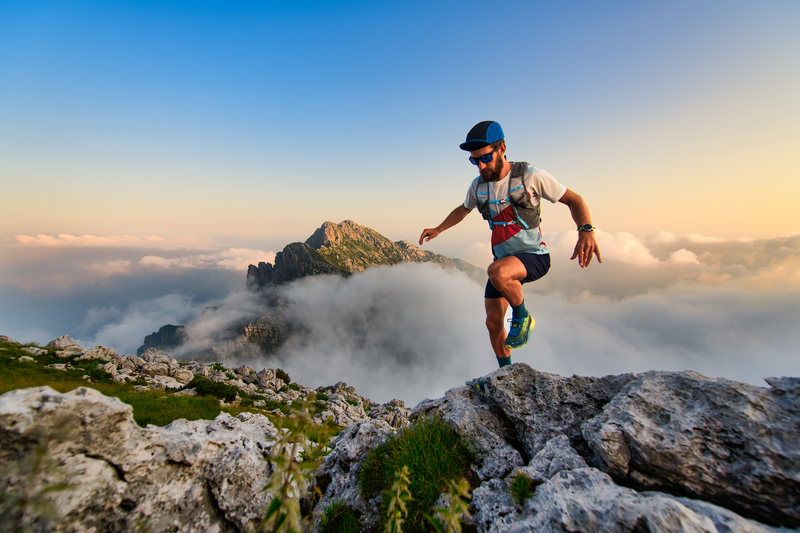
94% of researchers rate our articles as excellent or good
Learn more about the work of our research integrity team to safeguard the quality of each article we publish.
Find out more
ORIGINAL RESEARCH article
Front. Environ. Sci. , 18 October 2022
Sec. Environmental Economics and Management
Volume 10 - 2022 | https://doi.org/10.3389/fenvs.2022.955954
This article is part of the Research Topic The Nexus between Innovation and Environmental Sustainability View all 11 articles
In order to promote the green transformation of agricultural development, we used a partial linear function coefficient panel model to measure the impact of environmental regulations in 30 provinces and cities in China on agricultural green technology innovation and agricultural green total factor productivity. The advantage of this model is that it can take into account the heterogeneity of regional economic development levels, that is, by introducing variables that are functions of regional economic development levels as coefficients of environmental regulation. The research results show that: when the level of regional economic development is low, environmental regulation has a limited impact on agricultural green technology innovation and agricultural green total factor productivity, but as the level of regional economic development gradually increases, environmental regulation has a more significant impact on the two. And environmental regulation has a greater impact on agricultural green total factor productivity than on agricultural green technology innovation. Based on the research results, policy recommendations are suggested.
The rapid development of China’s agriculture is also accompanied by the emergence of environmental problems (Zhou and Li, 2021). An article in China Guangming Daily reported, The Institute of Geographical Sciences and Natural Resources Research released the research results of its Key Laboratory of Land Surface Pattern and Simulation (Xu et al., 2020), there is a 21.49 percent soil heavy metal spot in the main grain-producing areas of China, according to the Chinese Academy of Sciences. There were 13.97% of moderate pollution, 2.50% of heavy pollution, and 5.02% of very heavy pollution. Considering that soil pollution is often irreversible, the future cost of treatment and ecological pressure will be considerable. In this context, over the course of the “13th Five-Year Plan,” the word “green” was identified as the “development concept” for the first time, and one of the key sectors to achieve green growth Agriculture and rural areas are the top priority. General Secretary Xi Jinping has also repeatedly emphasized the need to fully realize the green transformation of agricultural development. In September 2021, China’s first special plan for agricultural green development was issued by six national ministries and commissions, the “14th Five-Year Plan for National Agricultural Green Development,” Showing China’s determination and courage to fully realize the comprehensive green transformation of agricultural development. At present, China’s economy has progressed from a stage of high-speed growth to a stage of high-quality development (Hao et al., 2021; Rauf et al., 2021; Abbasi et al., 2022). China has been highly valued by government departments in terms of green development. Figure 1 is a summary of the environmental vocabulary that appeared in the work reports of the provincial governments in China from 2009 to 2019. Low carbon, “carbon dioxide,” “sulfur dioxide,” “chemical oxygen demand,” “environmental protection,” “environmental protection,” “emission reduction,” “air,” “green,” “energy consumption,” “pollution,” “Ecology,” “Pollution,” a total of 15. According to Figure 1, the country’s attention to the environment is increasing overall. While lamenting the country’s urgent attention to green development, it is impossible not to think deeply about how to achieve the coordinated development of economic growth and environmental protection (Du et al., 2021). Balsa-Barreiro et al. (2019) can help us realize how was coupled the economic model of China with the emissions, and how in the last years these factors were decoupled. In this study, the study examined the location of the centers of gravity of four basic indicators over the period 1960–2016: GDP, CO2 emissions, total population, and urban population. As an important sector of green development, agriculture and rural areas, in the event that agricultural economic growth and ecological environment are coordinated, the green transformation of China’s agricultural development relies heavily on environmental regulation.
FIGURE 1. The total number of environmental vocabularies in the work reports of the provincial governments from 2009 to 2019.
Many previous studies by scholars have confirmed the important role of environmental regulation in the environmental field, but most of the literature studies only focus on a single indicator, the role of environmental regulation in promoting agricultural development’s green transformation comes from agricultural green technology innovation and agricultural green total factor productivity (Yu et al., 2022). Therefore, the paper integrates environmental regulation, agricultural green technology innovation, and agricultural green total factor productivity into a research framework, which enriches the current research and is of great significance.
In agricultural production, green technology innovation reduces pollution emissions from fossil fuels and increases the use of clean energy. Green total factor productivity balances agricultural production efficiency without exceeding environmental carrying capacity, this indicator measures the transformation of China’s agricultural green development. However, environmental regulation is controversial in the research on agricultural green technology innovation and agricultural green total factor productivity. The specific manifestations are as follows.
At present, Academic circles disagree on the impact of environmental regulation on green innovation (Liu et al., 2022). Some scholars such as (Porter, 1991) believe that environmental regulation can enable enterprises to avoid penalties due to environmental problems, update technical equipment, and expand R&D investment. As a result, many scholars have confirmed the “Porter Hypothesis” (Pang et al., 2019; Yu et al., 2019; Zhou et al., 2019; Zhang et al., 2020; Xie et al., 2021). Several scholars believe that environmental regulation is important, it makes enterprises increase their investment in environmental inspections and fines at a limited cost, which will squeeze corporate resources, reduce corporate R&D investment, and inhibit regional green innovation (Gollop and Roberts, 1983; Grey and Shadbegian, 2003; Ambec et al., 2013; Li et al., 2019).
Among the many factors affecting agricultural green total factor productivity, one of the simplest and most straightforward ways to address the issue of excessive factor use and the externality of environmental damage is through environmental legislation. There is conflicting evidence in the literature regarding whether environmental regulation affects agricultural green total factor productivity, and if so, whether it has a positive or negative impact. According to some studies (Cochard et al., 2005; Shadbegian and Gray, 2005), environmental regulation will have a negative impact on agricultural green total factor productivity. However, according to other studies (Jorge et al., 2015; Ye et al., 2018), environmental regulation is conducive to improving agricultural green total factor productivity. Still other researchers noted that environmental regulation did not significantly affect agricultural green TFP (Liang et al., 2012a).
Secondly, most of the research literature on environmental regulation only regards environmental regulation as a control variable in the empirical test, and pays no attention to how the internal environmental regulation system affects the production of green total factor in agriculture; and the existing literature only uses linear regression. The model is used to test the relationship between the two. In fact, with the dynamic change of regulatory intensity, environmental regulations’ effects on agricultural green TFP may also exhibit nonlinear traits. However, both the linear regression model and the nonlinear model research may be biased. Therefore, this paper uses a partial linear function coefficient model that takes into account both linear and nonlinear characteristics to describe the method by which environmental legislation affects agricultural development’s transition to a more sustainable model.
In addition, restricted by the level of economic development, there may be regional variations in how environmental legislation affects the development of agricultural green technologies and the productivity of agricultural green total factors (Liu et al., 2021a). Specifically, Investment in resources is crucial for the development of agricultural green technology. For areas with low levels of economic growth, due to limited resources, innovation and research and development will face greater challenges. Conversely, there are more resources and expertise available for R&D in areas with higher economic development levels. In addition, agricultural machinery is a necessary material and equipment for agricultural modernization, but it will also cause energy consumption and increase agricultural carbon emissions due to its large-scale use. Therefore, in order to reduce pollution, ER will inevitably affect the efficiency of agricultural production. In areas with low economic development levels, enterprises producing these machines may be squeezed out of the market due to ER, It will ultimately have an impact on the increase in productivity of the green total factor in agriculture (Yu et al., 2022). Figure 2 depicts the evolution of the real PGDP per capita log distribution across Chinese provinces. As can be seen from Figure 2, the differences in the level of economic development among provinces are quite significant however, the level of regional economic development as a whole is rising. Therefore, considering the different levels of economic development in different provinces, In this research, the heterogeneity of ER’s effects on the development of agricultural green technologies and agricultural green total factor productivity will be examined (Yu et al., 2022).
The following is a summary of the study’s major contributions: First, using the two key indicators of agricultural green technology innovation and agricultural green total factor productivity (Yu et al., 2022), this paper thoroughly analyzes the impact of agricultural environmental regulation (ER) on the green development of agriculture in 30 provinces and cities in China (Zhou and Li, 2022), which contributes to more Clear and more comprehensive understanding of the important role of ER in the transition of agricultural development to green. This is not studied in the previous literature and enriches the current research. Secondly, this paper examines the hypothesis that whether the favorable Different levels of regional economic growth will have different effects on how environmental regulations affect the green transition of agricultural production, which is rarely studied in the current agricultural literature. Third, In order to examine the nonlinear link between ER and two green development indicators, this paper makes use of Mr. Du Kerui’s partial linear function coefficient panel model. The model can guard against model miscalculation and improve the accuracy of the estimation outcome.
The following describes the paper’s organizational structure: the introduction is the first section, which introduces the research background, etc.; in the second part, it summarizes the related literature of agricultural environmental regulation and its relationship with agricultural green technology innovation and agricultural green total factor productivity (Yu et al., 2022). Section 3 provides a description of the models, variables, and data sources used in this study. Analyses and empirical findings are presented in Section 4. Conclusion and policy repercussions are presented in Section 5.
Du et al. (2021) think that many scholars at home and abroad have confirmed the positive role of environmental regulation in the field of energy and environment. Such as green efficiency (Galloway and Johnson, 2016; Curtis and Lee, 2019; Wang et al., 2019; Su and Zhang, 2020; Du et al., 2021), carbon emissions (Zhao et al., 2020a; Zhao et al., 2020b; Wang and Wei, 2020; Du et al., 2021), Environmental regulation plays a beneficial role in the fields of energy and the environment, according to numerous academics domestically and internationally (Li et al., 2022). For instance, carbon emissions (Zhao et al., 2020a; Zhao et al., 2020b; Wang and Wei, 2020); green efficiency (Curtis and Lee, 2019; Wang et al., 2019; Su and Zhang, 2020; Du et al., 2021); energy efficiency (Liu et al., 2018).
In different studies, Different studies have come to different findings about how environmental regulation affects technological innovation. Many academics think that environmental regulation can encourage innovation. For instance, researchers (Brunnermeier and Cohen, 2003) demonstrate that greater environmental innovation will result from an increase in pollution reduction spending; (Turken et al., 2020) believes that due to the promotion effect of green technology, Industry should prioritize investing in green technology. However, some scholars believe that environmental regulation can only take effect under certain conditions. According to Borsatto and Amui (2019), there is no consistent connection between environmental legislation and green innovation. According to Song et al. (2018), businesses can only attempt to raise the caliber of their workforce and continue to pursue green innovation by putting strong environmental rules into place.
Based on the above literature research, it is evident that there is ongoing disagreement on the research finding regarding the impact of environmental regulation on the development of green technologies (Wang and Yan, 2022). However, for the green technology innovation in the agricultural field, this indicator has not been utilized by any academics to evaluate the success of agricultural green development.
The Chinese governments have developed a number of environmental regulation measures to achieve the green transformation of agricultural development in line with the idea of agricultural green development. (Picazo-Tadeo et al., 2011; Deng and Gibson, 2019; Liu et al., 2021b; Iqbal et al., 2021; Irfan et al., 2021; Jiang, 2022). At present, the factors impacting agricultural green total factor productivity have been the subject of much investigation by both domestic and foreign academics. Agricultural pollution is one of many influencing elements that greatly jeopardize agricultural ecological efficiency (Irfan and Ahmad, 2022).
The most immediate and efficient factor is environmental regulation. However, there are not many studies looking at the effects of environmental regulation on agricultural green TFP. Moreover, there is conflicting evidence in the available research on the link between environmental legislation and agricultural green TFP. A fair agricultural environmental regulation, according to certain studies, can increase agricultural green total factor productivity (Zhan and Xu, 2019), and while believe that the impact of agricultural environmental regulation on agricultural green total factor productivity is that with the continuous increase of the threshold value (Yu et al., 2022), some scholars (Liang et al., 2012b) believe that the impact of ER on agricultural green total factor productivity is negative, the negative impact factors are gradually reduced (Yu et al., 2022). Environmental regulation is frequently used as a control variable in literature. This study examines environmental control as a significant explanatory factor.
In addition, the selection of environmental regulation variables varies greatly among different scholars. For example, the number of agricultural environmental protection policies is used to measure environmental regulation; (Zhan and Xu, 2019) using agricultural command and control (Xie et al., 2021) use agricultural carbon emissions and pollution emissions to measure. It is clear that there is still a significant gap in the literature on the two’s relationship.
In order to study the impact of environmental regulation ER on agricultural green total factor productivity and agricultural green innovation, this paper first considers the linear model shown below
Among them, Xit − 1 represents the ER level of environmental regulation in the ith city at t − 1. In order to consider the lag of environmental regulation, the key explanatory variable ER is lagged by one period. The variable Zit − 1 is a control variable, in order to avoid being affected by t − 1 At the same time, the influence of explanatory variables makes the empirical results biased, so the control variables are also lagged by one period. δi represents the unobserved individual effect and μit is the random error assumed to be i.i.d (0, σμ2).
However, considering that the impact of ER on agricultural green development may be affected by the level of economic development, most scholars may construct an interaction term between the level of economic development and environmental regulation ER, but this strategy may lead to model errors and estimation biases. For details, refer to (Du et al., 2020a; Du et al., 2020b; Du et al., 2021). Therefore, this paper introduces the variable coefficient Uit − 1 for the model. Uit − 1 specifically refers to the level of regional economic development in this paper, that is, PGDP. Part of the variable coefficient panel model is as follows:
The heterogeneity effect of different regional economic development levels on my country’s agricultural green transformation is expressed as γ(Uit − 1). Based on the nonparametric kernel method, (An et al., 2016) further extended the model, and extended the cross-sectional model to a partial linear variable coefficient panel data model with fixed effects using the series method. Specifically, please refer to the research methodology (An et al., 2016; Du et al., 2021).
First, use the difference to eliminate the fixed effect δ
Second, the substitution function coefficients γ(Uit − 1) are approximated by a linear combination of the k basis functions:
where p(Uit − 1) is a k × 1 basis function, and θ is an unknown parameter of k × 1. When k is large enough, there is a linear combination of pi(Uit − 1) that can approximately replace any smoothing coefficient γ(Uit − 1), and the mean square error MSE is the smallest. Eq. 4 can be rewritten as:
Among them, the error term
Finally, the least squares estimate:
Furthermore, the coefficient function γ(•) is estimated as:
Therefore, based on the research of (An et al., 2016) and with reference to (Du et al., 2020c) to estimate the partial linear panel model of the function coefficients, the Stata software package estimates the following models. The specific estimation equation in this paper is set as follows with reference to the reference model Eq. 2:
Among them, the following table i and t are the corresponding 30 provinces in China and the corresponding year, t − 1 is the corresponding year with a lag period, there are two explained variables in this paper, namely agricultural green total factor productivity (GTFP) and agricultural green For innovative GP, considering the lag of the impact of environmental regulation on ER, the key explanatory variable ER lag one period is expressed as ERit − 1, And γ(lnPGDPit − 1) is the function coefficient of ERit − 1. The variable Zit − 1 is the control variable, including HUMAN, EG, DR, TRA, MAC, and IND, Which are expressed as: the level of rural human capital, the intensity of environmental pollution control, the degree of disaster, the density of agricultural machinery, the gap between urban and rural income distribution, and the level of rural economic openness. Considering the consistency of the explanatory variables, there is also a lag of one period.
The BMLPI (Biennial Malmquist-Luenberger productivity index) proposed by Pastor et al. (2011), Wang (2011) is used to measure the agricultural green total factor productivity of the explanatory variables in this paper, that is, the two-period Manquist-Luenberger productivity index. GMLPI (Global MLPI), that is, the similarity of the global Manquist-Luenberger productivity index, is to solve the problem of unfeasible solutions for measuring green productivity with undesired output. Although GMLPI can solve the problem of infeasible solutions, when new data is added, for example, when data is added every year, the entire frontier needs to be rebuilt, and the overall technology changes accordingly. And miscellaneous, the model calculation number is not stable. However, BMLPI is different. It is a productivity index that can be used as a production technology to calculate efficiency and variables every two periods. Therefore, there is no need to repeat the calculation for each additional year of data. The already calculated data will not change, only the newly added data will be calculated. Once, its additional advantage is that it can take into account technological regressions, which are much looser than the harsh assumptions of previous models. Therefore, the BMLPI model formula for calculating agricultural green total factor productivity based on DDF is as follows:
The software for calculating agricultural green total factor productivity is the latest version of Stata17, which cannot be calculated in previous versions of Stata. The input indicators of the productivity are as follows: X1 represents the input of chemical fertilizers, which is measured by the amount of agricultural chemical fertilizers calculated by the pure method; X2 represents the input of agricultural machinery, which is measured by the total power of agricultural machinery; X3 represents the agricultural irrigation Input, this indicator is measured by the effective agricultural irrigation area; X4 represents land input, which is measured by the sown area of crops; X5 represents labor input, which is measured by the employees of agriculture, forestry, animal husbandry and fishery. The output indicators include the expected output expressed by the total output value of agriculture, forestry, animal husbandry and fishery, which takes 2000 as the base period to calculate the constant price; the non-expected output indicator is the measurement method of agricultural carbon emissions. Refer to Section 3.2.2 for the calculation method.
Another explanatory variable in this paper, agricultural green innovation, is expressed by the number of agricultural green patents (GP) in each province. The IPC classification number of the number of agricultural green technology patents is based on the “IPC Green Inventory” guidelines formulated by the IPC Committee1, obtained with reference to the agricultural part of the green patent list issued by the World Intellectual Property Organization (WIPO), and obtained through screening in the Patent Search and Analysis System (PSS-System) of the China Intellectual Property Office. Since patent examination takes a long time and there is a time lag in patent granting, the number of green patents is the sum of the data of invention patents and utility patent applications, rather than the data of patent grants.
The key explanatory variable studied in this paper is environmental regulation (ER). At present, scholars measure various types of environmental regulation variables. Specifically, some scholars in the field of agriculture use the number of agricultural environmental protection policies at the end of each year in each province in my country. In the same way, agricultural carbon emissions are used to measure the intensity of environmental regulation. The calculation method is based on. The formula for calculating carbon emissions is as follows:
In the formula, ER is the total amount of agricultural carbon emissions, and Ei is expressed as the emissions of six carbon sources, including the use of chemical fertilizers, pesticides, agricultural film, and diesel in agricultural production, as well as ploughing (measured by the actual sown area of crops) and The amount of carbon emissions produced by the use of machinery and equipment in the agricultural production process due to irrigation, Ti is the amount emitted by each carbon source, and δi is the coefficient of each carbon emission, which are fertilizers (0.8956 kg kg−1), pesticides (4.9341 kg kg−1), agricultural film (5.18 kg kg−1), diesel oil (05927 kg kg−1), tillage (312.6 kg km−1), agricultural irrigation (25 kg hm−1), but according to Li Bo’s calculation method of agricultural irrigation carbon emissions, this paper uses the data of China Statistical Yearbook from 2015 to 2019 to calculate the actual average thermal power coefficient of 0.7316, and the final calculation of the actual coefficient of agricultural irrigation is 18.291 kg hm−1.
According to the current situation of agricultural development in my country, the control variables selected in this paper are as follows:
1) The level of rural human capital (HUMAN). In general, the higher the level of rural labor culture, the more conducive to the mastery of agricultural production technology and the rational use of pesticides, fertilizers and other factors, so theoretically, it will have a positive impact on agricultural green innovation and agricultural total factor productivity. This article is based on the calculation method of (Qiao, 2018) Human = prim × 6 + midd × 9 + high × 12 + univ × 16, of which 6, 9, 12, and 16 are the primary, junior high, and high schools for workers in agricultural production, respectively. The number of years of education of secondary school and college education above, prim, midd, high, and univ respectively represent the proportion of residents aged six and above in each education stage.
2) Environmental pollution control intensity (EG). The quality of rural agricultural environment is closely related to the intensity of local environmental governance, so it is measured by the proportion of environmental pollution control investment in GDP.
3) Agricultural production environment (DR). In general, the more serious the agricultural disaster in a certain area is, the greater the damage to the local agricultural production environment. Therefore, this paper uses the proportion of the affected area of land in regional agricultural production to the total sown area of crops to measure.
4) Agricultural Machinery Density (MAC). Generally speaking, the higher the density of agricultural machinery may lead to an increase in carbon emissions and affect the way of local agricultural green transformation. Therefore, this paper uses the proportion of the total power of agricultural machinery to the sown area to measure.
5) Urban-rural income ratio (IND). Generally speaking, the size of the income gap between urban and rural residents indicates the degree of support of the local government for agricultural development. Therefore, this paper uses the ratio of the per capita net income of urban residents to rural residents in the region to measure this indicator.
6) Rural economic opening level (TRA). Generally speaking, the trade status of agricultural products in the region will affect the agricultural production environment, which in turn affects the green development of local agriculture. Therefore, this paper uses the proportion of the total import and export of agricultural products in the region to the total agricultural production to measure this indicator.
The main explanatory variable in this paper is agricultural green total factor productivity, which is calculated based on the data of “China Statistical Yearbook” and “China Rural Statistical Yearbook” and takes 2000 as the base period. Search and analysis system (PSS-System) screening. The data from 2001 to 2020 are selected for the two explained variables, and the environmental regulation and control variables are calculated using the data from 2000 to 2019. The data sources are China Statistical Yearbook, China Rural Statistical Yearbook, and China Population and Employment Statistical Yearbook. “China Agricultural Yearbook,” “China Environmental Yearbook,” and “China Agricultural Products Trade Development Report” and so on.
In addition, in order to study whether there is heterogeneity in the impact of environmental regulation on agricultural total factor productivity and agricultural green technology innovation under different economic development levels, this paper uses per capita GDP to represent the level of economic development (PGDP). And the base period is 2000. Except for the environmental regulation (ER) variable, all other variables are logarithmic, and the descriptive statistics of all variables are shown in Table 1.
The panel model estimation results with fixed effects in Eq. 1 are shown in Table 2. The ER estimated coefficients on both lnGP and lnGTFP are positive and significant, suggesting that if environmental regulation increases by 0.01, GP and GTFP will increase by 0.065% and 0.023%, respectively. These results preliminarily show that environmental regulation contributes to the green transition of agricultural development. On the one hand, environmental regulation can promote green technology innovation in the field of agricultural production and stimulate the application of green technology; on the other hand, environmental regulation can promote the improvement of agricultural green total factor productivity. As far as control variables are concerned, the significance of the effects of control variables on lnGP and lnGTFP in the previous period is basically the same. The estimated result in Table 2 is the impact of environmental regulation ER on the green development of agriculture without considering the level of economic development. In the next section, this paper will discuss the heterogeneity of two important indicators of ER in the development of agricultural green transformation at different levels of economic development.
Among the control variables, the variable of interest, the level of rural human capital (HUMAN), has a negative coefficient on both lnGP and lnGTFP. Theoretically, the higher the education level of farmers, the more favorable the development of green transformation in rural areas, but in fact, during the early development of China, the government focused more on economic development at the expense of environmental protection, and the phenomenon of “pollution first and treatment later” emerged. In recent years, however, the government has begun to pay more attention to environmental protection and the concept of green water and green mountains has begun to take root in people’s minds. It is believed that in the near future, the positive effects of human capital will be brought into full play. In fact, the coefficients of agricultural machinery density on lnGP and lnGTFP are both significantly positive, meaning that the higher the degree of agricultural mechanization, the more it contributes to the green transformation of agriculture. As the degree of agricultural mechanization increases, agricultural development is scaled up and resource utilization is maximized, reducing carbon emissions from agricultural production as the efficiency of agricultural production increases, thus protecting the ecological environment.
This section analyzes the impact of environmental regulation ER on agricultural green technology innovation and agricultural green total factor productivity under different economic development levels based on a partial linear panel model with functional coefficients. The estimation results of Eqs 2, 3 are shown in Table 3. Figures 3, 4 show the heterogeneous effects of environmental regulation of ER at different levels of economic development, that is, the impact of ER on agricultural green technology innovation and agricultural green TFP will vary with different levels of economic development. The 95% confidence interval indicates that the effect of ER in these intervals is significant and positively contributing. As far as the control variables are concerned, the coefficient of agricultural production environment (DR) is negative, and the deterioration of agricultural environment inhibits the development of lnGP and lnGTFP; therefore, it is necessary to develop ecological agriculture and disaster-avoidance agriculture to change the disadvantage into advantage and promote the green transformation of agricultural development. In addition, the coefficient of environmental pollution control intensity (EG) on lnGTFP is insignificant in Table 2, while it is significant in Table 3, but the coefficient is negative, increasing the strength of environmental control has a positive role in promoting green development, but in the early stage, with the increase of investment in green industries, there is an inhibitory effect on the development of green economy, but with the concept of universal energy conservation as well as ecological and environmental protection gradually It is believed that the development of green economy will be actively promoted in the future.
TABLE 3. Estimated results of the linear part of partially linear function-coefficient panel models.
FIGURE 3. The functional coefficient of environmental regulation ER to agricultural green technology innovation.
For agricultural green technology innovation, when the level of economic development is relatively low, the implementation of environmental regulation ER has an inhibitory effect, which is not conducive to the development of green innovation. However, with the further development of my country’s high-quality economy and the strong awareness of people’s environmental protection, the inhibitory effect will gradually weaken. When economic development reaches a higher level, ER will play a positive role, and people will begin to actively save energy and reduce emissions, green environmental protection, and low-carbon life. Therefore, the innovation of agricultural green technology can promote the realization of environmental protection in agriculture and rural areas, and there are always clear waters and lush mountains.
For agricultural green total factor productivity, when the level of economic development is low, environmental regulation ER not only has a crowding-out effect on economic development, but also has a crowding-out effect on non-green R&D companies because of increased R&D costs. Energy industry technology will be limited due to environmental regulation ER, so in the initial stage, agricultural green total factor productivity will be inhibited. However, with the continuous improvement of the level of economic development and the enhancement of people’s awareness of environmental protection, ER will instead stimulate the innovation and development of green production technology, improve environmental protection capabilities, product quality, and ultimately improve agricultural green productivity, so that the social economy and the environment can develop harmoniously.
This section will explore the impact of ER on agricultural green development based on the heterogeneity of economic development levels in each province. Referring to (Du at al., 2021), for the classification of the economic development level of each city in China, this paper divides 30 provinces and cities in China into three categories based on the average value of real per capita GDP. It shows the impact of ER on agricultural green technology innovation and agricultural green total factor productivity in three different time periods, 2001-2002, 2009-2010, and 2018-2019.
It can be seen from Figure 5A that in 2001-2002, for most provinces with low and medium levels of economic development, the impact of ER on agricultural green technology innovation and agricultural green TFP was not significant. In a few provinces with low levels of economic development, ER has a significant impact on agricultural green total factor productivity, but hardly contributes to agricultural green technology innovation. For most provinces with a high level of economic development, ER can significantly promote agricultural green technology innovation and agricultural green total factor productivity.
FIGURE 5. Functional coefficients of ER with respect to lnGP. (A) Function coefficients of ER in 2001-2002. (B) Function coefficients of ER in 2009-2010. (C) Function coefficients of ER in 2018-2019. Note: Squares indicate when real PGDP is low; triangles indicate when actual PGDP is moderate; circles indicate when actual PGDP is high. Red indicates that the effect of ER on lnGTFP and lnGP is not significant; yellow indicates that ER has a significant effect on lnGTFP; blue indicates that ER has a significant effect on lnGP; green indicates that ER has a significant effect on both lnGTFP and lnGP.
It can be seen from Figure 5B that in 2009-2010, for most provinces with low and medium economic development levels, the contribution of ER to agricultural green technology innovation and agricultural green TFP increased. For provinces with a higher level of economic development, the positive effect of ER on the impact of the two is also increasing.
From Figure 5C, in 2018-2019, in almost all provinces with higher economic development levels, ER can significantly promote agricultural green technology innovation and agricultural green total factor productivity. Due to the balance between the east and west of my country’s economic development level, there are still many provinces with low economic development levels. The impact of ER on agricultural green technology innovation and agricultural green total factor productivity is not significant. The pulling effect of technological innovation and agricultural green total factor productivity is increasing.
From Figures 5A–C, we can tell that, as time goes by, the economic role of ER’s agricultural green transition is increasing, and the impact is becoming more and more prominent. From 2000 to 2019, the economic development level of each province has been continuously improved, which verifies that the green transformation of my country’s agricultural development is affected by the level of economic development. A higher level of economic development will help ER play a more active role in agricultural green technology innovation and agricultural green total factor productivity, and promote the green transformation of my country’s agricultural development.
Agricultural green technology innovation and agricultural green total factor productivity are the two main indicators for the green transformation of agricultural development, while environmental regulation is a key measure to prevent and control pollution as well as agricultural green sustainable development goals.
Taking into account the differences in regional economic development levels, this paper adopts a partial linear panel model with functional coefficients to study the heterogeneous impact of environmental regulation on agricultural green technology innovation and agricultural green total factor productivity. The main conclusions are as follows: 1) With the improvement of the level of economic development, the role of environmental regulation in promoting agricultural green technology innovation and agricultural green total factor productivity has been significantly enhanced, and environmental regulation has played an increasingly active role. The impact of total factor productivity is more significant than that of agricultural green technology innovation. 2) This paper takes 2001-2002, 2009-2010, and 2018-2019 as examples, and empirically analyzes that with the passage of time, the role of environmental regulation in promoting the green transformation of agricultural development has become more and more prominent. . From the initial period of 2001-2002, only a few provinces with a relatively high level of economic development did environmental regulation significantly promote the development of agricultural green technology innovation and agricultural green total factor productivity. In lower provinces, the contribution of environmental regulation is not obvious.
The above empirical results show that under different levels of economic development, environmental regulation has different effects on agricultural green technology innovation and agricultural green total factor productivity. Therefore, agricultural green environmental protection policies should be adapted to regional economic development, so that relevant supporting policies can be promulgated to cooperate with the implementation of environmental regulations.
First of all, in order to better promote the innovation of agricultural green technology, the differences in the level of economic development in different regions should be considered. For regions with low economic development levels, appropriate policy support should be given to stimulate the innovation of agricultural green technology. The tools of policy regulation and gradual transformation of environmental regulation have changed from imperative to incentive. For example, the government provides financial support for agricultural green technology innovation and research and development capabilities, increases compensation for environmental protection, increases investment in agricultural green development from different channels, enhances the power of green technology to develop agriculture, and reduce the constraints of energy saving and emission reduction clean and environmental protection technologies. At the same time, strengthen regional cooperation and give full play to the spillover effect of technological innovation in regions with high economic development levels.
Secondly, the difference in the level of economic development makes the impact of environmental regulation on agricultural green total factor productivity heterogeneous. Going back to the past development experience, the green development of my country’s agriculture should avoid maintaining growth at the expense of the environment, and formulate a prudent and appropriate agricultural Green environmental protection policies, regulations and standards, vigorously promote green ecology, enable agriculture to develop circularly, and continuously increase the development and subsidies of agricultural green production behaviors. For example: replacing chemical fertilizers with organic fertilizers, promoting the development of green and energy-saving agricultural machinery, reducing energy consumption in agricultural production, developing comprehensive planting and breeding, aquaponics and other green measures to make the production model greener. Design a market-oriented operation system for environmental protection and ecological construction in line with the green development of agriculture, and at the same time cooperate with the government’s “visible hand” to jointly promote energy conservation, emission reduction, green and low carbon in the agricultural and rural sectors. An important part of carbon neutrality. Ultimately, the comprehensive green transformation of agricultural development will be achieved.
Finally, in order to promote the large-scale development of agricultural green planting technology, the government should take incentive measures to encourage relevant green planting enterprises to join in the promotion of green agricultural planting technology, further expand the development of import and export trade of agricultural products, increase farmers’ income, and strengthen The training on green production technology for agricultural and rural producers will increase the production and income of agricultural farmers, improve the efficiency of various agricultural production factors, save energy and reduce emissions, and accelerate the transformation and development of my country’s agriculture to green (Galloway and Johnson, 2016; Liu et al., 2018; Xiao et al., 2022).
The original contributions presented in the study are included in the article/Supplementary Material, further inquiries can be directed to the corresponding author.
CS: The author contributed to the drafting of the paper, participated in the conception and design of the study, collected the data, and performed the statistical analysis. The author has reviewed and approved the manuscript’s published form.
I am very grateful to the Guangzhou Office of Philosophy and Social Science (2021GZGJ29), Guangdong Provincial Key Scientific Research Platform Project (2020ZDZX1064), Guangdong Educational Science Planning Project (2022GXJK673).
I appreciate the editors’ and the two reviewers’ insightful comments of a previous draft of this manuscript.
The author declares that the research was conducted in the absence of any commercial or financial relationships that could be construed as a potential conflict of interest.
All claims expressed in this article are solely those of the authors and do not necessarily represent those of their affiliated organizations, or those of the publisher, the editors and the reviewers. Any product that may be evaluated in this article, or claim that may be made by its manufacturer, is not guaranteed or endorsed by the publisher.
The Supplementary Material for this article can be found online at: https://www.frontiersin.org/articles/10.3389/fenvs.2022.955954/full#supplementary-material
1IPC Green Inventory website: https://www.wipo.int/classifications/ipc/green-inventory/home.
Abbasi, K. R., Shahbaz, M., Zhang, J., Irfan, M., and Alvarado, R. (2022). Analyze the environmental sustainability factors of China: The role of fossil fuel energy and renewable energy. Renew. Energy 187, 390–402. doi:10.1016/j.renene.2022.01.066
Ambec, S., Cohen, M. A., Elgie, S., and Lanoie, P. (2013). The porter hypothesis at 20: Can environmental regulation enhance innovation and competitiveness? Rev. Environ. Econ. Policy 7 (1), 2–22. doi:10.1093/reep/res016
An, Y., Hsiao, C., and Li, D. (2016). Semiparametric Estimation of Partially Linear Varying Coefficient Panel Data Models Emerald Publishing Ltd. 36, 47–65. doi:10.1108/S0731-905320160000036011
Balsa-Barreiro, J., Li, Y., Morales, A., and Pentland, A. S. (2019). Globalization and the shifting centers of gravity of world's human dynamics: Implications for sustainability. J. Clean. Prod. 239, 117923. doi:10.1016/j.jclepro.2019.117923
Borsatto, J. M. L. S., and Amui, L. B. L. (2019). Green innovation: Unfolding the relation with environmental regulations and competitiveness. Resour. Conserv. Recycl. 149, 445–454. doi:10.1016/j.resconrec.2019.06.005
Brunnermeier, S. B., and Cohen, M. A. (2003). Determinants of environmental innovation in US manufacturing industries. J. Environ. Econ. Manag. 45 (2), 278–293. doi:10.1016/S0095-0696(02)00058-X
Cochard, F., Willinger, M., and Xepapadeas, A. (2005). Efficiency of nonpoint source pollution instruments:an experimental study. Environ. Resour. Econ. (Dordr). 30 (4), 393–422. doi:10.1007/s10640-004-5986-y
Curtis, E. M., and Lee, J. M. (2019). When do environmental regulations backfire? Onsite indus trial electricity generation, energy efficiency and policy instruments. J. Environ. Econ. Manage. 96, 174–194. doi:10.1016/j.jeem.2019.04.004
Deng, X., and Gibson, J. (2019). Improving eco-efficiency for the sustainable agricultural production: A case study in shandong, China. Technol. Forecast. Soc. Change 144, 394–400. doi:10.1016/j.techfore.2018.01.027
Du, K., Yu, Y., and Li, J. (2020a). Does international trade promote CO2 emission performance? An empirical analysis based on a partially linear functional-coefficient panel data model. Energy Econ. 92, 104983. doi:10.1016/j.eneco.2020.104983
Du, K., Yu, Y., and Wei, C. (2020b). Climatic impact on China’s residential electricity consumption: Does the income level matter? China econ. China Econ. Rev. 63, 101520. doi:10.1016/j.chieco.2020.101520
Du, K., Zhang, Y., and Zhou, Q. (2020c). Fitting partially linear functional-coefficient panel-data models with Stata. Stata J. 20 (4), 976–998. doi:10.1177/1536867X20976339
Du, K., Cheng, Y., and Yao, X. (2021). Environmental regulation, green technology innovation, and industrial structure upgrading: The road to the green transformation of Chinese cities. Energy Econ. 98, 105247. doi:10.1016/j.eneco.2021.105247
Galloway, E., and Johnson, E. P.(2016). Teaching an old dog new tricks: Firm learning from environmental regulation. Energy Econ. 59, 1–10. doi:10.1016/j.eneco.2016.06.023
Gollop, F. M., and Roberts, M. J. (1983). Environmental regulations and productivity growth: The case of fossil-fueled electric power generation. J. Polit. Econ. 91 (4), 654–674. doi:10.1086/261170
Grey, W. B., and Shadbegian, R. J. (2003). Plant vintage, technology, and environmental regulation. J. Environ. Econ. Manage. 46 (3), 384–402. doi:10.1016/S0095-0696(03)00031-7
Hao, Y., Gai, Z., Yan, G., Wu, H., and Irfan, M. (2021). The spatial spillover effect and nonlinear relationship analysis between environmental decentralization, government corruption and air pollution: Evidence from China. Sci. Total Environ. 763, 144183. doi:10.1016/j.scitotenv.2020.144183
Iqbal, W., Tang, Y. M., Chau, K. Y., Irfan, M., and Mohsin, M. (2021). Nexus between air pollution and NCOV-2019 in China: Application of negative binomial regression analysis. Process Saf. Environ. Prot. 150, 557–565. doi:10.1016/j.psep.2021.04.039
Irfan, M., and Ahmad, M. (2022). Modeling consumers’ information acquisition and 5G technology utilization: Is personality relevant? Personality individ. Pers. Individ. Dif. 188, 111450. doi:10.1016/j.paid.2021.111450
Irfan, M., Elavarasan, R. M., Hao, Y., Feng, M., and Sailan, D. (2021). An assessment of consumers’ willingness to utilize solar energy in China: End-users’ perspective. J. Clean. Prod. 292, 126008. doi:10.1016/j.jclepro.2021.126008
Jiang, G. (2022). How does agro-tourism integration influence the rebound effect of China’s agricultural eco-efficiency? An economic development perspective. Front. Environ. Sci. 10, 921103. doi:10.3389/fenvs.2022.921103
Jorge, M. L., Madueño, J. H., Martinez-Martinez, D., and Sancho, M. P. L. (2015). Competitiveness and environmental performance in Spanish small and medium enterprises: Is there a direct link? J. Clean. Prod. 101, 26–37. doi:10.1016/j.jclepro.2015.04.016
Li, J. T., Ji, J. Y., and Zhang, Y. (2019). Non-linear effects of environmental regulations on economic outcomes. Manag. Environ. Qual. 30 (2), 368–382. doi:10.1108/MEQ-06-2018-0104
Li, X., Shaikh, P. A., and Ullah, S. (2022). Exploring the potential role of higher education and ICT in China on green growth. Environ. Sci. Pollut. Res. 29, 64560–64567. doi:10.1007/s11356-022-20292-0
Liang, L., Qu, F., and Feng, S. (2012a). Measurement of agricultural technical efficiency based on the perspective of environmental pollution constraints. J. Nat. Resour. 27 (9), 1580–1589. doi:10.11849/zrzyxb.2012.09.015
Liang, L., Qu, F., and Feng, S. (2012b). Agricultural technical efficiency measurement under the environmental constraints. J. Nat. Resour. 27 (9), 1580–1589. doi:10.11849/zrzyxb.2012.09.015
Liu, Y., Li, Z. H., and Yin, X. M. (2018). Environmental regulation, technological innovation and energy consumption—A cross-region analysis in China. J. Clean. Prod. 203, 885–897. doi:10.1016/j.jclepro.2018.08.277
Liu, L., Yang, X., Meng, Y., Ran, Q., and Liu, Z. (2021a). Does the construction of national eco-industrial demonstration parks improve green total factor productivity? Evidence from prefecture level cities in China. Sustainability 14 (1), 26. doi:10.3390/su14010026
Liu, D., Zhu, X., and Wang, Y. (2021b). China's agricultural green total factor productivity based on carbon emission: An analysis of evolution trend and influencing factors. J. Clean. Prod. 278, 123692. doi:10.1016/j.jclepro.2020.123692
Liu, Q., Zhu, Y., Yang, W., and Wang, X. (2022). Research on the impact of environmental regulation on green technology innovation from the perspective of regional differences: A quasi-natural experiment based on China’s new environmental protection law. Sustainability 14 (3), 1714. doi:10.3390/su14031714
Pang, R., Zheng, D., Shi, M. J., and Zhang, X. L. (2019). Pollute first, control later? Exploring the economic threshold of effective environmental regulation in China’s context. J. Environ. Manage. 248, 109275. doi:10.1016/j.jenvman.2019.109275
Pastor, J. T., Asmild, M., and Lovell, C. A. K. (2011). The biennial malmquist productivity change index. Socio-Economic Plan. Sci. 45, 10–15. doi:10.1016/j.seps.2010.09.001
Picazo-Tadeo, A. J., Gómez-Limón, J. A., and Reig-Martínez, E. (2011). Assessing farming eco-efficiency: A data envelopment analysis approach. J. Environ. Manage. 92 (4), 1154–1164. doi:10.1016/j.jenvman.2010.11.025
Porter, M. E. (1991). Towards a dynamic theory of strategy. Strateg. Manag. J. 12 (S2), 95–117. doi:10.1002/smj.4250121008
Qiao, M. (2018). Dual effects of environmental regulation on R&D innovation efficiency:an empirical study based on inter-provincial industrial panel data. Mod. Econ. Discuss. 09, 79–89. doi:10.13891/j.cnki.mer.2018.09.012
Rauf, A., Ozturk, I., Ahmad, F., Shehzad, K., Chandiao, A. A., Irfan, M., et al. (2021). Do tourism development, energy consumption and transportation demolish sustainable environments? Evidence from Chinese provinces. Sustainability 13 (22), 12361. doi:10.3390/su132212361
Shadbegian, R. J., and Gray, W. B. (2005). Pollution abatement expenditures and plant-level productivity: A production function approach. Ecol. Econ. 54 (2-3), 196–208. doi:10.1016/j.ecolecon.2004.12.029
Song, M., Wang, S., and Sun, J. (2018). Environmental regulations, staff quality, green technology, R&D efficiency, and profit in manufacturing. Technol. Forecast. Soc. Change 133, 1–14. doi:10.1016/j.techfore.2018.04.020
Su, S., and Zhang, F. (2020). Modeling the role of environmental regulations in regional green economy efficiency of China: Empirical evidence from super efficiency DEA-Tobit model. J. Environ. Manag. 261, 110227. doi:10.1016/j.jenvman.2020.110227
Turken, N., Carrillo, J., and Verter, V. (2020). Strategic supply chain decisions under environmental regulations: When to invest in end-of-pipe and green technology. Eur. J. Operational Res. 283 (2), 601–613. doi:10.1016/j.ejor.2019.11.022
Wang, C. (2011). Sources of energy productivity growth and its distribution dynamics in China. Resour. Energy Econ. 33, 279–292. doi:10.1016/j.reseneeco.2010.06.005
Wang, H., and Wei, W. (2020). Coordinating technological progress and environmental regulation in CO2 mitigation: The optimal levels for OECD countries & emerging economies. Energy Econ. 87, 104510. doi:10.1016/j.eneco.2019.104510
Wang, L., and Yan, Y. (2022). Environmental regulation intensity, carbon footprint and green total factor productivity of manufacturing industries. Int. J. Environ. Res. Public Health 19 (1), 553. doi:10.3390/ijerph19010553
Wang, Y., Sun, X., and Guo, X. (2019). Environmental regulation and green productivity growth: Empirical evidence on the Porter Hypothesis from OECD industrial sectors. Energy Policy 132, 611–619. doi:10.1016/j.enpol.2019.06.016
Xiao, S., He, Z., Zhang, W., and Qin, X. (2022). The agricultural green production following the technological progress: Evidence from China. Int. J. Environ. Res. Public Health 19 (16), 9876. doi:10.3390/ijerph19169876
Xie, C., Huang, C., and Xu, H. (2021). Regional disparity and influencing factors of agricultural technology efficiency in China: Based on dual constraint of agricultural carbon and agricultural non-point source pollution. Sci. Technol. Manag. Res. 41 (15), 184–190. doi:10.3969/j.issn.1000-7695.2021.15.024
Xu, Z., Chen, J., Jin, Z., Li, H., and Chen, F. (2020). Representing model uncertainty by multi-stochastic physics approaches in the GRAPES ensemble. Adv. Atmos. Sci. 37 (4), 328–346. doi:10.1007/s00376-020-9171-1
Ye, C., Sun, C., and Chen, L. (2018). New evidence for the impact of financial agglomeration on urbanization from a spatial econometrics analysis. J. Clean. Prod. 200, 65–73. doi:10.1016/j.jclepro.2018.07.253
Yu, K., Hu, Y., and Song, Z. (2019). Environmental regulation, government support and green technological innovation: An empirical study based on resource—Based enterprises. J. Yunnan Univ. Finance Econ. 35 (04), 100–112. doi:10.16537/j.cnki.jynufe.000434
Yu, D., Liu, L., Gao, S., Yuan, S., Shen, Q., and Chen, H. (2022). Impact of carbon trading on agricultural green total factor productivity in China. J. Clean. Prod. 367, 132789. doi:10.1016/j.jclepro.2022.132789
Zhan, J., and Xu, Y. (2019). Environmental regulation, agricultural green productivity and food security. China Popul. Resour. Environ. 29 (03), 167–176. Available at: http://agri.ckcest.cn/file1/M00/0F/71/Csgk0F4RmkeAXD9wAAYj8fqX3bg639.pdf.
Zhang, M., Sun, X. R., and Wang, W. W. (2020). Study on the effect of environmental regulations and industrial structure on haze pollution in China from the dual perspective of independence and linkage. J. Clean. Prod. 256, 120748. doi:10.1016/j.jclepro.2020.120-748
Zhao, J., Jiang, Q. Z., Dong, X. C., and Dong, K. Y. (2020a). Would environmental regulation improve the greenhouse gas benefits of natural gas use? A Chinese case study. Energy Econ. 87, 104712. doi:10.1016/j.eneco.2020.104712
Zhao, X. M., Liu, C. J., Sun, C. W., and Yang, M. (2020b). Does stringent environmental regulation lead to a carbon haven effect? Evidence from carbon-intensive industries in China. Energy Econ. 86, 104631. doi:10.1016/j.eneco.2019.104631
Zhou, A., and Li, J. (2021). Impact of anti-corruption and environmental regulation on the green development of China's manufacturing industry. Sustain. Prod. Consum. 27, 1944–1960. doi:10.1016/j.spc.2021.04.031
Keywords: agricultural green total factor productivity, agricultural green technology innovation, environmental regulation, agricultural green transformation, partial linear function coefficient panel model
Citation: Sun Y (2022) Environmental regulation, agricultural green technology innovation, and agricultural green total factor productivity. Front. Environ. Sci. 10:955954. doi: 10.3389/fenvs.2022.955954
Received: 29 May 2022; Accepted: 03 October 2022;
Published: 18 October 2022.
Edited by:
Martin Siegert, Imperial College London, United KingdomReviewed by:
Morteza Maleki, Georgia Institute of Technology, United StatesCopyright © 2022 Sun. This is an open-access article distributed under the terms of the Creative Commons Attribution License (CC BY). The use, distribution or reproduction in other forums is permitted, provided the original author(s) and the copyright owner(s) are credited and that the original publication in this journal is cited, in accordance with accepted academic practice. No use, distribution or reproduction is permitted which does not comply with these terms.
*Correspondence: Yongchun Sun, eml4aWFvcm9uZ2VyQDE2My5jb20=
†ORCID: Yongchun Sun, orcid.org/0000-0001-8823-911X
Disclaimer: All claims expressed in this article are solely those of the authors and do not necessarily represent those of their affiliated organizations, or those of the publisher, the editors and the reviewers. Any product that may be evaluated in this article or claim that may be made by its manufacturer is not guaranteed or endorsed by the publisher.
Research integrity at Frontiers
Learn more about the work of our research integrity team to safeguard the quality of each article we publish.