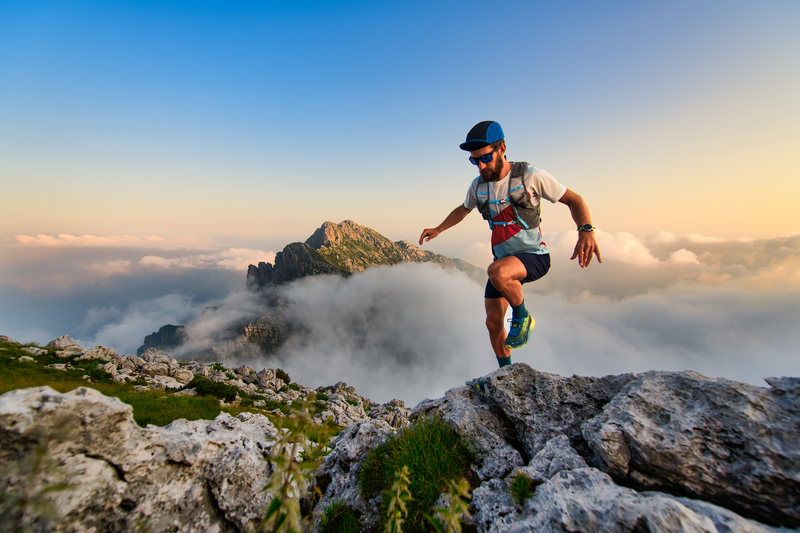
95% of researchers rate our articles as excellent or good
Learn more about the work of our research integrity team to safeguard the quality of each article we publish.
Find out more
BRIEF RESEARCH REPORT article
Front. Environ. Sci. , 06 September 2022
Sec. Environmental Economics and Management
Volume 10 - 2022 | https://doi.org/10.3389/fenvs.2022.962633
This article is part of the Research Topic Economic Development, Social Consequences, and Technological Innovation Under Climate Change COVID-19 Pandemic Conditions View all 48 articles
China’s Yangtze River Delta region has banned the approval of new thermal power projects except for combined heat and power (CHP) generation and is promoting technological innovation in CHP. However, technological innovation of energy technology has been evidenced to be often affected by spatial elements. The role of spatial agglomeration in the energy industry on technological innovation has not been discussed in the context of CHP. Therefore, this article studied the spatial agglomeration and evolution characteristics of CHP technological innovation point elements through the Global Moran's index, kernel density analysis, and thermal map analysis and analyzed the influencing factors and changes by the spatial regression model. The results show that environmental regulation and high-tech park agglomeration are the key factors influencing CHP technological innovation. This indicates that future policy making needs to consider the economic factors of green development and the role of high-tech parks in innovation.
With the increasing global energy demand, it is urgent to find new alternative energy or adjust the existing energy structure Chou et al. (2020); Lan et al. (2021). Combined heat and power generation (CHP), a mode of combined production of heat and electricity, is a technology for comprehensive energy utilization (Jimenez-Navarro et al., 2020). CHP is a new mode of production derived from the traditional thermal power industry. After the oil crisis in the 1970s, CHP received great attention from Western countries. With the global energy crisis and environmental deterioration, the research and development (R&D) in sustainable energy technologies has been gradually popularized around the world (He et al., 2020a, 2020b; Qian et al., 2021; Wei et al., 2021; Pu et al., 2022; Su et al., 2022). After steam is generated by a boiler of a traditional thermal power plant that drives the steam turbine generator set to generate electricity, the steam which has been discharged still contains most of the heat taken away by cooling water, so the thermal efficiency of the thermal power plant is only 30–40% (Vishwanathan et al., 2018). If the heat energy of the steam-driven steam turbine process or subsequent steam pumping or discharge can be used, it can generate both electricity and heat. This process has both electrical and thermal energy production. It is an efficient form of energy utilization at the same time as heat and electricity production. CHP has many advantages such as high efficiency of energy utilization and environmental protection and is regarded as the best centralized heating source (Zheng et al., 2021). In addition, due to the large capacity, high chimney, and high dust removal efficiency of the boilers that generate steam, the CHP unit is beneficial to the realization of desulfurization and denitrification in the furnace (Pu et al., 2019). Compared with traditional thermal power plants, their social and environmental benefits are significant. Although innovation discussion on energy technologies has spread to economic, behavioral, and policy areas (Bai et al., 2021; Zhang et al., 2022; Li et al., 2018), regional policy significantly has a huge impact on CHP technology. When compared with a single conventional power supply and heating system, the CHP projects have many advantages such as high energy utilization efficiency and environmental protection and are considered to be the best central heating source. After being aware of this, the National Development and Reform Commission of China officially issued a notice in 2016, which is on the development plan of urban agglomerations in the Yangtze River Delta. This plan aims to improve the coordination mechanism of air pollution prevention and control in the Yangtze River Delta region and solve air environmental problems in an integrated and coordinated manner. Among them, it is worth noting that the plan requires new projects in Shanghai, Jiangsu, and Zhejiang to prohibit the construction of self-provided coal-fired power stations, prohibit coal consumption projects to implement the replacement by coal reduction, and prohibit approval of new coal-fired power generation projects except the CHP (Zhang and Zheng, 2020). According to statistics, the CHP of the current heat source in China’s heating industry accounts for 62.9%, while the rest accounts for 37.1%.
Although the significant increase in the proportion of CHP is a good signal to achieve environmental protection requirements under climate change, further technological innovation in CHP technology is an important way to further achieve low-carbon development. At present, the categories of CHP unit mainly include the type of back-pressure, the type of steam pumping condensing, and the type of pumping back-pressure. But scholars do not limit their attention to traditional technological means or transformations. At present, more expectations are to integrate new and clean energy into CHP systems. For example, Suman (2018) believes that the study of new energy systems in which renewable energy and fossil fuels are complementary is extremely important for building efficient and safe modern energy systems. On the one hand, it is necessary to establish an overall performance model of the solar combined cycle unit to realize the online performance monitoring of ISCC units and master the operation characteristics of the unit. On the other hand, it is also necessary to study the variation of the peaks regulating the capacity of the ISCC system under CHP conditions, so as to provide a theoretical and design basis for operation optimization and application of ISCC units in CHP (Wang et al., 2021). Similarly, Zhou et al. (2019) have reviewed the types and research prospects of energy storage technology in the CCHP system and believe that the application methods of the power storage and thermal energy storage technology in the CCHP system are pluralistic. It has been pointed out that under the combination of traditional energy and renewable energy and the increasingly complex energy development trend of energy supply systems, system characteristics, optimization technology, and the formulation of operation plans for different scenarios are the future innovative directions of energy storage technology and CCHP integrated systems. It can be seen that CHP has great potential for technological innovation, and its innovation has multiple potential directions in technologies.
But how did the technological innovation of CHP happen? Bai et al. (2021) take the smart grid as an example and point out that there are obvious technological differences between the smart grid in China and the United States in terms of technology. China pays more attention to the innovation of hardware, while the United States pays more attention to intelligent scheduling. But more importantly, based on the analysis of China’s technology innovation patent data, they have found that the innovation of China’s smart grid technology has obvious spatial differentiation, which is related to regional characteristics. This study inspires this article to take CHP technology as an example to further verify the spatial differentiation of technological innovation and its motivations. Different from the study of the smart grid by Bai et al. (2021), the innovation of thermal technology in China is often significantly related to requirements of environmental protection and industrial policies, which has been mentioned by several scholars (Ouyang et al., 2020). This is mainly because thermal power, as a high-pollution industry, is often the most regulated industry and its production often needs to be carried out in specific industrial parks, which lead to the research purpose of this article, namely, the correlations among CHP technological innovations, green total elements, and industrial policies. In terms of industrial policies, taking the Yangtze River Delta as an example, China is presently promoting innovations such as the CHP technology by establishing high-tech industrial parks, the hope of which is mainly to gather innovation elements such as scientific research resources and achieve them. In recent years, more and more studies have used spatial detection methods. Spatial analysis is a process of cognition, interpretation, prediction, and regulation of spatial information. The results of spatial analysis depend on the distribution of events. The relationship between things with spatial attributes is detected according to the theory of spatial data analysis and spatial connection processes—both location information and attribute data (Ying ad Ning, 2005). Spatial analysis works well in describing and showing the unique spatial information, relationships, patterns, and processes contained in these data, exploring what’s behind the phenomenon taking spatial patterns as its occurrence mechanism. At the same time, space is the interface between the existence of things and interaction between humanity and nature, so geographical space is also the index of multisource information and the interface of man–land relationship. Taking the above situation into consideration, this article takes the Yangtze River Delta, an area that pays the most attention to CHP technology, as an example, discusses the spatial differentiation of CHP innovation by referring to the method of space detection proposed by Bai et al. (2021), and explores the impact of green total factors and high-tech industrial agglomeration on CHP innovation.
This article takes the Yangtze River Delta urban agglomeration as the research object, which includes 25 cities in the Shanghai, Jiangsu, Zhejiang, and Anhui provinces. The data of green total factor eco-efficiency used in this article are mainly from the China Statistical Yearbook and the Wind-Economic Database from 2015 to 2019. The data of the 25 cities in the Yangtze River Delta are selected, and the corresponding indicators are selected by referring to Chen et al. (2018). The patent data used in this study are from the patent database of PatSnap. This article searched the keyword “combined heat and power generation” in October 2021, and a total of 5,068 technological innovation patents from the Yangtze River Delta region in related fields were obtained before 31 December 2020. The point data of the industrial park was obtained from the Gaode geographic data platform by Python technology.
The Moran’s I index is often used to describe the spatial clustering characteristics of an attribute in the whole region and reflect the spatial correlation of the observed values in the spatial neighborhood. It also summarizes the number of enterprises in each unit of space to determine the degree of their spatial agglomeration. In this article, the Moran’s I index is used to test whether there is spatial dependence or spatial heterogeneity among the 25 cities selected in this study, so as to analyze the characteristics of spatial correlation among technological innovation efficiency of the 25 cities in the Yangtze River Delta. The formula is as follows:
In formula (1),
Kernel density estimation is a nonparametric method of estimation, which studies the distribution characteristics of data from the data sample itself and has strong adaptability. Combined with the spatial smoothing technology, kernel density estimation is widely used in the visualization and detection of spatial point patterns. Based on data samples, the density of point events within a certain radius of each sample is calculated to calculate the intensity of events. The output grid pixel is the smooth surface of the sum of all the core surface values superimposed on the center of the grid pixel, which can intuitively identify the differences in the spatial density distribution of the data. This article explores the spatial heterogeneity of the CHP energy industry in the Yangtze River Delta. The formula of the kernel density estimation method is as follows:
In the formula,
In this article, the method of analyzing hot spots is used to distinguish the local spatial clustering types of CHP enterprises in the Yangtze River Delta: Gi∗ statistical value of each grid unit in the statistical data set can be used to judge the spatial clustering of high and low values in the region, and low-value clusters and high-value clusters are the spatial distribution of cold spots and hot spots. The analysis of hot spots is more accurate in detecting local spatial autocorrelation, especially high-value agglomeration, and the calculation formula is as follows:
In the equation,
For the Moran' index of CHP technology innovation in the Yangtze River Delta, the values of I were, respectively, 0.027304, 0.001518, 0.063144, and 0.023341 in 2005, 2010, 2015, and 2020, while the values of Z were, respectively, 0.507122, 0.342052, 0.758221, and 0.469879. Judging from these results of global autocorrelation measurements, the four-year Global Moran’s I index has been between 0.0015 and 0.0631, has been positive, and has passed the significant test at the level of 1%, which indicates that the CHP technology innovation industry in the Yangtze River Delta, on the whole, has significant spatial agglomeration and dependence, that is, the level of CHP technology innovation industry in each city is positively affected by its neighboring districts and counties. In terms of temporal evolution, since 2005, the Global Moran’s I index has shown a trend of decreasing first, then increasing and again decreasing, with large decreasing amplitudes in 2010 and 2020, indicating that the degree of spatial agglomeration and dependence of industries on CHP technology innovation in the Yangtze River Delta has weakened, and the industrial layout has presented a trend of diffusion.
With the help of ArcGIS, the spatial kernel density of the point distribution of CHP technology innovation enterprises in the urban agglomeration of the Yangtze River Delta from 2005 to 2020 was estimated and visualized according to the natural fracture method classification in four selected years: 2005 (Figure 1A), 2010 (Figure 1B), 2015 (Figure 1C), and 2020 (Figure 1D). It can be seen from the figures that the spatial patterns of the CHP technology innovation industry in the urban agglomeration of the Yangtze River Delta presents the following characteristics: Shanghai’s core position has always been stable, the sub-centered position of Nanjing and Hangzhou has been increasingly prominent, the intensity of agglomeration continues to improve, and the radiation range has expanded significantly, which presents the evolution pattern of “One Core of Shanghai”–“Dual Cores of Shanghai and Nanjing”–“Three cores of Shanghai, Nanjing, and Hangzhou.” It has been indicated that the developmental gradient of the Jiangsu Province is increasingly obvious which is from the initial sprouting and unfinished climate to the emergence of small agglomerations in the main urban areas of the cities, and then to the scale and intensity of agglomerations in various cities, showing that the spatial heterogeneity distribution is strong in the south and weak in the north. Among them, Nanjing plays a prominent role as the core of the province and has become an important radiating point in the Yangtze River Delta. With Shanghai and Hangzhou in series to form the northwest–southeast axis belt, the Yangtze River Delta industrial belt begins to take shape. Generally speaking, from the presentation of the four selected years, the number of CHP technology innovation enterprises in the Yangtze River Delta urban agglomeration has surged, the distribution density has increased as a whole, and the spatial agglomeration scope has continued to expand. This has indicated that the cliff-like gap between Shanghai and its surrounding cities is gradually shrinking, and the pattern of “three cores and two axes” has gradually been formed and the regional development equilibrium has been improved, initially showing a trend of coordinated development.
FIGURE 1. Kernel density of CHP technological innovation in 2005 (A), 2010 (B), 2015 (C) and 2020 (D).
The hot spot results of the four selected years of 2005 (Figure 2A), 2010 (Figure 2B), 2015 (Figure 2C), and 2020 (Figure 2D) can effectively identify characteristics of the specific distribution and local variation of CHP technology innovation industry agglomeration in the Yangtze River Delta. From 2005 to 2020, the number of significant local cities in the CHP technology innovation industry layout in the Yangtze River Delta has shown an upward trend first and then a decline, which is in line with the previous changes’ trend of the Moran’s I index. The hot spots are concentrated in the northeast of the Yangtze River Delta, and significant types have gradually increased, indicating that the spatial dependence on local areas is becoming stronger and stronger. The specific performances are as follows: in 2005 (Figure 2A), the H-H cluster mainly spread around Shanghai and its surrounding cities, became concentrated in the downtown of Shanghai and Nantong city, and gradually extended to the surrounding areas, highlighting the vigorous development of the CHP technology innovation industry in Shanghai. In 2010 (Figure 2B), Hangzhou and Suzhou, which are located around Shanghai, also showed the H-H cluster, reflecting the enhancement of the radiation power of the Shanghai CHP technology innovation industry. From 2010 to 2020 (Figure 2C and Figure 2D), the H-H cluster shrank and was confined to Shanghai, indicating that its CHP technology innovation industry had relatively good development but limited radiation power, which is presented as an “isolated island” form.
FIGURE 2. Distribution of CHP technology innovation hotspots in the Yangtze River Delta in 2005 (A), 2010 (B), 2015 (C) and 2020 (D).
Considering the availability and operability of data, this article refers to the research related to energy industry agglomeration, and based on the comprehensive consideration of the principles such as being available, quantifiable, comparable, and so on, eight factor indicators were selected according to the original data of green all-factor TFP in 285 prefecture-level cities from 2003 to 2018 for specific construction to test the impact of the relevant factors on the CHP technology innovation industry, and further to clarify the effective intensity of different factors and analyze the influencing mechanism of the spatial pattern of the CHP technology innovation industry. We named the industrial soot emission (ton), discharge amount of wastewater, sulfur dioxide emissions (ton), PM2.5, quantity of employment, stocks of capital, total electricity consumption, constant-price GDP as Esoot, Ewas, Esul, Epm, Qe, Sc, Te, and C, respectively. At the same time, the hierarchical visualization was carried out according to the numerical values to obtain the characteristics of spatial differentiation of the eight indicators at four time nodes. Firstly, the visualized results of spatial differentiation of each index were compared with the results of kernel density analysis of the creative industry for visual translation, and the influencing factors were preliminarily determined. Then, the indicator graphs of the same time nodes in the visual translation results were superimposed with hierarchical assignment, and the result was the spatial differentiation pattern under the comprehensive action of each indicator, which has spatial coupling with the kernel density analysis results. For 2003–2005 (Figure 3A), the influencing factors included Esoot Qe; for 2005–2010 (Figure 3B), the influencing factors included Esul Qe C; for 2010–2015 (Figure 3C), the influencing factors included Esoot Esul Qe Te C; and for 2015–2018 (Figure 3D), the influencing factors included Esoot Esul Epm Qe Te C.
FIGURE 3. Spatial differentiation of influencing factors in 2003–2005 (A), 2005–2010 (B), 2010–2015 (C) and 2015–2018 (D).
The results show that the driving effect of energy technology innovation on total factor ecological efficiency is not monotonous (decreasing) but a nonlinear impact under the regulation of the economic development level, energy policy planning, and market level. Generally speaking, the results of the factors affecting the CHP technology innovation pattern of the four-time nodes in Figure 3 show that the region with the high economic development level, area with the high environmental protection management level, area with the high energy policy planning level, and area with the high market level effectively promote the optimal range of regional ecological efficiency of the total factors to stimulate energy technology innovation.
The development of the CHP technology innovation industry in the Yangtze River Delta has spatial heterogeneity. Based on the above global–local perspective on the detection results of the influencing factors, this article attempts to build the relationship between CHP technology innovation and agglomeration of high-tech industrial parks (Figure 4). It demonstrates that the influencing mechanism of the development of the CHP technology innovation industry in the Yangtze River Delta mainly includes three factors: aggregation of resources, social environment, and policy environment. Each factor contains many elements and has self-contained integration. The factors are interrelated and interact with each other, and jointly play a role in the development and evolution of the CHP technology innovation industry. Based on the analysis of multiple factors, it was found that the role of high-tech park agglomerations on CHP technology innovation enterprises is significantly higher than is for the other factors. CHP technology innovation enterprises prefer to gather in high-tech park agglomerations with more preferential policies, such as the High-Tech Park in northern Shanghai and the Fuhua High-Tech Park, which are at the national level near the Gonghe New Road, Central Shanghai. This shows that effective planning and guidance of the government has played an important role in the reasonable selection of the location of CHP technology innovation enterprises and the optimization of the industrial structure of CHP technology innovation.
FIGURE 4. Relationship between CHP technology innovation and agglomeration of high-tech industrial parks.
Taking enterprise development as the window and cities in the Yangtze River Delta region as the spatial scale, this article selects data from 2005 to 2020, makes use of kernel density estimation and spatial autocorrelation analysis to explore the evolution of spatial heterogeneity pattern and spatial dependence pattern of the CHP technology innovation industry in the Yangtze River Delta in the past 15 years, and uses green life. The main factors affecting the spatial location of CHP technology innovation enterprises were analyzed, and then the influencing mechanism of the layout of the CHP technology innovation industry in the Yangtze River Delta region was discussed. The main conclusions are as follows:
1) The overall Moran’s I index of the CHP technology innovation industry in the Yangtze River Delta is between 0.0015 and 0.0631 at four time nodes, which is positive and has passed the significant test at the level of 1%, indicating that the CHP technology innovation industry in the Yangtze River Delta has significant spatial agglomeration and dependence as a whole, that is, the level of CHP technology innovation industry in each city is greatly affected by the positive direction of the adjacent districts and counties.
2) The spatial pattern of the CHP technology innovation industry in the Yangtze River Delta urban agglomeration presents the following characteristics: Shanghai’s core position has always been stable. Over time, the subcenter status of Nanjing and Hangzhou has become increasingly prominent, the intensity of agglomeration continues to improve, and the radiation range has expanded significantly, presenting the evolution mode of “One Core of Shanghai”–“Dual Cores of Shanghai and Nanjing”–“Three cores of Shanghai, Nanjing, and Hangzhou."
3) The results of the analysis of cold and hot spots show that the H-H cluster mainly spreads around Shanghai and its surrounding cities. By 2010, Hangzhou and Suzhou, located around Shanghai, also showed H-H clusters, reflecting the enhancement of the radiation of Shanghai’s CHP technology innovation industry. In the decade from 2010 to 2020, H-H clusters shrank, basically limited to Shanghai, indicating that its CHP technology innovation industry has developed relatively well, but its radiation is limited, presenting an “island” form.
4) The results of the influencing factors of CHP technology innovation pattern through the analysis of green production show that areas with a high economic development level, areas with a high environmental protection management level, and areas with high energy policy planning and a high market level effectively promote the optimal range of regional all-factor ecological efficiency to stimulate energy technology innovation. The specific performance is that the higher the agglomeration degree of the high-tech parks, the more obvious are the agglomeration characteristics of the CHP technology innovation enterprises.
At present, energy development in China is facing very serious situations and challenges. However, based on the analysis of the great potential of institutional innovation and technological innovation in China’s energy field and the series of policies and measures that the Chinese government will implement to promote sustainable energy development, China can still achieve sustainable energy supply for a considerable period in the future. Cogeneration is a new industry with economic benefits, environmental benefits, and social benefits. It is an energy-saving and consumption-reducing mode encouraged by national policies. The construction of the Yangtze River Delta region must develop ecological industries such as cogeneration to purify the environment. As mentioned above, cogeneration is beneficial to the vital interests of both suppliers and users. Therefore, cogeneration enterprises should seize the opportunity, overcome difficulties, meet challenges, and continue to expand publicity to let more people know the advantages of cogeneration, accelerate the installation of heat and power pipe networks, further improve air quality, and promote the comprehensive and healthy development of the Yangtze River Delta.
The raw data supporting the conclusion of this article will be made available by the authors, without undue reservation.
All authors contributed to the study conception and design. Material preparation, data collection, and analysis were performed by CS, CW, and ZW. The first draft of the manuscript was written by CS and LZ, and all authors commented on previous versions of the manuscript. YT performed the data collection and final draft editing. All authors read and approved the final manuscript.
This work was supported by National Social Science Fund of China (The Research on the Construction and application of Regional Financial Risk Index in China, Grant No. 18ZDA093). The authors of this research are thankful for the comments from the reviewers and the translation help provided by Mr. Yue Wu from Queen Mary University of London.
The authors declare that the research was conducted in the absence of any commercial or financial relationships that could be construed as a potential conflict of interest.
All claims expressed in this article are solely those of the authors and do not necessarily represent those of their affiliated organizations, or those of the publisher, the editors, and the reviewers. Any product that may be evaluated in this article, or claim that may be made by its manufacturer, is not guaranteed or endorsed by the publisher.
Bai, Y., Chou, L., and Zhang, W. (2021). Industrial innovation characteristics and spatial differentiation of smart grid technology in China based on patent mining. J. Energy Storage 43, 103289. doi:10.1016/j.est.2021.103289
Chen, C., Lan, Q., Gao, M., and Sun, Y. (2018). Green total factor productivity growth and its determinants in China’s industrial economy. Sustainability 10 (4), 1052. doi:10.3390/su10041052
Chou, L. C., Zhang, W. H., Wang, M. Y., and Yang, F. M. (2020). The influence of democracy on emissions and energy efficiency in America: New evidence from quantile regression analysis. Energy & Environ. 31 (8), 1318–1334. doi:10.1177/0958305x19882382
He, P., Zeng, L., and Dai, J. (2020a). Energy enterprise management in China under the perspective of green development.” in E3S Web of Conferences, Shanghai, October 24, 2020 199. EDP Sciences, 00016.
He, P., Zeng, L., and Dai, J. (2020b). “Management strategy under the intelligent manufacturing initiative of Chinese coal machine equipment enterprises,” in International conference on mechanical Engineering and applied composite materials (Cham: Springer), 447
Jimenez-Navarro, J. P., Kavvadias, K., Filippidou, F., Pavičević, M., and Quoilin, S. (2020). Coupling the heating and power sectors: The role of centralised combined heat and power plants and district heat in a European decarbonised power system. Appl. Energy 270, 115134. doi:10.1016/j.apenergy.2020.115134
Lan, Z., Zhao, Y., Zhang, J., Jiao, R., Khan, M. N., Sial, T. A., et al. (2021). Long-term vegetation restoration increases deep soil carbon storage in the Northern Loess Plateau. Sci. Rep. 11, 13758. doi:10.1038/s41598-021-93157-0
Li, D., Zhao, Y., Zhang, L., Chen, X., and Cao, C. (2018). Impact of quality management on green innovation. J. Clean. Prod. 170, 462–470. doi:10.1016/j.jclepro.2017.09.158
Ouyang, X., Li, Q., and Du, K. (2020). How does environmental regulation promote technological innovations in the industrial sector? Evidence from Chinese provincial panel data. Energy Policy 139, 111310. doi:10.1016/j.enpol.2020.111310
Pu, G., Zhu, X., Dai, J., and Chen, X. (2022). Understand technological innovation investment performance: Evolution of industry-university-research cooperation for technological innovation of lithium-ion storage battery in China. J. Energy Storage 46, 103607. doi:10.1016/j.est.2021.103607
Pu, L., Wang, X., Tan, Z., Wu, J., Long, C., and Kong, W. (2019). Feasible electricity price calculation and environmental benefits analysis of the regional nighttime wind power utilization in electric heating in Beijing. J. Clean. Prod. 212, 1434–1445. doi:10.1016/j.jclepro.2018.12.105
Qian, X., Bai, Y., Huang, W., Dai, J., Li, X., and Wang, Y. (2021). Fuzzy technique application in selecting photovoltaic energy and solar thermal energy production in belt and road countries. J. Energy Storage 41, 102865. doi:10.1016/j.est.2021.102865
Su, C., Wei, H., Wang, Z., Ayed, H., Mouldi, A., and Shayesteh, A. A. (2022). Economic accounting and high-tech strategy for sustainable production: A case study of methanol production from CO2 hydrogenation. Int. J. Hydrogen Energy. doi:10.1016/j.ijhydene.2022.01.124
Suman, S. (2018). Hybrid nuclear-renewable energy systems: A review. J. Clean. Prod. 181, 166–177. doi:10.1016/j.jclepro.2018.01.262
Vishwanathan, G., Sculley, J. P., Fischer, A., and Zhao, J. C. (2018). Techno-economic analysis of high-efficiency natural-gas generators for residential combined heat and power. Appl. Energy 226, 1064–1075. doi:10.1016/j.apenergy.2018.06.013
Wang, Z., Duan, L., and Zhang, Z. (2021). Thermoeconomic cost analysis on operation strategies of gas turbine combined cycle under off-design conditions. Case Stud. Therm. Eng. 28, 101617. doi:10.1016/j.csite.2021.101617
Wei, H., Dai, J., Maharik, I., Ghasemi, A., Mouldi, A., and Brahmia, A. (2021). Simultaneous synthesis of H2, O2, and N2 via an innovatory energy system in coronavirus pandemic time: Design, techno-economic assessment, and optimization approaches. Int. J. Hydrogen Energy 47 (62), 26038–26052. doi:10.1016/j.ijhydene.2021.12.044
Ying, L., and Ning, Y. (2005). Spatial data: Nature, impact, and analytical methods. Adv. Earth Sci. 20 (1), 49. doi:10.11867/j.issn.1001-8166.2005.01.0049
Zhang, J., and Zheng, Y. (2020). The flexibility pathways for integrating renewable energy into China’s coal dominated power system: The case of Beijing-Tianjin-Hebei Region. J. Clean. Prod. 245, 118925. doi:10.1016/j.jclepro.2019.118925
Zhang, W. H., Chou, L. C., and Chen, M. (2022). Consumer perception and use intention for household distributed photovoltaic systems. Sustainable Energy Techn. Assessm. 51, 101895.
Zheng, P., Liu, P., and Zhang, Y. (2021). Economic assessment and control strategy of combined heat and power employed in centralized domestic hot water systems. Appl. Sci. 11 (10), 4326. doi:10.3390/app11104326
Keywords: combined heat and power, innovation, Moran’s I, high-tech parks, China, economics, green total factors
Citation: Su C, Wang C, Wang Z, Zhang L and Tian Y (2022) Technological innovation evolution and industrial modernization driven by green factors: Case of combined heat and power (CHP) industry in Yangtze River Delta, China. Front. Environ. Sci. 10:962633. doi: 10.3389/fenvs.2022.962633
Received: 06 June 2022; Accepted: 03 August 2022;
Published: 06 September 2022.
Edited by:
Faik Bilgili, Erciyes University, TurkeyReviewed by:
Muhammad Kamran Khan, Bahria University, PakistanCopyright © 2022 Su, Wang, Wang, Zhang and Tian. This is an open-access article distributed under the terms of the Creative Commons Attribution License (CC BY). The use, distribution or reproduction in other forums is permitted, provided the original author(s) and the copyright owner(s) are credited and that the original publication in this journal is cited, in accordance with accepted academic practice. No use, distribution or reproduction is permitted which does not comply with these terms.
*Correspondence: Lu Zhang, emhhbmdsdS5lZHVAb3V0bG9vay5jb20=
Disclaimer: All claims expressed in this article are solely those of the authors and do not necessarily represent those of their affiliated organizations, or those of the publisher, the editors and the reviewers. Any product that may be evaluated in this article or claim that may be made by its manufacturer is not guaranteed or endorsed by the publisher.
Research integrity at Frontiers
Learn more about the work of our research integrity team to safeguard the quality of each article we publish.