- 1School of International Education, Wuhan Business University, Wuhan, China
- 2Sustainable Real Estate Research Center, Department of Economics and Finance, Hong Kong Shue Yan University, Hong Kong, China
- 3School of Languages and Media, Anhui University of Finance and Economics, Bengbu, China
- 4School of Big Data and Intelligence Engineering, Southwest Forestry University, Kunming, China
- 5Institute of Mathematical Sciences, Faculty of Science, University of Malaya, Kuala Lumpur, Malaysia
- 6Department of Mathematics and Statistics, Washington State University, Pullman, WA, United States
- 7Jabalia Camp, United Nations Relief and Works Agency (UNRWA) Palestinian Refugee Camp, Gaza, Palestine
- 8Gofa Camp, Near Gofa Industrial College and German Adebabay, Addis Ababa, Ethiopia
Plants have a long history and diverse species. They play a critical role in the ecological chain, human production and life. With a vast territory, China has rich plant species and complex geographical regions, and research on the distribution of plant diversity in China is significant for utilising, developing, and protecting biological resources. This study aimed to study plant diversity in China. It obtained the plant diversity distribution data in China, then analysed it via spectral clustering and visualised it via Echarts. It was found that Yunnan records the most considerable plant diversity, followed by Sichuan and Tibet, meaning that the Southwestern part of China has the most extensive plant diversity. While it is expected that Tibet and Yunnan have backward economic development and hence a lower level of human destruction on plant diversity, Sichuan’s economic development is quite good. The land of abundance still has a wide variety of plants owing to excellent irrigation projects since 256BC. Besides, the Hengduan Mountains were the main route for Chinese plants to migrate from the west to the east, which might be closely related to the Himalayan orogeny. The Himalayan orogeny promotes the formation of the Qinghai–Tibet Plateau, which is a vital factor in the biodiversity formation in China. This study provides a basis for China’s conservation and study and enhances our understanding of plant biodiversity. As plant biodiversity increases soil organic carbon storage, it offers insights into carbon neutrality practitioners and researchers.
1 Introduction
Plant species arise from human-cultivated and spontaneously occurring species. Humans select cultivated species based on aesthetic, economic value, and services like visual buffering, trapping dust, and shading. Cultivated species diversity is usually associated with urban residents’ socioeconomic situations and personal preferences. In contrast, spontaneous species diversity can be influenced by macro-climatic variation and biogeographical processes, fine-scale processes like microhabitat heterogeneity, pesticides, soil characteristics, trampling intensity, and the distance to the city centre (Cheng et al., 2022). Recent studies have evidenced that biodiversity increases soil organic carbon storage in grasslands (Chen et al., 2018). Others studied plant diversity’s impact on soil physical and chemical characteristics, the effects on carbon storage processes and soil biological activity (Danise et al., 2022).
China has diverse plant species. According to Flora of China, there are 301 families, 3,408 genera, and 31,142 species of vascular plants in China, accounting for 56.9%, 24.5%, and 11.4% of the world’s families, genera, and species, respectively. In terms of the number of species, it follows the rich floral regions of Malaysia (approximately 45,000 species) and Brazil (approximately 40,000 species), ranking third globally (Editorial Committee of flora of China Chinese Academy of Sciences, 2004). Chinese plants with an ancient origin include many ancient or primitive families containing many relict plants. Chinese flora originates from the southern and northern ancient land and the ancient Mediterranean area, whose flora is of a unified tropical origin. Most modern botanists and paleobotanists agreed that various plants in the tropical distribution areas originate from the ancient southern continent. Nevertheless, irresponsible natural resources use, and environmental pollution has deteriorated environmental quality and harmed plant diversity. While it is high time to examine biodiversity for implementing policies and measures to enhance biodiversity, research on biodiversity in China via AI and data visualisation is limited. This study mainly analysed the spatial distribution characteristics of the plant diversity in China after a long period of evolution and migration via spectral clustering algorithm and visualisation, with the discussion ranging from the origin of plants to the reasons for the present distribution characteristics (Ying, 2001; Li et al., 2009; Chen et al., 2011; Zhumadillayeva et al., 2020).
The study is of practical value as that allows the related government departments to know more about the current biodiversity level and inform policymakers when they make related policies to preserve plant diversity and achieve carbon neutrality goals by balancing greenhouse gas or carbon dioxide emissions (Chen et al., 2022, forthcoming). It also fills the gap in research and contributes academically. In the following sections, section 2 describes the materials and methods of this article. Section 3 illustrates the results and discussion. Section 4 is the conclusion.
2 Materials and methods
2.1 Data
From January 2019 to March 2019, this study included data collected from the authoritative data displayed on the Flora of China website via Scrapy. It is an open-source and collaborative framework that uses non-blocking asynchronous code to achieve concurrency, which can quickly extract the required data from a website and is scalable. Previous research used and collected tweets written by 297 users (Park and Kwon, 2022, forthcoming) and online clothes retailer data (Muehlethaler and Albert, 2021).
A twisted framework was used to achieve asynchronous database writing in the configuration for speedy data collection. Using Scrapy for data acquisition, this study first parsed the webpage, found the location of the required information, and defined the XPath path. Then, it created a crawler using Scrapy and defined the field names for crawling the items. Finally, it defined the crawler rules and used the module to match the desired information, species name, and the subordinate category (phylum, class, order, family, race, genus, and group). Because the website of China Flora’s plant classification is only in Chinese, sample data collected is in Chinese only, as shown in Figure 1.
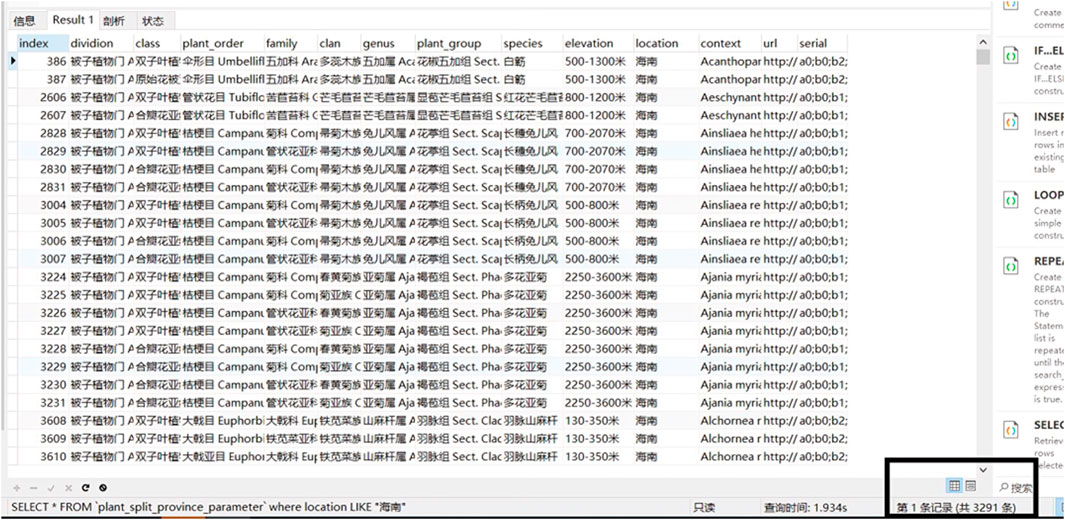
FIGURE 1. Screenshot some data in the first database (with the Hainan provincial administrative region as an example).
The plants shown in the figure are in order of Angiosperm, Dicotyledon, Protoperiana, Synaptophora, Umbellifera, Tubularia, Platycodon, Euphorbiaceae, Araliaceae, Gesneriaceae, Compositae, Euphorbiaceae, Asteroideae, Tupidanthus family, Mutisieae Cass family, Anthemis family, Acalypha family, Ainsliaea genus, Ajania Poljak genus, Alchornea, Achillea Impatiens group, Feather Vein group, Ainsliaea Henryi, Ainsliaea Reflexa, Ajania Myriantha, Alchornea rugosa.
It also shows the altitude information (500–1,300 m, 800–1,200 m, 700–2070 m, 500–800 m, respectively meters, 2,250–3,600 m, 130–350 m) correspond to the species, and the places of origin.
After data acquisition, this study filtered and classified the data. Various research has applied classification, such as remote sensing figure classification (Zhang et al., 2020) and concrete crack detection (Zhang et al., 2019). As the raw data collected was unclassified, we must classify data in each region and remove data that failed to indicate the origin. It then loaded the data to the data frame, read the origin, and determined whether a loop was null. The data was valid if the value was not null. Then, a new table was built based on the valid data and stored it in the database.
As the origin was a string in the database because of multiple origins in a species, this study splited the string, added other information about the species, inserted data into the database as a new piece of data, and completed the plant classification by region. After classifying by region, the classification was performed based on hierarchy.
2.2 Algorithms and models of network graphs
The geographical area varied considerably in different provincial administrative regions in China, and it had a specific correlation with the number of plant species. Furthermore, the number of plant species in each local administrative region affected the similarity of plant species between the provincial administrative regions. Therefore, this article considered all these three issues and proposed the following model. Some provincial administrative regions did not have plants of the same species but had plants of the same genus or larger units. As a result, we considered different plant categories when the number of plant species was consistent between the two provincial administrative regions. The plants were assigned different weights according to the categories from large to small: phylum, class, order, family, race, genus, group, and species. Larger categories were assigned with a smaller weight, and the reasonable weight was determined after multiple training rounds. Here, ω represents the category weight (ω i represents the weight corresponding to Ci, and higher i leads to smaller ω i). C is the similarity of plant species between the two provincial administrative regions (Ci represents the number of plants consistent between the two provincial administrative regions in phylum, class, order, family, race, genus, group, and species. A higher i represents a larger unit). J is the standardised plant species similarity between the two provincial administrative regions, and Q is the area in square kilometres. Further, m and n are two random provincial administrative regions, and O is the number of plant species in the provincial administrative region (species category).
During network graph establishment, this study used the similarity obtained from the above model between China’s pair-wise provincial administrative regions as the value between the two provincial administrative regions. Then, the algorithm first randomly determined one to two points. According to the value, the other points were added to the graph. Every time a point was added, the graph and the position of each point were automatically adjusted according to the value between the pair-wise provincial administrative regions, and a globally optimal network graph was finally generated.
2.3 Spectral clustering
Clustering has been applied to study the cluster of low carbon development (Du et al., 2018), construction safety research (Luo et al., 2022) and Tweets (Song et al., 2022). Compared with the traditional clustering algorithms, the spectral clustering algorithm based on the spectral graph theory could cluster on a sample space of arbitrary shape and converge to the optimal global solution. According to the given sample data set, the spectral clustering algorithm first defined an affinity matrix describing the similarity of the paired data points, calculated the eigenvalues and eigenvectors of the matrix, and then selected the appropriate eigenvectors to cluster different data points (Liu et al., 2020; Wen et al., 2020). The spectral clustering algorithm viewed each object in the dataset as the vertex V of the graph and measured the similarity between the vertices as the weight of the corresponding vertex connecting edge E, thus obtaining an undirected weighted graph G based on the similarity (V, E). Thus, the clustering problem could be transformed into a graph partitioning problem. It treated all data as points in space that edges could connect. The edge between two far-away points had a low weight value, while two close points had a higher weight value. By cutting the graph composed of all data points so that the sum of the edge weights between the subgraphs was as low as possible while the sum of the edge weights in the subgraphs was as high as possible, we achieved the purpose of clustering and built more accurate clusters in all circumstances (Ahmadi et al., 2021). The most used method for generating a similarity matrix was the full connection mode based on the Gaussian kernel distance. The most popular graph cutting method was N cut, and the most commonly used clustering method was K-means. Our graph was a globally optimal solution model based on spectral clustering and then K-means clustering was used this study. K-means is an effective and fast method to partition observations into k mutually exclusive clusters, and the observations in each cluster are as close to one another as possible.
After obtaining the variables’ similarity based on the model in the network graph, we performed spectral clustering on each local administrative region based on the similarity of plant species between the provincial administrative regions. P was set as the collection of provincial administrative regions in China. The following models were obtained to construct the similarity matrix. Here, Pi represents province i∈[1,34], i represents 34 provincial administrative regions in China. P11 represents the similarity between P1 provincial administrative region and itself, and P12 represents the similarity between P1 and P2 provincial administrative regions. The similarity matrix was derived (Figure 2) and Figure 3 shows the results of adjacency matrix.
Here, P11 represents the similarity between P1 provincial administrative region and itself, and P12 represents the similarity between P1 and P2 provincial administrative regions. The similarity matrix was derived (Figure 3).
At the same time, we built a degree matrix D according to the similarity matrix, calculated the Laplace matrix L from the degree matrix D and the adjacency matrix W, and then normalised it.
Thus, we obtained the normalised Laplacian matrix (Figure 4).
Finally, the eigenvectors corresponding to
2.4 Data visualisation by using echarts
Visualisation means the theory, method, and technology of using computer graphics and image processing technology to convert data into graphics or images and display them on the screen for interactive processing. Employing a graphical approach allows people to convey the information clearly and effectively. Andrienko and Andrienko used the intelligent visualisation method to conduct a situation analysis, find appropriate solutions to solve problems and make decisions (Luo et al., 2020).
There is abundant software capable of visualisation. This study chose the Echarts software to visualise graphics. Echarts represents an open-source visualisation library implemented using JavaScript, which can run smoothly on PC and mobile devices. Compatible with most current browsers, it uses the lightweight vector graphics library ZRender at the bottom to provide intuitive data visualisation charts with rich interaction that supports highly personalised customisation (ECharts, 2021). The ECharts visualised the pie charts and maps of the collected data.
This software showcased the relationship between the distribution characteristics of plant diversity in China and the corresponding geographic location by map visualisation. Echarts contain sections of the map of China. It attached the latitude and longitude coordinates to each provincial administrative region, and then loaded the total number of plant species in each administrative region from the database to ECharts to obtain the value of this administrative, administrative region. It selected a colour block and matched the values from the smallest to the biggest with a gradual change in the colour block from light to dark for visualising the data on the map of China. For pie charts, Echarts’ pie chart model was used similarly. However, only the top five provincial administrative regions regarding the number of plant species were filtered and indicated in five different colours.
3 Results and discussion
3.1 Distribution characteristics of plant diversity in China
Biodiversity is an essential subject in the current study of the biological environment in the world. The formation mechanism and the distribution pattern of biodiversity, its protection and utilisation have always been essential contents studied in biology and environmental sciences, wherein flora research plays a role. Research on the distribution pattern of species diversity is significant for protecting biodiversity. With a vast territory, China covers various terrains from low to high altitudes and climates from tropical and subtropical to temperate and cold regions. Mountain areas are essential but vulnerable centres of biodiversity. They also have substantial impacts on global climate change. Therefore, the variety of ecosystems indicates that China is one of the countries with the richest biological diversity in the world. The colourful flora of China includes many endemic species, which makes China the country with the highest plant diversity in the northern hemisphere. The first reason is the vast land area, long history of plant evolution, and a relatively stable climate. Second, China has continuous and complete vegetation types in tropical, subtropical, temperate, and cold regions. Third, along with the active geological structures, China’s vast land has been deeply cut and uplifted hundreds of years ago, resulting in complex and diverse habitats, making China a vital preservation centre, formation centre, and evolution centre for plant species.
The visual map is the most crucial form of spatial information visualisation, which can clearly and intuitively display the geographic location of the provincial administrative regions in China. Yunnan (17582) has an enormous diversity, followed by Sichuan (12465) and Tibet (7925). That is, the South Western part of China has the most considerable plant diversity (Figure 5). While it is expected that Tibet and Yunnan have backward economic development and hence lower level of human destruction on plant diversity, Sichuan’s economic development is quite good. It has been known as the land of abundance (天府之國) in Stratagems of the Warring States (戰國策). Dujiangyan Water Conservancy Project were built at the end of Zhou dynasty per Records of Huayang Kingdom volume Three “The Chronicle of Shu” (《華陽國志》卷三《蜀志》):
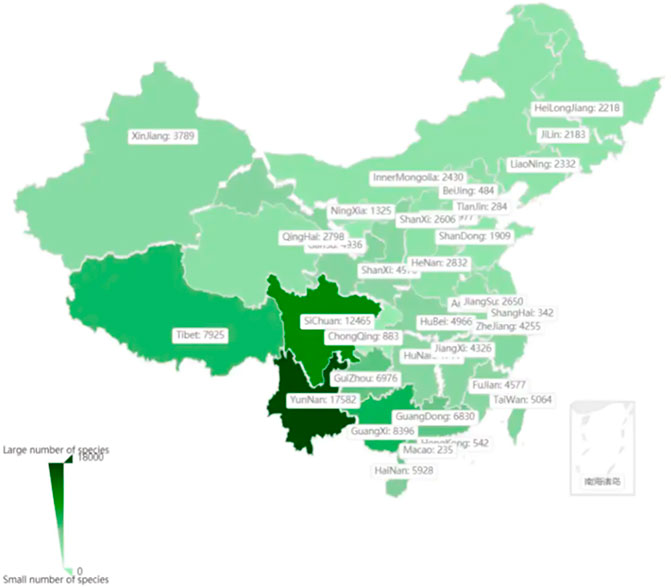
FIGURE 5. Distribution of plant species in various provincial administrative regions in China. The colour ranges from light to dark green to indicate the number of plant species from low to high. A darker colour refers to a more diversify plant species, while a lighter colour infers a smaller number of plant species.
“The fertile fields and thousands of miles are called the land and the sea. When there is drought, water is infiltrated, and the rain stops at the water gate. Therefore, it is recorded that the flood and drought follow people who do not know about famine. When there is no famine, the world is called Tianfu (nature’s storehouse)” In Chinese: “沃野千里,號為陸海,旱則引水浸潤,雨則杜塞水門,故記曰水旱從人,不知饑饉,時無荒年,天下謂之天府也。”
The results indicate the importance of irrigation facilities in reducing the harm to the natural environment.
Based on the visual map, we drew a pie chart for the top five provincial administrative regions regarding plant species and discussed and analysed the distribution of plant diversity concerning the pie chart and map (Figure 6). The network graph reflects the relationship between the points by connecting nodes, which can reflect the similarity in the plant species between various provincial administrative regions in China (Figure 7).
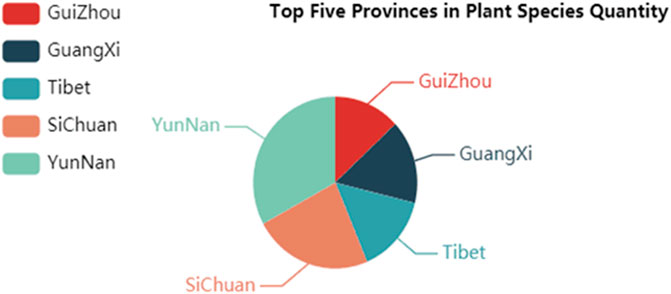
FIGURE 6. The top five provincial administrative regions regarding the number of plant species in China. Different provincial administrative regions correspond to different colours, and the fan size represents the scale of plant species.
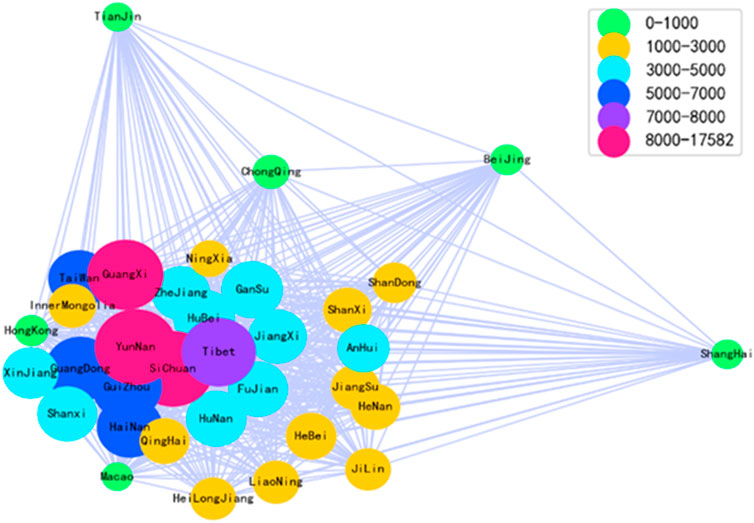
FIGURE 7. A network graph with a node represents a provincial administrative region in China. The node colour represents the range of the number of plant species in each provincial administrative region. The distance between the nodes represents the similarity in plant species between different provincial administrative regions. A closer distance between the two nodes indicates a higher similarity in the plant species between the two provincial administrative regions and vice versa.
Clustering classifies a collection of physical or abstract objects into multiple classes of similar objects. The cluster generated by clustering is a set of data objects. These objects are similar to those in the same cluster but different from those in the other clusters. Taking the similarity in plant species between various provincial administrative regions, we clustered the provincial administrative regions in China into three categories (Figure 8), which represent southwestern China and south of the Five Ridges (including Tibet, Yunnan, Sichuan, Guangdong, and Guangxi), central and southern China and regions south of the Yangtze River (including Hunan, Hubei, and Jiangxi), northern China (including Heilongjiang, Jilin, Liaoning, and Hebei), respectively. Hong Kong, Macau, and Ningxia were not representative because of the small area and few plant species. Thus, it was not considered separately in this study: we included Hong Kong and Macau in Guangdong Province and Ningxia in Gansu Province.
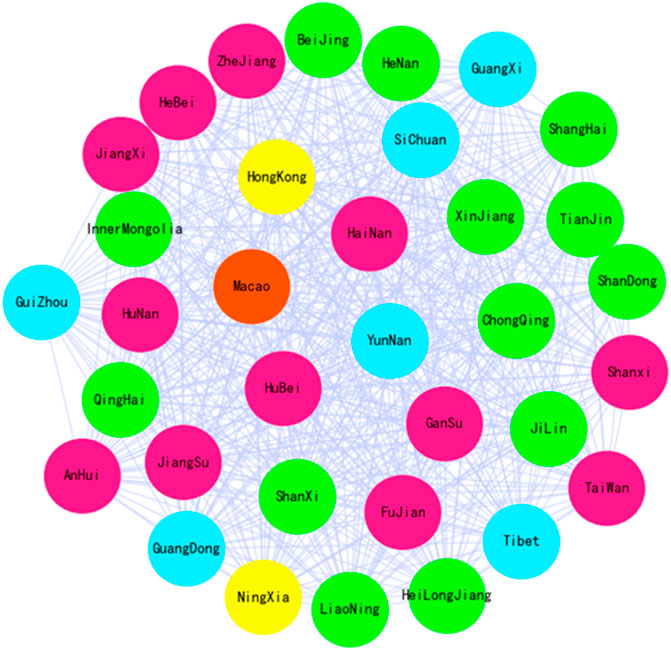
FIGURE 8. Cluster graph shows nodes representing China’s provincial administrative regions. The node colour indicates the category of the provincial administrative region. There are five categories, and the same colour indicates that these provincial administrative regions are classified as the same category according to their similarity.
3.2 Analysis and discussion on plant growth environment and propagation route in China
China has vibrant biodiversity. It features a north-south and east-south span with a distance of more than 4,000 km. There are different sizes of mountains across the country, forming a substantial environmental gradient. Such geographical conditions enable ecosystems from tropical rain forests to cold temperate forests, alpine vegetation, and arid deserts, covering almost all types of ecosystems. The distribution of plant diversity in China is very uneven. Plants are mainly concentrated in the southwest, south, and central China at the north latitude range of approximately 20°–35°. Hengduan Mountains spread across provinces and autonomous regions such as Tibet, Yunnan, and Sichuan, showing prominent diversity and endemism distribution (Yu et al., 2014).
Plant growth factors include light, water, air, temperature, and soil. According to the analysis of the biodiversity data of various provinces in China, the top five provincial administrative regions in China regarding the number of plant species, Tibet, Sichuan, Yunnan, and Guizhou belong to the southwestern region. In contrast, Guangxi, another province in the top five in plant species, is also geographically adjacent to Guizhou Province and Yunnan Province. According to the general geographical division, the Guangxi provincial administrative region also belongs to the southwest. It suggests that southwestern China has considerably abundant plant species. The southwest region in the subtropical and part of the tropical regions rarely suffers from catastrophic climate changes due to the subtropical and tropical biotic communities, long evolution time, and stable environmental conditions during the geological age; the communities are highly diverse. The southwest region with high macro and microheterogeneity has highly complex and diverse habitat types. Thus, it provides a place for diverse plants to grow. Its good water and heat conditions also contribute to the region’s high productivity and energy, thus supporting more creatures. These are consistent with traditional views.
Chen et al. reported that high species diversity might result from the recent rapid species formation in the ‘cradle’ or the long-term accumulation and preservation of species in the ‘museum’. China almost has the most diverse plant distributions in the world. Because many species have an ancient origin, China is often known as a museum of plants. It has many species because of the changes in the terrain or climate (for instance, the formation of the Qinghai–Tibet plateau and monsoon have provided new habitats and promoted the significant radiation). China is also recognised as the cradle of plant evolution. Furthermore, eastern China represents the botanical museum, while western China is the cradle of evolution. Species differentiation or new species mainly occur in western China, such as the Qinghai–Tibet Plateau, which gradually migrates or radiates to the other parts of China. Many plants widely distributed in China are initially differentiated or produced in western China (Lu et al., 2018).
Plants radiate or migrate from western China, such as the Qinghai–Tibet Plateau, to the other parts of China. The possible routes are the Qinghai–Tibet Plateau–Gansu–Shaanxi–Shanxi or Henan–North China or Northeast China, the Qinghai–Tibet Plateau–Sichuan–Chongqing–Hubei or Hunan–East China or South China, Qinghai–Tibet Plateau–Sichuan–Yunnan–Guizhou or Guangxi–Guangdong–South China or East China. Theoretically speaking, the first route is the shortest, and plants will first choose this route according to proximity. The third route is the longest and the most tortuous; therefore, plants are less likely to choose this route for migration. Nonetheless, from the perspective of the similarity-based clustering of plants in different provinces in China, Tibet, Sichuan, Yunnan, Guizhou, Guangxi, and Guangdong are clustered as a category, indicating an extremely high similarity between them exactly suggests that plants have chosen the third migration route. For example, if a plant enters Hubei or Hunan from Sichuan, it will inevitably pass through Chongqing, but Chongqing, which also belongs to the southwest region, merely has 883 plant species.
Moreover, from the perspective of the similarity shown in the network graph, the similarity in plant species is not high between Chongqing and the other four provincial administrative regions in the southwest region. Judging from the cluster graph, Chongqing is also in the same category as Henan, Shandong, and Beijing, but not in the same category as Southwest China. In other words, the plants did not migrate via the second route.
We found through a survey that the formation of the Qinghai–Tibet Plateau was inextricably linked to the Himalayan orogeny. This movement caused uplifted folds in the ancient Mediterranean (Tethys) of the Mesozoic East-West tiles, forming a tall, young fold mountain range across the East and the West. Under the Himalayan orogeny, the Qinghai–Tibet Plateau quickly rose to form a large detachment structure. A series of near east–west-directed overthrust faults developed above the detachment surface, of which the substantial Hengduan mountains east of the Qinghai–Tibet Plateau were particularly prominent. Under the background of the Himalayan orogeny and the combined influence of various factors, various climates and landforms are formed in the Qinghai–Tibet Plateau, which has become the main birthplace of plants in China because of its sufficient light and geothermal resources. By studying the similarity of plant species between provinces, this study revealed that plants initially spread from the Qinghai–Tibet Plateau, mainly along the Hengduan Mountains, and then passed through Yunnan, Sichuan, etc. However, biological species are prone to geographic isolation because of the mountain’s violent ups and downs and the big altitude difference. As a result, the plants’ propagation through the Hengduan Mountains quickly causes the generation and differentiation of numerous new species of animals and plants. Therefore, plant diversity may exceed its origin when plants spread to Sichuan and Yunnan through the Hengduan Mountains. After a plant spreads to Yunnan, it spreads along with Yunnan to Guizhou, Guangxi, Guangdong, etc. Yunnan becomes the most critical source of intermediate propagation in the propagation process because of its rich plant diversity. Hence, Sichuan and these places have a higher similarity of the plant species than Guizhou, Guangxi, Guangdong, etc.
The Hengduan Mountains (range), one of the youngest mountain ranges in the world, is the longest, most comprehensive, and the most typical north–south mountain range in China, the only area with both the Pacific and the Indian Ocean water systems. Located in the southeast of the Qinghai–Tibet Plateau, it is usually a general term for the north–south mountain ranges in the west of Sichuan and Yunnan and the east of the Tibet Autonomous Region. Moreover, located in the border area of Tibet, Qinghai, Sichuan, and Yunnan, it is the only route for plants to migrate from the Qinghai–Tibet Plateau to Sichuan, Guizhou, and Yunnan. Although the Hengduan Mountains only account for a small part of land of China, it is one of the regions with the richest and the most concentrated biodiversity in China and the world, known as both a ‘plant kingdom’ and an ‘animal kingdom’ (Qiu et al., 2005; Zhang et al., 2009; He and Jiang, 2014; Wen et al., 2016; Dong et al., 2020; Li et al., 2020). Biological, species evolution science and paleogeology have widely considered the Hengduan Mountains a vital channel for biological migration in China. Zhang (Zhang, 2002) once proposed the biological corridor hypothesis and the refuge hypothesis, considering the north–south-directed Hengduan Mountains as the migration pathways for animals in the Paleo-Northern and oriental realms, which means that the creatures of the two realms coexist here. By using a cluster similarity analysis of the plant species in the various provinces, this study found that most plants did enter Yunnan through the Hengduan Mountains during migration and then spread to Guangxi and Guangdong and then to the north in a roundabout manner, thus forming the current plant diversity distribution pattern in China (Figure 9).
4 Conclusion
Biodiversity is often related to the healthy growth of different plant species linked with carbon absorption and soil carbon storage. This paper intuitively reviews the current distribution characteristics of plant diversity in China based on extensive data statistics, comparisons, and material references. Chinese plants’ origins and propagation routes and the reasons for their current distribution characteristics are discussed and analysed based on the cluster spectrum analysis of the plant species similarity.
It obtained the plant diversity distribution data in China, and analysis indicated that plant diversity in Yunnan records the highest, followed by Sichuan and Tibet, meaning that the Southwestern part of China has the most significant plant diversity. Tibet and Yunnan have backward economic development and hence experience a lower level of human destruction on plant diversity. On the other hand, Sichuan’s economic development is quite good. The land of abundance still has a wide variety of plants owing to excellent irrigation projects since 256BC. It showcases the importance of facilities in reducing the harmful effects due to human activities after years. It offers crucial insights to government officials and policymakers when they spend money on public irrigation and water facilities and implement related water policies in the modern days: the benefits can last for many years.
Besides, the Hengduan Mountains were the main route for Chinese plants to migrate from the west to the east and an important reason for forming Chinese biodiversity. This study provides a more intuitive and sufficient basis for the research on the plant distribution characteristics in China and the causes of the distribution pattern. In addition, the methods adopted provide new ideas for future biodiversity research. For example, how does the direction of plant migration affect future Sustainability and Green Development? Suppose different types of plants absorb different levels of heat. When we raise the scale of research to the global landscape, are there any implications of plant diversity on the heat island effect and global warming?
Data availability statement
The raw data supporting the conclusions of this article will be made available by the authors, without undue reservation.
Author contributions
PL, RL, HC, YC, MK-writing, data collection and visualisation. All authors contributed to the article and approved the submitted version.
Funding
The authors are indebted to the support of the Ministry of Education’s “Research on the Institutional Construction of Medical-Pension Integration and Old-age Security” (18YJA790065), the Anhui Provincial Social Science Planning Fund’s major project “Anhui Province’s Comprehensive Construction of a Well-off Society” (AHSKZD2019D04), the Anhui Provincial Social Science Planning Project “Research on the Obstacles and Pathways of Migrant Workers’ Family Migration in Anhui Province” (AHSKY2020D44), and the Key Scientific Research Project of Anhui University of Finance and Economics “Research on Language Skills, Education Human Capital and Migrant Workers’ Income” (ACKYB20016), Anhui Provincial Teaching Quality Project “Western Culture Tutorial Ideological and Political Demonstration Course” (2020szsfkc0005), “Innovation Project for cultivating Top-notch Students in Basic Disciplines of English Major” (2020zyrc023), Anhui University of Finance and Economics Outstanding Talent Training Innovation Project “Business English Professional Top Talent Education and Training Program” (aclzy2020009), Anhui Provincial Teaching Quality Project “Social Security Professional Teaching Team” (2019jxtd056), “Innovation Project for Cultivating Top-notch Students in Basic Disciplines of Labor and Social Security” (2020zyrc018), “Demonstration Course on Thinking about Politics in Public Economics Courses” (2020szsfkc0004), “Virtual Teaching and Research Department for Labor and Social Security Majors” (acxnjys2021013), “Demonstration Course on Public Economics Thinking and Politics” for Teaching Quality Engineering of Anhui University of Finance and Economics (ackcsz2020015), and “Research on Effective Online Teaching of Public Foreign Language Courses for Postgraduates in the Context of Major Emergencies” (cxjhjyzdi2001) supported by Anhui University of Finance and Economics.
Acknowledgments
The authors would like to thank reviewers’ useful comments.
Conflict of interest
The authors declare that the research was conducted in the absence of any commercial or financial relationships that could be construed as a potential conflict of interest.
Publisher’s note
All claims expressed in this article are solely those of the authors and do not necessarily represent those of their affiliated organizations, or those of the publisher, the editors and the reviewers. Any product that may be evaluated in this article, or claim that may be made by its manufacturer, is not guaranteed or endorsed by the publisher.
References
Ahmadi, M., Taghavirashidizadeh, A., Javaheri, D., Masoumian, A., Jafarzadeh Ghoushchi, S., and Pourasad, Y. (2021, in press). DQRE-SCnet: A novel hybrid approach for selecting users in federated learning with deep-Q-reinforcement learning based on spectral clustering. J. King Saud Univ. - Comput. Inf. Sci.. doi:10.1016/j.jksuci.2021.08.019
Chen, L., Msigwa, G., Yang, M., Osman, A. I., Fawzy, S., Rooney, D. W., et al. (2022). Strategies to achieve a carbon neutral society: A review. Environ. Chem. Lett., 1–34. doi:10.1007/s10311-022-01435-8
Chen, S., Ouyang, Z., Fang, Y., and Li, Z. (2011). Geographic patterns of endemic seed plant genera diversity in China. Biodivers. Sci. 19 (4), 414–423. doi:10.3724/sp.j.1003.2011.10289
Chen, S., Wang, W., Xu, W., Wang, Y., Wan, H., Chen, D., et al. (2018). Plant diversity enhances productivity and soil carbon storage. Proc. Natl. Acad. Sci. U. S. A. 115 (16), 4027–4032. doi:10.1073/pnas.1700298114
Cheng, X.-L., Padullés Cubino, J., Balfour, K., Zhu, Z.-X., and Wang, H.-F. (2022). Drivers of spontaneous and cultivated species diversity in the tropical city of Zhanjiang, China. Urban For. Urban Green. 67, 127428. doi:10.1016/j.ufug.2021.127428
Danise, T., Innangi, M., Curcio, E., and Fioretto, A. (2022). Covariation between plant biodiversity and soil systems in a European beech forest and a black pine plantation: The case of mount faito, (campania, southern Italy). J. For. Res. (Harbin). 33 (1), 239–252. doi:10.1007/s11676-021-01339-3
Dong, F., Hung, C.-M., and Yang, X.-J. (2020). Secondary contact after allopatric divergence explains avian speciation and high species diversity in the Himalayan-Hengduan Mountains. Mol. Phylogenetics Evol. 143, 106671. doi:10.1016/j.ympev.2019.106671
Du, H., Chen, Z., Mao, G., Li, R. Y. M., and Chai, L. (2018). A spatio-temporal analysis of low carbon development in China’s 30 provinces: A perspective on the maximum flux principle. Ecol. Indic. 90, 54–64. doi:10.1016/j.ecolind.2018.02.044
ECharts (2021). Apache ECharts [online]. Availableat: https://echarts.apache.org/zh/download-map.html.
Editorial Committee of flora of China Chinese Academy of Sciences (2004). Flora of China. China: Science Press.
He, K., and Jiang, X. (2014). The Sky Islands of Western China: An overview of systematic geography. Sci. Bull. 59 (12), 1055–1068.
Li, G., Shen, Z., Ying, T., and Fang, J. (2009). The spatial pattern of species richness and diversity centers of gymnospermin China. Biodivers. Sci. 17 (3), 272. doi:10.3724/sp.j.1003.2009.08327
Li, J., Milne, R. I., Ru, D., Miao, J., Tao, W., Zhang, L., et al. (2020). Allopatric divergence and hybridization withinCupressus chengiana(Cupressaceae), a threatened conifer in the northern Hengduan Mountains of Western China. Mol. Ecol. 29 (7), 1250–1266. doi:10.1111/mec.15407
Liu, E., Zhang, Z. Z., Cheng, X., Liu, X., and Cheng, L. (2020). SCNrank: Spectral clustering for network-based ranking to reveal potential drug targets and its application in pancreatic ductal adenocarcinoma. BMC Med. Genomics 13 (5), 50. doi:10.1186/s12920-020-0681-6
Lu, L.-M., Mao, L.-F., Yang, T., Ye, J.-F., Liu, B., Li, H.-L., et al. (2018). Evolutionary history of the angiosperm flora of China. Nature 554 (7691), 234–238. doi:10.1038/nature25485
Luo, F., Li, R. Y. M., Crabbe, M. J. C., and Pu, R. (2022). Economic development and construction safety research: A bibliometrics approach. Saf. Sci. 145, 105519. doi:10.1016/j.ssci.2021.105519
Luo, Y.-M., Liu, W., Yue, X.-G., and Rosen, A. M. (2020). Sustainable emergency management based on intelligent information processing. Sustainability 12 (3), 1081. doi:10.3390/su12031081
Muehlethaler, C., and Albert, R. (2021). Collecting data on textiles from the internet using web crawling and web scraping tools. Forensic Sci. Int. 322, 110753. doi:10.1016/j.forsciint.2021.110753
Park, J.-H., and Kwon, H.-Y. (2022). Cyberattack detection model using community detection and text analysis on social media. ICT Express. doi:10.1016/j.icte.2021.12.003
Qiu, Z., Li, C., and Net, Z. (2005). Evolution of Chinese mammalian faunal regions and elevation of the Qinghai-Xizang (Tibet) Plateau. Sci. China Ser. D-Earth. Sci. 48 (8), 1246–1258. doi:10.1360/03yd0523
Song, L., Li, R. Y. M., and Yao, Q. (2022). An informal institution comparative study of occupational safety knowledge sharing via French and English tweets: Languaculture, weak-strong ties and AI sentiment perspectives. Saf. Sci. 147, 105602. doi:10.1016/j.ssci.2021.105602
Wen, J., Xu, Y., and Liu, H. (2020). Incomplete multiview spectral clustering with adaptive graph learning. IEEE Trans. Cybern. 50 (4), 1418–1429. doi:10.1109/TCYB.2018.2884715
Wen, Z., Yang, Q., Quan, Q., Xia, L., Ge, D., Lv, X., et al. (2016). Multiscale partitioning of small mammal β-diversity provides novel insights into the Quaternary faunal history of Qinghai–Tibetan Plateau and Hengduan Mountains. J. Biogeogr. 43 (7), 1412–1424. doi:10.1111/jbi.12706
Ying, J. (2001). Species diversity and distribution pattern of seed plants in China. Biodivers. Sci. 9 (4), 393–398. doi:10.17520/biods.2001058
Yu, H., Zhang, Y. l., Liu, L. S., and Qi, W. (2014). Spatial genetic patterns of four alpine plants in Tibetan Plateau based on GIS. Chin. J. Ecol. 33 (5), 1157–1163.
Zhang, D.-C., Boufford, D. E., Ree, R. H., and Sun, H. (2009). The 29°N latitudinal line: An important division in the hengduan mountains, a biodiversity hotspot in southwest China. Nordic J. Bot. 27 (5), 405–412. doi:10.1111/j.1756-1051.2008.00235.x
Zhang, J., Lu, C., Wang, J., Wang, L., and Yue, X.-G. (2019). Concrete cracks detection based on FCN with dilated convolution. Appl. Sci. 9 (13), 2686. doi:10.3390/app9132686
Zhang, J., Lu, C., Wang, J., Yue, X.-G., Lim, S.-J., Al-Makhadmeh, Z., et al. (2020). Training convolutional neural networks with multi-size images and triplet loss for remote sensing scene classification. Sensors 20 (4), 1188. doi:10.3390/s20041188
Zhang, R. (2002). Geological events ad mammalian distribution in China. Chin. J. Zoology 48 (2), 141–153.
Keywords: plant diversity distribution, spectral clustering, hengduan mountains, himalayan orogeny, carbon neutrality
Citation: Li P, Li RYM, Chen H, Cao Y and Kaabar MKA (2022) Achieving carbon neutrality in China: Spectral clustering analysis of plant diversity. Front. Environ. Sci. 10:955884. doi: 10.3389/fenvs.2022.955884
Received: 31 May 2022; Accepted: 11 July 2022;
Published: 07 September 2022.
Edited by:
Xiao-Guang Yue, European University Cyprus, CyprusReviewed by:
Jiajun Zhao, Guangxi Arts University, ChinaHu Jiachong, Jiangxi University of Finance and Economics, China
Copyright © 2022 Li, Li, Chen, Cao and Kaabar. This is an open-access article distributed under the terms of the Creative Commons Attribution License (CC BY). The use, distribution or reproduction in other forums is permitted, provided the original author(s) and the copyright owner(s) are credited and that the original publication in this journal is cited, in accordance with accepted academic practice. No use, distribution or reproduction is permitted which does not comply with these terms.
*Correspondence: Hong Chen, Q2hlbmhvbmc4OF9jaGluYUAxNjMuY29t