- 1School of Physical Education, Huaqiao University, Quanzhou, China
- 2School of Physical Education, Nanjing Xiaozhuang University, Nanjing, China
- 3Basic Teaching Department, Wenzhou Business College, Wenzhou, China
In China, sports parks, and green spaces are often spatially integrated to realize the multiple functions of shared green spaces and play an important role in the production and living services of its residents. In this article, it is collectively referred to as green sport space (GSS). Whether the distribution of GSS is equal has an important impact on the sustainable lifestyle and the rehabilitation under the pandemic. Based on the POI data of the Shanghai urban area, it is preliminarily found that the areas with extremely high and high production and living densities are mainly distributed in downtown Shanghai. Polarization of the GSS distribution area and the high heat of points of interest can be seen. When the service radius of the GSS in Shanghai is 500, 750, and 1,000 m, the green space ecological service area can reach 2089.08, 3164.62, and 4469.75 km2, covering 26.17, 39.64, and 55.99% of the total area, respectively. The coverage for walking accessibility of GSSs in Shanghai is extremely uneven. Based on network analysis, the overall accessibility of GSS under the walking mode in each residential district fails to meet the standard of a 15-min living circle, with an average of 15.37 min. The evaluation results of this plan demonstrate that Shanghai needs to further provide GSS space for the public in future to improve public wellbeing and diversify sports spaces.
1 Introduction
Urban green space is an important part of urban public service facilities, and its layout is closely related to people’s life and sustainable urban development (Wüstemann et al., 2017; Žlender and Thompson, 2017). Therefore, the layout of urban green spaces should reflect the concept of equity so that all classes of society have equal opportunities to enjoy urban green spaces (Haughton, 1999; Agyeman et al., 2002; Stessens et al., 2017). City Park was first born in the United Kingdom. It is not only an inevitable product of the progress of cities and human civilization under the background of industrialization but also reflects people’s long-term pursuit of a better living environment and healthy lifestyle. The first city park in the world was Birkenhead Park in Liverpool, built by the British government in 1843. The birth of Birkenhead Park not only promoted the construction of parks in Britain but also affected places such as Europe and the United States. In addition to the first city park Birkenhead Park, Central Park in New York also has an important influence on the construction of city parks today.
The research on the spatial equity of urban park supply has always drawn the attention of Western academic circles, and accessibility analysis is a common method to measure spatial equity. In the 1990s, geographers first introduced the accessibility model to evaluate the rationality of public facility distribution. Scott and Soja (1996) believe that there is a correlation between social interaction and the accessibility of green open space. They take socioeconomic status (income and education), block variables (block location and residence time), and leisure time (daily outdoor activities, marital status, and number of children) as parameters and apply the method of generalized estimation equation to analyze the relationship between social interaction and accessibility of the game field. Lee and Hong (2013) believe that urban accessibility is regional, and poor accessibility in many cities is due to the large population density and the small number of parks in the region. Through accessibility assessment, it is helpful for determining the location of people whose potential needs cannot be met and areas with poor accessibility, which will help city planners understand the service level and scope of the park in the planned area. Since the 21st century, with the development of measurement technology, research tends to adopt quantitative methods such as mathematical models and GIS spatial analysis to evaluate spatial equity. The literature on environmental justice from the perspective of sociology has also gradually increased. Boone et al. (2009) believe that the distribution of public service facilities needs to reflect the fairness of social distribution. Combined with the needs of users with different attributes, they analyze the fairness of the spatial distribution of urban parks in Baltimore, Maryland. It is found that within the 400-m buffer zone, White people have more opportunities to reach the park on foot than Black people. Scarcity of parks in black residential areas is higher than that in white residential areas. This phenomenon reflects the unfairness of the environment. Dadashpoor et al. (2016) believe that everyone in society should enjoy the services of public facilities fairly without being restricted by their identities and status.
The issue of fairness and justice of ecological services in China has gradually received attention. Meanwhile, China’s discussion of green parks has focused on their fitness and athletic functions, while helping realize its National Fitness Program. For this, in China, green spaces and sports parks are spatially integrated to realize the multiple functions of shared green spaces and therefore play an important role in the production and living services of its residents. In this article, it is collectively referred to as green sport space (GSS). For example, in the master plans of major cities such as Beijing and Shanghai, it is required to achieve “a 15-min community fitness circle”. As an important and basic public service facility in the community, the accessibility of green spaces with fitness facilities has become a significant improvement indicator. In addition, large-scale public green space is expected to meet the requirements of 5-min walk accessibility (Liu et al., 2008; Fan et al., 2016).
Fan et al. (2017) provided planning suggestions for Shanghai Xinhua Street in terms of accessibility, functionality, and sharing level with the goal of a 15-min living and fitness circle. At present, in many cities in China, the indicator system guiding urban GSS planning and construction still focuses on urban-scale equality, that is, the system uses four indicators: GSS rate, per capita green area, per capita GSS area, and urban–rural greening rate to guide urban greenbelt construction (Zhou and Rana, 2012; Shen et al., 2017). It can be found that although these indicators are conducive to the overall analysis of the quantitative characteristics of urban GSSs, they do not consider whether the service level of a park GSS can meet the actual needs of residents. If to only analyze the amount of GSS from a macro perspective without analyzing the rationality of the layout of urban GSSs based on the city’s real economic conditions, traffic conditions, crowd characteristics, and social needs, the analysis results will be divorced from reality (Annerstedt van den Bosch et al., 2016; Gupta et al., 2016; Friedman, 2020). Therefore, to solve this problem, based on spatial analysis, this study selects Shanghai as a sample to explore the spatial layout of urban GSS and evaluates the fairness of the spatial distribution of urban GSSs in Shanghai, hoping to provide some reference value for the future location and construction of urban GSSs.
2 Materials and Methods
2.1 Overview of Shanghai
2.1.1 Research Data
This study takes Shanghai as the research object because Shanghai has implemented a series of urban greening constructions since the 1980s. According to the 2020 Shanghai Municipal Statistical Yearbook, the current green area of Shanghai is 157,800 hm2. By specifying parks with fitness facilities, there are 352 with an area of 21,400 hm2. The green coverage rate of the built city is 39.7%, and the per capita GSS area reaches 13m2. Two types of data are mainly used in this research. The first type is spatial data, including Shanghai administrative boundary, regional parks, GSS data, regional road traffic network data, and regional residential area data. All spatial data acquisition follows scientific and systematic methods, making full use of official portals such as Shanghai Urban Planning and Natural Resources Bureau, Shanghai Municipal People’s Government, and electronic maps such as Gaode map. A unified spatial coordinate system is used to calibrate the data to ensure that all data are compatible. Urban economic data are also supplemented by reference to environmental economic studies (Islam et al., 2021; Khan et al., 2021; Zhang et al., 2021; Godil et al., 2022; Rehman et al., 2022). The spatial point data of all green parks in Shanghai and the descriptive text of relevant parks were crawled at the same time, and we screened out the parks with fitness facilities in the description by searching. Finally, 607 GSSs were found in Shanghai. In order to help analysis, the government statistical data were also used, which mainly come from the Shanghai Statistical Yearbook released by the Shanghai Municipal Bureau of Statistics in November 2020.
2.2 Research Methods
2.2.1 Kernel Density Estimation
Points of interest cover the location and attribute information of all facilities related to production and life in the city, which can provide decision-making services for urban planning and management and obtain the spatial distribution characteristics of urban facilities and events from a macro perspective (Bai et al., 2021; Guerrero et al., 2016). Generally speaking, the higher the concentration of points of interest, the more intensive the production and living activities in the region and vice versa (Wang et al., 2018). Compared with methods such as the quadrant density and Voronoi diagram density, the kernel density method has more advantages in the expression of spatial features of points of interest (Bielecka et al., 2020). Therefore, this study uses the kernel density analysis method to simulate the heat map of the point of interest. The specific calculation formula is as follows:
In the formula,
2.2.2 Spatial Analysis of ArcGIS
The spatial analysis method is mainly applicable to the empirical analysis of the research on the fairness of urban public GSS. Urban public GSS has geospatial attributes. To reflect and display the spatial distribution pattern of urban public GSS, the GIS spatial platform is needed for multidimensional analysis (Sathyakumar et al., 2020). At the same time, combined with other spatial data and socioeconomic data with spatial attributes, overlay analysis, spatial statistical analysis, and other methods are adopted to further discuss the unfairness of public GSS. The accessibility of urban public GSS is drawn in the form of a map to accurately locate the areas of public GSS unfair. In this study, ArcGIS 10.2 is used as the operation platform, and the kernel density analysis tool in spatial analysis is adopted to analyze the points of interest in Shanghai. In practice, the setting of the search radius (related to the distance attenuation threshold) has an important impact on the results.
To scientifically reflect the heat of production and life in the region on a macro level, the natural grading method is adopted, dividing the kernel density values of points of interest in the study area into five grades from high to low: extremely high density, high density, medium density, relatively low density, and low density. Then, overlapped with the GSS entity, the spatial distribution of the GSS and POI dense area is preliminarily analyzed (Dong et al., 2018).
Generally speaking, public GSS can provide good ecological services for residents living within 500 m (walking time is less than 15 min), relatively good services for residents living within 500–750 m (walking time is about 15–25 min), average services for residents living within 750–1,000 m (walking time is about 20–30 min), and few services for those living beyond 1,000 m (Dong et al., 2018; Chen et al., 2020). Therefore, after a simple analysis of the spatial distribution characteristics of the GSS entity and POI heat zone, this study made three buffer zones with different radii of 500, 750, and 1,000 m for the GSS and coupled the analysis with the POI heat map. This article discusses relevant issues from the perspective of the supply of ecological services in GSS and the demand for ecological services in POI hot areas.
2.2.3 Multimode Two-step Floating Catchment Area Method
The two-step floating catchment area method is adopted to evaluate the accessibility of GSS from the perspective of GSS supply and population demand, that is, the more GSS the community residents can enjoy, the higher the GSS accessibility level. However, the two-step floating catchment area method based on ArcGIS network analysis cannot reflect the accessibility of GSS in multiple travel modes. Also, it is especially difficult to simulate and calculate the accessibility through public transportation and cannot reflect the real-time and objective road network conditions (Yang et al., 2021). Therefore, based on the traditional two-step floating catchment area method, combined with the application of TIQS, this study constructs and adopts a multi-mode two-step floating catchment area method (M2SFCA) to measure the accessibility of multi-scale GSSs in Shanghai, considering two travel modes of walking and cycling. The specific calculation process is as follows:
First, the travel time to reach grade
The first step is to calculate the supply-demand ratio of GSS, that is, the service capacity of GSS:
In the formula,
The second step is to calculate the sum of the supply-demand ratio of the accessible GSS for each community within the time threshold, that is, the accessibility of GSS for community
In the formula,
3 Results
3.1 Basic Characteristics of Green Sport Space Distribution
The patches of GSS in the urban area of Shanghai are relatively fragmented, and the green patches are distributed from the Bund in downtown Shanghai, and the density decreases successively. The green patches are most densely distributed between the inner ring and outer ring of the city. In Hongkou, Changning, and Xuhui districts, there are more green patches. The area of a single GSS is small, and the distribution is relatively scattered, showing bands, rings, and radials. Outside the urban core area, the green patches are mainly concentrated in the Shanghai Binhai Forest Park area, Jinqiao Park area, Chuansha New City area in Pudong New Area, Yangpu Xinjiangwancheng area, Gucun Park in Baoshan District, Dongtan Wetland Park area in Chongming District, and the Maqiao area of Minhang District. From the aspect of orientation, the GSS in Shanghai is characterized by more in the north and less in the south, more in the east, and less in the west. In the east of Xuhui District along the Huangpu River, Shanghai Botanical Garden, Longhua Park, riverside GSS, Houtan Park, and other public GSS resources are densely distributed, and financial services, business, and entertainment formats concentrate along the Huangpu River, with a relatively small residential population. Zhangjiang Science and Technology Park, as one of the important centers for the future urban layout of Pudong, is under development and construction. There has not yet been a large-scale concentrated population there. However, there are district-level parks such as Zhangjiang Theme Park, Guanglan Park, and Ziwei Park in the surrounding area.
3.2 Basic Characteristics of Point of Interest Heat Distribution
Through the kernel density analysis of POI in Shanghai, the kernel density distribution map of POI in Shanghai is shown in Figure 1. From the graph, the points of interest in Shanghai are mainly distributed in the city center. The closer to the city center, the higher the density of points of interest. In the area within the central ring road of Shanghai, the density of interest points is generally high. In the areas outside the central ring road of Shanghai, the density of points of interest gradually decreases with the increase of the distance from the city center, and the density of points of interest outside the outer ring road is generally low. From the aspect of orientation, as a whole, the central area has a higher density of points of interest than the surrounding areas in the four directions of east, west, south, and north, showing a trend of central agglomeration. On the other hand, the distribution of points of interest in the north is more concentrated, while that in the south is relatively scattered. Compared with the west of the city, the distribution of points of interest in the east is relatively concentrated, while that in the west is relatively scattered. As a social and economic complex, Shanghai’s agglomeration effect and economies of scale are its most basic characteristics, which can be better reflected by the characteristics of POI kernel density.
3.3 Analysis of Different Service Radii of GSS Based on Point of Interest Heat Map
The spatial overlay of the POI kernel density grading distribution map in Figure 1, and the GSS is shown in Figure 2.
As can be seen from Figure 2, the areas with extremely high and high production and living density are mainly distributed in the south of Hongkou District, Jing’an District, the east of Changning District, and the northeast of Xuhui District. At the same time, there are some areas with low interest, where GSS has an advantage, such as Shenlong Ecological Park in Fengxian District, Shanghai Gulf National Forest Park, Binhai Forest Park in Pudong New Area, and Golf Park. In the extremely high-density, high-density, and medium-density areas, the urban GSS area is 28.71hm2, accounting for 5.10% of the total GSS area; in the low-density area, the urban GSS has an area of 512.48hm2, accounting for 91.13% of the total area of GSS, showing polarization of the GSS distribution area and the high heat of points of interest. Urban GSSs and intensive residential production and living areas inevitably overlap in space, but urban GSSs should be able to give full play to their effective service radius. Therefore, by further analyzing the buffer zones with different radii of urban GSSs (Figure 3), the study obtains the coverage of the buffer zones with a radius of 500 m (Figure 3A), 750 m (Figure 3B), and 1,000 m (Figure 3C) in POI heat areas in the study area, and the GSS service areas enjoyed by different levels of heat areas are as follows: 1) lowest density area: it has a total area of 5692.80 km2, and its 500-, 750-, and 10,000-m ecological service areas are 1942.78, 2982.94, and 4205.62, respectively; 2) lower density area: it has a total area of 1562.36 km2, and its service areas are 70.31, 86.68, and 105.12; 3) medium-density area: it has a total area of 433.69 km2, and its service areas are 45.52, 52.16, and 76.52; 4) high-density area: it has a total area of 190.09 km2, and its service areas are 21.02, 28.25, and 48.22; 5) extremely high-density area: it has a total area of 104.82 km2, and its service areas are 9.46, 14.59, and 34.26.
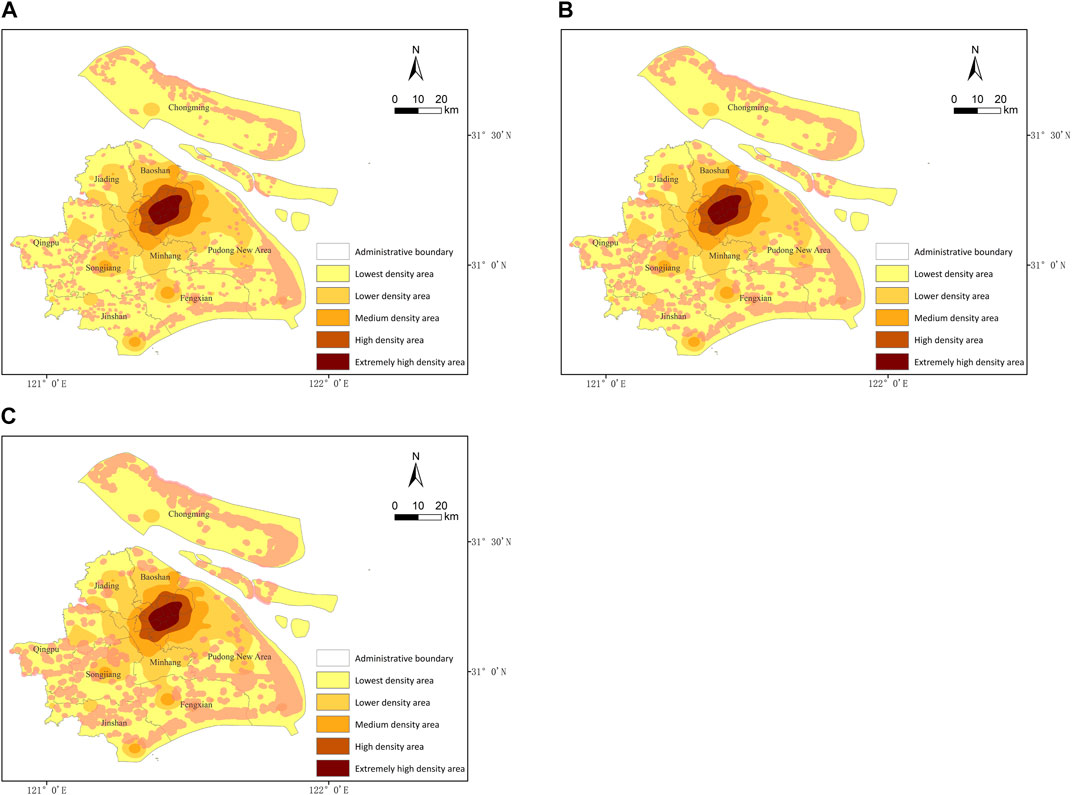
FIGURE 3. Coverage of different radii ecological service areas of GSS. (A) 500 m, (B) 750 m, and (C) 1,000 m.
When the service radius of GSS is 500, 750, and 1,000 m, the GSS ecological service area can reach 2089.08, 3164.62, and 4469.75 km2, covering 26.17%, 39.64%, and 55.99% of the total area, respectively. From the perspective of the service supply of GSS, its spatial distribution pattern is relatively even. At the same time, within the 500 m service radius of GSS, only 9.02% of the extremely high-density area, 11.06% of the high-density area, and 10.50% of the medium-density area in the POI heat map are covered, which shows the uneven spatial distribution pattern of GSS from the perspective of service demand.
3.4 Analysis of Accessibility
3.4.1 Walking Mode
According to Formula 3, the accessibility evaluation results of public GSS in Shanghai in the walking mode are calculated, as shown in Figure 4A. According to the results, the walking accessibility of urban public GSSs presents a clear trend of agglomeration distribution with park GSS as the core. The areas with higher walking accessibility levels are mainly distributed in the surrounding area of urban public GSS and gradually expand outward with public GSS as the center, and the accessibility level decreases successively. The accessibility level in most peripheral areas tends to be zero. From the perspective of specific spatial distribution, the coverage for walking accessibility of public GSSs in Shanghai is extremely uneven. Based on network analysis, the overall walking accessibility of urban parks in each residential area is poor, with an average of 15.37 min. Among them, the residential area with the accessibility of over 30 min 1956) is the least, accounting for about 3.33% of the total residential area, and the residential area with the accessibility of less than 10 min (23,603) is the most, followed by those with accessibility between 10 and 20 min (22,180), accounting for 40.15 and 37.73%, respectively. From the spatial distribution, the accessibility of residential parks in the central level area of Shanghai presents a cobweb pattern. Although the surrounding large-scale comprehensive parks can guarantee service coverage, there is a lack of small parks as a supplement.
3.4.2 Cycling Mode
According to Formula 4, the accessibility evaluation results of public GSS in Shanghai in the cycling mode are shown in Figure 4B. Compared with the results of walking accessibility, the level of cycling accessibility in public GSS has significantly improved, and the distribution of high accessibility areas is irregular and diffuse. Compared with the results of walking accessibility, the riding accessibility level of public GSS has significantly improved, and the distribution of high accessibility areas is irregular and diffuse. Due to the high commuting efficiency of the cycling mode, the coverage of the high-value cycling accessibility area is much bigger than that of the walking accessibility area. Based on the network analysis method, the accessibility level of parks in each residential area in the cycling mode is obtained. Also, the overall accessibility is relatively high, with an average of 13.41 min. Among them, the residential communities with accessibility less than 10 min (32,962) accounted for 56.07% of the total residential communities, followed by the residential communities with the accessibility less than 10–20 min (32.63%), and the residential communities with accessibility greater than 30 min were rare.
4 Conclusion
From the view of orientation, the GSS in Shanghai is characterized by more in the north and less in the south, more in the east, and less in the west. The central area has a higher density of points of interest than the surrounding areas in the four directions of east, west, south, and north, showing a trend of central agglomeration.
The areas with extremely high and high production and living densities are mainly distributed in downtown Shanghai, which includes the south of Hongkou District, Jing’an District, the east of Changning District, and the northeast of Xuhui District. Polarization of the GSS distribution area and the high heat of points of interest can be seen.
When the service radius of the urban GSS in Shanghai is 500 m, 750 m, and 1,000 m, the GSS ecological service area can reach 2089.08, 3164.62, and 4469.75 km2, covering 26.17%, 39.64%, and 55.99% of the total area, respectively.
As shown in the accessibility evaluation results of public GSS in Shanghai in the walking mode, the coverage for walking accessibility of public GSSs in Shanghai is extremely uneven. Based on the network analysis, the overall accessibility of urban parks under the walking mode in each residential district fails to meet the standard of a 15-min living circle, with an average of 15.37 min.
As shown in the accessibility evaluation results of public GSS in Shanghai in the cycling mode, compared with the results of walking accessibility, the level of cycling accessibility in public GSS has significantly improved, and the distribution of high accessibility areas is irregular and diffuse. Based on the network analysis method, the accessibility level of parks in each residential area in the cycling mode is obtained, and the overall accessibility is relatively high, with an average of fewer than 15 min.
Data Availability Statement
The raw data supporting the conclusion of this article will be made available by the authors, without undue reservation.
Author Contributions
All authors contributed to the study’s conception and design. Material preparation, data collection, and analysis were performed by TQ and DZ. The first draft of the manuscript was written by TQ and WL, and all authors commented on previous versions of the manuscript. All authors read and approved the final manuscript.
Conflict of Interest
The authors declare that the research was conducted in the absence of any commercial or financial relationships that could be construed as a potential conflict of interest.
Publisher’s Note
All claims expressed in this article are solely those of the authors and do not necessarily represent those of their affiliated organizations, or those of the publisher, the editors, and the reviewers. Any product that may be evaluated in this article, or claim that may be made by its manufacturer, is not guaranteed or endorsed by the publisher.
Acknowledgments
We acknowledge the comments from reviewers.
References
Agyeman, J., Bullard, R. D., and Evans, B. (2002). Exploring the Nexus: Bringing Together Sustainability, Environmental Justice and Equity. Space polity 6 (1), 77–90. doi:10.1080/13562570220137907
Annerstedt van den Bosch, M., Mudu, P., Uscila, V., Barrdahl, M., Kulinkina, A., Staatsen, B., et al. (2016). Development of an Urban Green Space Indicator and the Public Health Rationale. Scand. J. Public Health 44 (2), 159–167. doi:10.1177/1403494815615444
Bai, Y., Chou, L., and Zhang, W. (2021). Industrial Innovation Characteristics and Spatial Differentiation of Smart Grid Technology in China Based on Patent Mining. J. Energy Storage 43, 103289.
Bielecka, E., Pokonieczny, K., and Borkowska, S. (2020). GIScience Theory Based Assessment of Spatial Disparity of Geodetic Control Points Location. Int. J. Geo Inf. 9 (3), 148. doi:10.3390/ijgi9030148
Boone, C. G., Buckley, G. L., Grove, J. M., and Sister, C. (2009). Parks and People: An Environmental Justice Inquiry in Baltimore, Maryland. Ann. Assoc. Am. Geogr. 99 (4), 767–787. doi:10.1080/00045600903102949
Chen, Y., Yue, W., and La Rosa, D. (2020). Which Communities Have Better Accessibility to Green Space? an Investigation into Environmental Inequality Using Big Data. Landsc. Urban Plan. 204, 103919. doi:10.1016/j.landurbplan.2020.103919
Dadashpoor, H., Rostami, F., and Alizadeh, B. (2016). Is Inequality in the Distribution of Urban Facilities Inequitable? Exploring a Method for Identifying Spatial Inequity in an Iranian City. Cities 52, 159–172. doi:10.1016/j.cities.2015.12.007
Dong, R. C., Jiang, T. Q., Li, H. H., Li, S. Y., Zhang, Y. L., and Fu, X. (2018). Green Space Service Radius Analysis of Beijing Urban Area Based on Electronic Navigation Map POI. Acad. J. Ecol. 38 (23), 8536–8543.
Fan, P., Xu, L., Yue, W., and Chen, J. (2017). Accessibility of Public Urban Green Space in an Urban Periphery: The Case of Shanghai. Landsc. Urban Plan. 165, 177–192. doi:10.1016/j.landurbplan.2016.11.007
Fan, Y., Zhao, M.-y., Ma, L., and Zhao, L.-y. (2016). Research on the Accessibility of Urban Green Space Based on Road Network- A Case Study of the Park Green Space in City Proper of Nanjing. J. For. Environ. Sci. 32 (1), 1–9. doi:10.7747/jfes.2016.32.1.1
Godil, D. I., Sarwat, S., Khan, M. K., Ashraf, M. S., Sharif, A., and Ozturk, I. (2022). How the Price Dynamics of Energy Resources and Precious Metals Interact with Conventional and Islamic Stocks: Fresh Insight from Dynamic ARDL Approach. Resour. Policy 75, 102470. doi:10.1016/j.resourpol.2021.102470
Guerrero, P., Møller, M. S., Olafsson, A. S., and Snizek, B. (2016). Revealing Cultural Ecosystem Services through Instagram Images: The Potential of Social Media Volunteered Geographic Information for Urban Green Infrastructure Planning and Governance. Urban Plan. 1 (2), 1–17. doi:10.17645/up.v1i2.609
Gupta, K., Roy, A., Luthra, K., Maithani, S., and Mahavir, (2016). GIS Based Analysis for Assessing the Accessibility at Hierarchical Levels of Urban Green Spaces. Urban For. urban Green. 18, 198–211. doi:10.1016/j.ufug.2016.06.005
Haughton, G. (1999). Environmental Justice and the Sustainable City. J. Plan. Educ. Res. 18 (3), 233–243. doi:10.1177/0739456x9901800305
Islam, M. M., Khan, M. K., Tareque, M., Jehan, N., and Dagar, V. (2021). Impact of Globalization, Foreign Direct Investment, and Energy Consumption on CO2 Emissions in Bangladesh: Does Institutional Quality Matter? Environ. Sci. Pollut. Res. 28 (35), 48851–48871. doi:10.1007/s11356-021-13441-4
Khan, M. K., Trinh, H. H., Khan, I. U., and Ullah, S. (2021). Sustainable Economic Activities, Climate Change, and Carbon Risk: an International Evidence. Environ. Dev. Sustain. 24, 1–23. doi:10.1007/s10668-021-01842-x
Lee, G., and Hong, I. (2013). Measuring Spatial Accessibility in the Context of Spatial Disparity between Demand and Supply of Urban Park Service. Landsc. Urban Plan. 119, 85–90. doi:10.1016/j.landurbplan.2013.07.001
Liu, Z., Mao, F., Zhou, W., Li, Q., Huang, J., and Zhu, X. (2008). “Accessibility Assessment of Urban Green Space: A Quantitative Perspective,” in IGARSS 2008-2008 IEEE International Geoscience and Remote Sensing Symposium, Boston, MA, USA, 07-11 July 2008 (IEEE), II–1314. doi:10.1109/igarss.2008.4779245
Rehman, A., Ma, H., Khan, M. K., Khan, S. U., Murshed, M., Ahmad, F., et al. (2022). The Asymmetric Effects of Crops Productivity, Agricultural Land Utilization, and Fertilizer Consumption on Carbon Emissions: Revisiting the Carbonization-Agricultural Activity Nexus in Nepal. Environ. Sci. Pollut. Res., 29:39827–39837. doi:10.1007/s11356-022-18994-6
Sathyakumar, V., Ramsankaran, R., and Bardhan, R. (2020). Geospatial Approach for Assessing Spatiotemporal Dynamics of Urban Green Space Distribution Among Neighbourhoods: A Demonstration in Mumbai. Urban For. Urban Green. 48, 126585. doi:10.1016/j.ufug.2020.126585
A. J. Scott, and E. W. Soja (Editors) (1996). The City: Los Angeles and Urban Theory at the End of the Twentieth Century (California: Univ of California Press).
Shen, Y., Sun, F., and Che, Y. (2017). Public Green Spaces and Human Wellbeing: Mapping the Spatial Inequity and Mismatching Status of Public Green Space in the Central City of Shanghai. Urban For. Urban Green. 27, 59–68. doi:10.1016/j.ufug.2017.06.018
Stessens, P., Khan, A. Z., Huysmans, M., and Canters, F. (2017). Analysing Urban Green Space Accessibility and Quality: A GIS-Based Model as Spatial Decision Support for Urban Ecosystem Services in Brussels. Ecosyst. Serv. 28, 328–340. doi:10.1016/j.ecoser.2017.10.016
Wang, Q., Phillips, N. E., Small, M. L., and Sampson, R. J. (2018). Urban Mobility and Neighborhood Isolation in America's 50 Largest Cities. Proc. Natl. Acad. Sci. U.S.A. 115 (30), 7735–7740. doi:10.1073/pnas.1802537115
Wüstemann, H., Kalisch, D., and Kolbe, J. (2017). Access to Urban Green Space and Environmental Inequalities in Germany. Landsc. Urban Plan. 164, 124–131. doi:10.1016/j.landurbplan.2017.04.002
Yang, W., Li, X., Chen, H. L., and Cao, X. S. (2021). Multi-Scale Accessibility of Green Spaces and Its Equity in Guangzhou Based on MultiMode Two-Step Floating Catchment Area Method (M2SFCA). Acta Eco Sin. 41. doi:10.5846/stxb202004080829
Zhang, L., Godil, D. I., Bibi, M., Khan, M. K., Sarwat, S., and Anser, M. K. (2021). Caring for the Environment: How Human Capital, Natural Resources, and Economic Growth Interact with Environmental Degradation in Pakistan? A Dynamic ARDL Approach. Sci. Total Environ. 774, 145553. doi:10.1016/j.scitotenv.2021.145553
Zhou, X., and Rana, M. P. (2012). Social Benefits of Urban Green Space: A Conceptual Framework of Valuation and Accessibility Measurements. Manag. Environ. Qual. Int. J. 23, 173. doi:10.1108/14777831211204921
Keywords: green sport space, equity, culture, Shanghai, planning, human geography, community
Citation: Qiu T, Zhou D and Li W (2022) Fitness Culture and Green Space Equity: Accessibility Evaluation of Shanghai Communities. Front. Environ. Sci. 10:958649. doi: 10.3389/fenvs.2022.958649
Received: 31 May 2022; Accepted: 16 June 2022;
Published: 18 July 2022.
Edited by:
Vishal Dagar, Great Lakes Institute of Management, IndiaReviewed by:
Muhammad Imran Khan, University of Agriculture, PakistanMuhammad Kamran Khan, Northeast Normal University, China
Copyright © 2022 Qiu, Zhou and Li. This is an open-access article distributed under the terms of the Creative Commons Attribution License (CC BY). The use, distribution or reproduction in other forums is permitted, provided the original author(s) and the copyright owner(s) are credited and that the original publication in this journal is cited, in accordance with accepted academic practice. No use, distribution or reproduction is permitted which does not comply with these terms.
*Correspondence: Wen Li, bGl3ZW5lbmdsaXNoQG91dGxvb2suY29t, MDAyMDExMDZAd3piYy5lZHUuY24=