- 1Institute of Shandong Development, Shandong University, Ji’nan, China
- 2Center for Economic Research, Shandong University, Ji'nan, China
- 3School of Civil Engineering, Shandong University, Ji’nan, China
- 4Center for Urban and Rural Human Settlements, Shandong University, Ji’nan, China
Spatial misallocation is an essential reason for the low utilization efficiency of construction land. Optimizing the spatial pattern of construction land allocation can improve the efficiency of economic operations and resilience to food crisis and climate change challenges. This study constructs a quantitative measurement method for the spatial misallocation of construction land (SMCL), proposes a new government market society support (GMSS) analytical framework for the contributing factors with interlocked relationships, and conducts empirical research in Shandong, China, which is a typical area with a rapid development of construction land and significant regional disparity. It is concluded that the SMCL ensues through the interaction and coevolution of the GMSS system, which plays a key role in adjusting the construction land use sequence, structure, and efficiency under the regulation of the upper government. Effectively using the estimation method based on the equal marginal output principle, the SMCL in Shandong is established as a downward trend, with evident temporal and spatial differentiation characteristics and spatial morphological mode changes, although most sub-regions are significantly approaching the adaptation interval with fluctuation. Furthermore, the empirical results of the regression model indicate that there are different effects and intensities on the SMCL among the contributing factors under the GMSS framework, wherein the local government force has an aggravating and the greatest effect, the market forces have a dual and second-ranking effect, the social forces play a positive but still weak role, and the support system has a differentiated improvement effect. However, the impacts of various dimensional factors on the SMCL also have heterogeneity in the development stages and different regions. Generally, in the low-level development stage and underdeveloped areas, the effect of local government intervention is stronger, the market forces’ importance is lower, and the social forces and support systems remain insufficiently robust.
1 Introduction
The intensive utilization of land resources is not only an important reflection of a country or a region’s economic and social development quality but also a necessary condition for sustainable development (Estoque et al., 2021; Lu et al., 2021; Hjalager et al., 2022). As the spatial carrier of rapid industrialization and urbanization in developing countries, construction land has maintained a rapid growth trend in recent decades, thereby posing increasingly severe challenges to global climate change, food security, and ecological environment protection, thus forcing them to pay more attention to the improvement of construction land utilization efficiency (Kissinger et al., 2019; Mendoza-Ponce et al., 2021; Pal et al., 2021). However, owing to various reasons, such as immature factor markets, excessive administrative intervention, and improper regional competition, there are serious misallocations of construction land among regions, industries, and enterprises in developing countries, hence resulting in a massive reduction of production efficiency and waste of land resources (Huang and Du, 2017; Jian Wang et al., 2020; Luo et al., 2022).
As one of the developing countries in the world with the largest total amount of construction land, fastest growth rate, and most evident human–land contradiction, China’s construction land misallocation (CLM) is even more prominent. In particular, the differences among regions in China are particularly obvious: local governments have to launch continuous central–local games and fierce inter-regional competition to obtain more construction land quotas under the institutional logic of the stimulation of economic growth by the central government via local decentralization (Song et al., 2020; Fu et al., 2021). However, it is very difficult to allocate construction land quotas that perfectly match the goal of maximizing output efficiency, especially when the central government tries to balance economic efficiency and regional development rights, which makes the spatial misallocation of construction land (SMCL) in China particularly typical and has been a remarkable obstacle to the intensive use of construction land (Chien, 2015; Bo, 2020; Liu et al., 2021; Zhou et al., 2022).
There are multidimensional negative impacts of the SMCL in China, including not only the direct consequences of loss of economic efficiency and waste of land resources but also the indirect consequences, such as industrial convergence among regions, industrial upgrading dilemma, public service insufficiency, ecological environment decline, food crises, social conflicts, and government corruption (Fang and Tian, 2020; Han Wang et al., 2020). While China is in a critical period of transforming its development mode, optimizing its economic structure, and transforming its growth drivers, ensuring the equal development rights of different regions and establishing a more effective governance mechanism to improve the overall utilization efficiency of construction land have become urgent issues. Therefore, the research on quantitatively measuring the SMCL and identifying its contributing factors and effects is fundamental to relative theoretical and practical targets.
By summarizing and sorting the existing literature, this study selects a quantitative measurement method and establishes a new analytical framework for the contributing factors of the SMCL. It takes Shandong province, China, as the study area to conduct the empirical research. The marginal contribution of this study is three-fold. First, based on the principle of equal marginal output, this study estimates the degree of the SMCL in the study area, which clearly reveals the volatile coupling characteristics of the SMCL and the regional development model, effectively identifies the spatial–temporal differentiation pattern and spatial correlation combination pattern of the SMCL, and further deepens the cognition of the evolution law of the SMCL in regions with rapid growth in construction land and significant internal differences. Second, the article establishes a government market society support (GMSS) analytical framework composed of government, market, social, and supporting system forces and discusses the moderating effect of the coevolutionary relationship of mutual influence and cross-connection on the SMCL, which presents a clearer theoretical summary of the system of factors influencing the SMCL. Third, by selecting representative indicators and constructing an econometric regression model, this study conducts a multi-angle empirical test on the role nature, role strength, and spatiotemporal changes of contributing factors in different dimensions. It provides a deeper understanding of the reasons behind the formation of the SMCL and its spatiotemporal changes, which can provide references for a more accurate regional construction land allocation strategy.
The remaining of this article is organized as follows: Section 2 reviews the existing literature and provides a summary and an evaluation. Section 3 proposes a new analytical framework of the contributing factors of the SMCL and clarifies the strategies of quantitative measurement and econometric model construction. In Section 4, the empirical results are analyzed and evaluated via a robustness test. Section 5 provides an analysis of the spatiotemporal heterogeneity of the contributing factors. The article is concluded and discussed in the final section.
2 Literature Review
2.1 Performances of the Spatial Misallocation of Construction Land
Construction land allocation has a diversity of participating subjects, allocation modes, functional uses, and time sequences of the realization process; therefore, its misallocation characteristics can be presented in different forms and fields (Opp et al., 2014; Wang et al., 2019; Fu et al., 2021). Notably, CLM performances are ultimately manifested as idle, inefficient, or overutilization of construction land. Hsieh and Klenow (2009) pointed out that the unequal marginal benefits of land between sectors or regions are the key identifying features of the regional misallocation of land resources. Other scholars also agreed with this viewpoint and believed that land misallocation typifies the violation of the efficiency principle, and it is manifested as marginal output differences among regions and sectors (Dheera-Aumpon, 2014; Aumpon, 2018). Banerjee and Moll (2010) further categorized resource misallocation into connotative and extensional misallocation, arguing that the former is characterized by the unequal marginal output of land utilization, while the latter is characterized by the fact that the marginal output is equal but has not yet reached the optimal state. This has also become a theoretical reference for SMCL research.
However, some scholars have indirectly analyzed the characteristics of land misallocation by economic and social consequences occasioned by land resource misallocation. They generally believe that resource misallocation can lead to significant productivity differences and efficiency losses, the degree of which can reflect the level of misallocation (Banerjee and Moll, 2010; Restuccia and Rogerson, 2017; Song et al., 2020). Kimura et al. (2011) analyzed the failure of the land factor market caused by transaction costs and believe that CLM not only leads to differences in the output efficiency but also hinders the free flow of factors and causes evident land externality losses. Chen (2017) established a two-sector general equilibrium model to quantify the loss in production efficiency engendered by land misallocation. Some scholars have conducted empirical analyses of China’s land market and found that the government’s strategic land supply behavior not only causes CLM among sectors and regions but also wastes a large amount of land resources (Du and Peiser, 2014; Wu et al., 2018; Hui Wang et al., 2020). In addition, scholars who discussed related fields, such as capital, labor, and housing market misallocation, could also provide abundant references vis à vis the characteristics of the SMCL (Midrigan and Xu, 2014; Liu and Wong, 2015; Dai and Cheng, 2019; Wu et al., 2020; Shi, 2022).
2.2 Measurement Methodology of the Spatial Misallocation of Construction Land
The existing literature mainly includes three types of measurement methods for the CLM. The first is the characteristic index method, which mainly selects one or several key indicators to characterize the CLM level. Huang and Du (2017) used the ratio of the average price of commercial land to the average price of industrial land to measure land misallocation. Some scholars use the proportion of land sold to the total land area (Li et al., 2016) or the ratio of the average price of land for a certain industry to the average price of industrial land (Huang and Du, 2017) to measure land misallocation. The data of the characteristic index method are easy to obtain, and the method is simple and direct, but it often only reflects one aspect of land misallocation and cannot fully reveal the overall state (Zhang et al., 2021). The second method is the parametric or non-parametric estimation approach. Guanchun Liu et al. (2018) used stochastic frontier analysis to measure the relative efficiency of construction land, while Song et al. (2022) used data envelopment analysis to measure the efficiency of urban land use output. From an efficiency level perspective, these methods show the overall state of CLM, but they can only reflect the relative degree of misallocation among different regions and cannot obtain the actual gap with the optimal allocation state. The third method is the multivariate linear equation solving technique. Song et al. (2020) studied the input–output relationship of land resources in different regions based on the calculated value and economic output of various land resource assets and reflected the regional misallocation of land resources by the differences in land output efficiency between regions. Other scholars have developed econometric equations to measure differences in the marginal output of land or productivity share covariance (Olley and Pakes, 1996), the relative productivity of factors (Yansui Liu et al., 2018), and the productivity gap caused by resource misallocation (Brandt et al., 2013), which are used to measure the level of land misallocation. However, these measurement methods can only be used to evaluate the allocation efficiency of land resources and cannot determine the specific state and source of land misallocation.
2.3 Contributing Factors and Determining Mechanism of the Spatial Misallocation of Construction Land
The research on the contributing factors and determination mechanisms of the SMCL covers a wide range. 1) Government intervention: through the monopoly of the land market and the inclined land supply policy, the government has sacrificed factor productivity and caused a loss of allocation efficiency while achieving the goal of macro-control (Brandt et al., 2013). However, Breton (1996) finds that local governments have increasingly become the actual controllers of land resources and compete with other regions with superior institutional arrangements and lower land prices, resulting in an SMCL. Meanwhile, the government’s differentiated policies in different sectors and regions, including state-owned and non-state–owned sectors, as well as government corruption and other reasons, can exacerbate the misallocation of resources (Shuhong Wang et al., 2020). It also further strengthens CLM (Brandt et al., 2013). 2) Market environment: distorted factor prices or imperfect factor markets have always been a significant reason behind land misallocation in developing countries (Banerjee and Moll, 2010; Aoki, 2012; Shenoy, 2017; Tang et al., 2020). Harrison (1982) and Wen et al. (2022) believed that accelerating the construction of a unified construction land market and promoting the process of land marketization will help alleviate the SMCL and improve land utilization (Baross and Mesa, 1986). Kimura et al. (2011) believed that reducing the transaction costs of market players is an important means to play the role of the land market. In addition, the lack of land property rights is an institutional reason that hinders the free trade of land, thereby causing land misallocation (Chen, 2017; Huang and Du, 2017; Chen et al., 2021; Gao et al., 2021). 3) Regional economic structure and development level: the characteristics of regional economic development and its structural characteristics, such as population agglomeration, economic development level, industrial structure, technology level, and land fiscal policy, have a significant impact on CLM (Huang and Du, 2017). Song et al. (2022) researched on resource-based cities using the Tobit model and found that it is more effective for local governments to improve land use efficiency by investing in road networks and science and technology. Britos et al. (2022) also confirmed that the degree of distortion in the land market in each region is related to road accessibility, ethnicity, and education level. Based on the data of listed manufacturing enterprises in China from 2010 to 2019, Li et al. (2022) also concluded that information and communications technology is positively correlated with the total factor productivity of manufacturing enterprises, which indirectly proves that the supply of regional science and technology has a positive impact on land allocation.
From the literature review, it is apparent that the current research results on the characteristics, measurement methods, and contributing factors of land misallocation have accumulated significantly, and this can provide valuable references for the research on the SMCL. However, the research has two drawbacks. First, most of the existing studies only discuss CLM among sectors, and there are still relatively few discussions on the SMCL issue. This may be more meaningful for areas with the rapid growth of construction land and significant regional development gaps. Second, the contributing factors proposed by the existing research involve a wide range of factors, and the types of factors are relatively complex, albeit they lack a highly concise and systematic analytical framework, which is also necessary to form targeted theories in related fields and guide the policy practices of developing countries.
3 Theoretical Framework and Empirical Strategies
3.1 Theoretical Framework
With the gradual improvement in the level of economic and social development in developing countries, the diversification of governance subjects and participation methods provides conditions for various stakeholders to jointly improve resource misallocation (Lu and Zhang, 2019; Chen and Lin, 2021; Silal and Saha, 2021). Previous studies have often regarded government power as the leading factor in the SMCL in developing countries, but practical cases and theoretical studies have begun to pay increasing attention to the role of market players and social forces in recent years (Calic and Ghasemaghaei, 2021; Yang and Lee, 2021). According to the classic theory of national and regional governance (Kollintzas et al., 2018; Lin et al., 2022), this study proposes a system of factors that influence the SMCL, composed of government, market, and social forces. Nonetheless, considering the basic support conditions for the three types of factors to play their respective roles, a GMSS theoretical framework is constructed wherein the GMSS system jointly affects the SMCL. In China, the upper-level government mainly controls the construction land in the lower-level area by planning approval, issuing the number of available quotas, and specifying the investment and tax intensity density of the construction land. However, under the condition of the same management and control framework and management and regulation robustness, the final SMCL level is determined by the adjustment mechanism within each region, which is also the research strategy and premise of most research institutes (Huang and Du, 2017; Dai and Cheng, 2019; Shuhong Wang et al., 2020; Wu et al., 2020; Fu et al., 2021). Under the GMSS analytical framework, there are: 1) local government forces: they intervene in the allocation of construction land mainly by implementing favorable policies for specific industries, improving the level of infrastructure and public service support, setting the benchmark price of local construction land, ordering and structuring the administrative grant, issuing permits for construction activities, and supervising the construction process. Because of these effective interventions, the government can impose critical external constraints on the overall efficiency of construction land use. 2) Market power refers to micro-market entities, such as enterprises and professional service institutions that provide enterprises with other production factors. They use the existing market system to maximize economic benefits under the condition of government intervention by lobbying the government, flexibly controlling production and investment decisions, conducting mutual transactions between market entities, and adjusting locations. The role of these micro-market players can ultimately be reflected in the macro-level structural features, such as regional industrial structure, factor supply environment, and spatial agglomeration structure, which represent the pattern of market power comparison. 3) Social forces are social subjects, including residents and non-profit organizations. To enjoy high-quality regional public services, they express their interests and sensitize the society on the rationality of government intervention and the compliance of enterprises to play an indirect regulatory role as a third party and affect the final SMCL degree. 4) Support systems, including regional natural conditions, ecological environment carrying capacity, completeness of infrastructure construction, public supply of scientific and technological innovation, and other factors. In a certain period, they can play a supporting or restricting function on the influence and interaction of the three forces and also determine the upper or lower limit of the utilization efficiency of producing factors to some extent, which also constitutes an important force that affects the SMCL. The four forces of the GMSS form a coevolutionary action system through mutual influence and causality to adjust the SMCL implementation, structure, and efficiency status. The interaction between them is easy to understand. Taking market adjustment forces as an example, they not only affect the government decision-making, social welfare level, and investment structure supporting system construction through lobbying, product supply, capital investment, and so on but also are significantly constrained by government regulation, social supervision, and regional investment carrying and return capacity. Thus, there is a pattern of interactive influence is formed between market regulatory forces and other forces. The analytical framework for the contributing factors of the SMCL is illustrated in Figure 1.
3.2 Empirical Strategies
3.2.1 The Spatial Misallocation of Construction Land Measurement Method
The quantitative measurement of the SMCL is a challenge that needs to be addressed before the empirical analysis herein. However, most of the existing methods are based on the relative evaluation of the allocation efficiency after resource input and can neither determine the nature and actual level of the misallocation nor obtain the optimal allocation size and more specific and clear implications for the regulation strategy. According to the basic economic theory, in a multiregional competition process, the marginal output of construction land input should be equal among regions, which can be an ideal equilibrium state for effective construction land allocation. Based on this logic, we can measure the SMCL in four steps.
First, we construct an estimation equation for the marginal output elasticity of regional construction land. The Cobb–Douglas production function has the attributes of stability, convenience of calculation, and linearizability. This study takes construction land as an independent factor of production and incorporates it into the Cobb–Douglas regional production function together with the input of capital and labor, and we obtain:
We take the logarithm on both sides of the equation to obtain the estimated marginal output elasticity of the construction land input as follows:
where A represents the regional comprehensive technical level and is defined as a constant to simplify the relevant calculation;
Among them,
Second, we empirically estimate the parameters by collecting years of actual data in the estimation equation presented earlier and using the panel data regression method to empirically estimate the marginal output elasticity of construction land in the study area.
Third, according to the principle of the equal marginal output of construction land between regions and referring to the calculation methods of Zhang et al. (2021) and Xie et al. (2022), the following simultaneous equations are established:
where
Finally, by comparing the scale of the actual and theoretical construction land in each region, the SMCL rate expressed by specific numerical values in a certain area can be calculated, denoted by
If
3.2.2 Model Setting and Variable Selection
Based on the aforementioned information, we take the measurement results of the SMCL rate as the explained variable and use the various contributing factors under the GMSS framework as the explanatory variables. Then, the econometric regression equation for the SMCL in typical regions of China can be constructed further. A quantitative empirical test is conducted on the role nature of different factors and their influence strength. In addition, this study undertakes heterogeneity analysis and robustness tests in different periods and sub-regions to gain a deeper understanding of the impact of different factors on the SMCL and its dynamic changes, thereby enriching existing research.
According to the GMSS analytical framework, each explanatory variable of the econometric model is determined by considering the literature references and data availability. 1) Government power factors: generally, the level of dependence of local governments on construction land leasing can reflect their motivation to intervene in construction land allocation, which can be calculated by the proportion of land leasing income to total fiscal revenue, denoted as
As all the explanatory variables are positive values and the sign of the misallocation rate represents whether the construction land allocation is excessive or short, its absolute value can also measure the degree of misallocation. Therefore, the explained variable is expressed by the absolute value of the SMCL rate in each sub-region calculated earlier, denoted as
Finally, the econometric regression model is constructed as follows:
The subscript i represents different regions in Shandong, and the subscript t represents different times.
3.2.3 Study Area and Data Sources
This study selects Shandong province, China, as the empirical research area and investigates the measurement of the SMCL, the evolution characteristics of the spatiotemporal pattern and its contributing factors. The typical value of the study area is mainly reflected in three aspects.
First, Shandong is a province with a large population and economy along the eastern coast of China. It not only plays an important role in China’s regional development pattern but also is highly similar to China’s national conditions due to its natural resource endowment, climatic conditions, population, and industrial structure. It is considered to be a classic epitome of the evolution of China’s economic and social development (Lu et al., 2011; Chen et al., 2022). In 2020, Shandong achieved a GDP of RMB 8.31 trillion, while the three industrial structures were in the ratio of 7.3:39.9:52.8, the permanent population at the end of the year was 101.70 million, and the urbanization level reached 63.94%. Overall, Shandong is still in a rapid industrialization and urbanization stage. Since 2000, the scale of urban construction land in Shandong has increased from 1,058.92 km2 to 3,951.85 km2, with an average annual growth rate of 8.1%, significantly exceeding the growth rate of urban population, and the pressure of the low level of construction land utilization and the large contradiction between supply and demand always exists (Figure 2). Therefore, taking Shandong as the research area clearly shows the evolution of the SMCL process during the rapid growth period.
Second, Shandong has formed a gradient development pattern of three major regions as the Jiaodong region (JDR), Jinan metropolitan-coordinating region (JNR), and Lunan region (LNR). The differences in the regional economic development levels and industrial structure characteristics are significant, which is helpful in identifying the characteristics of the SMCL and the regional heterogeneity of its contributing factors (Figure 3).
Third, as a northern province in China, governments at all levels in Shandong have a profound historical tradition of intervening and regulating the allocation of construction land (Qu et al., 2021). However, in recent years, Shandong has continued to promote a market-oriented process of construction land allocation in accordance with the requirements of the central government for comprehensively deepening reforms. A new type of allocation mechanism, led by the government, with enterprises as the main body and extensive participation of social forces and supported by scientific and technological innovation, is taking shape, which is also a typical value for examining the role of contributing factors and their dynamic changes under the GMSS construction land misallocation analytical framework.
This article takes 2000–2019 as the research period, and the required data are obtained from public statistical databases, such as “Shandong Statistical Yearbook,” “China Land and Resources Statistical Yearbook,” and “China Urban Construction Statistical Yearbook,” which are all available at https://data.cnki.net/ and http://tjj.shandong.gov.cn/. Even though the Laiwu region was merged into the Jinan region in January 2019, this study still considers the Laiwu region as a separate administrative area.
4 Results
4.1 The Measurement Result of the Spatial Misallocation of Construction Land
Before estimating the main parameters of Eq. 2, the Hausman test was performed. The test results rejected the null hypothesis, and the fixed effects model was used to estimate Eq. 2. The results of the regression using the panel data of 17 cities in Shandong during the study period are shown in Table 1.
Among them, the marginal product elasticity coefficient of the capital factor
Calculations are performed based on the obtained functions. Owing to the large sample size of cities in the study area, we display the measurement results and their time series changes according to three economic regions of Shandong. As shown in Figure 4, from 2000 to 2019, the SMCL degree in Shandong generally showed a declining trend. In 2000, the highest value of the SMCL rate in the province reached 0.59, which meant that the actual construction land usage in Laiwu exceeded approximately 59% of its theoretical construction land usage. The lowest value is −0.78, which means that the actual construction land in Linyi is much lower than its theoretical construction land scale, and the shortage in misallocation is very serious. In 2019, the distribution range of the SMCL is compressed between −0.35 and 0.31, which indicates that the SMCL of Shandong has been significantly reduced.
From the perspective of regions, except for a few areas such as Jinan and Liaocheng, the urban construction land in most areas significantly approaches the adaptation range. This shows that with the gradual improvement of market mechanisms and governance capacity, the SMCL has improved as a whole. Among them, cities with moderate and low levels of economic development, regions with a higher proportion of agriculture or traditional industries, and those with slower rates of industrialization and urbanization have a higher probability of shortage misallocation. However, the SMCL of none of the regions continuously improves or deteriorates without fluctuation. This shows that the regulation level of the provincial government still needs to be further strengthened.
Simultaneously, there were two regions that reversed their nature of misallocation during the study period. Among them, the Zibo region is a traditional industrial region. In 2000, the actual construction land use exceeded 24.57% of its theoretical value, but it dropped to −3.22% in 2019. In the context of governments all over China competing for construction land permit indicators, this also means that its regional competitiveness is significantly declining, while the Heze region is a traditional agricultural region. Industrialization and urbanization have developed rapidly in recent years. During the study period, the level of misallocation increased rapidly from −0.38 to 0.21. This proves that industrialization and urbanization strategies are still an apparent driving force for local governments to compete for construction land resources.
From the perspective of the three major regional plates in Shandong, the utilization of urban construction land within each plate is obviously close to the adaptation range. Although there are regions of excessive misallocation and shortage misallocation within each region, on the whole, the numerical distribution of the SMCL level in JDR is biased toward excessive misallocation, and most cities have obvious characteristics of agglomeration in a suitable range. The numerical distribution of the LNR is relatively discrete, but the overall bias is toward shortage misallocation, and the numerical distribution of the JNR is centered at zero and distributed symmetrically up and down, but the convergence trend is not obvious. It can be seen that it is suitable for the overall economic development level and development stage of the regional economic sector, and there are also certain regional differences in the misallocation pattern of urban construction land, while it provides a basis for the regional heterogeneity test of contributing factors in the following.
Combined with the spatial distribution map of the SMCL in some years, it can be seen more clearly that the SMCL in the province tends to ease, and the number of extremely high and extremely low values progressively decrease. In terms of the spatial pattern, in the base period of the study, Shandong generally presented an excessive misallocation agglomeration area with the east–west central zone as the core and a shortage misallocation agglomeration area on the north and south flanks. By 2019, it had been transformed into a high-value surface agglomeration area in JDR with Qingdao as the center, a high-low combination area in JNR with Jinan as the center, and an LNR with a staggered distribution of high and low values. In the new century, Shandong has implemented the breakthrough Yantai, breakthrough Jinan, and breakthrough Heze strategies. Shandong also has regional development ideas, such as double centers and solid provincial capitals. In these practices, the government plays an important role in the SMCL (Figure 5).
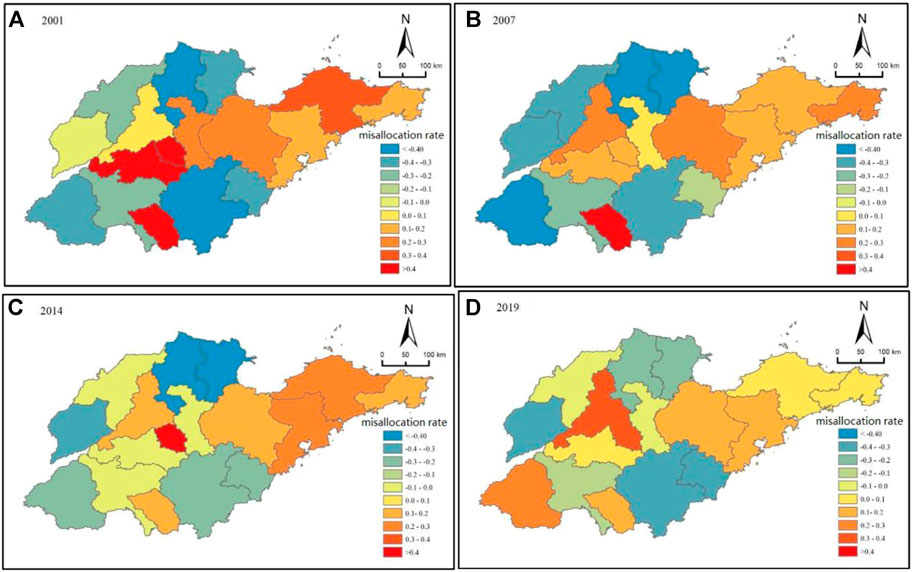
FIGURE 5. Spatial pattern and evolution of the SMCL in Shandong province between 2001 and 2019. (A) 2001; (B) 2007; (C) 2014; (5) 2019. Note: This figure shows only the results of 4 years, and the others can be obtained by contacting the correspondence author.
4.2 Empirical Analysis Results
This study adopts the stepwise regression method, adds variables in steps, and uses STATA 15 software for regression analysis. The regression results show that the explanatory power of the model significantly improves after adding variables in steps, and all variables pass the 5% significance test except for the insignificant level of land finance dependence in the final regression model, proving the overall rationality of the variable selection. We judged the specific influence of each factor based on three aspects. First, the sign of the regression coefficient represents the influence direction of the corresponding factor; a positive value means that the factor has an aggravating effect on the SMCL, and a negative value means that it has a mitigating effect. Second, the magnitude of the absolute value of the regression coefficient represents the impact strength of this factor on CLM, and the larger the absolute value, the stronger the aggravation or mitigation effect. Third, regardless of whether it is marked with “*” or not indicates whether the influence of the factor has passed the statistical test of the significance level, and the regression coefficient without marked “*” indicates that although the factor has a certain influence on the spatial mismatch of construction land, but the actual probability of having an impact is low.
The final empirical results show that each dimension of the GMSS system has a significant impact on the SMCL. In terms of the role of the government, the degree of land financial dependence is positively correlated with the SMCL rate, but it is not significant. For every 1% increase in the land financial dependence level, the SMCL rate increases by 0.022%. This indicates that the motives of land transfer in various regions of Shandong cannot influence the scale of construction land allocation by higher-level governments in the region, and it shows the policy rigidity of the tightening construction land supply adopted by the Chinese government to ensure food security and prevent the tendency of urban real estate (Fan et al., 2021; Huang et al., 2022). The degree of government intervention has a significant positive impact on the SMCL rate, and its elasticity coefficient reaches 1.728, indicating that although the government cannot determine the scale of available construction land, local governments can greatly affect the utilization efficiency of urban construction land and exacerbate the misallocation contradiction, such as formulating local industrial policies, adjusting the supply price and supply structure of construction land, and other intervention measures, which is consistent with the conclusions of most studies (Cheng et al., 2022).
In terms of market power, the proportion of the secondary industry has a significant positive correlation with the SMCL rate, with an elasticity coefficient of 0.63. The fundamental reason is China’s low-priced industrial land policy to implement the industrialization strategy, which will inevitably lead to the basic fact that the higher the proportion of the secondary industry, the higher the SMCL rate. However, compared with the results in some studies, the proportion of the secondary industry and the SMCL rate have no significant correlation, or even a significant negative correlation, which is obviously more in line with theoretical logic and practical experience (Huang and Du, 2017). Of course, the significant positive correlation between the two also means that more attention should be paid to the balance between the industrialization strategy and the improvement of the comprehensive output efficiency of urban construction land, especially to curb the extensive use of construction land in the secondary industry to achieve sustainable urban development under the constraints of land resources. At the same time, the growth of the regional credit scale has a significant aggravating effect on the SMCL level, indicating that a loose credit environment will lower the market entry threshold to a certain extent, resulting in inefficient land use. The urbanization rate is significantly negatively correlated with the SMCL rate. This indicates that it can help the agglomeration-based economic development model to improve the efficiency and vitality of economic operations by promoting mechanisms such as the sharing of infrastructure and service facilities, accelerating knowledge spillover, and reducing information asymmetry, thereby improving the SMCL.
In terms of social factors, the regional consumption capacity is significantly negatively correlated with the SMCL rate, and the deviation correction effect coefficient reaches −0.135. It is confirmed that social consumption can force enterprises to continuously transform and upgrade from the demand side, which helps to improve the mismatch of construction land and promotes the optimization of regional industrial structure and high-quality economic development. In addition, the level of export-oriented economic development is an important manifestation of social openness. It also helps to significantly improve the SMCL level, and its improvement coefficient reaches −0.147.
In terms of support systems, the level of scientific and technological development is negatively correlated, with the SMCL rate. This shows that technological innovation can help to improve the output of enterprises’ construction land. Infrastructure construction can significantly reduce the efficiency of market operations and transaction costs, thereby improving the SMCL, with the improvement coefficients reaching −0.045 and −0.284, respectively.
Overall, except that the impact of land financial dependence is not in line with the expected judgment, other factors have played a significant role, thus confirming the validity of the GMSS analytical framework. Among the factors that exacerbate the misallocation of resources, the influence of local government intervention is the strongest, followed by the proportion of the secondary industry, and the influence of the financial credit scale is the weakest. Among the factors with corrective effects, urbanization has the strongest improvement effect, followed by road network density, openness to the outside world, consumption vitality, and level of technological innovation. Although the specific effects of different indicators cannot be comprehensively calculated based on the numerical distribution characteristics of the regression coefficients in each dimension, government power is the biggest reason for the SMCL in Shandong, followed by market power, and social power is still relatively weak. Owing to its diverse composition, the support system may exhibit a significant impact strength difference. However, transportation infrastructure has a stronger influence as a physical support system, and the level of scientific and technological innovation, as a soft factor, has a weak influence, which is in line with the actual situation of the current development of the Shandong’s industrialization stage (Tan et al., 2021).
4.3 Robustness Test
To ensure the robustness of the measurement results, we choose the feasible general least squares model and mixed effects model for the test. The results show that the role and nature of each variable are completely consistent with the original model, which confirms the reliability of the analysis conclusion of each contributing factor. In particular, except for the weakening of the influence coefficient of the support system in the mixed effects model, the remaining variables still maintain the basic conclusion that the government has the most vital role, the market power is second, the social force is weak, and the support system exhibits type differentiation. This provides a credible empirical basis for the analysis of the contributing factors of the SMCL and subsequent governance.
5 Heterogeneity Analysis
5.1 Temporal Heterogeneity Analysis
To study the changes in the effect of various factors on the SMCL at different stages, we used 2010 as the split point and regressed the data from 2001 to 2010 and 2011 to 2019, respectively. The results are presented in Table 2.
The results show that compared with the previous stage, the fitting efficiency of the model is significantly improved, indicating that the explanatory power of the GMSS analytical framework for the SMCL in Shandong is further enhanced. In particular, the intensification of the misallocation in the role of the government has weakened, the promoting role of market factors and social factors has increased, and the role of the support system has remained relatively stable, but compared with each other, the situation has not essentially changed.
Specifically, in terms of the role of the government, from 2011 to 2019, the impact of Shandong’s land financial dependence on the SMCL has changed from an insignificant to a significant positive impact, which should be related to Shandong’s active implementation of the policy, which means consolidation of fragmented rural land to shift newly obtained quotas to urban districts in the same region. Originally, this policy is in line with the industrialization and urbanization trends of Shandong, which is an effective way to improve the utilization efficiency of regional construction land. However, to a certain extent, it has also become a local government strategy that can prevent the national reduction in the scale of new construction land, which has exacerbated the degree of the SMCL (Chien, 2015). The weakening of the influence of government intervention on the SMCL rate should be related to the market-oriented reform of land transactions in China. During this period, the government paid more attention to the role of market supply and demand in the allocation of factors, and the proportion of construction land transferred by agreement decreased. However, an increasing number of market-oriented allocation methods, such as bidding, listing, and auction, have been adopted, which has slightly alleviated the distortion of construction land allocation.
In terms of market factors, the role of the industrial structure has weakened, and the roles of urbanization and financial credit have increased significantly. This is related to the rapid development of the tertiary industry and the industrial evolution trend that gradually exceed the proportion of the secondary industry in Shandong. In addition, in recent years, the development of emerging industries, such as big data and information technology, has accelerated, and the output efficiency of unit construction land in the secondary industry has significantly improved. Therefore, the negative contributions to the SMCL tend to be moderate. In 2011, the level of urbanization in Shandong exceeded 50%, the agglomeration economic effect produced by urbanization was released faster, and the improvement effect on the SMCL was also more obvious. Simultaneously, China issued an economic stimulus plan in response to the 2008 global financial crisis. The loose financing environment has enabled the rapid development of local traditional industries and backward production capacity and has aggravated the impact on the SMCL.
In terms of the social role, the correcting effect of the degree of opening to the outside world on the SMCL rate has weakened. This is related to the accelerated improvement of the socially open environment brought about by China’s accession to the World Trade Organization in 2001 and the decline of marginal benefits in the later period. Reflecting that with the improvement in people’s income level, the promotion effect of the vitality of private development on the utilization of construction land has been further enhanced.
The influence of the support system has not changed much, indicating that China’s market-oriented reform has entered a critical period. The optimization relying on the external forces of the regional allocation of construction land has entered a stable stage, and in the future it will need to rely more on the changes in the system itself.
5.2 Regional Heterogeneity Analysis
According to the overall pattern of the regional economy in Shandong, the study area is divided into JNR, JDR, and LNR. Regression analysis is performed on three regions, and the results are shown in Table 3.
The heterogeneity of the GMSS system on the SMCL in the three regions is complex. Among them, the government’s reliance on land finance exacerbates the misallocation in LNR and JNR. However, there is a certain misallocation improvement effect in JDR. This shows that economically developed areas are more inclined to adopt the strategy of sorting and excavating the potential of existing urban construction land, thereby helping to improve their SMCL level. The level of government intervention in urban development has a significant positive impact on all the three regions, consistent with the previous discussion in this study, but the influence coefficient in LNR is as high as 4.43, reflecting that government intervention in economically underdeveloped areas is an extremely important reason for the SMCL.
Among the market power factors, the proportion of the secondary industry still played a significant positive role in increasing the misallocation, but the role was weaker in regions with higher levels of economic development. This is consistent with the fact that the secondary industry’s development level is relatively high, and the output efficiency of construction land is relatively more robust in economically developed areas. The level of urbanization is similar to this. The high level of urbanization in economically developed areas has a more apparent improvement effect on the SMCL, which reflects the necessity of continuing to accelerate the upgrading of the industrial structure and the urbanization process. The impact of the local financial environment is greatest in JNR, which is related to the centralized distribution of Chinese financial institutions in the provincial capital city as the administrative center and easy access to financial resources.
However, although the effects of the social force factors in the three regions are all improvement effects, the regression results are not significant. Among them, the regression coefficients of social openness and social consumption level in LNR are the smallest, indicating that although the social openness and social vitality of the underdeveloped areas are low, they tend to form stronger marginal improvement contributions because of their weak foundation.
In terms of the influence of the support system, the power of science and technology has a significant and the strongest improvement effect on JNR. This shows that the provincial capital has gathered a large number of educational technology resources due to its status as an administrative center and has formed a spillover effect on the surrounding areas through close-range scientific and technological cooperation, such that the role of scientific and technological innovation can be effectively played. The level of infrastructure construction represented by highway density has the strongest effect on the improvement of construction land mismatch in LNR, but it is not significant, which shows that infrastructure construction in LNR is still relatively lagging behind; thus, it has stronger improvement elasticity. However, while infrastructure improvement accelerates the flow of factors and alleviates the SMCL, the imperfect system and mechanism of promoting high-quality economic development in LNR may also lead to the transfer of low-end industries to the local area and aggravate the misallocation of land use. Therefore, although the improvement of infrastructure has a positive effect on improving the SMCL in LNR, it is clear that this effect is not sufficiently robust.
In general, the effects of various dimensions of the GMSS system in each region are still in line with theoretical expectations. Owing to the level of economic development and its structural characteristics, the role of the government is stronger and the role of market forces in promoting adaptation is lower than in backward regions, while social forces and support systems may have a stronger potential for improving misallocation. However, owing to the constraints of various factors, such as the imperfect system and mechanism, the degree of its role is not sufficiently stable.
6 Conclusion
Spatial misallocation among regions is an important contributor to the inefficient use of construction land. This study quantitatively measures and analyzes the spatial and temporal characteristics of the SMCL in Shandong, China. It also proposes and empirically tests the actual effects of the factors affecting the SMCL and its dynamic changes under the GMSS analytical framework. The main conclusions of this study are presented as follows:
(1) The calculation results based on the principle of equal marginal output can more accurately reflect the nature and intensity of the SMCL in the study area. During the study period, the urban construction land in the study area grows rapidly, but the overall level shows a downward trend. In most areas, the urban construction land significantly approaches the adaptation range. Overall, industrialization and urbanization remain as evident driving forces for local governments to compete for construction land resources. Cities with a moderate or low level of economic development, regions with a high proportion of agriculture or traditional industries, and regions with slower rates of industrialization and urbanization have a higher probability of shortage misallocation. The spatial pattern of the SMCL level in Shandong shifts from a numerical combination of the middle corridor and the north–south wings to a numerical combination of the central city as the core and the interlaced distribution of other regions.
(2) With the gradual improvement in developing countries’ economic and social development levels, government forces, market forces, social forces, and their supporting systems will form a multi-agent symbiosis and coevolution regulation system. Under the management and control framework of the superior government, it adjusts the timing, structure, and efficiency of construction land utilization, which ultimately affects the SMCL status.
(3) The empirical results confirm the validity of the GMSS analytical framework for the contributing factors of the SMCL. Among them, government power not only aggravates the contradiction of the SMCL but also has the strongest effect. The role of social forces remains weak, and the nature and strength of the support system are internally differentiated. However, with the changes in the development stage, the intensification of misallocation in the role of the government has weakened, the role of market and social factors has been enhanced, and the role of the support system has remained relatively stable. However, the comparative situation has not fundamentally changed. The influence of different dimensions on the heterogeneity of the SMCL in the three major regions is more complex. In general, the role of the government in backward regions is stronger, the role of market forces in adaptation promotion is lower, and social forces and supporting systems are not sufficiently steady.
The aforementioned conclusions not only have various policy implications for Shandong but also have rich reference values for other regions with rapid growth of construction land and significant internal differences. First, the fact that construction land in Shandong is growing rapidly but the level of SMCL is declining is closely related to the continuous promotion of the systematic reform of construction land allocation models in China and Shandong in recent years. This shows that in the future, relative regions should accelerate the construction of a policy environment, in which market and social forces can effectively participate in the allocation of construction land and constantly improve the mechanism design for multi-agents to play a coordinated role to avoid the risk of misallocation caused by improper government intervention. Second, the SMCL level is significantly related to factors such as the regional development level, industrial structure, spatial structure of agglomeration, and completeness of supporting systems. This means that the regional allocation of construction land should be fully integrated with the development characteristics of different regions. Nevertheless, it also reminds all regions to continuously improve their technological innovation system and infrastructure construction, actively promote the layout optimization of their industrial and spatial structures, and accelerate the transformation to high-quality development. Third, the process of improving the spatial misallocation of construction land in most regions is unstable and there may be large or small fluctuations. This requires that each region should also strengthen its statistical analysis, monitoring and early warning, and dynamic policy adjustment of construction land allocation to respond in a timely manner to the risk of loss of economic and social benefits caused by improper coordination of various dimensional factors.
Because the measurement method used in this study is a statistical estimation based on the ideal state, the measurement results may deviate from the actual situation in different regions. In addition, there are many contributing factors involved in the SMCL; therefore, this study cannot include more variables for a more systematic investigation, and the conclusions obtained also have certain limitations. Undoubtedly, the measurement ideas, analytical framework, and new discoveries of this study have theoretical and policy significance for understanding the evolution law of the SMCL pattern in rapid industrialization and urbanization areas, constructing a better regulatory framework, and improving the utilization efficiency of construction land (Table 4, Table 5, Table 6, Table 7).
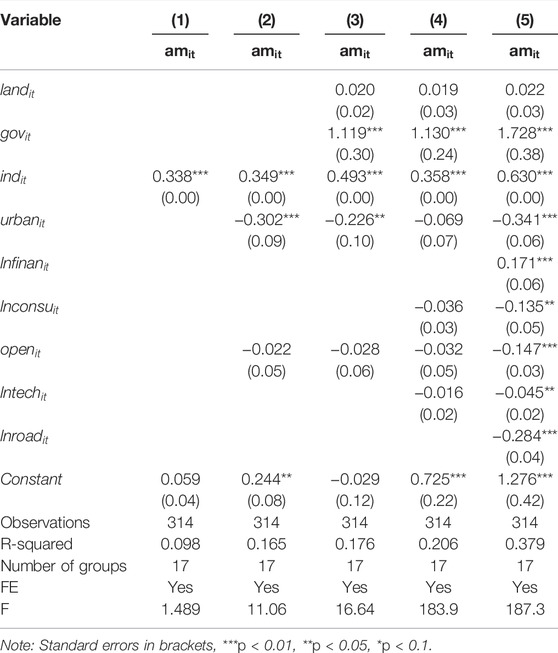
TABLE 6. Stepwise regression results of the econometric model for the effects of multidimensional contributing factors of the SMCL.
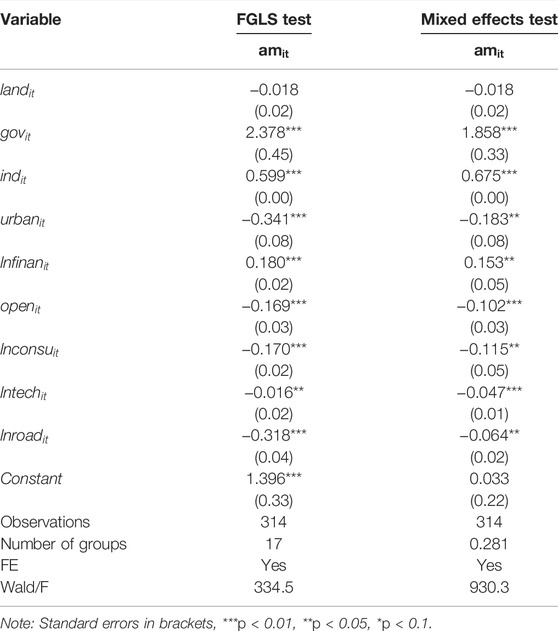
TABLE 7. Robustness test of the econometric regression results via the FGLS model and mixed effects model.
Data Availability Statement
Publicly available datasets are analyzed in this study. This data can be found here: https://data.cnki.net/, http://tjj.shandong.gov.cn/.
Author Contributions
All authors contributed to the study conception and design. Conceptualization, SL and HC; methodology, SL and HC; software, HC and XW; validation, SL, HC, and XW; formal analysis, SL and HC; investigation, JW; resources, SL and HC; data curation, HC and XW; writing—original draft preparation, SL and HC; writing—review and editing, SL, HC, and XW; supervision, SL; project administration, SL. All authors read and approved the final manuscript.
Funding
This work was supported by the Natural Science Fund General Project of Shandong Province (Grant Number ZR2020MD010) and Future Plan for Young Scholars of Shandong University.
Conflict of Interest
The authors declare that the research was conducted in the absence of any commercial or financial relationships that could be construed as a potential conflict of interest.
Publisher’s Note
All claims expressed in this article are solely those of the authors and do not necessarily represent those of their affiliated organizations, or those of the publisher, the editors, and the reviewers. Any product that may be evaluated in this article, or claim that may be made by its manufacturer, is not guaranteed or endorsed by the publisher.
Acknowledgments
We would like to thank editors, reviewers, and the National Natural Science Foundation of Shandong Province.
References
Aoki, S. (2012). A Simple Accounting Framework for the Effect of Resource Misallocation on Aggregate Productivity. J. Jpn. Int. Econ. 26 (4), 473–494. doi:10.1016/j.jjie.2012.08.001
Aumpon, S. D. (2018). Resource Misallocation and Rice Productivity in Thailand. Montenegrin J. Econ. 14 (2), 143–153. doi:10.14254/1800-5845/2018.14-2.9
Banerjee, A. V., and Moll, B. (2010). Why Does Misallocation Persist? Am. Econ. J. Macroecon. 2 (1), 189–206. doi:10.1257/mac.2.1.189
Baross, P., and Mesa, N. (1986). From Land Markets to Housing Markets. Habitat Int. 10 (3), 153–170. doi:10.1016/0197-3975(86)90047-0
Bo, S. (2020). Centralization and Regional Development: Evidence from a Political Hierarchy Reform to Create Cities in China. J. Urban Econ. 115, 103182. doi:10.1016/j.jue.2019.06.005
Brandt, L., Tombe, T., and Zhu, X. (2013). Factor Market Distortions across Time, Space and Sectors in China. Rev. Econ. Dyn. 16 (1), 39–58. doi:10.1016/j.red.2012.10.002
Breton, A. (1996). Competitive Governments: An Economic Theory of Politics and Public Finance. Cambridge University Press.
Britos, B., Hernandez, M. A., Robles, M., and Trupkin, D. R. (2022). Land Market Distortions and Aggregate Agricultural Productivity: Evidence from Guatemala. J. Dev. Econ. 155, 102787. doi:10.1016/j.jdeveco.2021.102787
Calic, G., and Ghasemaghaei, M. (2021). Big Data for Social Benefits: Innovation as a Mediator of the Relationship between Big Data and Corporate Social Performance. J. Bus. Res. 131, 391–401. doi:10.1016/j.jbusres.2020.11.003
Chen, B., and Lin, J. Y. (2021). Development Strategy, Resource Misallocation and Economic Performance. Struct. Change Econ. Dyn. 59, 612–634. doi:10.1016/j.strueco.2021.10.003
Chen, C. (2017). Untitled Land, Occupational Choice, and Agricultural Productivity. Am. Econ. J. Macroecon. 9 (4), 91–121. doi:10.1257/mac.20140171
Chen, C., Restuccia, D., and Santaeulàlia-Llopis, R. (2021). The Effects of Land Markets on Resource Allocation and Agricultural Productivity. Rev. Econ. Dyn. 45, 41–54. doi:10.1016/j.red.2021.04.006
Chen, Y., Chen, A., and Zhang, D. (2022). Evaluation of Resources and Environmental Carrying Capacity and its Spatial-Temporal Dynamic Evolution: A Case Study in Shandong Province, China. Sustain. Cities Soc. 82, 103916. doi:10.1016/j.scs.2022.103916
Cheng, Y., Jia, S., and Meng, H. (2022). Fiscal Policy Choices of Local Governments in China: Land Finance or Local Government Debt? Int. Rev. Econ. Finance 80, 294–308. doi:10.1016/j.iref.2022.02.070
Chien, S.-S. (2015). Local Farmland Loss and Preservation in China-A Perspective of Quota Territorialization. Land Use Policy 49, 65–74. doi:10.1016/j.landusepol.2015.07.010
Dai, X., and Cheng, L. (2019). Aggregate Productivity Losses from Factor Misallocation across Chinese Manufacturing Firms. Econ. Syst. 43 (1), 30–41. doi:10.1016/j.ecosys.2018.08.006
Dheera-Aumpon, S. (2014). Misallocation and Manufacturing TFP in Thailand. Asia Pac Econ. Lit. 28 (2), 63–76. doi:10.1111/apel.12073
Du, J., and Peiser, R. B. (2014). Land Supply, Pricing and Local Governments' Land Hoarding in China. Regional Sci. Urban Econ. 48, 180–189. doi:10.1016/j.regsciurbeco.2014.07.002
Estoque, R. C., Ooba, M., Togawa, T., Hijioka, Y., and Murayama, Y. (2021). Monitoring Global Land-Use Efficiency in the Context of the UN 2030 Agenda for Sustainable Development. Habitat Int. 115, 102403. doi:10.1016/j.habitatint.2021.102403
Fan, J., Zhou, L., Yu, X., and Zhang, Y. (2021). Impact of Land Quota and Land Supply Structure on China's Housing Prices: Quasi-Natural Experiment Based on Land Quota Policy Adjustment. Land Use Policy 106, 105452. doi:10.1016/j.landusepol.2021.105452
Fang, L., and Tian, C. (2020). Construction Land Quotas as a Tool for Managing Urban Expansion. Landsc. Urban Plan. 195, 103727. doi:10.1016/j.landurbplan.2019.103727
Fu, S., Xu, X., and Zhang, J. (2021). Land Conversion across Cities in China. Regional Sci. Urban Econ. 87, 103643. doi:10.1016/j.regsciurbeco.2021.103643
Gao, X., Shi, X., and Fang, S. (2021). Property Rights and Misallocation: Evidence from Land Certification in China. World Dev. 147, 105632. doi:10.1016/j.worlddev.2021.105632
Guanchun Liu, G., Liu, Y., and Zhang, C. (2018). Factor Allocation, Economic Growth and Unbalanced Regional Development in China. World Econ. 41 (9), 2439–2463. doi:10.1111/twec.12572
Han Wang, H., He, C., Li, W., Nie, X., Zhong, H., and Wen, L. (2020). Will Transferable Development Rights (TDR) Increase Regional Economic Imbalance? - A Quota Transaction Case of Cultivated Land Conversion and Reclamation in Guangxi, China. Habitat Int. 104, 102254. doi:10.1016/j.habitatint.2020.102254
Harrison, A. (1982). Land Policies in the Member States of the European Community. Agric. Adm. 11 (3), 159–174. doi:10.1016/0309-586x(82)90113-3
Hjalager, A.-M., Staunstrup, J. K., Sørensen, M. T., and Steffansen, R. N. (2022). The Densification of Second Home Areas - Sustainable Practice or Speculative Land Use? Land Use Policy 118, 106143. doi:10.1016/j.landusepol.2022.106143
Hsieh, C.-T., and Klenow, P. J. (2009). Misallocation and Manufacturing TFP in China and India*. Q. J. Econ. 124 (4), 1403–1448. doi:10.1162/qjec.2009.124.4.1403
Huang, X., Wang, H., and Xiao, F. (2022). Simulating Urban Growth Affected by National and Regional Land Use Policies: Case Study from Wuhan, China. Land Use Policy 112, 105850. doi:10.1016/j.landusepol.2021.105850
Huang, Z., and Du, X. (2017). Government Intervention and Land Misallocation: Evidence from China. Cities 60, 323–332. doi:10.1016/j.cities.2016.10.006
Hui Wang, H., Qiao, L., Tian, C., and Lin, Q. (2020). Utilisation Efficiency of Construction Land in China's Coastal Cities Based on Debt Level. Complexity 2020, 1–10. doi:10.1155/2020/3428968
Jian Wang, J., Wu, Q., Yan, S., Guo, G., and Peng, S. (2020). China's Local Governments Breaking the Land Use Planning Quota: A Strategic Interaction Perspective. Land Use Policy 92, 104434. doi:10.1016/j.landusepol.2019.104434
Kimura, S., Otsuka, K., Sonobe, T., and Rozelle, S. (2011). Efficiency of Land Allocation through Tenancy Markets: Evidence from China. Econ. Dev. Cult. Change 59 (3), 485–510. doi:10.1086/649639
Kissinger, G., Gupta, A., Mulder, I., and Unterstell, N. (2019). Climate Financing Needs in the Land Sector under the Paris Agreement: An Assessment of Developing Country Perspectives. Land Use Policy 83, 256–269. doi:10.1016/j.landusepol.2019.02.007
Kollintzas, T., Papageorgiou, D., and Vassilatos, V. (2018). Market and Political Power Interactions in Greece: A Theory. Eur. J. Political Econ. 53, 59–83. doi:10.1016/j.ejpoleco.2017.07.003
Li, D., Chen, Y., and Miao, J. (2022). Does ICT Create a New Driving Force for Manufacturing?-Evidence from Chinese Manufacturing Firms. Telecommun. Policy 46 (1), 102229. doi:10.1016/j.telpol.2021.102229
Li, L., Huang, P., and Ma, G. (2016). Misallocation of Land Resources and Productivity Differences of Chinese Industrial Enterprises. Manag. World (08), 86–96. (in Chinese). doi:10.19744/j.cnki.11-1235/f.2016.08.008
Lin, C. L., Chan, E. H. W., and Chiang, W.-H. (2022). Urban Renewal Governance and Manipulation of Plot Ratios: A Comparison between Taipei, Hong Kong and, Singapore. Land Use Policy 119, 106158. doi:10.1016/j.landusepol.2022.106158
Liu, R., and Wong, T.-C. (2015). The Allocation and Misallocation of Economic Housing in Beijing: Target Groups versus Market Forces. Habitat Int. 49, 303–315. doi:10.1016/j.habitatint.2015.06.002
Liu, Y., Liu, G., and Zhang, C. (2021). Local Land Supply and Fiscal Incentives for R&D: Evidence from a Quasi-Natural Experiment in China. China Econ. Rev. 68, 101630. doi:10.1016/j.chieco.2021.101630
Lu, M., and Zhang, X. (2019). Towards an Intelligent Country: China's Higher Education Expansion and Rural Children's Senior High School Participation. Econ. Syst. 43 (2), 100694. doi:10.1016/j.ecosys.2019.100694
Lu, Q., Liang, F., Bi, X., Duffy, R., and Zhao, Z. (2011). Effects of Urbanization and Industrialization on Agricultural Land Use in Shandong Peninsula of China. Ecol. Indic. 11 (6), 1710–1714. doi:10.1016/j.ecolind.2011.04.026
Lu, X., Zhang, Y., Lin, C., and Wu, F. (2021). Analysis and Comprehensive Evaluation of Sustainable Land Use in China: Based on Sustainable Development Goals Framework. J. Clean. Prod. 310, 127205. doi:10.1016/j.jclepro.2021.127205
Luo, J., Wu, Y., Choguill, C. L., and Zhang, X. (2022). A Study on Promoting the Intensive Use of Industrial Land in China through Governance: A Game Theoretical Approach. J. Urban Manag. (In Press). doi:10.1016/j.jum.2022.02.003
Mendoza-Ponce, A., Corona-Núñez, R. O., Nava, L. F., Estrada, F., Calderón-Bustamante, O., Martínez-Meyer, E., et al. (2021). Impacts of Land Management and Climate Change in a Developing and Socioenvironmental Challenging Transboundary Region. J. Environ. Manag. 300, 113748. doi:10.1016/j.jenvman.2021.113748
Midrigan, V., and Xu, D. Y. (2014). Finance and Misallocation: Evidence from Plant-Level Data. Am. Econ. Rev. 104 (2), 422–458. doi:10.1257/aer.104.2.422
Olley, G. S., and Pakes, A. (1996). The Dynamics of Productivity in the Telecommunications Equipment Industry. Econometrica 64 (6), 1263–1297. doi:10.2307/2171831
Opp, M. M., Parlour, C. A., and Walden, J. (2014). Markup Cycles, Dynamic Misallocation, and Amplification. J. Econ. Theory 154, 126–161. doi:10.1016/j.jet.2014.09.001
Pal, S. C., Chakrabortty, R., Roy, P., Chowdhuri, I., Das, B., Saha, A., et al. (2021). Changing Climate and Land Use of 21st Century Influences Soil Erosion in India. Gondwana Res. 94, 164–185. doi:10.1016/j.gr.2021.02.021
Qu, Y., Zhan, L., Jiang, G., Ma, W., and Dong, X. (2021). How to Address "Population Decline and Land Expansion (PDLE)" of Rural Residential Areas in the Process of Urbanization:A Comparative Regional Analysis of Human-Land Interaction in Shandong Province. Habitat Int. 117, 102441. doi:10.1016/j.habitatint.2021.102441
Restuccia, D., and Rogerson, R. (2017). The Causes and Costs of Misallocation. J. Econ. Perspect. 31 (3), 151–174. doi:10.1257/jep.31.3.151
Shenoy, A. (2017). Market Failures and Misallocation. J. Dev. Econ. 128, 65–80. doi:10.1016/j.jdeveco.2017.05.004
Shi, X. (2022). Land Reallocation Responses to China's New Rural Pension Scheme: Evidence from a Regression Discontinuity Design. J. Rural Stud. 92, 35–49. doi:10.1016/j.jrurstud.2022.03.018
Shuhong Wang, S., Zhao, D., and Chen, H. (2020). Government Corruption, Resource Misallocation, and Ecological Efficiency. Energy Econ. 85, 104573. doi:10.1016/j.eneco.2019.104573
Silal, P., and Saha, D. (2021). Impact of National E-Participation Levels on Inclusive Human Development and Environmental Performance: The Mediating Role of Corruption Control. Gov. Inf. Q. 38 (4), 101615. doi:10.1016/j.giq.2021.101615
Song, M., Ma, X., Shang, Y., and Zhao, X. (2020). Influences of Land Resource Assets on Economic Growth and Fluctuation in China. Resour. Policy 68, 101779. doi:10.1016/j.resourpol.2020.101779
Song, Y., Yeung, G., Zhu, D., Xu, Y., and Zhang, L. (2022). Efficiency of Urban Land Use in China's Resource-Based Cities, 2000-2018. Land Use Policy 115, 106009. doi:10.1016/j.landusepol.2022.106009
Tan, R., Zhang, T., Liu, D., and Xu, H. (2021). How Will Innovation-Driven Development Policy Affect Sustainable Urban Land Use: Evidence from 230 Chinese Cities. Sustain. Cities Soc. 72, 103021. doi:10.1016/j.scs.2021.103021
Tang, M., Li, Z., Hu, F., and Wu, B. (2020). How Does Land Urbanization Promote Urban Eco-Efficiency? the Mediating Effect of Industrial Structure Advancement. J. Clean. Prod. 272, 122798. doi:10.1016/j.jclepro.2020.122798
Wang, S., Tan, S., Yang, S., Lin, Q., and Zhang, L. (2019). Urban-Biased Land Development Policy and the Urban-Rural Income Gap: Evidence from Hubei Province, China. Land Use Policy 87, 104066. doi:10.1016/j.landusepol.2019.104066
Wen, L., Chatalova, L., and Zhang, A. (2022). Can China's Unified Construction Land Market Mitigate Urban Land Shortage? Evidence from Deqing and Nanhai, Eastern Coastal China. Land Use Policy 115, 105996. doi:10.1016/j.landusepol.2022.105996
Wu, Y. (2016). China ’S Capital Stock Series by Region and Sector. Res. Article 11 (1), 156–172. doi:10.3868/s060-005-016-0010-5
Wu, J., Li, N., and Shi, P. (2014). Benchmark Wealth Capital Stock Estimations across China's 344 Prefectures: 1978 to 2012. China Econ. Rev. 31, 288–302. doi:10.1016/j.chieco.2014.10.008
Wu, J., Wu, Y., and Wang, B. (2018). Local Government Debt, Factor Misallocation and Regional Economic Performance in China. China & World Econ. 26 (4), 82–105. doi:10.1111/cwe.12250
Wu, Y., Heerink, N., and Yu, L. (2020). Real Estate Boom and Resource Misallocation in Manufacturing Industries: Evidence from China. China Econ. Rev. 60, 101400. doi:10.1016/j.chieco.2019.101400
Xie, Q., Yang, L., Liu, G., and Wang, H. (2022). Study on Misallocation and Benefit Loss of Urban Construction Land in Guangdong. Res. Land Nat. Resour. 2022 (02), 1–6. (in Chinese). doi:10.16202/j.cnki.tnrs.2022.02.001
Yang, C.-H., and Lee, W.-C. (2021). Establishing Science Parks Everywhere? Misallocation in R&D and its Determinants of Science Parks in China. China Econ. Rev. 67, 101605. doi:10.1016/j.chieco.2021.101605
Yansui Liu, Y., Zhang, Z., and Zhou, Y. (2018). Efficiency of Construction Land Allocation in China: An Econometric Analysis of Panel Data. Land Use Policy 74, 261–272. doi:10.1016/j.landusepol.2017.03.030
Zhang, J., Wang, C., Xu, L., and Weng, Y. (2021). Spatiotemporal Characteristics, Driving Mechanisms and Spatial Effects of China's Construction Land Mismatch: An Empirical Analysis Based on 235 Cities. Trop. Geogr. 41 (02), 217–228. (in Chinese). doi:10.13284/j.cnki.rddl.003327
Keywords: spatial misallocation of construction land, equal marginal output, GMSS analytical framework, influencing factors, Shandong, China
Citation: Li S, Cao H, Wang J and Wang X (2022) Assessment and Contributing Factors of the Spatial Misallocation of Construction Land: A Case Study of Shandong, China. Front. Environ. Sci. 10:957013. doi: 10.3389/fenvs.2022.957013
Received: 30 May 2022; Accepted: 14 June 2022;
Published: 13 July 2022.
Edited by:
Yanbo Qu, Shandong University of Finance and Economics, ChinaReviewed by:
Kai Liu, Shandong Normal University, ChinaXiaosong Tu, Jiangxi University of Finance and Economics, China
Helin Liu, Huazhong University of Science and Technology, China
Copyright © 2022 Li, Cao, Wang and Wang. This is an open-access article distributed under the terms of the Creative Commons Attribution License (CC BY). The use, distribution or reproduction in other forums is permitted, provided the original author(s) and the copyright owner(s) are credited and that the original publication in this journal is cited, in accordance with accepted academic practice. No use, distribution or reproduction is permitted which does not comply with these terms.
*Correspondence: Shaoxing Li, bGlzeEBzZHUuZWR1LmNu
†These authors have contributed equally to this work and share first authorship