s0377-2217(99)00407-5
Tone, K., and Tsutsui, M. (2010). Dynamic DEA: A slacks-based measure approach. Omega 38 (3-4), 145–156. doi:10.1016/j.omega.2009.07.003
Wang, X., Wu, X., Yan, P., Gao, W., Chen, Y., and Sui, P. (2016). Integrated analysis on economic and environmental consequences of livestock husbandry on different scale in China. J. Clean. Prod. 119, 1–12. doi:10.1016/j.jclepro.2016.01.084
Yan, Z., Wang, C., and Liu, T. (2020). An analysis of the environmental efficiency of pig farms and its determinants-a field study from China. Environ. Sci. Pollut. Res. 27 (30), 38084–38093. doi:10.1007/s11356-020-09922-7
Yao, C., Qian, S., Li, Z., and Liang, L. (2017). Provincial animal husbandry carbon emissions in China and temporal-spatial evolution mechanism. Res. Sci. 39, 698–712. doi:10.18402/resci.2017.04.11
Yu, X.Associate (2012). Productivity, efficiency and structural problems in Chinese dairy farms. China Agric. Econ. Rev. 4 (2), 168–175. doi:10.1108/17561371211224755
Zheng, J., Liu, X., and Bigsten, A. (1998). Ownership structure and determinants of technical efficiency: An application of data envelopment analysis to Chinese enterprises (1986–1990). J. Comp. Econ. 26 (3), 465–484. doi:10.1006/jcec.1998.1540
Keywords: environmental efficiency, dairy farming, scale difference, club convergence, logt test
Citation: Xu J, Wang J, Wang H and Li C (2022) Evolution trend and promotion potential of environmental efficiency of dairy farming in china from the perspective of “club convergence”. Front. Environ. Sci. 10:967150. doi: 10.3389/fenvs.2022.967150
Received: 12 June 2022; Accepted: 25 July 2022;
Published: 16 August 2022.
Edited by:
Faik Bilgili, Erciyes University, TurkeyReviewed by:
Fangrong Ren, Nanjing Forestry University, ChinaXin Zhao, Anhui University of Finance and Economics, China
Copyright © 2022 Xu, Wang, Wang and Li. This is an open-access article distributed under the terms of the Creative Commons Attribution License (CC BY). The use, distribution or reproduction in other forums is permitted, provided the original author(s) and the copyright owner(s) are credited and that the original publication in this journal is cited, in accordance with accepted academic practice. No use, distribution or reproduction is permitted which does not comply with these terms.
*Correspondence: Cuixia Li, bGljdWl4aWEuODgzQDE2My5jb20=
†These authors have contributed equally to this work and share first authorship