- 1Institute of Geographic Sciences and Natural Resources Research, Chinese Academy of Sciences, Beijing, China
- 2College of Resources and Environment, University of Chinese Academy of Sciences, Beijing, China
- 3Key Laboratory of Carrying Capacity Assessment for Resource and Environment, Ministry of Natural Resources, Beijing, China
The Chinese inland basins (CIBs) are vulnerable to global warming and human activities due to low precipitation and high potential evaporation. Terrestrial water storage (TWS) is an important component of the hydrological cycle and essential for evaluating the water resource security of the CIBs. Although some studies have focused on water storage trends in sub-basins of the CIBs, only few studies have analyzed water storage trends in the CIBs as a whole. In this study, trends and magnitudes of precipitation, TWS, and actual evapotranspiration (AET) were detected by the rank-based non-parametric Mann–Kendall test and trend magnitude method. Based on the hydrological budget of the closed inland basin, the monthly series of AET were simulated and the main factors affecting TWS changes in the CIBs and each closed basin were identified. Results showed that both precipitation and AET significantly increased in the CIBs. Precipitation decreased from the northwest and southeast regions to the central region in the CIBs. Moreover, the annual TWS in the CIBs significantly decreased mainly due to the increased AET. Approximately 60% AET increase was attributed to increased irrigation diversions. At the basin scale, similar to the CIBs, changes in AET were the predominant factor influencing changes in TWS in the Tarim basin (TRB), Turpan basin (TPB), and Hexi Corridor basin (HCB). Qiangtang Plateau basin (QPB) Qaidam basin (QDB) the increase in precipitation contributed more than 60% increase in TWS glacier melting and irrigation diversion.
1 Introduction
Terrestrial water storage (TWS), which includes snow water storage, canopy water storage, surface water storage, soil moisture storage, and groundwater storage, comprehensively reflects regional precipitation, runoff, evapotranspiration, groundwater, and human activities (Scanlon et al., 2018). It is an important variable for global hydrological cycle observation (Liu et al., 2016; Deng and Chen, 2017). Although TWS accounts for only 3.5% water in the global hydrological cycle, it is an important component of the terrestrial and global water cycle that provides important control over water, energy, and biotic ecosystem processes (Hu et al., 2018; Chen et al., 2022), and thus plays an important role in the global climate system (Bierkens, 2015). TWS and their components control various hydrometeorological, ecological, and biogeochemical processes on different spatial and temporal scales (Koster et al., 2004; Seneviratne et al., 2010; Dong et al., 2022), and thus there is great spatial and temporal heterogeneity in the global scale (Güntner et al., 2007; Güntner, 2008).
In the past decades, extreme climatic events (such as droughts and floods) and human activities (such as groundwater extraction) have influenced TWS beyond normal thresholds on a global scale. For example, TWS has significant decreased due to groundwater over-exploitation in Illinois (Yeh et al., 2006), the Central Valley (Famiglietti et al., 2011; Scanlon et al., 2012; He et al., 2017), northern India (Xiang et al., 2016), the Middle East (Voss et al., 2013), and northern China (Feng et al., 2013). In addition, severe droughts in the southern U.S. High Plains (Scanlon et al., 2012), eastern Texas (Long et al., 2013), Brazil (Getirana, 2016), and southeastern and northern Africa (Ramillien et al., 2014; Rodell et al., 2018) have significantly decreased TWS, particularly groundwater storage. On the contrary, TWS has also increased at other regions, including the Qaidam basin (QDB) (Bibi et al., 2019; Meng et al., 2019) and Qiangtang Plateau basin (QPB) (Liu et al., 2019; Meng et al., 2019) in the Chinese inland basins (CIBs). However, only few studies have examined the attributes of the underlying factors driving these trends.
Remote sensing products, such as gravity recovery and climate experiment (GRACE) satellite data, are widely used to assess global hydrology. GRACE satellites have been likened to giant weighing scales in the sky that monitor monthly changes in mass as water storage increases or decreases due to climate variability and human impacts (Scanlon et al., 2018). GRACE satellites provide global total water storage anomaly (TWSA) data since their launch in 2002. These satellites provide a more direct estimate than global TWSA change models derived from monitoring the time variable gravity field (Wahr et al., 2004). The coarse spatial resolution of GRACE data (∼100,000 km2) may actually be beneficial when estimating changes in TWSA at continental to global scales.
Inland basins, also referred to as endorheic basins, are defined as regions where runoff in the basin has no direct hydraulic connection with the ocean (Liu, 2022). Thus, inland river basin runoff is isolated from the ocean and eventually enters inland lakes or is absorbed by evapotranspiration. These areas are among the most sensitive to climate change and human activities (Huang et al., 2016; Wang et al., 2018). The CIBs are located in the hinterland of Asia. Accurately monitoring the TWSA changes of a large area is difficult by traditional stations. GRACE satellite monitoring becomes a feasible solution in this case. Since the CIBs have temperate continental climate characterized by low precipitation and high evapotranspiration, the hydrology and ecosystem of CIBs are sensitive to changes in precipitation, actual evapotranspiration (AET), and TWSA. Therefore, analysis of terrestrial TWSA variability and attribution in the CIBs is important for water resource management, ecosystem health, and sustainable irrigated agriculture in China. However, few studies have focused on the variation and attribution of CIBs as a whole. In addition, there were some inconsistent conclusions on the TWSA trends in CIB sub-basins. Wang et al. (2020) reported that TWSA decreased during 2002–2016 in the Hexi Corridor basin (HCB) using one TWSA product. Cao et al. (2018) found that the basin TWSA significantly increased during 2002–2013 using another TWSA product. Thus, opposite results might be detected from different datasets. This study used multiple GRACE datasets to reduce the uncertainty caused by using only a single dataset. The hydrological budget (hydrologic gains and losses) is effective for analysis at the basin scale (Liu et al., 2014; Liu et al., 2016). Unlike outflow basins, which include AET and runoff in hydrologic losses, inland river basins include only AET. In other words, the hydrologic budget in inland river basins can only be expressed in terms of precipitation, AET, and TWSA. Therefore, this method is more suitable for analyzing the TWSA variability and its main attribution in inland river basins.
This study uses multi-source of data in the CIBs and each closed basin to simulate the monthly AET series of each inland basin using the hydrologic budget method, detect the spatiotemporal characteristics of annual and monthly precipitation, TWSA, and AET in each basin using a non-parametric test, and identify the main attributes of TWSA change in each closed basin using the water balance principle. The results obtained in this study could be useful to regional water resource management, ecosystem health, and sustainable agricultural irrigation in China.
2 Study area
The CIBs are located in northwest China (Figure 1), covering 2.61 × 1011 km2 area, accounting for 27% Chinese land area; however, it takes up only 5.5% total water resource of China. It is one of the most arid regions worldwide and includes five closed inland basins: the Turpan basin (TPB), QDB, QPB, HCB, and Tarim basin (TRB) (Figure 1). The terrain within the basin is complex. The landform is mainly plateau and inland basin. The CIBs are affected by the plateau monsoon and East Asian monsoon climate systems. The climate is complex and changeable. Except in Yili and Tacheng in northern CIBs, annual precipitation in most areas is less than 200 mm. The annual precipitation in the CIBs is 164.5 mm. The annual precipitation of TPB, QDB, QPB, HCB, and HCB are 86.2, 147.8, 264.8, 153.3, and 112.9 mm, respectively.
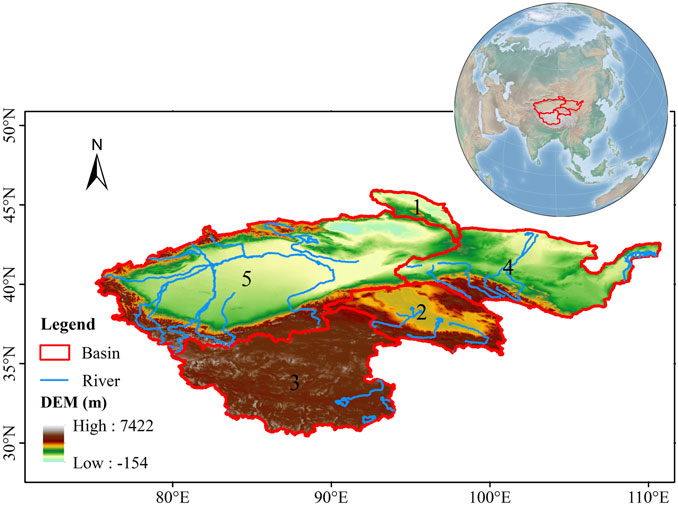
FIGURE 1. Location and distribution of each basin in the Chinese inland basins (CIBs). 1–5 represents the Turpan basin (TPB), Qaidam basin (QDB), Qiangtang Plateau basin (QPB), Hexi Corridor basin (HCB), and Tarim basin (TRB), respectively.
3 Materials and methods
3.1 Gravity recovery and climate experiment data
3.1.1 The RL06 spherical harmonics
The GRACE satellites, launched by National Aeronautics and Space Administration (NASA) and Deutsches Zentrum für Luft- und Raumfahrt in March 2002, allows us to measure large scale TWSA trends with monthly temporal resolution. Temporal variations in the gravity field over land are primarily due to TWSA, which is the vertically integrated measure of groundwater, soil moisture, snow, ice, and surface water.
This study uses data from March 2002 to February 2021 (39 months of data are missing). The gridded GRACE TWSA data were obtained from the Center for Space Research at the University of Texas (CSR), the Geo-Forschungs-Zentrum in Potsdam (GFZ), and the Jet Propulsion Laboratory (JPL).
The spatial resolution of all three datasets is 1°. More than 300 grid points covering the study area were chosen for estimating the values. To reduce uncertainty, the missing data were obtained via simple temporal interpolation using adjacent data (Andrew et al., 2017).
3.1.2 The global mascons
Mass concentration blocks (mascons) are another form of gravity field basis function. Compared to the standard spherical harmonic method of empirical post-processing filtering, “mascons” eases the implementation of geophysical constraints and are a more rigorous method (Watkins et al., 2015; Wiese et al., 2016). The JPL mascons (JPLM) and CSR mascons (CSRM) solutions are available at 0.5° and 0.25°. In this study, the GRACE TWSA solution was compared for the period from 2002 to 2020.
3.2 Meteorological data
This study estimated the precipitation trend in the CIBs from 2002 to 2020 using the China Monthly Surface Precipitation 0.5°×0.5° Gridded Dataset V2.0 (http://data.cma.cn/), which is a gridded dataset released by the National Meteorological Information Center of the China Meteorological Administration. This dataset was derived by interpolating the observed precipitation data from 2,472 stations over China since 1961. Owing to the high quality of this dataset, it has been widely used in the CIBs (Zhu et al., 2015; Bueh et al., 2016; Zhang et al., 2017; Wang et al., 2019).
3.3 Methodology
3.3.1 Monthly actual evapotranspiration simulation
According to the hydrological budget in an inland basin, the difference between precipitation and AET corresponds to the variation in water storage in the basin; therefore, the monthly AET in the basin can be simulated as (Liu, 2022):
Where AETi, Pi, and Si are the monthly series (in mm) of AET, precipitation, and storage change, respectively. The average gridded precipitation and TWSA data series for each closed basin was calculated based on each grid area weight within the basin. The area weighting of the boundary grids was shown by the proportion of the area within the basin boundary.
The consistency of the time series for each variable should be considered while simulating monthly AET. Monthly AET and precipitation are averaged within a month, calculating between the beginning and end of the month. ∆S is the difference between the water storage at the end and beginning of the month. However, the TWSA data used in this study represent the average water storage within a month. ∆S was calculated as:
where TSWAi+1 and TWSAi−1 represent water storage at the end and beginning of the simulated month, respectively. The accuracy of this calculation has been verified by Long et al. (2014).
3.3.2 Time series decomposition
The TWSA outputs reveal the monthly variations of total water storage; therefore, seasonality should be first removed from the original TWSA series to estimate TWSA trends (Andrew et al., 2017). Precipitation and evapotranspiration data should be processed similarly. The seasonal trend decomposition uses the local regression to decompose the time series was proposed by Cleveland and Cleveland (1990). In this study, local regression was used to decompose the TWSA monthly time series. Local regression smoothing is the key of the local regression method. It fits the smoothed series Xj to the input time series Xj = Xtj, where tj is the discrete sampling time series. The smoothed value for each point j is given by the value of the polynomial at time tj increased by j. The local regression consists of an outer and inner loop with a sequence of smoothing operators and generates three components from the time series:
where Stotal indicates the original signal, Slong-term is the trend signal, Sseasonal is the seasonal signal, and Sresidual is indicated as the sub-seasonal signal and noise.
3.3.3 Trend detection and identification of its main attribution methods
The Mann–Kendall (M–K) test, one of the commonly used time series trend tests in meteorology and hydrology (Hirsch et al., 1982), applied for trend detection in this study. It also rejects a few outliers and the sample does not need to follow a certain distribution and is suitable for non-normally distributed data (Bibi et al., 2019).
Based on the hydrological budget in inland closed basins, the main factors causing AET and TWSA variations were identified. Based on the water source consumed by the AET, AET variation is attributed to alterations in precipitation and the consumption of other water supply sources. It can be calculated as follows (Liu, 2022):
Where ΔAET, ΔP, and ΔOthers indicate changes in AET, precipitation, and other water supply sources (such as irrigation from ground water and glacial melt water), respectively.
Based on the hydrological budget within a closed basin, changes in TWSA are mainly attributable to precipitation and AET, while changes in AET are mainly caused by precipitation and potential evaporation. Precipitation and potential evaporation positively and negatively contribute to AET, respectively. Furthermore, precipitation and AET positively and negatively affect TWSA, respectively. Increased precipitation will promote an increase in TWSA, whereas increased AET will exacerbate the decrease in TWSA, and vice versa. Thus, the contribution of precipitation and other factors to TWSA changes can be semi-quantified by analyzing the trend between AET and precipitation. The study roadmap for the entire study is shown in Figure 2.
4 Results
4.1 Trends and spatial distribution of terrestrial water storage anomalies in the Chinese inland basins
The TWSA time series in CIBs derived from five GRACE solutions are highly consistent according to Figure 3A. Overall, the TWSA decreased significantly. Specifically, the TWSA increased during 2002–2005, continuously declined from 2005 to 2010, slightly increased from 2010 to 2012, then decreased during 2012–2016, further rising in a fluctuation way. Across the entire time scale, the TWSA of CIBs decreased significantly by 26 km3/yr.
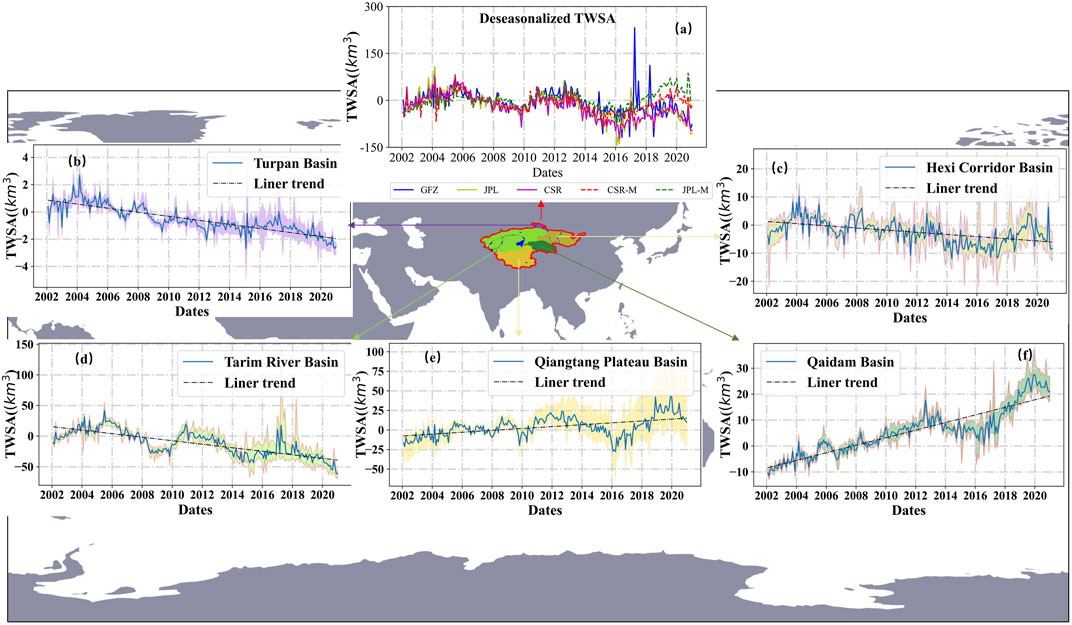
FIGURE 3. Total water storage anomaly (TWSA) trends and Gravity recovery and climate experiment (GRACE) product trend ranges in the Chinese inland basins (CIBs) and its sub-basins. (A) The comparison of deseasonalized TWSA in the CIBs; (B–F) the TWSA trend range in the Turpan Basin, Hexi Corridor Basin, Tarim Basin, Qiangtang Basin, and Qaidam Basin, respectively. The black dashed lines indicate their linear trends.
Figures 3B–F shows the monthly series of TWSA in each sub-basin, obtained from different GRACE solutions. The TWSA significantly increased in the QDB and QPB, while it significantly decreased in other sub-basins. Specifically, the TWSA of the TPB decreased with 0.15 km3/yr fluctuations throughout the time scale. The TWSA of the HCB briefly increased from 2002 to 2004, declined from 2004 to 2007, and then continuously fluctuated from 2007 to 2020. Overall, the TWSA of HCB decreased at 0.4 km3/yr throughout the time scale. TWSA trend in the TRB is similar to that in the CIBs, with TWSA decreasing at 2.9 km3/yr over the entire time scale. The TWSA trends for QPB and QDB significantly fluctuated from 2002 to 2020, with 1.2 and 1.5 km3/yr growth rates, respectively.
Figure 4 shows the spatial distribution of TWSA trends derived from different GRACE solutions. The spatial distribution of the five solutions were highly consistent. It showed a significant loss of water storage at the northwestern and southwestern CIB regions during the study period. Water storage significantly increased in the central and southeastern CIB regions. According to the sub-basins, TWSA significantly increased in the northeastern QPB and the entire QDB, while it significantly decreased in the TPB and the HCB. Moreover, the JPLM solution-produced TWSA series deviated from the other four solution-produced TWSAs in the CIBs (Figure 4). This finding is also consistent with previous finding that decreasing and increasing TWSA trends of JPLM solution are larger than those of CSR, JPL, and GFZ globally (Scanlon et al., 2018).
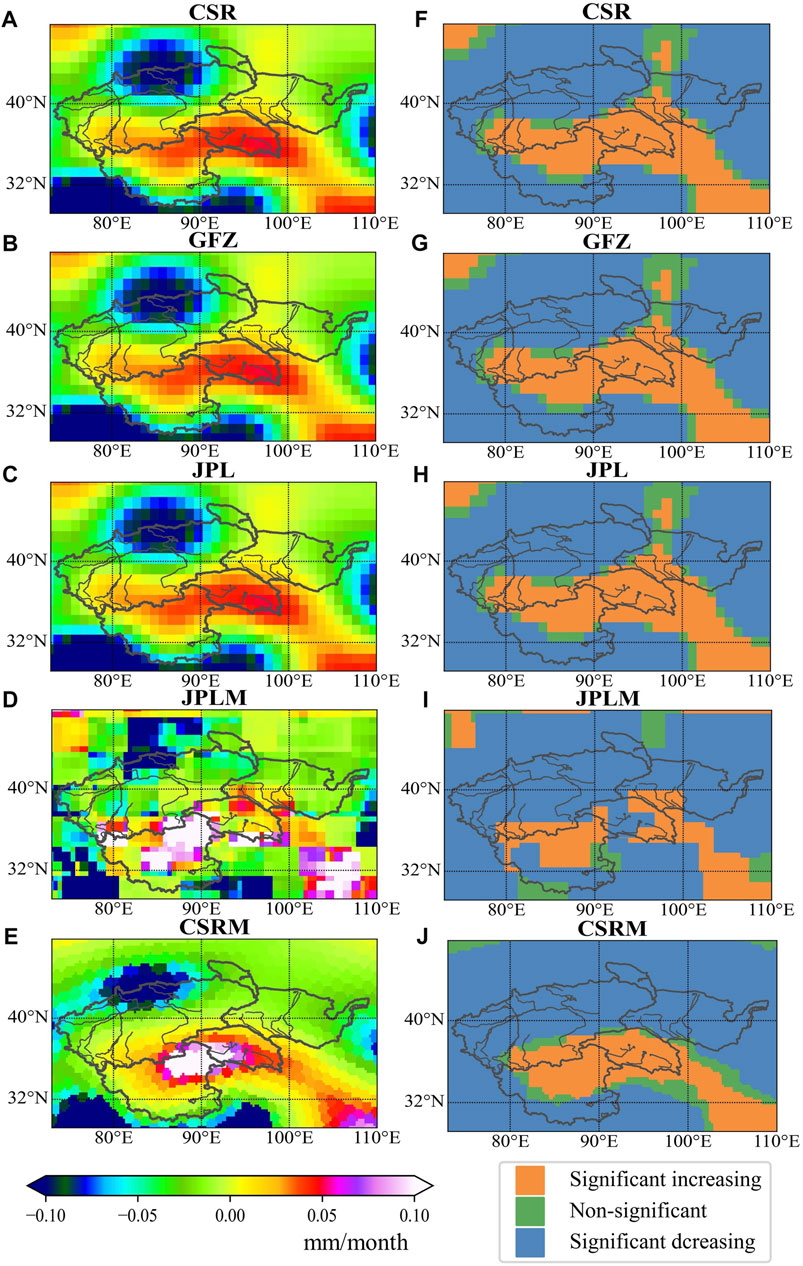
FIGURE 4. The spatial pattern of total water storage anomaly (TWSA) trends (left) and significant (right) derived from different Gravity recovery and climate experiment (GRACE) solution: TWSA trends and significant are calculated using M–K. (A,F) indicate the GRACE resolution of RL06 spherical harmonics from the Center for Space Research at the University of Texas (CSR). (B,G) indicate the GRACE resolution of RL06 spherical harmonics from the Geo-Forschungs-Zentrum in Potsdam (GFZ). (C,H) indicate the GRACE resolution of RL06 spherical harmonics from the Jet Propulsion Laboratory (JPL). (D,I) indicate the GRACE resolution of mass concentration blocks (mascons) from the JPL. (E,J) indicate the GRACE resolution of mass concentration blocks (mascons) from the CSR.
Viewing the time change of the TWSA in the CIBs revealed that the similarity between the five GRACE solutions started to decrease from 2016 (Figure 3A). The periodic change of TWSA in sub-basins also indicated that TWSA fluctuation increased in the five basins in 2016 for the five solutions (Figures 3B–F). Therefore, the TWSA of GRACE were calculated according to the time for data variance and median changes (Figure 5). From 2002 to 2007, the median GRACE data continued to decline, while the variance increased and then decreased. The median GRACE data began to rise but did not rise above the original median from 2007 to 2011. The variance change did not change much from the previous time period. The median GRACE data began to fluctuate around the initial median line from 2012 to 2016; however, the overall variance was low. The median GRACE data suddenly increased and reached the maximum in 2017. Simultaneously, the variance reached the maximum. Then, the median data continuously decreased from 2018 to 2020. The median GRACE data was lower than the initial median value since 2019, and the variance decreased gradually.
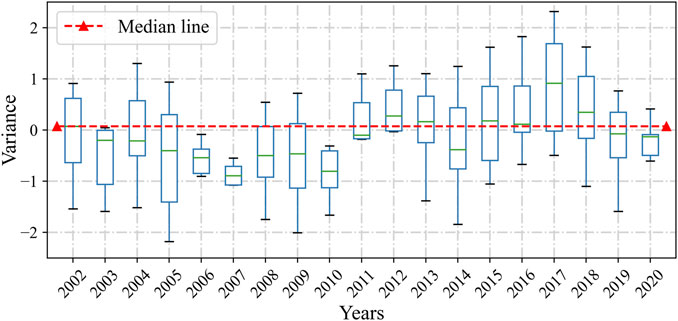
FIGURE 5. Trends of Gravity recovery and climate experiment (GRACE) total water storage anomaly (TWSA) data by time variance and median. Red line indicated median of initial month data.
4.2 Trends and spatial distribution of precipitation and actual evapotranspiration simulation in the Chinese inland basins
Figure 6 indicates the spatial distribution of precipitation trends in the CIBs from 2002 to 2020. Macroscopically, precipitation increased in the northeast, northwest and southeast edges of the CIBs, while it decreased in the inner regions of the basin.
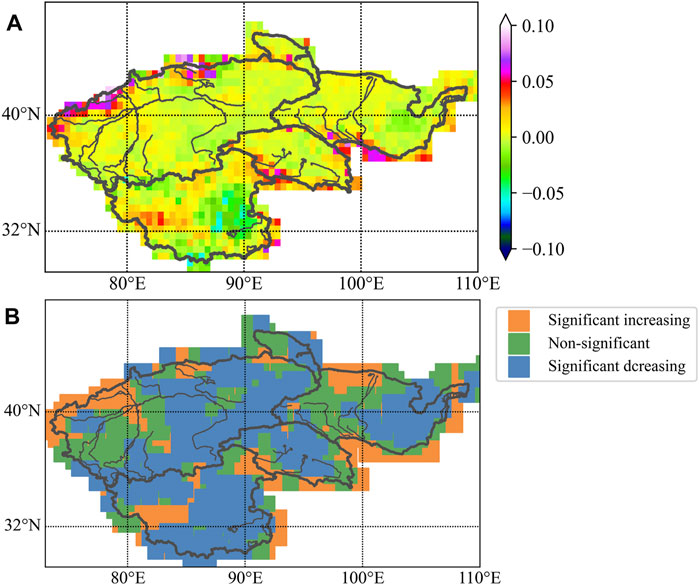
FIGURE 6. The spatial pattern of (A) M–K trends and precipitation significance (B) in the Chinese inland basins (CIBs).
Precipitation significantly increased in the northern part of the HCB, the eastern edge of the southwestern margin of the QDB, the central-eastern part of the QPB, the central-western and northwestern marginal regions of the TRB, and the southwestern part of the TPB; however, it significantly decreased in the central-eastern part of the HCB, the central and southwestern marginal regions of the QPB, the central and southeastern part of TRB, and the eastern marginal regions of the TPB. Furthermore, it did not change significantly in the remaining regions.
Overall, the annual precipitation significantly increased in the CIBs (11.1 mm/10a), as well as all sub-basins except the QPB (4.5 mm/10a). The increase was the highest in the HCB (9.7 mm/10a). The TRB, QDB, HCB, QPB, and TPB contributed approximately 40%, 30%, 20%, 8%, and 2% precipitation, respectively.
Monthly AETs were simulated using precipitation data and average monthly series of five GRACE products according to the methodology of the hydrological budget (Figure 7). Overall, the annual AET significantly increased in the CIBs (20.3 mm/10a), as well as all sub-basins except the QPB (3.6 mm/10a) and QDB (21 mm/10a). The increase was the highest in the HCB (29.5 mm/10a). Since the increase in precipitation is less than that in AET, combined with the principle of water balance, precipitation in the CIBs was insufficient to meet the demand from the AET during this period.
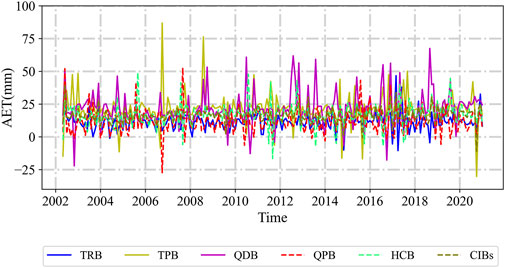
FIGURE 7. The deseasonalized series of actual evapotranspiration (AET) in the Chinese inland basins (CIBs) and each sub-basin. The results were calculated based on water balance combined with five Gravity recovery and climate experiment (GRACE) solutions and precipitation data. TRB, TPB, QDB, QPB, and HCB indicates Tarim Basin, Turpan Basin, Qaidam Basin, Qiangtang Basin, and Hexi Corridor Basin, respectively.
4.3 The main attribution of the terrestrial water storage anomalies and actual evapotranspiration trends in the Chinese inland basins
Figure 8 shows the TWSA, precipitation and AET M–K significance and trends for the whole CIBs and each closed basin. This study reports that the precipitation and TWSA of the whole CIBs significantly increased and decreased, respectively (9 and 11 mm/10a, respectively). Based on the water balance of the closed basin, quantifying the magnitude of the changes in precipitation and TWSA showed that the decreased TWSA of the whole CIBs was mainly due to AET increase. The main factors affecting changes in AET changes were analyzed based on the attribution identification method described in Section 3.3.3. Additionally, the contribution of precipitation and other water sources to changes in the AET were semi-quantified by comparing the trend magnitudes of AET and precipitation. The increase in precipitation in the CIB accounted for 40% AET, indicating that the increasing consumption of other water sources was the dominant factor for increasing the AET in the CIB. This increased consumption, which might be mainly due to irrigation diversion from ground water and glacial melt runoff, explained 60% AET increase. This increased consumption may be due to a combination of mechanisms including increased evaporation rates due to rising temperatures and increased irrigation diversion from ground water and glacial melt runoff, thus increasing AET. Interestingly, 60% AET increase was due to the increased precipitation and the rest might be influenced by the glacial melting and river irrigation in the basin.
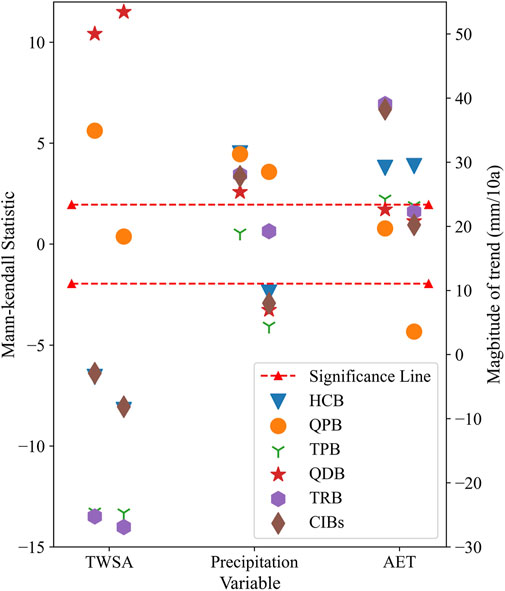
FIGURE 8. Significance (left) and trend (right) of total water storage anomaly (TWSA), precipitation and actual evapotranspiration (AET) M–K in the Chinese inland basins (CIBs) and each closed basin. The left side indicates the significance statistic obtained after M–K calculation, the right side indicates the magnitude of change obtained from M–K calculation. The red dashed line indicates the cut-off line of significance. TRB, TPB, QDB, QPB, and HCB indicates Tarim Basin, Turpan Basin, Qaidam Basin, Qiangtang Basin, and Hexi Corridor Basin, respectively.
The changes in AET primarily influenced the changes in TWSA in each closed basin, similar to that in the CIBs. Among them, the increased AET of the HCB, TRB, and TPB primarily decreases the TWSA in the basin. Precipitation remains the main reason for the increased AET in HCB (>60%). In the TRB and TPB, the proportion of precipitation increase contributing to AET increase was <20%. This indicates that increased precipitation had made a relatively small contribution to the AET increase. The glacier melting and irrigation diversion caused by enhanced evaporation rates played a dominant role in AET increase. In the QPB and QDB, AET reduction in the basins increased TWSA. More than 50% AET decrease in the QDB is caused by precipitation. This indicates that increased precipitation had made a relatively small contribution to the AET decrease. The decrease in the consumption of other water sources caused by weakened evaporation rates played a dominant role in AET decrease.
5 Discussion
5.1 Comparison with previous study results
Although few studies have focused on the whole CIBs, some studies have focused on the attribution of TWSA changes in the CIB sub-basins. These results were verified with those of this study.
The significantly increased TWSA in the QDB and QPB was verified with the results of previous studies (Bibi et al., 2019; Liu et al., 2019; Meng et al., 2019). However, the main reasons attributed to this trend differed from those identified in previous studies. Previous studies believed that the TWSA increased in QDB primarily due to the increase of precipitation, while the TWSA of QPB was dominated by decreased AET. In this study, TWSA increase in the QDB is mainly due to the decrease of AET, while the increase of TWS in the QPB is mainly due to the increase of AET. One of the reasons for the different results is that previous studies analyzed relatively short TWSA time series (5–8 years), while this study focused on the whole 19-year time series analysis.
The significantly decreased TWSA in the TRB has also been confirmed by previous studies (Wang et al., 2021; Zuo et al., 2021). Increased AET is also the main factor influencing changes in the TWSA. However, previous studies have considered that temperature is the main reason for the increase in AET, while the increase of AET in this study was mainly due to the increase of water consumption caused by human activities and glacier retreat. Human activities were considered in this study as an factor affecting AET.
5.2 Data uncertainty analyses
Due to the lack of actual measurement data for validation, determining the product more suitable for the CIBs is difficult; however, the correlation coefficient between the time series of the five data sets in the first 15 years is >0.87 (Figure 3A). Moreover, the five data sets are consistent according to the spatial distribution map (Figure 4). Interestingly, differences between the solutions were detected when processing the five GRACE solutions (Figure 4). There was large data fluctuation in different basins around 2017 (Figure 3). The following attempts were made to explain this phenomenon.
For the differences of the five solutions, the overall performance of the five solutions in the five basins was first analyzed, and the variance of the tertiary solutions processed by the first three institutions was statistically significantly smaller than that of the last two mascons. Considering that the spherical harmonic coefficient method removes some real geophysical signals when smoothing through the empirical smoothing function while processing the noise of the GRACE data signal, the entire amplitude and variance of the data will be smaller than that of the mascons method, which applies a priori knowledge (Zhang et al., 2019). This can be corresponded to the results in Figure 4, where the results of the first three products solved by the spherical harmonic coefficient method are similar. The range of trend of the last two products is significantly larger than that of the first three. Therefore, using these two GRACE solutions to integrate the analysis will not only increase the overall information, but also cause errors in the final analysis results.
The GRACE satellite started functioning in April 2002 (Figure 5). The median value of that year was used as the starting reference and data stability is increasing over time. From 2012 to 2016, the median data fluctuated in the initial median line, probably because the GRACE satellite data was more volatile than those of the previous years as the satellite service life increased. The satellite data quality fluctuation increased and reached the current maximum in 2017 because the satellite terminated the data in June 2017. Then, the GRACE-FO satellite took over the GRACE satellite mission and continued to transmit data from June 2018. The median data value in 2018 was slightly higher than the initial median value, but the median data value immediately dropped below the initial median value in the following 2 years, indicating that the quality of GRACE-FO satellite data has been improving except for the first year.
Although the average sequence of five TWSA was used in this study to reduce the uncertainty caused by a single solution, studies on water resources research should consider a depth study of the inversion of TWSA of GRACE. When simulating AET monthly series, ΔS indicates the change of monthly water storage, that is, the difference of water storage between the end and beginning of the month. However, the TWSA of GRACE is the intra-month water storage. In this study, the difference between the TWSA of the previous month and the next month is used to represent the monthly water storage variability within the basin, which increases the uncertainty of the experiment to some extent.
6 Conclusion
Trends of annual and monthly precipitation, TWSA, and AET were detected at each basin by the M–K test. The main attribution of TWSA variability for the CIBs and each of its closed basins was identified.
Annual precipitation in the CIBs increased from 2002 to 2020. It generally increased at the eastern, northwestern and southeastern regions of the CIBs, while decreasing in the central regions of the basin. It also increased in each sub-basin. The increase was significant in all sub-basins except the QPB. The magnitude of increase was highest in the HCB. The TRB received the most precipitation with a weight of 40%, while the TPB received the least.
The TWSA and AET showed significantly decreased and increased in the CIBs and most of its sub-basins, respectively. TWSA increased in the QPB and QDB. TWSA decreased in the CIBs primarily due to the increased AET. Precipitation caused more than 50% increased AET. Similar to that in the CIBs, AET change was the main factor driving TWSA change in each closed basin. In particular, the increased AET in the HCB, TRB, and TPB was the main factor for the decreased TWSA in these basins. The increased AET in the HCB was mainly due to precipitation. However, melting glaciers and irrigation diversion primarily caused the increased AET in the TRB and TPB. Interestingly, AET reduction in the QPB and QDB decreased TWSA. More than 50% AET reduction in the QDB was caused by precipitation, while more than 70% AET reduction in the QPB was caused by the decreased consumption of other water resources.
Data availability statement
Publicly available datasets were analyzed in this study. This data can be found here: https://grace.jpl.nasa.gov/data/get-data/monthly-mass-grids-land/, http://data.cma.cn/.
Author contributions
XL conceived and designed the concept, reviewed the literature, collected data, and wrote the manuscript. ZL conceived the concept, edited the article, and supervised the study. HW helped to receive technical support. All authors have read and agreed to the published version of the manuscript.
Funding
This study was supported and funded by the Second Tibetan Plateau Scientific Expedition and Research Program (2019QZKK1006), the National Natural Science Foundation of China (42171029), and the Strategic Priority Research Program of the Chinese Academy of Sciences (XDA23090302).
Acknowledgments
We thank NASA for their contribution to GRACE data production and the China Meteorological Administration for their support of China’s meteorological data. The GRACE data can be obtained from https://grace.jpl.nasa.gov/data/get-data/monthly-mass-grids-land/. The meteorological data can be obtained from http://data.cma.cn/.We appreciate reviewers and editors for their critical and constructive comments to improve the paper quality.
Conflict of interest
The authors declare that the research was conducted in the absence of any commercial or financial relationships that could be construed as a potential conflict of interest.
Publisher’s note
All claims expressed in this article are solely those of the authors and do not necessarily represent those of their affiliated organizations, or those of the publisher, the editors and the reviewers. Any product that may be evaluated in this article, or claim that may be made by its manufacturer, is not guaranteed or endorsed by the publisher.
Abbreviations
CIBs, Chinese inland basins; GRACE, Gravity recovery and climate experiment; TWS, Terrestrial water storage; TWSA, Terrestrial water storage anomalies; AET, Actual evapotranspiration; TRB, Tarim basin; TPB, Turpan basin; HCB, Hexi Corridor basin; QDB, Qaidam basin; QPB, Qiangtang Plateau basin; NASA, National Aeronautics and Space Administration; CSR, Center for Space Research at the University of Texas; GFZ, Geo-Forschungs-Zentrum in Potsdam; JPL, Jet Propulsion Laboratory; Mascons, Mass concentration blocks; JPLM, JPL mascons; CSRM, CSR mascons.
References
Andrew, R., Guan, H., and Batelaan, O. (2017). Estimation of GRACE water storage components by temporal decomposition. J. Hydrol. X. 552, 341–350. doi:10.1016/j.jhydrol.2017.06.016
Bibi, S., Wang, L., Li, X., Zhang, X., and Chen, D. (2019). Response of groundwater storage and recharge in the Qaidam Basin (Tibetan Plateau) to climate variations from 2002 to 2016. JGR. Atmos. 124, 9918–9934. doi:10.1029/2019JD030411
Bierkens, M. F. P. (2015). Global hydrology 2015: state, trends, and directions. Water Resour. Res. 51, 4923–4947. doi:10.1002/2015WR017173
Bueh, C., Li, Y., Lin, D., and Lian, Y. (2016). Interannual variability of summer rainfall over the northern part of china and the related circulation features. J. Meteorol. Res. 30, 615–630. doi:10.1007/s13351-016-5111-5
Cao, Y., Nan, Z., Cheng, G., and Zhang, L. (2018). Hydrological variability in the arid region of northwest china from 2002 to 2013. Adv. Meteorology 2018, 1–13. doi:10.1155/2018/1502472
Chen, L., Hu, Z., Du, X., Khan, M. Y. A., Li, X., and Wen, J. (2022). An optimality based spatial explicit ecohydrological model at watershed scale: model description and test in a semiarid grassland ecosystem. Front. Environ. Sci. 10, 798336. doi:10.3389/fenvs.2022.798336
Cleveland, R. B., and Cleveland, W. S. (1990). Stl: a seasonal-trend decomposition procedure based on loess. J. Official Statistics 6, 3
Deng, H., and Chen, Y. (2017). Influences of recent climate change and human activities on water storage variations in Central Asia. J. Hydrol. X. 544, 46–57. doi:10.1016/j.jhydrol.2016.11.006
Dong, Z., Hu, H., Wei, Z., Liu, Y., Xu, H., Yan, H., et al. (2022). Estimating the actual evapotranspiration of different vegetation types based on root distribution functions. Front. Earth Sci. 10, 893388. doi:10.3389/feart.2022.893388
Famiglietti, J. S., Lo, M., Ho, S. L., Bethune, J., Anderson, K. J., Syed, T. H., et al. (2011). Satellites measure recent rates of groundwater depletion in california’s central Valley. Geophys. Res. Lett. 38, 2010GL046442. doi:10.1029/2010GL046442
Feng, W., Zhong, M., Lemoine, J.-M., Biancale, R., Hsu, H.-T., and Xia, J. (2013). Evaluation of groundwater depletion in North China using the gravity recovery and climate experiment (GRACE) data and ground-based measurements. Water Resour. Res. 49, 2110–2118. doi:10.1002/wrcr.20192
Getirana, A. (2016). Extreme water deficit in Brazil detected from space. J. Hydrometeorol. 17, 591–599. doi:10.1175/JHM-D-15-0096.1
Güntner, A. (2008). Improvement of global hydrological models using GRACE data. Surv. Geophys. 29, 375–397. doi:10.1007/s10712-008-9038-y
Güntner, A., Stuck, J., Werth, S., Döll, P., Verzano, K., and Merz, B. (2007). A global analysis of temporal and spatial variations in continental water storage. Water Resour. Res. 43, 5207. doi:10.1029/2006WR005247
He, X., Wada, Y., Wanders, N., and Sheffield, J. (2017). Intensification of hydrological drought in california by human water management. Geophys. Res. Lett. 44, 1777–1785. doi:10.1002/2016GL071665
Hirsch, R. M., Slack, J. R., and Smith, R. A. (1982). Techniques of trend analysis for monthly water quality data. Water Resour. Res. 18, 107–121. doi:10.1029/WR018i001p00107
Hu, H., Chen, L., Liu, H., Ali Khan, M., Tie, Q., Zhang, X., et al. (2018). Comparison of the vegetation effect on ET partitioning based on eddy covariance method at five different sites of northern china. Remote Sens. 10, 1755. doi:10.3390/rs10111755
Huang, J., Yu, H., Guan, X., Wang, G., and Guo, R. (2016). Accelerated dryland expansion under climate change. Nat. Clim. Chang. 6, 166–171. doi:10.1038/nclimate2837
Koster, R. D., Dirmeyer, P. A., Guo, Z., Bonan, G., Chan, E., Cox, P., et al. (2004). Regions of strong coupling between soil moisture and precipitation. Science 305, 1138–1140. doi:10.1126/science.1100217
Liu, W., Wang, L., Zhou, J., Li, Y., Sun, F., Fu, G., et al. (2016). A worldwide evaluation of basin-scale evapotranspiration estimates against the water balance method. J. Hydrol. X. 538, 82–95. doi:10.1016/j.jhydrol.2016.04.006
Liu, Z. (2022). Causes of changes in actual evapotranspiration and terrestrial water storage over the eurasian inland basins. Hydrol. Process. 36, e14482. doi:10.1002/hyp.14482
Liu, Z., Yao, Z., Huang, H., Wu, S., and Liu, G. (2014). Land use and climate changes and their impacts on runoff in the yarlung zangbo river basin, china. Land Degrad. Dev. 25, 203–215. doi:10.1002/ldr.1159
Liu, Z., Yao, Z., and Wang, R. (2019). Automatic identification of the lake area at Qinghai–Tibetan Plateau using remote sensing images. Quat. Int. 503, 136–145. doi:10.1016/j.quaint.2018.10.023
Long, D., Longuevergne, L., and Scanlon, B. R. (2014). Uncertainty in evapotranspiration from land surface modeling, remote sensing, and GRACE satellites. Water Resour. Res. 50, 1131–1151. doi:10.1002/2013WR014581
Long, D., Scanlon, B. R., Longuevergne, L., Sun, A. Y., Fernando, D. N., and Save, H. (2013). GRACE satellite monitoring of large depletion in water storage in response to the 2011 drought in Texas. Geophys. Res. Lett. 40, 3395–3401. doi:10.1002/grl.50655
Meng, F., Su, F., Li, Y., and Tong, K. (2019). Changes in terrestrial water storage during 2003–2014 and possible causes in Tibetan Plateau. J. Geophys. Res. Atmos. 124, 2909–2931. doi:10.1029/2018JD029552
Ramillien, G., Frappart, F., and Seoane, L. (2014). Application of the regional water mass variations from GRACE satellite gravimetry to large-scale water management in Africa. Remote Sens. (Basel). 6, 7379–7405. doi:10.3390/rs6087379
Reager, J. T., Gardner, A. S., Famiglietti, J. S., Wiese, D. N., Eicker, A., Thonicke, K., et al. (2016). Enhanced seasonal CO2 exchange caused by amplified plant productivity in northern ecosystems. Science 6, 696–699. doi:10.1126/science.aac4971
Rodell, M., Famiglietti, J. S., Wiese, D. N., Reager, J. T., Beaudoing, H. K., Landerer, F. W., et al. (2018). Emerging trends in global freshwater availability. Nature 557, 651–659. doi:10.1038/s41586-018-0123-1
Scanlon, B. R., Faunt, C. C., Longuevergne, L., Reedy, R. C., Alley, W. M., McGuire, V. L., et al. (2012). Groundwater depletion and sustainability of irrigation in the US high Plains and Central Valley. Proc. Natl. Acad. Sci. U. S. A. 109, 9320–9325. doi:10.1073/pnas.1200311109
Scanlon, B. R., Zhang, Z., Save, H., Sun, A. Y., Müller Schmied, H., van Beek, L. P. H., et al. (2018). Global models underestimate large decadal declining and rising water storage trends relative to GRACE satellite data. Proc. Natl. Acad. Sci. U. S. A. 115, E1080–E1089. doi:10.1073/pnas.1704665115
Seneviratne, S. I., Corti, T., Davin, E. L., Hirschi, M., Jaeger, E. B., Lehner, I., et al. (2010). Investigating soil moisture–climate interactions in a changing climate: a review. Earth. Sci. Rev. 99, 125–161. doi:10.1016/j.earscirev.2010.02.004
Voss, K. A., Famiglietti, J. S., Lo, M., de Linage, C., Rodell, M., and Swenson, S. C. (2013). Groundwater depletion in the middle east from GRACE with implications for transboundary water management in the Tigris-Euphrates-Western Iran region. Water Resour. Res. 49, 904–914. doi:10.1002/wrcr.20078
Wahr, J., Swenson, S., Zlotnicki, V., and Velicogna, I. (2004). Time-variable gravity from GRACE: first results. Geophys. Res. Lett. 31 (11). doi:10.1029/2004GL019779
Wang, F., Chen, Y., Li, Z., Fang, G., Li, Y., Wang, X., et al. (2021). Developing a long short-term memory (LSTM)-based model for reconstructing terrestrial water storage variations from 1982 to 2016 in the Tarim River Basin, Northwest China. Remote Sens. (Basel). 13, 889. doi:10.3390/rs13050889
Wang, J., Song, C., Reager, J. T., Yao, F., Famiglietti, J. S., Sheng, Y., et al. (2018). Recent global decline in endorheic basin water storages. Nat. Geosci. 11, 926–932. doi:10.1038/s41561-018-0265-7
Wang, T.-Y., Wang, P., Zhang, Y.-C., Yu, J.-J., Du, C.-Y., and Fang, Y.-H. (2019). Contrasting groundwater depletion patterns induced by anthropogenic and climate-driven factors on Alxa Plateau, northwestern China. J. Hydrol. X. 576, 262–272. doi:10.1016/j.jhydrol.2019.06.057
Wang, X., Chen, Y., Li, Z., Fang, G., Wang, F., and Liu, H. (2020). The impact of climate change and human activities on the aral sea basin over the past 50 years. Atmos. Res. 245, 105125. doi:10.1016/j.atmosres.2020.105125
Watkins, M. M., Wiese, D. N., Yuan, D.-N., Boening, C., and Landerer, F. W. (2015). Improved methods for observing Earth’s time variable mass distribution with GRACE using spherical cap mascons: improved gravity observations from GRACE. J. Geophys. Res. Solid Earth 120, 2648–2671. doi:10.1002/2014JB011547
Wiese, D. N., Landerer, F. W., and Watkins, M. M. (2016). Quantifying and reducing leakage errors in the JPL RL05M GRACE mascon solution. Water Resour. Res. 46, 7490–7502. doi:10.1002/2016WR019344
Xiang, L., Wang, H., Steffen, H., Wu, P., Jia, L., Jiang, L., et al. (2016). Groundwater storage changes in the Tibetan Plateau and adjacent areas revealed from GRACE satellite gravity data. Earth Planet. Sci. Lett. 449, 228–239. doi:10.1016/j.epsl.2016.06.002
Yeh, P. J.-F., Swenson, S. C., Famiglietti, J. S., and Rodell, M. (2006). Remote sensing of groundwater storage changes in Illinois using the Gravity Recovery and Climate Experiment (GRACE): rapid communication. Water Resour. Res. 42. doi:10.1029/2006WR005374
Zhang, C., Tang, Q., and Chen, D. (2017). Recent changes in the moisture source of precipitation over the Tibetan Plateau. J. Clim. 30, 1807–1819. doi:10.1175/JCLI-D-15-0842.1
Zhang, L., Yi, S., Wang, Q., Chang, L., Tang, H., and Sun, W. (2019). Evaluation of GRACE mascon solutions for small spatial scales and localized mass sources. Geophys. J. Int. 218, 1307–1321. doi:10.1093/gji/ggz198
Zhu, X., Zhang, M., Wang, S., Qiang, F., Zeng, T., Ren, Z., et al. (2015). Comparison of monthly precipitation derived from high-resolution gridded datasets in arid Xinjiang, central Asia. Quat. Int. 358, 160–170. doi:10.1016/j.quaint.2014.12.027
Keywords: hydrological budget, inland closed basin, water balance, continental river basin, terrestrial water storage
Citation: Liu X, Liu Z and Wei H (2022) Trends of terrestrial water storage and actual evapotranspiration in Chinese inland basins and their main affecting factors. Front. Environ. Sci. 10:963921. doi: 10.3389/fenvs.2022.963921
Received: 08 June 2022; Accepted: 20 July 2022;
Published: 30 August 2022.
Edited by:
Xiuping Li, Institute of Tibetan Plateau Research (CAS), ChinaReviewed by:
Jie Wang, Nanjing University of Information Science and Technology, ChinaMohd Yawar Ali Khan, King Abdulaziz University, Saudi Arabia
Copyright © 2022 Liu, Liu and Wei. This is an open-access article distributed under the terms of the Creative Commons Attribution License (CC BY). The use, distribution or reproduction in other forums is permitted, provided the original author(s) and the copyright owner(s) are credited and that the original publication in this journal is cited, in accordance with accepted academic practice. No use, distribution or reproduction is permitted which does not comply with these terms.
*Correspondence: Zhaofei Liu, emZsaXVAaWdzbnJyLmFjLmNuJiN4MDIwMGE7