- 1Department of Molecular Biology and Biochemistry, Simon Fraser University, Burnaby, BC, Canada
- 2Department of Biochemistry and Microbiology, University of Victoria, Victoria, BC, Canada
- 3Group for Research and Education on Marine Mammals, Tadoussac, QC, Canada
- 4Centre de recherche en toxicologie de l’environnement (TOXEN), Département des sciences biologiques, Université du Québec à Montréal, Montréal, QC, Canada
The endangered beluga (Delphinapterus leucas) population in the St. Lawrence Estuary (SLE) in eastern Canada, the largest estuary in the world, is declining. Elevated tissue concentrations of a wide range of environmental contaminants, for example, halogenated flame retardants (HFRs) including polybrominated diphenyl ethers (PBDEs), might play a role in the non-recovery of this population. In mammals, HFRs have been reported to impair the metabolic regulation, including amino acid and fatty acid pathways. In the present study, we collected both blubber and skin swab samples from tissue biopsies from 56 adult SLE belugas and analyzed their blubber for the concentrations of a comprehensive suite of PBDEs and other HFRs. Using 16S rRNA marker and shotgun metagenomic approaches using skin swabs, we investigated, for the first time, the SLE beluga skin microbiome and the SLE water microbiome, providing valuable comparative taxonomic and functional microbiome information. We found that belugas have a unique skin microbiome that is distinct from surrounding SLE water, regardless of the beluga sex or location in the SLE. We further characterized the core microbiome of SLE beluga skin and surrounding SLE water, and identified bacterial taxa and gene functional pathways associated with the skin microbiome that correlated with beluga blubber HFR concentrations. Namely, we identified the phylum Nitrospinae and candidate phylum PAUC34f as potential taxa of interest that are associated with blubber HFR concentrations. We hypothesize that the biodegradation of HFRs within the beluga blubber and skin results in an increase in local metabolite concentrations that leads to the proliferation of Nitrospinae and PAUC32f. This work demonstrates the utility of studying the core microbiome of the SLE beluga skin using a swab method that could be adapted to field sampling. Further studies of the temporal effects of contaminant exposure on SLE beluga skin and SLE water microbiomes is warranted for potentially better monitoring and protecting this marine mammal which is at risk.
1 Introduction
Belugas (Delphinapterus leucas) are cetaceans that are well adapted to life in cold waters. In the St. Lawrence Estuary (SLE) in eastern Canada, there is a small resident population of belugas, geographically isolated from other populations. In 1885, there were >10,000 belugas in the SLE, but there has been a rapid decline in their population size due to commercial hunting that depleted this population to <1,000 by 1979 (Mosnier et al., 2015). While hunting has been banned since 1979, the SLE beluga population has shown no signs of recovery in the last four decades (Hammill, 2007; Simond et al., 2017). The last census in 2012 identified less than 900 mammals in the SLE and documented a slow population decline of 1% per year (Mosnier et al., 2015). This trend has likely continued given the abnormally elevated number of calves found dead in recent years (Fisheries, 2017). The SLE beluga population is classified as endangered under the Committee on the Status of Endangered Wildlife (COSEWIC) in Canada and the federal Species at Risk Act (SARA) since 2015 and 2017, respectively (Fisheries, 2017). The non-recovery of the SLE beluga population has mainly been tied to the impact of anthropogenic activities. The potential factors contributing to this decline were identified as increased maritime traffic and commercial activities, food scarcity, toxic algal blooms, climate warming, and most importantly, exposure to high levels of environmental contaminants (Hammill, 2007; Lebeuf et al., 2014; Lesage et al., 2020).
The SLE beluga’s critical habitat (Figure 1) is located downstream of the Laurentian Great Lakes, and the St. Lawrence River in Canada and the United States. It is surrounded by several large cities and agricultural regions, and is located in an urbanized waterway exposed to a plethora of both organic and inorganic contaminants released by human activities. Halogenated flame retardants (HFRs) such as polybrominated diphenyl ethers (PBDEs) and other HFRs, including replacement chemicals known as emerging HFRs, have been reported at high levels in SLE beluga blubber. These chemicals are known to disrupt the regulation of thyroid and steroid hormones in a range of experimental animal models and wildlife species including marine mammals (Zhang et al., 2016; Thambirajah et al., 2022). Recently, studies have examined the metabolomic profiles of SLE belugas with respect to organohalogen contaminant exposure and identified potential mechanisms of toxicity and metabolic disruption (Simond et al., 2020). By examining the skin biopsy concentrations of targeted metabolites, ecological factors such as local prey availability and diet composition played a key role in explaining the metabolite profiles of belugas (Simond et al., 2020). Furthermore, the concentrations of HFRs were notably greater in SLE belugas compared to other beluga populations from the Arctic; however, no significant PBDE concentration trends in the blubber were observed, perhaps due to the recent introduction of PBDE regulations globally (Simond et al., 2017).
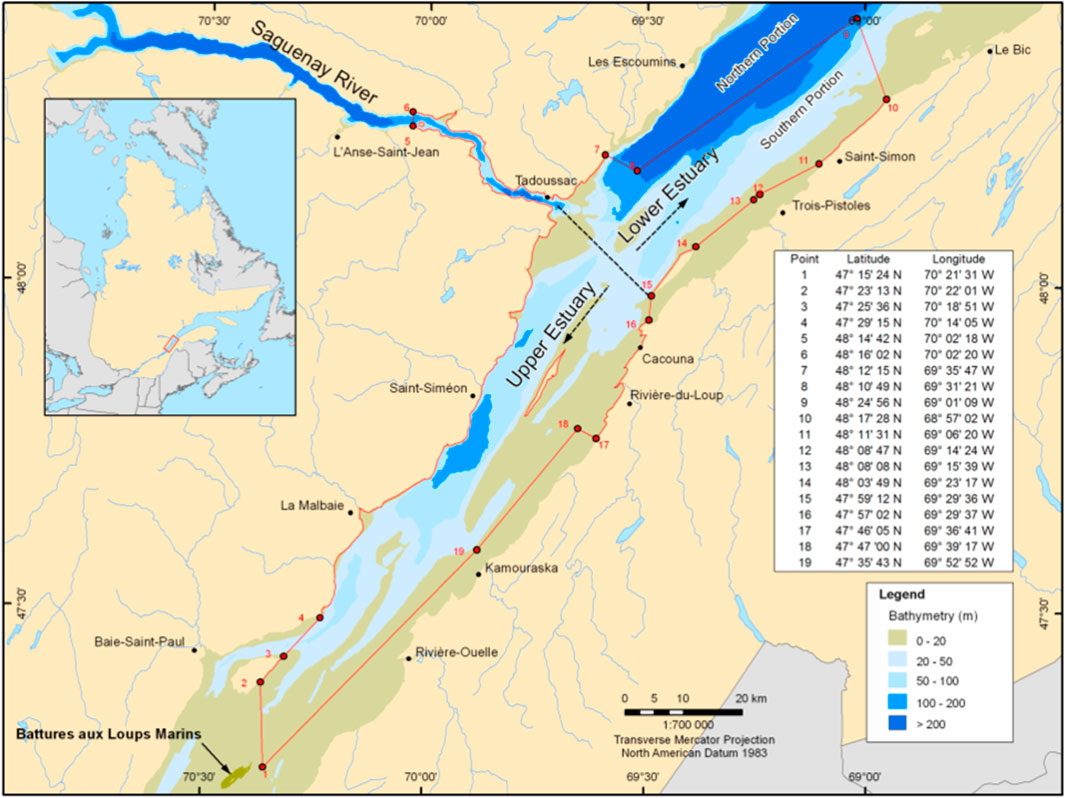
FIGURE 1. Critical habitat of the beluga (Delphinapterus leucas) in the St. Lawrence Estuary (SLE) (Fisheries, 2017). The beluga is a marine mammal well adapted to life in cold waters. A small, endangered population resides within the SLE in eastern Canada, geographically isolated from other populations. Two critical habitats exist in the upper and lower estuary, and the depths are as indicated by the figure legend. The coordinates of each habitat are marked by the red polygon.
The skin is often associated with highly diverse microbial communities that are host-species specific and distinct from their surrounding habitat (Chiarello et al., 2017). While the characterization of skin microbiome has largely been focused on humans and model organisms to date (Grice et al., 2009; Ross et al., 2019), there is increasing interest in exploring the skin microbiota of wildlife species. The skin usually acts as the first line of defense from surrounding contaminants to modulate immunity and support antagonistic effects against stressors (Cogen et al., 2010; Nakatsuji et al., 2013; Wang et al., 2017). Studies have shown that the skin surface microbiota is dynamic in both vertebrates and invertebrates. As reviewed by Ross et al. (2019), the skin microbiome of vertebrates, including amphibians, reptiles, aquatic/terrestrial mammals, and fish, was linked to changes in the host life stages, diet, environmental pollution, health state, and geographical locations. A similar linkage was also observed in invertebrates. In coral reefs, water temperature, overfishing, and nutrient pollution were shown to destabilize microbiomes and elevate putative pathogen loads (Lema et al., 2014; Salerno et al., 2016). Furthermore, while the coral reef community can be dynamic, bacteria with likely important functional roles remained stable throughout their life stages (Zaneveld et al., 2016). Past studies have well demonstrated the ability of the microbiome to act as a biomarker for the association with certain phenotypes. For example, gram-negative bacteria were associated with halitosis and periodontitis in the human mouth (Dewhirst et al., 2010), betaproteobacteria were associated with increased parasite susceptibility in honeybees (Schwarz et al., 2016), and Gallionellaceae were associated with copper-contaminated water streams (Rossum et al., 2016).
A previous study (Cise et al., 2020) compared the derived microbiomes from samples collected over a multi-year span from a stable Alaskan beluga population (Bristol Bay) to an endangered population (Cook Inlet). Using amplicon sequence variant (ASV) analyses based upon the 16S rRNA gene, the researchers identified considerable microbiome variability amongst the two beluga populations. A comparison of 14 healthy to 15 belugas with skin disease in the Bristol Bay population revealed no significant differences in potential pathogenic ASV composition but did show significant differences in abundance of 11 genera including Klebsiella and Psychrobacter spp. This study was limited to ASV analyses and did not generate functional metagenome profiles. Moreover, it was not possible to determine if the variability observed in the populations’ microbiomes were influenced by the surrounding seawater microbiota as no seawater controls were used.
In the present study, we hypothesized that the skin microbiome of SLE belugas obtained from surface swabs is influenced by their exposure to elevated concentrations of a range of HFRs, either through contact with the water or the animals’ innate defense against accumulated HFRs in their tissues. Thus, the objectives of the present study were to 1) characterize the SLE belugas’ skin microbiome, versus SLE water microbiome, and describe the core skin microbiota with respect to both taxa and functional profiles using 16S and shotgun metagenomic sequencing, respectively; 2) understand the potential linkages between the blubber concentrations of HFRs and the skin microbiota in this highly contaminant-exposed population; and 3) identify potential microbial taxa within the skin microbiota that correlate with the blubber HFR levels. To our knowledge, this is the first study that assesses the skin microbiome of the SLE beluga population with the additional unique attribute of assessing the burden of contaminant exposure on the microbiome.
2 Methods
2.1 Field sampling
A total of 42 male and 14 female belugas from the SLE were biopsied from the dorsal region in September 2016 and 2018 using sharpened 8 × 25 or 8 × 35 mm stainless steel tips, pre-cleaned with acetone, 95% ethanol and Virkon, and fired from a MK24C Paxarms dart projector with 0.22 caliber blank charges (Domett, New Zealand). Each biopsy consisted of the outer skin layer and inner blubber layers. Immediately after sampling, the swab samples from the biopsy skin surface (8 mm diameter) were obtained using sterile cotton tipped applicators, transferred into sterile cryovials, and stored in liquid nitrogen in the field and at −80°C in the laboratory until DNA extraction (Methods 2.3). For each beluga skin swab, four swabs from the SLE water in which each beluga was found were also collected as background controls as well as four unused swabs that were only exposed to ambient air as additional controls. Control swabs were similarly transferred into sterile cryovials and stored in liquid nitrogen in the field and at −80°C in the laboratory until DNA extraction. Blubber from each biopsy was then separated from the skin using disposable DNAse/RNAse-free scalpels and forceps pre-cleaned with acetone and 70% ethanol, transferred into cryovials, and stored in liquid nitrogen in the field and at −80°C in the laboratory until chemical analysis (Methods 2.2). The biopsy skin was also stored in cryovials in liquid nitrogen in the field and at −80°C in the laboratory until sexing. The GPS coordinates of the biopsy sampling location were recorded for each beluga. Animal age was not determined.
The present study was conducted under permits granted by Parks Canada (SAGMP-2013-14734) and Fisheries and Oceans Canada (IML-2015-13 and IML-2016-021). The sampling methods were approved by the animal care committee of Fisheries and Oceans Canada, which is accredited by the Canadian Council on Animal Care (Ottawa, ON, Canada).
The sampling locations were recorded and used to classify the belugas into sampling areas using two methods. For the first grouping, each sample was identified as originating from either the upper or lower estuary (Figure 1) (Canada and Change, 2015). Then, each sample was segregated into upper versus lower estuary as shown in Figure 1. For the second grouping, the belugas were clustered in an area identified by their nearest land marker (see Supplementary Table S1).
2.2 Chemical analysis
The SLE beluga blubber samples from the 42 males (samples for females had been used for other analyses) were analyzed for 33 PBDE congeners and 12 other HFRs at the Université du Québec à Montréal (Montréal, QC, Canada; Supplementary Table S2). Methods for sample extraction and clean-up as well as HFR identification and quantification were described by Simond et al. (2020) and applied with the following minor modifications. Briefly, blubber aliquots (50–75 mg) were homogenized with diatomaceous earth (J.T. Baker, Phillipsburg, NJ, United States) and spiked with an internal standard solution (BDE-30, BDE-156, 13C-BDE-209, and 13C-syn-DP). Extraction was performed using n-hexanes:dichloromethane (1:1, volume ratio) using a pressurized liquid extraction system (Fluid Management Systems, Watertown, MA, United States), and the extracts were cleaned up using PBDE-free acid-basic-neutral silica and neutral alumina columns (Fluid Management Systems). The total extractable lipid content was measured gravimetrically. Analytes were identified and quantified using a gas chromatograph coupled into a single quadrupole mass spectrometer (Agilent Technologies 5975C Series, Palo Alto, CA, United States) operating in an electron capture negative ionization mode (GC/MS-ECNI). The column was a fused silica DB-5 HT capillary column (15 m × 0.2 mm × 0.10 mm; J & W Scientific, Brockville, ON, Canada).
Quality control included method blanks and standard reference material (NIST 1945 Whale Blubber, Gaithersburg, MD, United States). Internal standard recoveries (mean ± SD) were as follows: BDE-30 (96 ± 8%), BDE-156 (99 ± 8%), 13C-BDE-209 (73 ± 19%), and 13C-syn-DP (98 ± 11%). The method limits of detection (MLODs; defined as signal to noise ratio S/N = 3) and the method limits of quantification (MLOQs; minimum amount of analyte producing a peak with S/N = 10) were based on replicate analyses (n = 8) of matrix samples spiked at a concentration of 3–5 times the estimated detection limit. A quantification approach using internal standards was used for HFRs, and hence, all analytes were inherently recovery corrected. All contaminant concentrations were reported in ng/g wet weight (w/w). The sum of all measured PBDE congeners is represented as ∑33PBDE.
2.3 DNA extraction
DNA was extracted from the beluga skin (including both male and female samples), SLE water, and sterile swab samples using the DNeasy PowerLyzer PowerSoil® Kit (Cat.#12855-50; QIAGEN Inc., Mississauga, ON, Canada), according to the manufacturer’s protocol with the following modifications. Briefly, we used filter tips for all pipetting to prevent contamination. Forceps were rinsed in ethanol and flamed before transferring each sample to the glass bead tubes. After adding 750 µl bead solution to samples in the glass bead tube, we vortexed samples for 2 min. The samples were incubated in 60 µl solution C1 of the extraction kit for 10 min at 60°C, then vortexed at maximum speed for 15 min. Following the addition of 100 µl solution C6 of the extraction kit to the spin columns, we incubated the columns for 5 min at room temperature for increased DNA yield. DNA quality and quantity was assessed by NanoDrop (Thermo Fisher Scientific, Surrey, BC, Canada). A positive control of predetermined mock community and negative controls of distilled water and sterile swabs were included along with all samples in the DNA extraction process.
2.4 16S metagenomic sequencing and analysis
16S ribosomal RNA (rRNA) sequencing was performed on 56 beluga skin swab samples (42 male and 14 female) and 52 SLE water samples. Three sterile swabs, four positive controls consisting of a ZymoBiomics predefined communities (Zymobiomics microbial community standard, 2017), and six negative controls (nuclease-free water) were also included as part of the two sequencing experiments. The V6-V7 region of the 16S rDNA was amplified using the primer pair BSF784/15 and R1064/18 (Huber et al., 2007). Forward: BSF784/15: 5′ RGG ATT AGA TAC CC 3′ and Reverse: R1064/18: 5′ CGA CRR CCA TGC ANC ACC T 3′. The standard 314F/784R V3-V4 region primers unexpectedly amplified the beluga host DNA.
After amplification, the amplicons were prepared for sequencing, following the Illumina 16S Metagenomic Sequencing Library Preparation guide. The success of library preparation was verified by agarose gels. Equimolar amount of all samples with an additional PhiX-positive control was pooled and sequenced in two independent runs with the Illumina MiSeq platform using the MiSeq Reagent Kit V2 (Illumina Inc. San Diego, CA, United States), producing 251 bases paired-end reads. The sequence data are available in NCBI BioProject ID PRJNA842171.
Raw sequencing reads were demultiplexed and processed within the QIIME2 (Ver. 2018.4) framework (Bolyen et al., 2019) following the standard workflow. Briefly, amplicon sequence variants (ASVs) were identified with DADA2 (Callahan et al., 2016). Taxonomic assignment of ASVs was done using VSEARCH against the Silva 16s rRNA database (ver. 132, 99%) (Quast et al., 2012). The samples were filtered with respect to the negative control; those samples with less than 1,000 reads were discarded and all unclassified reads were removed from further analysis.
In summary, we identified 13,347 amplicon sequencing variants (ASVs) classified into 35 bacterial phyla and 655 genera across all samples with 18.5M total reads (median reads/sample was 91,317). The ASVs’ taxonomic classification and frequency can be found in Supplementary Table S1. At the taxonomy level, singlet taxa that appeared in only one sample were removed. Alpha diversity of microbiomes was calculated using the Shannon Diversity Index. Compositional dissimilarity was calculated using the Bray–Curtis Dissimilarity Index and visualized using non-metric multidimensional scaling with three ordinates.
2.5 Shotgun metagenomic sequencing and analysis
Forty of the 56 beluga skin swab samples and 17 of the 52 SLE water samples were sequenced using the shotgun metagenomic approach. One of each sterile swab, positive, and negative control were also included. Extracted DNA of each beluga skin and SLE water sample was prepared for sequencing using the Illumina DNA Prep kit (Illumina Inc., San Diego, CA, United States). The success of library preparation was similarly verified by agarose gels. Equimolar amount of 57 skin and water samples with an additional PhiX positive control was pooled and sequenced in two independent runs with the Illumina MiSeq platform using the MiSeq Reagent Kit V3 (Illumina Inc., San Diego, CA, United States), producing 301 base paired-end reads. Following sequencing, reads were trimmed with BBduk (v38.87) (Bushnell et al., 2017). Beluga genomic sequences were removed using bowtie2 (v2.3.4.3) (Langmead and Salzberg, 2012) against the reference beluga genome PRJNA360851A (Jones et al., 2017), following a filtering method that has been utilized and characterized by other shotgun metagenomic projects, for example, in humans, bovines, and worms (Chen et al., 2018; Czajkowski et al., 2018; Yap et al., 2020). Taxonomic and functional profiles of the samples were determined using DIAMOND BLASTX (ver. 2.0.6), in the “more-sensitive” mode, (Buchfink et al., 2014) against NCBI non-redundant and NCBI prokaryotic RefSeq database and summarized using MEGAN6 (Huson et al., 2016). In short, taxonomy of a read was assigned using the source organism of its best aligned hit. Function was assigned using the NCBI COG (Galperin et al., 2020) and the SEED database (Overbeek et al., 2013) of the best aligned hit. Taxa and functions with less than five reads assigned were discarded for downstream analysis.
2.6 Contaminant and metadata correlation analysis
Metadata analysis, including contaminant/microbiome differential abundance analysis, was performed using the 16S-based taxonomic profile and Shotgun metagenomic-based functional profile of the beluga skin microbiome in R (ver 3.4.2) using the libraries phyloseq (McMurdie and Holmes, 2014), microbiomeseq (Ssekagiri et al., 2017), qiime2R, and DESeq2 (Love et al., 2014), and was used to integrate the microbiome data with the sampling metadata, including beluga sex, sampling area, sampling date, and individual HFR concentrations (Supplementary Table S2). All taxa/ASVs that are present in only a single sample were removed to avoid false positive correlations. Pearson correlations between the beluga blubber HFR levels and the taxa relative abundances/functional pathway relative abundances were tested using the 42 male belugas only, as HFR concentrations were only available for males. Multiple testing correction was performed using the Benjamini–Hochberg procedure. Statistical significance was determined when post adjusted p-values were <0.05. All correlations were visualized using GlobeCorr (globecorr.ca), and heatmaps were plotted using R (ver. 3.4.2).
3 Results
3.1 The SLE beluga skin microbiome is unique and distinct from the surrounding SLE water microbiome
The SLE beluga skin microbiome was largely dominated by the phylum Proteobacteria (mean ± SD; 73 ± 24%) together with four other phyla: Actinobacteria (9 ± 10%), Firmicutes (7 ± 16%), Bacteroidetes (6 ± 10%), and Cyanobacteria (5 ± 6%) combined to make up >97% of the SLE beluga skin microbiome (Figure 2A and Supplementary Figure S1). Four genera: Psychrobacter (31 ± 16%), Pseudoalteromonas (11 ± 9%), Escherichia-Shigella (5 ± 7%), and Staphylococcus (4 ± 8%) comprised 50% of all amplicon-sequencing variants (ASVs) identified (Figure 2B). An average of 15 ± 19% of ASVs could not be classified down to the genus level in this community, likely due to the lack of sequence resolution to distinguish between different genera or previously unknown bacteria species.
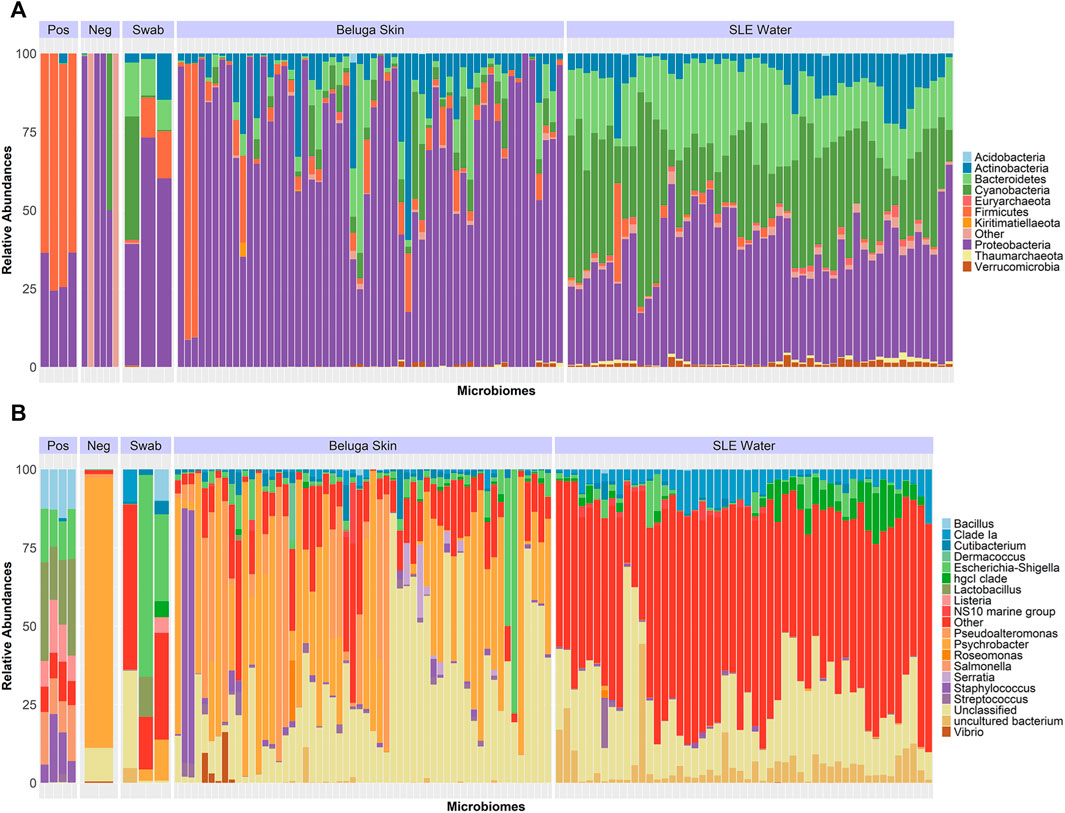
FIGURE 2. Relative abundance of the SLE beluga skin and the SLE water bacterial 16S microbiome. (A) Relative abundance of the top 10 bacterial phyla present across all samples represents >99% of all sequenced reads. The beluga skin microbiome consisted predominately of Proteobacteria (Purple), whereas the SLE water microbiome was dominated by a more diverse combination of Proteobacteria, Cyanobacteria, and Bacteroidetes. (B) Relative abundance of the top 20 most abundant bacterial genera identified represented >99% of all sequences. Four and 36 genera combined represented 50% of the SLE beluga skin and the SLE water microbiomes, respectively. The beluga skin microbiomes consisted of predominantly Psychrobacter (Orange). The water microbiomes did not have any dominant bacterial genera. The relative distribution of taxa in the positive control (Pos) was as expected (Supplementary Figure S2). Sterile swabs and negative controls (Neg) contained <2,000 reads.
In the SLE water microbial community, three phyla, Proteobacteria (38 ± 10%), Cyanobacteria (29 ± 14%), and Bacteroidetes (20 ± 7%), co-existed at relatively similar proportions. Overall, eight phyla made up >97% of the SLE water microbiome (Figure 2A and Supplementary Figure S1). There were no dominant genera in the SLE water microbiome. The most abundant genus was Proteobacteria SAR11 Clade Ia (6 ± 2%), followed by Actinobacteria clade hgcl (4 ± 2%). Thirty-six genera comprised 50% of all ASVs identified in the SLE water (Figure 2B).
Taxon abundance profiles of the positive control samples, consisting of a pre-determined “mock community” of seven bacterial species at known proportions, were found at similar proportions to the referenced ZymoBIOMICs positive control data. Mean deviation from reference was 2–6% (Supplementary Figure S2). Minor variations were likely caused by the sequencing primers targeting a different region of 16S (V3-V4 in ZymoBIOMICs data versus V6-V7 in the present study). Furthermore, the microbiome profile and its taxonomic composition also remained consistent within 35 beluga skin swab samples that were independently sequenced twice (Supplementary Figure S3).
Using 16S taxa abundance, a clear distinction between the SLE beluga skin and the surrounding SLE water microbiomes was observed. The two microbiomes did not share any common bacterial genera that represented >5% abundance. The SLE beluga skin samples, on average, exhibited reduced alpha diversity compared to the SLE water samples (mean Shannon Diversity Index, H = 1.6 and 3.5 ; p < 0.001; Figure 3). However, a higher variation in individual taxa’s relative abundance was found between the SLE beluga skin microbiomes compared to that of the SLE water microbiomes. Using Bray–Curtis dissimilarity index and non-metric multidimensional scaling (NMDS), we fitted the SLE beluga skin and the SLE water microbiomes into three ordinates. Separated clustering of the SLE beluga skin and the SLE water microbiome was observed, especially in the first dimension (stress = 0.128, rmse = 0.006; max residual = 0.034; Figure 4A).
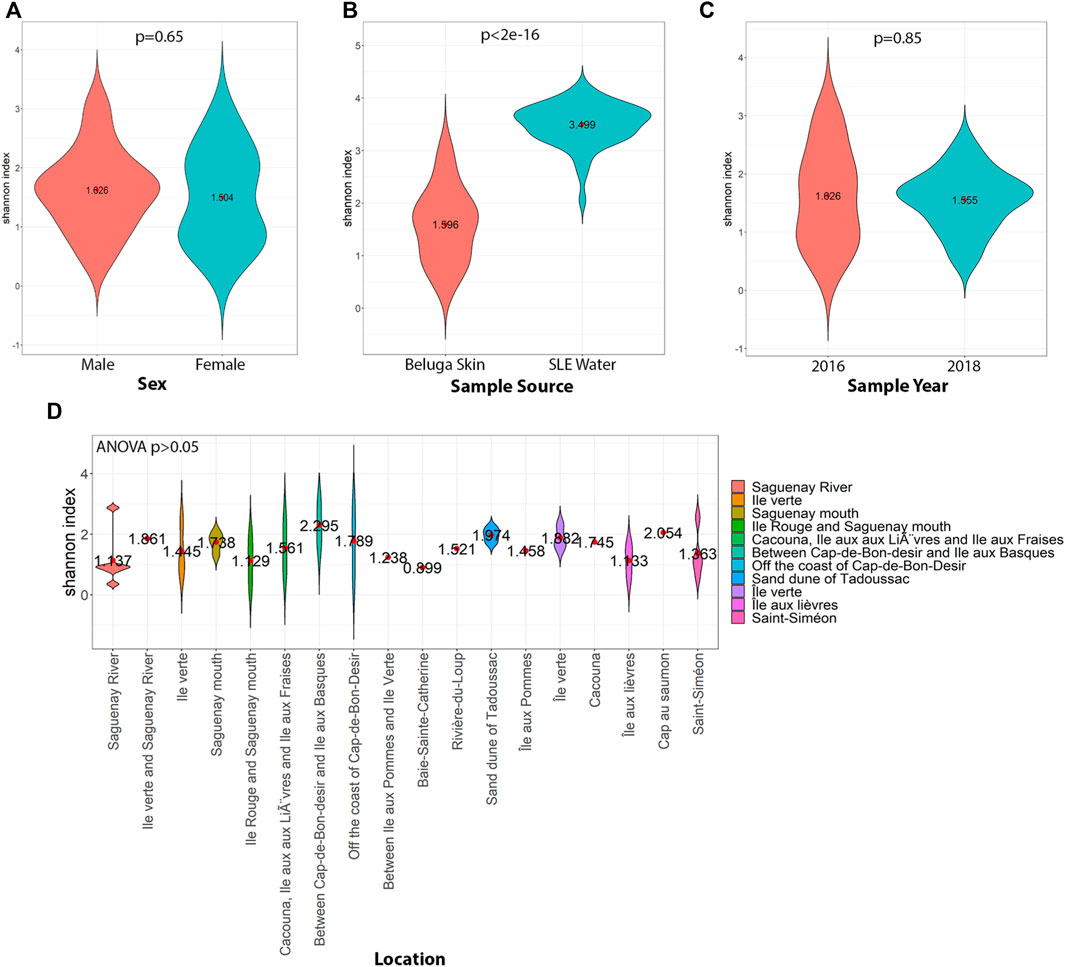
FIGURE 3. Shannon Diversity Index of the SLE beluga skin and the SLE water microbiomes comparing different conditions. (A) Beluga sex was not related to a significant change in diversity. (B) SLE water microbiomes (blue) had a 2-fold increase in diversity compared to the beluga skin microbiome (Orange; p < 0.001). (C) No significant changes in diversity were observed temporally. The skin microbiomes from samples taken in both 2016 and 2018 had similar diversity (Avg. H = 1.6, p = 0.85). (D) For the skin microbiome samples, the area in the St. Lawrence Estuary in which the beluga was sampled resulted in no significant changes in the diversity of the microbiome (Avg H = 1.6; p > 0.1).
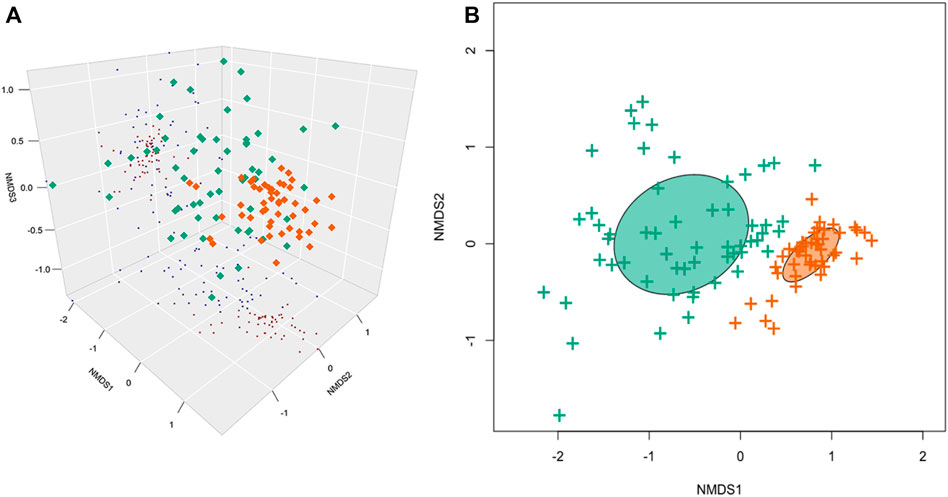
FIGURE 4. Non-metric multidimensional scaling (NMDS) of the SLE beluga skin and the SLE water microbiomes. (A) Three-dimensional NMDS using the Bray–Curtis dissimilarity index indicates that the beluga skin microbiomes (Green) and the SLE water microbiomes (Orange) are significantly different. The first ordinate contributed to the most separation (stress = 0.128, rmse = 0.006; max residual = 0.034). (B) K-means clustering separated all samples into two distinct clusters: one cluster of the beluga skin microbiomes and one of the SLE water microbiomes.
Using the shotgun metagenome, 25% of the SLE water metagenome could be classified as archaea, simple eukaryotes (i.e., algae and fungi) and viruses. Thaumarchaeota (2 ± 2%) was the most abundant archaea; Viridiplantae (2 ± 3%) and Sar (4 ± 5%) were the predominant eukaryotic phyla; and Duplodnaviria (2 ± 3%) and Monodnaviria (1 ± 3%) were dominant viruses (Figure 5). At a lower classification, Thalassiosirales (3 ± 5%), SAR86 (2 ± 2%), and Ostreococcus (2 ± 1%) were the most abundant eukaryotes. Urovircota (3 ± 2%) was the most abundant virus (Figure 5). Similar to the 16S sequencing results, the positive control in shotgun sequencing experiments classified to the genus level at expected proportions (Supplementary Figure S2). The SLE beluga skin samples contained a significant abundance of the beluga host DNA. Specifically, >70% of beluga skin shotgun metagenomes consisted of reads assigned to the superfamily of Delphinoidea, of which the beluga is a part. In total, non-beluga eukaryote organisms made up <4 of the sequenced reads. Interestingly, the virus Cossaviricota and the protozoa Sporadotrichida made up 1% of the skin metagenome (Figure 5). Lastly, we characterized the functional profile of both the bacterial microbiomes of the SLE beluga skin and surrounding the SLE water. Across all samples, 15% of all reads were assigned to a functional pathway using the database of Clusters of Orthologous Groups (COGs) (Supplementary Figure S4).
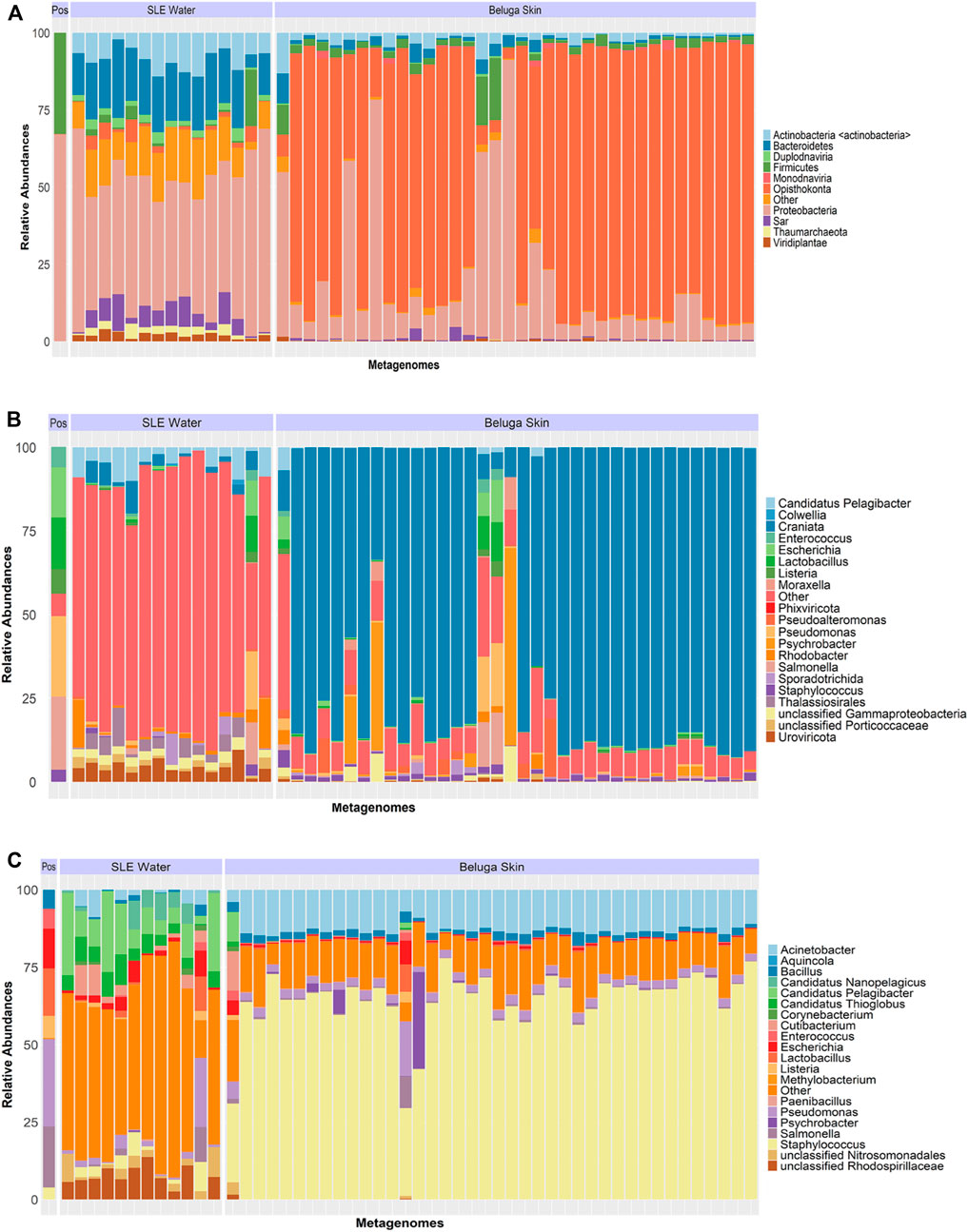
FIGURE 5. Relative abundance of the shotgun metagenome including both bacterial and non-bacterial taxa. (A) Taxa classified at the phylum level showed that the majority of the filtered SLE beluga skin metagenomes were sequences from the phylum Opisthokonta, the superfamily classification in which the belugas belong. Other non-bacterial organisms made up <4% of the community. The SLE water shotgun metagenomes were more diverse with 25% of the community consisting of non-bacterial organism consisting of Sar, Thaumarchaeota, and Viridiplantae. The relative distribution of phylum in the positive control (Pos) contained only bacterial sequences. (B) Majority of non-bacterial sequences in the SLE beluga skin metagenomes were again belonging to a higher classification of beluga whales. Sporadotrichia was the only eukaryotic genus that had >1% in abundance. In the SLE water metagenomes, three eukaryotes (Thalassiosirales, SAR86, and Ostreococcus) made up 5% of the metagenome. Urovircota (3.4%) was the most abundant virus. (C) Focusing only on the bacterial components of the metagenome, Staphylococcus (yellow) had >65% abundance across all beluga samples. The SLE water shotgun bacterial metagenome was more diverse in taxa abundance.
3.2 Changes within the SLE beluga skin microbiome were not associated with sex, sampling location, and sampling year
Within the beluga skin samples, alpha and beta diversities of the microbiomes were not significant between different sampling areas (see Methods 2.1), sexes, or sampling years (Figure 3). Similarly, the compositional dissimilarity NMDS was not able to converge when fitting the dissimilarity matrices using sampling year, sampling area (both upper versus lower estuary classification and nearest land marker classification), and beluga sex using three dimensions. This suggests that variations within the microbiome cannot be resolved using these metadata. As noted previously, the SLE beluga skin microbiome composition was more variable between animals, and thus more dissimilar to each other compared to that of the SLE water across the first two ordinates (Figure 4B). Therefore, the skin microbiome may be influenced by factors other than sex, sampling area, or sampling year.
3.3 The abundance of several bacterial taxa correlates with HFR exposure in the beluga skin microbiome
The phylum Nitrospinae abundance positively correlated with the male SLE beluga blubber ∑33PBDE concentrations (Pearson’s correlation coefficient r = 0.5, p < 0.001) and some individual HFR compounds, namely, anti- and syn-DP (Figure 6). Abundance of seven bacterial genera exhibited positive correlations with the beluga blubber concentrations of ∑45HFR. When considering individual PBDE congeners (Figure 7), three Actinobacteria genera: Williamsia abundance correlated with BDE-139, Scardovia correlated with BDE-138, and the genus CL500-29 marine group correlated with five PBDEs (BDE-47, -66, -99, -100, and -153), and most interestingly, positively correlated with ∑33PBDE in the blubber sample (r = 0.56, p < 0.01). Proteobacteria, Porticoccus, and Nitrospinae LS-NOB further correlated with the emerging HFR pentabromoethylbenzene (PBEB; r = 0.55, p < 0.005). At the species or subspecies level, many taxa with significant correlations between their abundance and blubber HFR concentration were either classified as an unknown and/or uncultured species or could not be identified beyond the genus level. Among those that could be identified, two species under Proteobacteria (Oblitimonas alkaliphila and JGI 0000113-D06) showed moderate correlations with syn- and anti-DP concentrations (r = 0.50 and 0.56, respectively; p < 0.01). However, the average absolute abundance of these two species was less than 100 reads on average across samples, with range of 0–96. Actinobacteria and Bacteroidetes contained the greatest number of genera that correlated with the HFR levels in the SLE beluga blubber with a coefficient of >0.3 (Figure 7A). There was a single functional pathway (carbohydrate transport and metabolism) that negatively correlated with ∑33PBDE (r = −0.65, p < 0.05). Furthermore, a positive correlation (r = 0.81, p < 0.01) between the emerging HFR Dec-604 CB concentrations and the pathway “posttranslational modification, protein turnover, and chaperone” was identified. No significant non-bacterial taxa were correlated with any HFR concentrations.
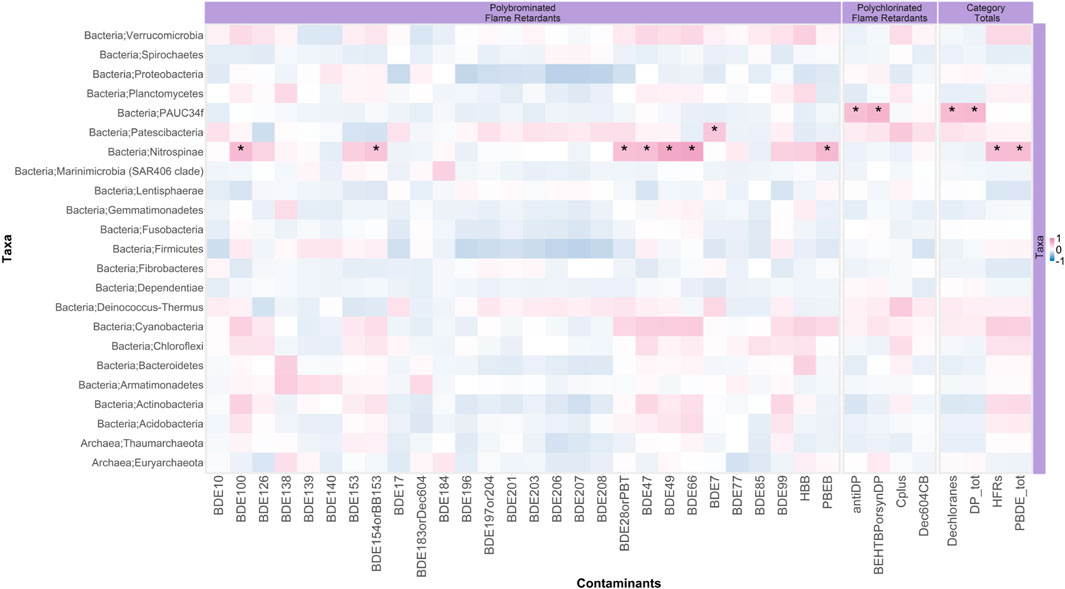
FIGURE 6. Correlation between the SLE beluga skin microbiome phylum abundances and their blubber halogenated flame retardant (HFR) concentrations. Each block represents the Pearson correlation between the indicated taxa (Y-axis) and an HFR compound (X-axis). The gradient of the color represents the strength of the Pearson correlation coefficient (red is positive, and blue is negative). Asterisks represent significant correlations. This plot reveals that PAUC34f and Nitrospinae were positively correlated with the blubber concentrations of anti-DP and syn-DP, and ∑33PBDE (PBDE tot), respectively.
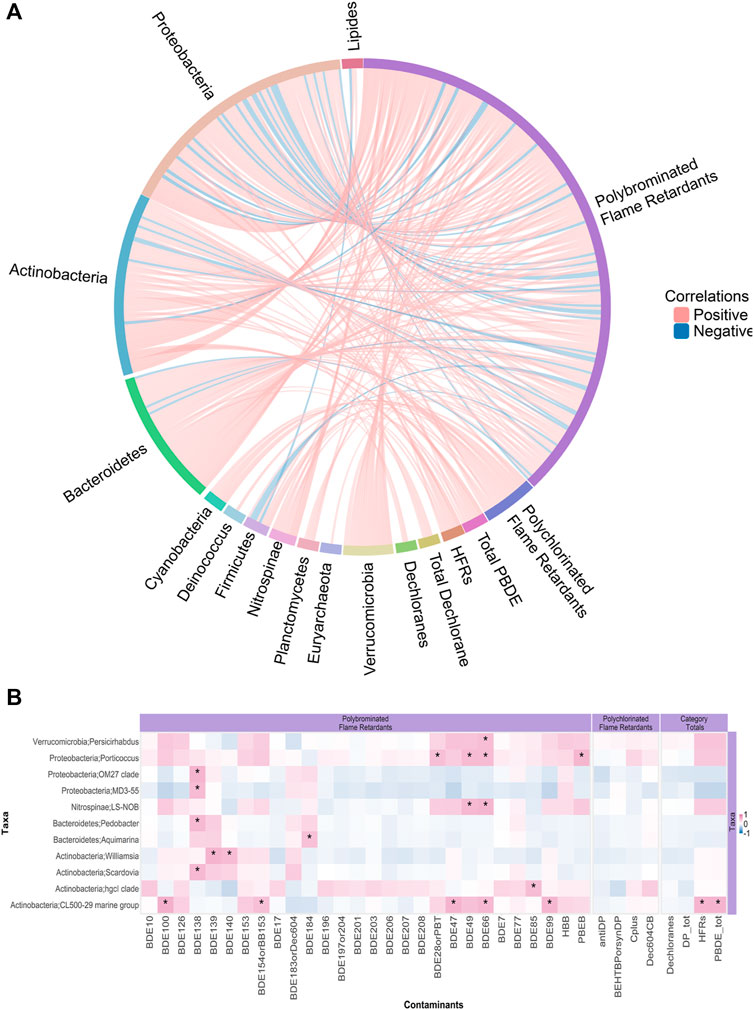
FIGURE 7. Correlations between SLE beluga skin microbiome genera abundances and halogenated flame retardant (HFR) levels in the beluga blubber. (A) GlobeCorr Plot (globecorr.ca) highlighting relationships between all genera and HFRs without including significance. Each line represents a pairwise Pearson correlation between a taxon and a contaminant. This plot shows only those relationships with an absolute coefficient of >0.3. A positive correlation appears red, while a negative correlation appears blue. Intensity of the color indicates the strength of the Pearson correlation coefficient. Actinobacteria and Bacteroidetes contained the most genera that correlated with HFR levels in the beluga male blubber with a coefficient of >0.3. For an interactive visualization, allowing further perusal of the data, please visit: https://globecorr.ca/belugaGlobe. (B) Heatmap highlighting genera with significant correlations with HFRs. Each block represents a Pearson correlation between genera (Y-axis) and a HFR compound (X-axis). The gradient of the color represents the strength of the Pearson correlation coefficient (red = positive, blue = negative). Asterisks represent the significance of the correlation (each additional star represents p < 0.05, 0.01, and 0.001 respectively). Similar to the GlobeCorr plot, four Actinobacteria and two Bacteroidetes genera significantly correlated with selected HFRs in the beluga male blubber.
4 Discussion
To our knowledge, the present study provided the first report of the microbial community from the SLE beluga population’s skin and surrounding seawater, and the first report of the beluga’s functional microbiome. Moreover, this is the first indication that skin swabs from biopsies can be effectively used to query the beluga skin microbiome, opening the possibility of obtaining skin swabs from beluga for contaminant assessments. The present study investigated the skin microbiome of the endangered SLE beluga population and the surrounding SLE water microbiome, as well as identified several correlations between skin bacterial abundance in the SLE beluga (males) and HFR concentrations in their blubber.
4.1 The SLE beluga skin microbiome is unique and has a distinctive composition
Analysis of the SLE beluga skin microbiome revealed the large dominance of Proteobacteria with Psychrobacter spp. and Pseudoalteromonas spp. being the most abundant species present in their core microbiome. Four bacterial genera (Psychrobacter, Pseudoalteromonas, Escherichia, and Staphylococcus) were consistently detected and made up >50% of the skin microbiome, regardless of sampling area or the year it was sampled. The dominant phylum, Proteobacteria, found in the SLE beluga skin microbiome was similar in proportions with the skin microbiome of Alaskan beluga and microbiomes from the closely related bottle-nose dolphins’ (Tursiops truncatus) exhaled blow breath and humpback whale’s (Megaptera novaeangliae) skin (Apprill et al., 2014; Bik et al., 2016; Cise et al., 2020). Furthermore, more than 95% of bacteria taxa identified in the beluga skin microbiome, including Psychrobacter and Pseudoalteromonas, were detected in the killer whale of the eastern North Pacific Ocean exhaled breath samples (Apprill et al., 2014; Raverty et al., 2017). These genera were also identified in the colder marine Antarctic oceanic habitats (HolmstrÃm and Kjelleberg, 1999; Kim et al., 2012). The presence of these bacteria likely reflects the dynamics of their marine environment. Our shotgun data further provided an insight into the non-bacterial skin microbiome community. As such, the protozoan group, Sporadotrichida, was identified as the most abundant eukaryotic organism present on SLE beluga skin. This protozoan was first identified in brackish waters from a stream in South Korea. The SLE, similarly, is defined by brackish waters that may promote the growth of Sporadotrichida (Kwon and Shin, 2011). By taking samples of water from the same sites where belugas were sampled in the SLE, we were able to identify that the SLE beluga skin microbiome is unique and distinct compared to the surrounding SLE water microbiome. As expected, the beluga skin microbiome was considerably less diverse compared to water of the SLE. Specifically, while four genera made up 50% of the skin microbiome, 36 genera combined made up 50% of the SLE water microbiome.
There were no species that represented >1% of relative abundance shared between the SLE beluga skin microbiome and the surrounding SLE water, suggesting that variability in skin microbiome due to contribution from SLE water microbes is somewhat limited. This observation could be explained by the fact that belugas having a mucosal layer on their skin protects their microbiome from intrusion by opportunistic microbes from the marine environment. Moreover, Bray–Curtis dissimilarity indices showed that there was little divergence between individual SLE belugas. Specifically, the SLE beluga skin microbiome was similar enough that NMDS was not able to fit the dissimilarities between animals when stratified based on the belugas’ sex, sampling location, nor sampling year. This suggests that these factors likely had limited influence on the SLE beluga skin microbiome composition. Some of this marked similarity in the beluga skin microbiome, regardless of the animal ID or sampling location, may be due to the isolation of this beluga population in the relatively small area encompassed by the SLE. This observation requires further examination and confirmation in other beluga populations that are not geographically restricted, for example, those from the Arctic regions. In further support of this similarity, previous studies in humans and other mammals have shown that cohabiting family members typically share similar microbiota (Song et al., 2013). Belugas, like humans, are social animals that intermix, which may, in addition to characteristics of their skin, cause their skin microbiome to be highly similar.
4.2 Correlations between contaminants and the SLE beluga skin microbiome
Among all HFRs quantified in the SLE male beluga blubber (HFRs were not analyzed in females), the abundance of phylum, Nitrospinae, correlated with ∑33PBDE concentrations and candidate phylum PAUC34f with anti- and syn-DP. Nitrospinae is a novel, largely uncharacterized, uncultured oceanic bacterial phylum first identified in metagenome assembled genomes from anoxic waters but have since been reported as the major nitrite-oxidizing bacteria in surface waters in oceans (Lücker et al., 2013; Sun et al., 2019). These bacteria utilize the metabolism of nitrite to nitrate as their main energy source. However, it is unknown whether there is a direct relationship between the enzyme activity of Nitrospinae and PAUC34f, and their ability to proliferate on the skin of belugas with elevated concentrations of HFRs in their blubber. A study by Yan et al. (2017) demonstrated that elevated levels of nitrate were associated with the biodegradation of a PBDE congener (BDE-99) in municipal wastewater, and the wastewater microbial community was influenced by changes in nitrate concentrations. SLE beluga blubber BDE-99 concentrations were observed to positively correlate (r = 0.38, p < 0.01) with Nitrospinae abundance. It could be suggested that the biodegradation of PBDEs in blubber of the belugas contributed, at least in part, to the elevated skin nitrate concentrations that promoted growth of Nitrospinae on their skin. PAUC34f, originally discovered in marine sponges, has also been linked to complex carbon degradation in low-dissolved oxygen water of the Gulf of Mexico, in which nitrogen was the major source of energy (Thrash et al., 2017). Furthermore, in other sponges that reside in or near oxygenated ocean surfaces (H. heliophila), PAUC34f was part of the core symbionts and nitrogen cycling taxa that contribute to nitrogen transformation in sponge holobiont (Hentschel et al., 2002; Cuvelier et al., 2014; Weigel and Erwin, 2017). This link between nitrogen cycling ability of PAUC34f and the biodegradation of PBDEs leading to an increase in nitrate may play a role in the proliferation of PAUC34f on the beluga skin with elevated levels of HFRs. At the genera level, among the most interesting findings, the Actinobacteria, CL500-29 Marine clade, showed a strong positive correlation with ∑33PBDE and five individual PBDEs (BDE-47, -66, -99, -100, and -154). Again, to our knowledge, no direct links between this bacterial genus and PBDE exposures in wildlife or in experimental studies could be found in the literature. It had been observed that certain Actinobacteria have a strong ability to utilize phenolic compounds in bioreactors and wastewater treatment plants (Gómez-Acata et al., 2016; Baskaran et al., 2017). These phenolic compounds may include products derived from the degradation or enzyme-mediated biotransformation of PBDEs. As such, several hydroxylated PBDEs (OH-PBDE) congeners have been detected to be accumulating in beluga (McKinney et al., 2006). The bulk of environmental contaminants, including HFRs, in the SLE originate from wastewater treatment plant effluents along the Great Lakes and St. Lawrence River basin. Marine-associated Actinobacteria, such as CL500-29 Marine clade, may proliferate as part of the beluga skin microbiome due to this exposure. Through shotgun metagenomic sequencing, we were able to identify one COG functional pathway, carbohydrate transport, and metabolism, which negatively correlated with ∑33PBDE concentrations in the SLE beluga blubber. Another pathway, posttranslational modification, protein turnover, and chaperone, was positively correlated with the blubber concentration of the HFR Dec-604 CB. Neither of these changes in pathway abundance could be linked to a particular species and could represent host-associated functional pathways. In vitro laboratory studies have demonstrated that PBDEs can exhibit neurotoxicant potential by interfering with mitochondrial activity through the induction of oxidative stress as PBDEs are degraded in a cell (Meerts et al., 2001; Dingemans et al., 2011). The reduced carbohydrate metabolism and increased protein turnover/chaperone could be an effect of neutralizing oxidative stress-related toxicity due to increased contaminant exposure. However, this would remain speculative and would require further study.
5 Conclusions and limitations
In this study, we were able to perform the first investigation of the SLE beluga skin microbiome and its surrounding water microbiome, including both taxonomic and functional microbial profiles, showing that the skin microbiome is unique to the belugas versus the surrounding water microbiome. We found that the skin microbiome does not appreciably change as a function of beluga sex or location; however, we identified taxa that were associated with blubber concentrations of a comprehensive suite of ubiquitous HFRs. Based on these associations, we hypothesize that the biodegradation of HFRs within the beluga resulted in an increased formation of metabolites (i.e., OH-PBDEs and nitrate) on the skin, which lead to the proliferation of Nitrospinae and PAUC32f. Limitations exist for both our analysis and microbiome studies. First, taxonomic characterization relies on the previously studied taxa with known classified genomic sequences. Environmental microbiome characterization is often a challenge due to the large number of unknown environmental bacterial species present within it. This is especially true for belugas that reside in the SLE, an estuary where salt and freshwater mixes and represents a niche that may promote the growth of bacteria unknown to other environments. This was demonstrated in our results in which lower-level taxa classifications (i.e., species level) were difficult. In fact, many ambiguous or uncultured bacterial species were also identified in the SLE beluga skin surface as well as the brackish SLE water. Certainly, as this environment’s microbiome is studied better, such analyses should improve. Second, shotgun metagenomic analysis was performed using a metagenome that had >80% of sequenced reads belonging to the host beluga. The present study used multiple steps of filtering to better characterize the bacterial function profile. However, this may have removed some reads of bacterial origin to fully elucidate these functional profiles. This work indicates that deeper shotgun metagenomic sequencing using either short or, preferably, long read methods would be useful to further characterize the SLE beluga skin microbiome. Next, while we performed metabolite characterization of the blubber and identified correlative taxa, we did not investigate occurrence of these metabolites in the surrounding seawater. It is possible that the taxa that correlated with HFR metabolites reflect degradation that occurred in water rather than within the blubber and skin tissues. Future metabolite profiling of seawater would further elucidate these linkages. Last, both the blubber HFR concentrations and skin 16S metagenomics data were only available for 42 male SLE belugas. While being an impressive sample size for studies of an endangered wild cetacean populations, due to the large number of features found in taxonomic profiles (13,347 ASVs, 655 genera), only linear correlation-based analysis could be performed to identify relationships. Further in vitro experiments are also warranted to investigate the identified bacterial taxa of interest that correlated with changes in the blubber PBDE concentrations in the SLE belugas and understand its potential mechanisms. Nevertheless, this work provides an enticing first insight into the composition of the core microbiome of the SLE beluga skin and the surrounding water in the SLE—work that forms the basis for future experiments to study temporal effects of contaminant exposure on the beluga skin microbiome as well as changes in the SLE water microbiome due to anthropogenic activities. The conservation and management of this endangered population rely upon the health monitoring of individuals, and such methods generally necessitate invasive sampling. Similar to the drone-captured whale blow microbiome (Apprill et al., 2014), the routine utilization of the skin microbiome, in addition to other biological characteristics, may provide an alternative and innovative surveillance approach worldwide. These approaches can provide a framework for contaminant exposure monitoring that could be done at higher frequencies, and is less invasive than blubber sampling, to understand the influence of contaminants on endangered cetacean populations.
Data availability statement
The datasets presented in this study can be found in online repositories. The names of the repository/repositories and accession number(s) can be found below: https://www.ncbi.nlm.nih.gov/, PRJNA842171, https://osf.io/thknm, thknm.
Ethics statement
The reviewed and approved animal study conducted by the present study was performed under permits granted by Parks Canada (SAGMP-2013-14734) and Fisheries and Oceans Canada (IML-2015-13 and IML-2016-021). Sampling methods were approved by the animal care committee of Fisheries and Oceans Canada, which is accredited by the Canadian Council on Animal Care (Ottawa, ON, Canada).
Author contributions
CH, FB, and BJ devised the original experimentation and acquired funding; BJ, CH, JV, and FB contributed to the conceptualization; BJ, RM, RL, MA, and JR performed the sampling, extraction, sequencing, investigation, and formal analysis; BJ, EG, RM, CH, JV, and FB interpreted the results; and BJ, CH, JV, and FB wrote the original draft; all authors reviewed and edited the manuscript.
Funding
This study was funded in part by the National Contaminants Advisory Group of Fisheries and Oceans Canada. Supplemental funding for biopsy sampling was provided by the Species at Risk program of Fisheries and Oceans Canada. BJ holds Canadian Institutes of Health Research (CIHR) doctoral scholarship and Simon Fraser University Omics and Data Sciences fellowship. FB holds an SFU Distinguished Professorship. Additionally, this work was partially supported by Genome Canada and NSERC grants to FB.
Acknowledgments
The authors would like to thank M. Moisan and R. Michaud (Group for Research and Education on Marine Mammals, Tadoussac, QC, Canada) as well as A. Simond and A. Bernier-Graveline (Université du Québec à Montréal) for sample collection and handling, and L. Wang (Université du Québec à Montréal) for HFR analyses. The authors would further like to thank the Simon Fraser University Research Computing Group and Compute Canada for computing resource support.
Conflict of interest
The authors declare that the research was conducted in the absence of any commercial or financial relationships that could be construed as a potential conflict of interest.
Publisher’s note
All claims expressed in this article are solely those of the authors and do not necessarily represent those of their affiliated organizations, or those of the publisher, the editors, and the reviewers. Any product that may be evaluated in this article, or claim that may be made by its manufacturer, is not guaranteed or endorsed by the publisher.
Supplementary material
The Supplementary Material for this article can be found online at: https://www.frontiersin.org/articles/10.3389/fenvs.2022.954060/full#supplementary-material
References
Apprill, A., Robbins, J., Eren, A. M., Pack, A. A., Reveillaud, J., Mattila, D., et al. (2014). Humpback whale populations share a core skin bacterial community: Towards a health index for marine mammals? PLoS ONE 9, e90785. doi:10.1371/journal.pone.0090785
Baskaran, R., Subramanian, T., Zuo, W., Qian, J., Wu, G., and Kumar, A. (2017). Major source of marine actinobacteria and its biomedical application. Microb. Appl. 2, 55–82. doi:10.1007/978-3-319-52669-0_3
Bik, E. M., Costello, E. K., Switzer, A. D., Callahan, B. J., Holmes, S. P., Wells, R. S., et al. (2016). Marine mammals harbor unique microbiotas shaped by and yet distinct from the sea. Nat. Commun. 7, 10516. doi:10.1038/ncomms10516
Bolyen, E., Rideout, J. R., Dillon, M. R., Bokulich, N. A., Abnet, C. C., Al-Ghalith, G. A., et al. (2019). Reproducible, interactive, scalable and extensible microbiome data science using QIIME 2. Nat. Biotechnol. 37, 852–857. doi:10.1038/s41587-019-0209-9
Buchfink, B., Xie, C., and Huson, D. H. (2014). Fast and sensitive protein alignment using DIAMOND. Nat. Methods 12, 59–60. doi:10.1038/nmeth.3176
Bushnell, B., Rood, J., and Singer, E. (2017). BBMerge – accurate paired shotgun read merging via overlap. PLOS ONE 12, e0185056. doi:10.1371/journal.pone.0185056
Callahan, B. J., McMurdie, P. J., Rosen, M. J., Han, A. W., Johnson, A. J. A., and Holmes, S. P. (2016). DADA2: High-resolution sample inference from illumina amplicon data. Nat. Methods 13, 581–583. doi:10.1038/nmeth.3869
Chen, B., Yu, T., Xie, S., Du, K., Liang, X., Lan, Y., et al. (2018). Comparative shotgun metagenomic data of the silkworm bombyx mori gut microbiome. Sci. Data 5, 180285. doi:10.1038/sdata.2018.285
Chiarello, M., Villéger, S., Bouvier, C., Auguet, J. C., and Bouvier, T. (2017). Captive bottlenose dolphins and killer whales harbor a species-specific skin microbiota that varies among individuals. Sci. Rep. 7, 15269. doi:10.1038/s41598-017-15220-z
Cise, A. M. V., Wade, P. R., Goertz, C. E. C., Burek-Huntington, K., Parsons, K. M., Clauss, T., et al. (2020). Skin microbiome of beluga whales: Spatial, temporal, and health-related dynamics. Anim. microbiome 2, 39. doi:10.1186/s42523-020-00057-1
Cogen, A. L., Yamasaki, K., Sanchez, K. M., Dorschner, R. A., Lai, Y., MacLeod, D. T., et al. (2010). Selective antimicrobial action is provided by phenol-soluble modulins derived from staphylococcus epidermidis, a normal resident of the skin. J. Investigative Dermatology 130, 192–200. doi:10.1038/jid.2009.243
Cuvelier, M. L., Blake, E., Mulheron, R., McCarthy, P. J., Blackwelder, P., Thurber, R. L. V., et al. (2014). Two distinct microbial communities revealed in the sponge cinachyrella. Front. Microbiol. 5, 581. doi:10.3389/fmicb.2014.00581
Czajkowski, M. D., Vance, D. P., Frese, S. A., and Casaburi, G. (2018). GenCoF: A graphical user interface to rapidly remove human genome contaminants from metagenomic datasets. Bioinformatics 35, 2318–2319. doi:10.1093/bioinformatics/bty963
Dewhirst, F. E., Chen, T., Izard, J., Paster, B. J., Tanner, A. C. R., Yu, W. H., et al. (2010). The human oral microbiome. J. Bacteriol. 192, 5002–5017. doi:10.1128/jb.00542-10
Dingemans, M. M., van den Berg, M., and Westerink, R. H. (2011). Neurotoxicity of brominated flame retardants: (in)direct effects of parent and hydroxylated polybrominated diphenyl ethers on the (developing) nervous system. Environ. Health Perspect. 119, 900–907. doi:10.1289/ehp.1003035
Fisheries, C. O. (2017). St. Lawrence Estuary Beluga. A Science based review of recovery actions for three at-risk whale populations. Ottawa, Canada: Government of Canada.
Galperin, M. Y., Wolf, Y. I., Makarova, K. S., Alvarez, R. V., Landsman, D., and Koonin, E. V. (2020). COG database update: Focus on microbial diversity, model organisms, and widespread pathogens. Nucleic Acids Res. 49, D274–D281. doi:10.1093/nar/gkaa1018
Gómez-Acata, S., Esquivel-Ríos, I., Pérez-Sandoval, M. V., Navarro-Noya, Y., Rojas-Valdez, A., Thalasso, F., et al. (2016). Bacterial community structure within an activated sludge reactor added with phenolic compounds. Appl. Microbiol. Biotechnol. 101, 3405–3414. doi:10.1007/s00253-016-8000-z
Grice, E. A., Kong, H. H., Conlan, S., Deming, C. B., Davis, J., Young, A. C., et al. (2009). Topographical and temporal diversity of the human skin microbiome. Science 324, 1190–1192. doi:10.1126/science.1171700
Hammill, M. O. (2007). Lack of recovery in St. Lawrence estuary beluga. Canadian Science Advisory Secretariat. Ottawa, Canada: Government of Canada.
Hentschel, U., Hopke, J., Horn, M., Friedrich, A. B., Wagner, M., Hacker, J., et al. (2002). Molecular evidence for a uniform microbial community in sponges from different oceans. Appl. Environ. Microbiol. 68, 4431–4440. doi:10.1128/aem.68.9.4431-4440.2002
HolmstrÃm, C., and Kjelleberg, S. (1999). Marine pseudoalteromonas species are associated with higher organisms and produce biologically active extracellular agents. FEMS Microbiol. Ecol. 30, 285–293. doi:10.1111/j.1574-6941.1999.tb00656.x
Huber, J. A., Mark Welch, D. B., Morrison, H. G., Huse, S. M., Neal, P. R., Butterfield, D. A., et al. (2007). Microbial population structures in the deep marine biosphere. Science 318, 97–100. doi:10.1126/science.1146689
Huson, D. H., Beier, S., Flade, I., Górska, A., El-Hadidi, M., Mitra, S., et al. (2016). MEGAN community edition - interactive exploration and analysis of large-scale microbiome sequencing data. PLoS Comput. Biol. 12, e1004957. doi:10.1371/journal.pcbi.1004957
Jones, S., Taylor, G., Chan, S., Warren, R., Hammond, S., Bilobram, S., et al. (2017). The genome of the beluga whale (delphinapterus leucas). Genes 8, 378. doi:10.3390/genes8120378
Kim, S. J., Shin, S. C., Hong, S. G., Lee, Y. M., Choi, I. G., and Park, H. (2012). Genome sequence of a novel member of the genus psychrobacter isolated from Antarctic soil. J. Bacteriol. 194, 2403. doi:10.1128/jb.00234-12
Kwon, C. B., and Shin, M. K. (2011). First records of two aquatic oxytrichid ciliates (ciliophora: Sporadotrichida: Oxytrichidae) from Korea. Animal Syst. Evol. Divers. 27, 59–65. doi:10.5635/kjsz.2011.27.1.059
Langmead, B., and Salzberg, S. L. (2012). Fast gapped-read alignment with bowtie 2. Nat. Methods 9, 357–359. doi:10.1038/nmeth.1923
Lebeuf, M., Measures, L., Noël, M., Raach, M., and Trottier, S. (2014). A twenty-one year temporal trend of persistent organic pollutants in st. lawrence estuary beluga, Canada. Sci. total Environ. 485, 377–386. doi:10.1016/j.scitotenv.2014.03.097
Lema, K. A., Bourne, D. G., and Willis, B. L. (2014). Onset and establishment of diazotrophs and other bacterial associates in the early life history stages of the coralAcropora millepora. Mol. Ecol. 23, 4682–4695. doi:10.1111/mec.12899
Lesage, V., Lair, S., Turgeon, S., and Béland, P. (2020). Diet of st. lawrence estuary beluga (Delphinapterus leucas) in a changing ecosystem. Can. Field. Nat. 134, 21–35. doi:10.22621/cfn.v134i1.2421
Love, M. I., Huber, W., and Anders, S. (2014). Moderated estimation of fold change and dispersion for RNA-seq data with DESeq2. Genome Biol. 15, 550. doi:10.1186/s13059-014-0550-8
Lücker, S., Nowka, B., Rattei, T., Spieck, E., and Daims, H. (2013). The genome of nitrospina gracilis illuminates the metabolism and evolution of the major marine nitrite oxidizer. Front. Microbiol. 4, 27. doi:10.3389/fmicb.2013.00027
McKinney, M. A., Guise, S. D., Martineau, D., Béland, P., Lebeuf, M., and Letcher, R. J. (2006). Organohalogen contaminants and metabolites in beluga whale (DELPHINAPTERUS leucas) liver from two CANADIAN populations. Environ. Toxicol. Chem. 25, 1246. doi:10.1897/05-284r.1
McMurdie, P. J., and Holmes, S. (2014). Shiny-phyloseq: Web application for interactive microbiome analysis with provenance tracking. Bioinformatics 31, 282–283. doi:10.1093/bioinformatics/btu616
Meerts, I. A., Letcher, R. J., Hoving, S., Marsh, G., Bergman, A., Lemmen, J. G., et al. (2001). In vitro estrogenicity of polybrominated diphenyl ethers, hydroxylated PDBEs, and polybrominated bisphenol a compounds. Environ. Health Perspect. 109, 399–407. doi:10.1289/ehp.01109399
Mosnier, A., Doniol-Valcroze, T., Gosselin, J. F., Lesage, V., Measures, L., and Hammill, M. (2015). Insights into processes of population decline using an integrated population model: The case of the st. lawrence estuary beluga (delphinapterus leucas). Ecol. Model. 314, 15–31. doi:10.1016/j.ecolmodel.2015.07.006
Nakatsuji, T., Chiang, H. I., Jiang, S. B., Nagarajan, H., Zengler, K., and Gallo, R. L. (2013). The microbiome extends to subepidermal compartments of normal skin. Nat. Commun. 4, 1431. doi:10.1038/ncomms2441
Overbeek, R., Olson, R., Pusch, G. D., Olsen, G. J., Davis, J. J., Disz, T., et al. (2013). The SEED and the rapid annotation of microbial genomes using subsystems technology (RAST). Nucleic Acids Res. 42, D206–D214. doi:10.1093/nar/gkt1226
Quast, C., Pruesse, E., Yilmaz, P., Gerken, J., Schweer, T., Yarza, P., et al. (2012). The SILVA ribosomal RNA gene database project: Improved data processing and web-based tools. Nucleic Acids Res. 41, D590–D596. –D596. doi:10.1093/nar/gks1219
Raverty, S. A., Rhodes, L. D., Zabek, E., Eshghi, A., Cameron, C. E., Hanson, M. B., et al. (2017). Respiratory microbiome of endangered southern resident killer whales and microbiota of surrounding sea surface microlayer in the eastern north Pacific. Sci. Rep. 7, 394. doi:10.1038/s41598-017-00457-5
Ross, A. A., Hoffmann, A. R., and Neufeld, J. D. (2019). The skin microbiome of vertebrates. Microbiome 7, 79. doi:10.1186/s40168-019-0694-6
Rossum, T. V., Pylatuk, M. M., Osachoff, H. L., Griffiths, E. J., Lo, R., Quach, M., et al. (2016). Microbiome analysis across a natural copper gradient at a proposed northern canadian mine site. Front. Environ. Sci. 3. doi:10.3389/fenvs.2015.00084
Salerno, J. L., Bowen, B. W., and Rappé, M. S. (2016). Biogeography of planktonic and coral-associated microorganisms across the Hawaiian archipelago. FEMS Microbiol. Ecol. 92, fiw109. doi:10.1093/femsec/fiw109
Schwarz, R. S., Moran, N. A., and Evans, J. D. (2016). Early gut colonizers shape parasite susceptibility and microbiota composition in honey bee workers. Proc. Natl. Acad. Sci. U. S. A. 113, 9345–9350. doi:10.1073/pnas.1606631113
Simond, A. E., Houde, M., Lesage, V., Michaud, R., and Verreault, J. (2020). Metabolomic profiles of the endangered st. lawrence estuary beluga population and associations with organohalogen contaminants. Sci. Total Environ. 717, 137204. doi:10.1016/j.scitotenv.2020.137204
Simond, A. E., Houde, M., Lesage, V., and Verreault, J. (2017). Temporal trends of PBDEs and emerging flame retardants in belugas from the st. lawrence estuary (Canada) and comparisons with minke whales and canadian arctic belugas. Environ. Res. 156, 494–504. doi:10.1016/j.envres.2017.03.058
Song, S. J., Lauber, C., Costello, E. K., Lozupone, C. A., Humphrey, G., Berg-Lyons, D., et al. (2013). Cohabiting family members share microbiota with one another and with their dogs. eLife 2, e00458. doi:10.7554/elife.00458
Ssekagiri, A., and Sloan, W. T.Umer Zeeshan Ijaz (2017). microbiomeseq: An r package for analysis of microbial communities in an environmental context. Unpublished. doi:10.13140/RG.2.2.17108.71047
Sun, X., Kop, L. F. M., Lau, M. C. Y., Frank, J., Jayakumar, A., Lücker, S., et al. (2019). Uncultured nitrospina-like species are major nitrite oxidizing bacteria in oxygen minimum zones. ISME J. 13, 2391–2402. doi:10.1038/s41396-019-0443-7
Thambirajah, A. A., Wade, M. G., Verreault, J., Buisine, N., Alves, V. A., Langlois, V. S., et al. (2022). Disruption by stealth-interference of endocrine disrupting chemicals on hormonal crosstalk with thyroid axis function in humans and other animals. Environ. Res. 203, 111906. doi:10.1016/j.envres.2021.111906
Thrash, J. C., Seitz, K. W., Baker, B. J., Temperton, B., Gillies, L. E., Rabalais, N. N., et al. (2017). Metabolic roles of uncultivated bacterioplankton lineages in the northern gulf of Mexico “dead zone”. mBio 8, e01017. doi:10.1128/mbio.01017-17
Wang, Z., Mascarenhas, N., Eckmann, L., Miyamoto, Y., Sun, X., Kawakami, T., et al. (2017). Skin microbiome promotes mast cell maturation by triggering stem cell factor production in keratinocytes. J. Allergy Clin. Immunol. 139, 1205–1216. e6. doi:10.1016/j.jaci.2016.09.019
Weigel, B. L., and Erwin, P. M. (2017). Effects of reciprocal transplantation on the microbiome and putative nitrogen cycling functions of the intertidal sponge, hymeniacidon heliophila. Sci. Rep. 7, 43247. doi:10.1038/srep43247
Yan, Y., Ma, M., Zhang, W., Ma, W., Li, M., and Yan, L. (2017). Effect of elevated nitrate on biodegradation of pentabromodiphenyl ether (BDE-99) and change in microbial communities during groundwater recharge with tertiary-treated municipal wastewater. Int. Biodeterior. Biodegrad. 124, 128–137. doi:10.1016/j.ibiod.2017.03.022
Yap, M., Feehily, C., Walsh, C. J., Fenelon, M., Murphy, E. F., McAuliffe, F. M., et al. (2020). Evaluation of methods for the reduction of contaminating host reads when performing shotgun metagenomic sequencing of the milk microbiome. Sci. Rep. 10, 21665. doi:10.1038/s41598-020-78773-6
Zaneveld, J. R., Burkepile, D. E., Shantz, A. A., Pritchard, C. E., McMinds, R., Payet, J. P., et al. (2016). Overfishing and nutrient pollution interact with temperature to disrupt coral reefs down to microbial scales. Nat. Commun. 7, 11833. doi:10.1038/ncomms11833
Zhang, Q., Wang, J., Zhu, J., Liu, J., Zhang, J., and Zhao, M. (2016). Assessment of the endocrine-disrupting effects of short-chain chlorinated paraffins in in vitro models. Environ. Int. 94, 43–50. doi:10.1016/j.envint.2016.05.007
Keywords: bacteria, belugas, Delphinapterus leucas, halogenated flame retardants, metagenomics, functional microbiome, St. Lawrence Estuary, skins swab
Citation: Jia B, Garlock E, Allison MJ, Michaud R, Lo R, Round JM, Helbing CC, Verreault J and Brinkman FSL (2022) Investigating the relationship between the skin microbiome and flame retardant exposure of the endangered St. Lawrence Estuary beluga. Front. Environ. Sci. 10:954060. doi: 10.3389/fenvs.2022.954060
Received: 26 May 2022; Accepted: 30 September 2022;
Published: 18 October 2022.
Edited by:
Kun-Yi Andrew Lin, National Chung Hsing University, TaiwanReviewed by:
Liesbeth Weijs, Griffith University, AustraliaChloe Victoria Robinson, Ocean Wise, Canada
Copyright © 2022 Jia, Garlock, Allison, Michaud, Lo, Round, Helbing, Verreault and Brinkman. This is an open-access article distributed under the terms of the Creative Commons Attribution License (CC BY). The use, distribution or reproduction in other forums is permitted, provided the original author(s) and the copyright owner(s) are credited and that the original publication in this journal is cited, in accordance with accepted academic practice. No use, distribution or reproduction is permitted which does not comply with these terms.
*Correspondence: Fiona S. L. Brinkman, YnJpbmttYW5Ac2Z1LmNh