Introduction
Decarbonization is on the agenda of most countries of the world (Lau, 2022; Luo et al., 2022; Mathur et al., 2022; Obrist et al., 2022; Zhao et al., 2022). The global GHG emission reduction agenda was launched in November 2021 at the UN (2022) Climate Change Conference in Glasgow (COP26). At this conference, environmental degradation and the importance of decarbonization were recognized by 120 world leaders (Adebayo, 2022a; Adebayo et al., 2022; Adebayo, 2022b).
The problem is that, despite the significant progress made in decarbonizing the economy by individual countries, their results are contradictory (Doğan et al., 2022; Li et al., 2022; Nasir et al., 2022). On the one hand, the benefits of reducing the carbon footprint of the economy for the environment and quality of life are obvious (the tip of the iceberg) (Murshed et al., 2021; Hamid et al., 2022a; Alam et al., 2022; Hamid et al., 2022b; Murshed et al., 2022; Popkova, 2022).
On the other hand, deep contradictions of decarbonization remain undisclosed, but gradually are coming to the surface (Ratner et al., 2022), since the existing technological structure does not allow for completely replacing natural energy resources with “green” analogues (Sisodia et al., 2020; Teichmann et al., 2020). A vivid manifestation of these contradictions is that the countries that are leaders in the field of decarbonization of the economy—the United States, Japan, the European Union and the United Kingdom—are experiencing energy crises (Gallo et al., 2020; Ratner et al., 2021; Štreimikienė et al., 2022).
This contradiction is becoming more acute in the era of artificial intelligence. In the works of Kovacova and Lăzăroiu (2021), Popkova et al. (2021), Sergi and Popkova (2022), the AI era is defined as a modern period of world history, the distinctive feature of which is the gap between people and automation tools. For the first time in the history of industrial revolutions, automation does not improve the human use of means of production but completely isolates and excludes him from economic processes.
As noted in the works of Chen et al. (2021), Faheem and Butt (2022), Matsunaga et al. (2022), Oks et al. (2022), the systematic nature of cyber-physical systems achieved in the AI era reflects the revolutionary communications of inanimate objects (digital technology) with each other, with physical objects (for example, buildings, equipment) and even with wildlife (for example, natural resources in various industries or plants and animals in agriculture). But this integration does not apply to a man who is outside of Industry 4.0 systems.
This article uses a broad interpretation of the AI era, which is not limited to AI alone and covers the whole set of disruptive (subversive) technological innovations of Industry 4.0, in particular, robots, Big Data and data analytics, as well as the Internet of Things (IoT). All technologies of the AI era are characterized by high energy intensity, which was the criterion for their generalization in the works of Hao et al. (2022), Miśkiewicz et al. (2021), Reshetnikova and Pugacheva (2022), Wang et al. (2022).
Full automation, taking place in the AI era, brings the energy intensity of the economy to a critical level even with the use of energy-saving technologies (Berner et al., 2022; Chen et al., 2022; Muhammad et al., 2022). And “clean” energy today is not able to cover the growing demands of the economy in energy, which does not allow reduction and may even lead to a forced growth in carbon emissions. In this regard, in the works of Doroshuk (2021), Maggiore et al. (2021), Parmar et al. (2022), Qi et al. (2022), the AI era is considered an unfavourable time and an unfriendly technological landscape for decarbonization.
Thus, the relevance of the study is explained by the fact that the era of artificial intelligence associated with total automation creates increased risks of climate change and, at the same time, opens up high-tech opportunities for energy saving. The main reason for conducting this study is the need to form a systematic understanding of the special context of decarbonization, which consists in the era of artificial intelligence, taking into account its advantages and disadvantages, as well as the formation of a balanced (providing both deficit-free and environmentally friendly energy) approach to the development of an ecological AI-economy in support of decarbonisation.
The research question of this article is as follows: “How to achieve a reduction in carbon emissions and decarbonization of the economy and at the same time avoid an energy crisis (energy shortage)?” To answer the research question posed in this article, the hypothesis H0 is put forward that artificial intelligence allows achieving a reduction in carbon emissions and decarbonization of the economy and at the same time avoid an energy crisis (energy shortage).
The purpose of the article is to reveal the features of the decarbonization of the economy in the era of artificial intelligence, taking into account the challenges of developing “clean” energy and the prospects for reducing carbon emissions using robots, Big Data, IoT and AI. After the above introduction, this article reveals the challenges of the AI era for “clean” energy and decarbonization of the economy. Using the regression analysis method, the impact of robot density on CO₂ emissions and the share of renewable energy in the leading countries in industrial robotization in 2021 is modelled, according to the International Federation of Robotics (2022b). Then describes the prospects and recommendations for decarbonization of the AI economy. Further, the results obtained are discussed in comparison with the existing literature, and a conclusion is made at the end of the article.
The contribution of the study to the literature consists of a systematic analysis and rethinking of the consequences of the spread and use of disruptive innovations of Industry 4.0 for “clean” energy in the era of artificial intelligence. The author’s systematization and classification of these innovations made it possible to identify and clearly distinguish between the innovations of the era of artificial intelligence that contribute to the reduction of carbon emissions (AI, IoT and Big Data), as well as the innovations of this era that slow down decarbonization (robots). In this way, the article opens up opportunities for more environmentally responsible dissemination and application of the disruptive innovations of Industry 4.0, as well as their flexible combination in support of decarbonization.
Theoretical mechanism and hypothesis built-up
The fundamental research framework in this article is the Theory of decarbonization of the economy. Comparison and contrast of different studies within the limits of this theory has revealed the controversial aspects of decarbonization as a scientific and economic category. Camarasa et al. (2022), Lau (2022), Luo et al. (2022) in their writings point out that decarbonization is essential since it is one of the most important vectors of development of ecological economics.
As opposed to them, Fulzele et al. (2022), Pilloni et al. (2022), Vatsa and Miljkovic (2022) in their writings point out the increasingly growing need for energy resources and, in particular, fossil fuel energy, to accelerate the recovery of the global economy from the COVID-19 crisis. Gargallo et al. (2022), Ram and Webler (2022), Tan et al. (2021), Zhang et al. (2022) in their publications maintain that the world community is not ready to completely abandon fossil fuel energy and that clean energy involves certain constraints that are associated with its incomplete coverage of economic entities (constraints of infrastructure), high cost (financial constraints), as well as low and unpredictable performance (natural and climatic constraints of renewable energy sources).
The literature review has identified its main gaps that need to be worked upon. The first gap is the contradiction of decarbonization as a catalyst for sustainable development while also an obstacle to economic growth. The second gap is that while the existing literature by Favi et al. (2022), Mastrocinque et al. (2022), Matsunaga et al. (2022), Morelli et al. (2022), Vitiello et al. (2022), Wachnik et al. (2022) points out the special context of energy development that has formed at present in the context of the AI economy, this context has been left out of consideration in decarbonization planning.
This allows defining the study’s problem statement as the uncertainty of the prospects for achieving decarbonization of the economy in the AI era. The significance of this research is attributable the fact that decarbonization is declared as an official priority of the countries across the world, most of which have already approved their national decarbonization strategies by now. However, given acute energy deficiency and unprecedentedly high global energy prices, these strategies have been jeopardized. In this respect, the potential of various factors to support decarbonization strategies should be identified. Among these factors, the AI economy is of paramount importance, since it allows taking advantage of the opportunities created by the new technological mode (Industry 4.0).
The research question of the article consists in methods of achieving carbon reduction and decarbonization of the economy while also avoiding an energy crisis (energy shortage). Based on the writings by Ahmed et al. (2022), Dimou and Vakalis (2022), Ragulina et al. (2022), Xuan and Ocone (2022) which point out the benefits of AI-based energy automation, this article puts forward the H0 hypothesis that carbon reduction and decarbonization of the economy while avoiding an energy crisis (energy shortage) can be achieved through the use of Artificial Intelligence.
The contribution of the article to the systematic research literature in this area of investigation consists in precise definition of the features—challenges and prospects—of the decarbonization of the economy in the new context of modernity, that has formed in the era of Artificial Intelligence. The theoretical mechanism for testing the hypothesis advanced in this article is involved with a qualitative-quantitative analysis analysis of the cause-and-effect relationships of the development of clean energy and carbon reduction using robots, Big Data, IoT and AI based on the systematic approach.
Challenges of the AI era for “clean” energy and decarbonization of the economy
To test the proposed hypothesis H0, the Systematic Approach methodology is used, based on which a quantitative and qualitative analysis of the impact of disruptive technological innovations of the era of artificial intelligence on reducing carbon emissions and decarbonizing the economy is carried out. The quantitative analysis includes econometric modelling of the impact of the spread of robots as the advanced technology of the AI era on climate change. The qualitative analysis is related to expert analytics on the contribution of the Internet of Things (IoT) and Big Data as disruptive technological innovations in the era of artificial intelligence to the decarbonization of the economy.
The quantitative analysis has used such variables as Robot Density according to the International Federation of Robotics (2022a) as a factor in the AI economy, as well as CO₂ emissions and the share of renewable energy according to the UNDP (2022) as results in the field of decarbonization (development of clean energy and carbon reduction).
The choice of indicators is explained by the fact that industrial robots are the technology of the AI era, which is the most widespread and used in practice today. Also, thanks to the global efforts of the UN, serious progress has been made today in the development of “clean” energy and the decarbonization of the economy (Figure 1).
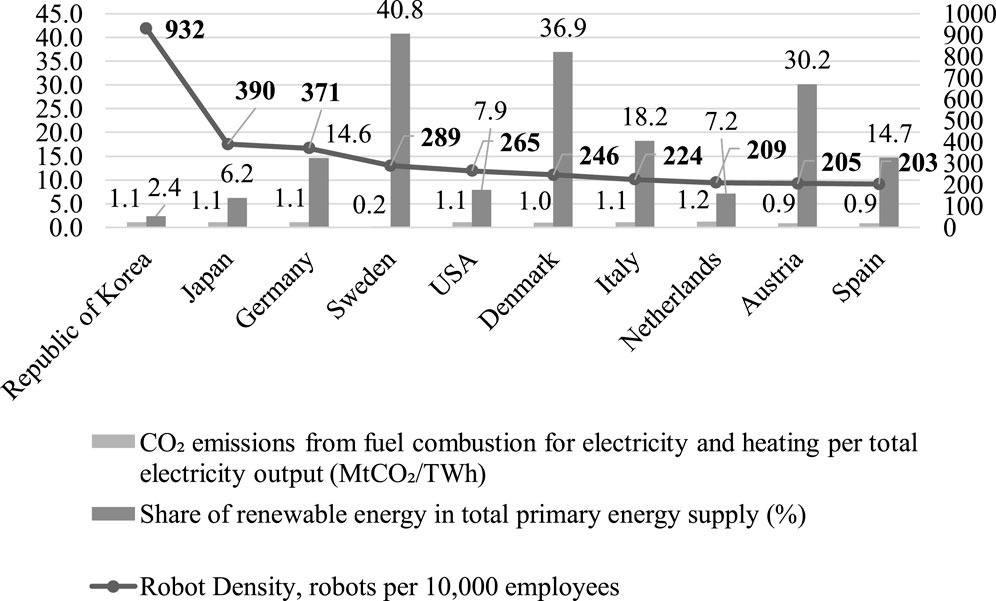
FIGURE 1. Robot density (rb), CO2 emissions (carb) and the share of renewable energy sources (renew) in the leading countries in the robotics industry in 2021. Source: Compiled by the authors based on materials from the International Federation of Robotics (2022a), UNDP (2022).
A simple linear regression allowed us to obtain models (carb = 0.93 + 0.0001*rb and renew = 27.01–0.03rb), according to which, with an increase in robot density by 1 robot per 10,000 employees, CO₂ emissions from fuel combustion for electricity and heating per total electricity output increase by 0.0001 MtCO₂/TWh (correlation 11.43%), and the share of renewable energy in total primary energy supply is reduced by 0.03% (correlation 44.42%).
According to the International Federation of Robotics (2022b), the global average in 2021 robot density is 435 robots per 10,000 employees, and by 2024 it will increase to 518 robots per 10,000 employees, that is, by 19.08%. Based on the regression models obtained above, it can be expected that this will increase CO₂ emissions from fuel combustion for electricity and heating per total electricity output by 1% and reduce the share of renewable energy in the total primary energy supply by 9.68%.
Consequently, robotization represents a serious challenge in the AI era for “clean” energy and decarbonization of the economy. At the same time, other disruptive technological innovations are also actively developing. Thus, according to Mordor Intelligence (2022), IoT active connections will increase from 2.65 million units in 2022 to 3.09 million units by 2025—and this is only in retail. According to IDC Corporate USA (2022), global spending on Big Data in 2021-2025 will increase by 12.8% annually. This opens up favourable opportunities for “smart” analytics of “clean” energy with the help of artificial intelligence.
Thus, the obtained results revealed the challenges of the era of artificial intelligence for “clean” energy and the decarbonization of the economy. The first one is related to the fact that as robotization increases, CO₂ emissions increase and the share of renewable energy decreases. The second challenge is that as the Internet of Things (IoT) advances and Big Data spreads, new opportunities for decarbonization are opening up that need to be exploited to mitigate and offset the negative impact of robots on energy and the environment.
A systematic look at the identified challenges showed that the era of artificial intelligence opens up new opportunities for decarbonization to a greater extent than increases its risks. This is explained by the fact that robotization is a factor of energy and environmental risks developing at a slower pace (6.36% per year) than two factors for the development of “clean” energy and reducing greenhouse gas emissions: IoT (5.53% per year) and Big Data (12.8% per year).
Prospects and recommendations for the decarbonization of the AI economy
Recommendations for standard business regulation policies include stimulating entrepreneurial innovation and supporting high-tech economic growth. In the field of innovation policy, it is recommended to stimulate the balanced development of disruptive technological innovations - robots, the Internet of Things (IoT), Big Data and artificial intelligence (AI). In the field of energy policy, it is proposed to tighten the requirements for energy efficiency of disruptive technological innovations in support of energy saving. In the field of environmental policy, it is recommended to expand the use of high technology opportunities in the implementation of economic decarbonization strategies.
Big Data and IoT form the information base for “smart” AI-enabled analytics. The promising role that artificial intelligence can play in the transition to “clean” and alternative energy, as well as in improving the energy efficiency of the economy, is associated with the identification of the potential of disruptive innovations of the AI economy to support decarbonization. That is, artificial intelligence is designed to become an intermediary, a mediator between the Fourth Industrial Revolution and the decarbonization of the economy.
Many advanced solutions in the field of decarbonization of low-tech economic processes have already been found and are successfully practised, but in the field of high technology, there is a shortage of environmentally friendly solutions. Artificial intelligence is able (unlike humans) to take into account the subtleties of Industry 4.0 technologies and therefore offer non-standard and revolutionary solutions for their use in order to decarbonize the economy. The following applied recommendations are proposed for managing the decarbonization of the AI-based economy to perform the role described above in practice:
˗ Automated statistical monitoring of the contribution of high technologies of Industry 4.0 (for example, robots) to the decarbonization of the economy. The “smart” AI-enabled analytics allows identifying individual contributions to the decarbonization of each technology and technical device. This will demonstrate the strengths and weaknesses of decarbonization in the context of enterprises and markets;
˗ Highly detailed decarbonization planning in Industry 4.0. Artificial intelligence will be able to select unique decarbonization solutions for each enterprise and market, as well as offer highly detailed plans for their transition to clean energy and reduction of carbon emissions;
˗ Search for solutions to complex optimization problems to correspond to the specified decarburization criteria using high technologies of Industry 4.0 (for example, robots). Artificial intelligence, thanks to its high-performance and record-fast analytics, can find optimal solutions for decarbonization, for example, when saving the required number of jobs or a given amount of required profit in case of a limited budget.
The advantages of reducing carbon emissions and decarbonizing the AI-based economy include, firstly, support for decarbonization by high-tech sectors of the economy, which, without the use of artificial intelligence, make a small or zero contribution to decarbonization and may even hinder it. Secondly, they involve system solutions for decarbonization, covering the entire set of processes for the production of “clean” energy, its distribution and optimal consumption, if necessary in combination with fossil energy.
Discussion
The contribution of the article to the literature consists in rethinking the prospects of decarbonization, taking into account the peculiarities of the AI era. Following the research results, it has been revealed that new challenges arise for “clean” energy and decarbonization of the economy in the era of Artificial Intelligence. On the one hand, there is an increasing energy intensity of housekeeping and a growing need for fossil fuels for the maintenance of robots. This gives rise to a challenge associated with the need to increase the energy intensity of robotic production works.
On the other hand, other disruptive technological innovations of the era of Artificial Intelligence, including Artificial Intelligence as such, as well as the Internet of Things (IoT) and Big Data, open up new opportunities for decarbonization, supporting the development of “clean” energy and carbon reduction.
The prospects for decarbonization of the AI economy are involved with the use of a systematic approach to the national economic policy. This systematic approach should comprise and ensure the integrated management of the development of disruptive technological innovations, energy and environmental economics. The advantage of the proposed systematic approach will consist in the support of the balanced and sustainable development, as well as consistent progress in the implementation of SDG7 and SDG13.
The contribution of the paper to the improvement of scientific knowledge in the field of decarbonization and combating climate change is as follows. Unlike Hao et al. (2022), Miśkiewicz et al. (2021), Reshetnikova and Pugacheva (2022), Wang et al. (2022), it has been proved that disruptive technological innovations are not homogeneous, but are characterized by serious differences in terms of energy efficiency. A new classification of these innovations according to the criterion of consequences for decarbonization has been proposed; technologies with high energy intensity (for example, robots) and technologies that support decarbonization (for example, IoT, Big Data and AI) have been identified. The advantage of the author’s classification is that it allows flexible use of technologies of the AI era, achieving the best practical results in decarbonization.
Unlike Doroshuk (2021), Maggiore et al. (2021), Parmar et al. (2022), Qi et al. (2022), it has been proven that in the era of artificial intelligence there are no obstacles to decarbonization, but on the contrary, new opportunities have been created for this. Using only disruptive innovations of industry 4.0 (for example, only robots) can hinder the reduction or even increase the number of carbon emissions, as well as slow down the transition to “clean” energy. But with the complex use of technologies in the AI era, the disadvantages of some technologies are compensated by the advantages of others. And artificial intelligence can play a system-forming role in this process, providing intellectual support for maximizing the cumulative contribution of disruptive innovations of Industry 4.0 to the decarbonization of the AI economy.
The contribution of the findings to the growth of scientific knowledge lies in the fact that they allow us to take a fresh look at the process and prospects for the decarbonization of the economy in the era of artificial intelligence, taking into account both the advantages and disadvantages of the disruptive innovations of Industry 4.0. This allows robots, Big Data and AI to spread more consciously, with clear control and high-precision prediction of their impact on carbon emissions and clean energy. Due to this, the article more deeply revealed the cause-and-effect relationships of the development of ecological economics and management in the AI era, showing the place of the disruptive innovations of Industry 4.0 in this process.
Conclusion
The results of the study revealed the challenges of the AI era for “clean” energy and decarbonization of the economy, associated, on the one hand, with an increase in carbon emissions and a reduction in the share of “clean” energy as robotics develops, and, on the other hand, with support for the decarbonization of the AI economy based on Big Data, IoT and AI. The author’s conclusions that the disruptive innovations of Industry 4.0 can both accelerate and slow down the processes of reducing carbon emissions and the transition to “clean” energy allow a more objective and systematic look at the AI era as a decarbonization context and take into account the peculiarities of this context in the state and corporate managing the development of ecological economics and management.
The theoretical significance of the study consists in revealing the consequences of the spread of disruptive innovations of Industry 4.0 for environmental AI economics and management through the author’s classification based on the criteria of contribution to decarbonization. The article develops and complements the scientific strategies of decarbonization, presented in the writing by Hamid et al. (2022). The practical significance of the author’s conclusions and proposed recommendations on the use of artificial intelligence for the decarbonization of the economy makes it possible to accelerate the transition to “clean” energy and increase the scale of carbon emissions reduction through the involvement of high-tech sectors of the AI economy, which were previously considered inaccessible for decarbonization.
The social significance of the research results is that they support the systematic practical implementation of SDG 7 and SDG 13 in environmental AI economics and management. Suggestions for policy makers include flexible use of the disruptive innovations of Industry 4.0: increased use of IoT, Big Data and AI as catalysts for carbon reduction and clean energy transition, and more thoughtful, careful and responsible distribution of robots as potential inhibitors of these processes.
A limitation of the results obtained is that this study focuses on decarbonization, while there are many other promising areas for the development of environmental economics and management. Among these areas is the reduction of production and consumption waste, recycling, reducing the consumption of natural resources and increasing the resource efficiency of the economy, etc. Each of these areas needs an independent in-depth study of the prospects for its implementation in the AI era. In this regard, Future directions of research are related to the disclosure of the consequences (both challenges and prospects) of the spread of disruptive innovations of Industry 4.0 for other areas of development of ecological economics and management, as well as to identify the prospects for more responsible and sustainable use of these innovations.
Author contributions
All authors listed have made a substantial, direct, and intellectual contribution to the work and approved it for publication.
Acknowledgments
The work was supported by the Ministry of Agriculture of Russia and financed by the federal government as a part of the programme of research activities of Russian State Agrarian University - Moscow Timiryazev Agricultural Academy in year 2022.
Conflict of interest
The author declares that the research was conducted in the absence of any commercial or financial relationships that could be construed as a potential conflict of interest.
Publisher’s note
All claims expressed in this article are solely those of the authors and do not necessarily represent those of their affiliated organizations, or those of the publisher, the editors and the reviewers. Any product that may be evaluated in this article, or claim that may be made by its manufacturer, is not guaranteed or endorsed by the publisher.
References
Adebayo, T. S., Oladipupo, S. D., Kirikkaleli, D., and Adeshola, I. (2022). Asymmetric nexus between technological innovation and environmental degradation in Sweden: An aggregated and disaggregated analysis. Environ. Sci. Pollut. Res. 29, 36547–36564. doi:10.1007/s11356-021-17982-6
Adebayo, T. S., Oladipupo, S. D., Rjoub, H., Kirikkaleli, D., and Adeshola, I. (2022b). Asymmetric effect of structural change and renewable energy consumption on carbon emissions: Designing an SDG framework for Turkey. Environ. Dev. Sustain., 1–29. doi:10.1007/s10668-021-02065-w
Adebayo, T. S. (2022a). Renewable energy consumption and environmental sustainability in Canada: Does political stability make a difference? Environ. Sci. Pollut. Res. Int. doi:10.1007/s11356-022-20008-4
Ahmed, Q. W., Garg, S., Rai, A., Jhanjhi, N. Z., Masud, M., Baz, M., et al. (2022). AI-based resource allocation techniques in wireless sensor internet of things networks in energy efficiency with data optimization. Electron. Switz. 11 (13), 2071. doi:10.3390/electronics11132071
Alam, M., Alam, M., Murshed, M., Mahmood, H., and Alam, R. (2022). Pathways to securing environmentally sustainable economic growth through efficient use of energy: A bootstrapped ARDL analysis. Environ. Sci. Pollut. Res. 29, 50025–50039. doi:10.1007/s11356-022-19410-9
Berner, A., Bruns, S., Moneta, A., and Stern, D. I. (2022). Do energy efficiency improvements reduce energy use? Empirical evidence on the economy-wide rebound effect in europe and the United States. Energy Econ. 110, 105939. doi:10.1016/j.eneco.2022.105939
Camarasa, C., Mata, É., Navarro, J. P. J., Bezerra, P., Angelkorte, G. B., Wanemark, J., et al. (2022). A global comparison of building decarbonization scenarios by 2050 towards 1.5–2 °C targets. Nat. Commun. 13 (1), 3077. doi:10.1038/s41467-022-29890-5
Chen, H., Shi, Y., Xu, M., and Zhao, X. (2022). Investment in renewable energy resources, sustainable financial inclusion and energy efficiency: A case of us economy. Resour. Policy 77, 102680. doi:10.1016/j.resourpol.2022.102680
Chen, M., Sinha, A., Hu, K., and Shah, M. I. (2021). Impact of technological innovation on energy efficiency in Industry 4.0 era: Moderation of shadow economy in sustainable development. Technol. Forecast. Soc. Change 164, 120521. doi:10.1016/j.techfore.2020.120521
Dimou, A., and Vakalis, S. (2022). Technoeconomic analysis of green energy transitions in isolated grids: The case of Ai Stratis – green Island. Renew. Energy 195, 66–75. doi:10.1016/j.renene.2022.06.039
Doğan, B., Ferraz, D., Gupta, M., Duc Huynh, T. L., and Shahzadi, I. (2022). Exploring the effects of import diversification on energy efficiency: Evidence from the OECD economies. Renew. Energy 189, 639–650. doi:10.1016/j.renene.2022.03.018
Doroshuk, H. (2021). Prospects and efficiency measurement of artificial intelligence in the management of enterprises in the energy sector in the era of Industry 4.0. Polityka Energetyczna –. Energy Policy J. 24 (4), 61–76. doi:10.33223/epj/144083
Faheem, M., and Butt, R. A. (2022). Big Datasets of optical-wireless cyber-physical systems for optimizing manufacturing services in the internet of things-enabled Industry 4.0. Data Brief 42, 108026. doi:10.1016/j.dib.2022.108026
Favi, C., Marconi, M., Mandolini, M., and Germani, M. (2022). Sustainable life cycle and energy management of discrete manufacturing plants in the industry 4.0 framework. Appl. Energy 312, 118671. doi:10.1016/j.apenergy.2022.118671
Fulzele, R., Fulzele, V., and Dharwal, M. (2022). Mapping the impact of COVID-19 crisis on the progress of sustainable Development Goals (SDGs) - a focus on global environment and energy efficiencies. Mater. Today Proc. 60, 873–879. doi:10.1016/j.matpr.2021.09.517
Gallo, E., Wu, Z., and Sergi, B. S. (2020). China's power in its strategic energy partnership with the Eurasian economic union. Communist Post-Communist Stud. 53 (4), 200–219. doi:10.1525/j.postcomstud.2020.53.4.200
Gargallo, P., Lample, L., Miguel, J., and Salvador, M. (2022). Dynamic comparison of portfolio risk: Clean vs dirty energy. Finance Res. Lett. 47, 102957. doi:10.1016/j.frl.2022.102957
Hamid, I., Alam, M. S., Kanwal, A., Jena, P. K., Murshed, M., and Alam, R. (2022a). Decarbonization pathways: The roles of foreign direct investments, governance, democracy, economic growth, and renewable energy transition. Environ. Sci. Pollut. Res. 29, 49816–49831. doi:10.1007/s11356-022-18935-3
Hamid, I., Alam, M. S., Murshed, M., Jena, P. K., and Sha, N. (2022b). The roles of foreign direct investments, economic growth, and capital investments in decarbonizing the economy of Oman. Environ. Sci. Pollut. Res. 29, 22122–22138. doi:10.1007/s11356-021-17246-3
Hao, A., Tan, J., Ren, Z., and Zhang, Z. (2022). A spatial empirical examination of the relationship between agglomeration and green total-factor productivity in the context of the carbon emission peak. Front. Environ. Sci. 10, 829160. doi:10.3389/fenvs.2022.829160
IDC Corporate USA (2022). Global spending on Big data and analytics solutions will reach $215.7 billion in 2021, according to a new IDC spending guide. Available at: https://www.idc.com/getdoc.jsp?containerId=prUS48165721.
International Federation of Robotic (2022a). World robot report: Robot density nearly doubled globally. Available at: https://ifr.org/ifr-press-releases/news/robot-density-nearly-doubled-globally#:∼:text=This%20is%20according%20to%20the,Japan%2C%20Germany%2C%20and%20Sweden (Accessed 05 15, 2022).
International Federation of Robotics (2022b). IFR presents World Robotics 2021 reports. Available at: https://ifr.org/ifr-press-releases/news/robot-sales-rise-again.
Kovacova, M., and Lăzăroiu, G. (2021). Sustainable organizational performance, cyber-physical production networks, and deep learning-assisted smart process planning in Industry 4.0-based manufacturing systems. Econ. Manag. Financial Mark. 16 (3), 41–54. doi:10.22381/emfm16320212
Lau, H. C. (2022). Decarbonization roadmaps for ASEAN and their implications. Energy Rep. 8, 6000–6022. doi:10.1016/j.egyr.2022.04.047
Li, G., Luo, T., and Song, Y. (2022). Climate change mitigation efficiency of electric vehicle charging infrastructure in China: From the perspective of energy transition and circular economy. Resour. Conservation Recycl. 179, 106048. doi:10.1016/j.resconrec.2021.106048
Luo, S., Hu, W., Liu, W., Bai, C., Huang, Q., Chen, Z., et al. (2022). Study on the decarbonization in China's power sector under the background of carbon neutrality by 2060. Renew. Sustain. Energy Rev. 166, 112618. doi:10.1016/j.rser.2022.112618
Maggiore, S., Realini, A., Zagano, C., Gobbi, E., and Borgarello, M. (2021). Energy efficiency in industry 4.0: Assessing the potential of industry 4.0 to achieve 2030 decarbonisation targets. Int. J. Eq. 6 (4), 371–381. doi:10.2495/EQ-V6-N4-371-381
Mastrocinque, E., Ramírez, F. J., Honrubia-Escribano, A., and Pham, D. T. (2022). Industry 4.0 enabling sustainable supply chain development in the renewable energy sector: A multi-criteria intelligent approach. Technol. Forecast. Soc. Change 182, 121813. doi:10.1016/j.techfore.2022.121813
Mathur, S., Gosnell, G., Sovacool, B. K., Griffiths, S., Bazilian, M., Kim, J., et al. (2022). Industrial decarbonization via natural gas: A critical and systematic review of developments, socio-technical systems and policy options. Energy Res. Soc. Sci. 90, 102638. doi:10.1016/j.erss.2022.102638
Matsunaga, F., Zytkowski, V., Valle, P., and Deschamps, F. (2022). Optimization of energy efficiency in smart manufacturing through the application of cyber-physical systems and industry 4.0 technologies. J. Energy Resour. Technol. 144 (10), 102104. doi:10.1115/1.4053868
Miśkiewicz, R., Rzepka, A., Borowiecki, R., and Olesińki, Z. (2021). Energy efficiency in the Industry 4.0 era: Attributes of teal organisations. Energies 14 (20), 6776. doi:10.3390/en14206776
Mordor Intelligence (2022). Internet of things (iot) market - growth, trends, COVID-19 IMPACT, and forecasts. Available at: https://www.mordorintelligence.com/industry-reports/internet-of-things-moving-towards-a-smarter-tomorrow-market-industry.
Morelli, G., Magazzino, C., Gurrieri, A. R., Pozzi, C., and Mele, M. (2022). Designing smart energy systems in an industry 4.0 paradigm towards sustainable environment. Sustain. Switz. 14 (6), 3315. doi:10.3390/su14063315
Muhammad, S., Pan, Y., Agha, M. H., Umar, M., and Chen, S. (2022). Industrial structure, energy intensity and environmental efficiency across developed and developing economies: The intermediary role of primary, secondary and tertiary industry. Energy 247, 123576. doi:10.1016/j.energy.2022.123576
Murshed, M., Mahmood, H., Ahmad, P., Rehman, A., and Alam, M. S. (2022). Pathways to Argentina’s 2050 carbon-neutrality agenda: The roles of renewable energy transition and trade globalization. Environ. Sci. Pollut. Res. 29, 29949–29966. doi:10.1007/s11356-021-17903-7
Murshed, M., Rahman, M. A., Alam, M. S., Ahmad, P., and Dagar, V. (2021). The nexus between environmental regulations, economic growth, and environmental sustainability: Linking environmental patents to ecological footprint reduction in south asia. Environ. Sci. Pollut. Res. 28, 49967–49988. doi:10.1007/s11356-021-13381-z
Nasir, M. H., Wen, J., Nassani, A. A., Igharo, A. E., Musibau, H. O., Waqas, M., et al. (2022). Energy security and energy poverty in emerging economies: A step towards sustainable energy efficiency. Front. Energy Res. 10, 834614. doi:10.3389/fenrg.2022.834614
Obrist, M. D., Kannan, R., Schmidt, T. J., and Kober, T. (2022). Long-term energy efficiency and decarbonization trajectories for the Swiss pulp and paper industry. Sustain. Energy Technol. Assessments 52, 101937. doi:10.1016/j.seta.2021.101937
Oks, S. J., Jalowski, M., Lechner, M., Merklein, M., Vogel-Heuser, B., Möslein, K. M., et al. (2022). Cyber-physical systems in the context of industry 4.0: A review, categorization and outlook. Inf. Syst. Front. doi:10.1007/s10796-022-10252-x
Parmar, B., Vishwakarma, A., Padbhushan, R., Kumar, R., Kumari, R., Kaviraj, M., et al. (2022). Hedge and alder-based agroforestry systems: Potential interventions to carbon sequestration and better crop productivity in Indian sub-himalayas. Front. Environ. Sci. 10, 858948. doi:10.3389/fenvs.2022.858948
Pilloni, M., Kádár, J., and Hamed, T. A. (2022). The impact of COVID‐19 on energy start‐up companies: The use of global financial crisis (GFC) as a lesson for future recovery. Energies 15 (10), 3530. doi:10.3390/en15103530
Popkova, E. (2022). Advanced issues in the green economy and sustainable development in emerging market economies (elements in the economics of emerging markets). Cambridge: Cambridge University Press. doi:10.1017/9781009093408
Popkova, E., Bogoviz, A. V., and Sergi, B. S. (2021). Towards digital society management and ‘capitalism 4.0’ in contemporary Russia. Humanit. Soc. Sci. Commun. 8 (1), 77. doi:10.1057/s41599-021-00743-8
Qi, H., Sun, L., Long, F., Gao, X., and Hu, L. (2022). Does forest resource protection under the carbon neutrality target inhibit economic growth? Evidence of poverty-stricken county from China. Front. Environ. Sci. 10, 858632. doi:10.3389/fenvs.2022.858632
Ragulina, Y. V., Dubova, Y. I., Litvinova, T. N., and Balashova, N. N. (2022). The environmental AI economy and its contribution to decarbonization and waste reduction. Front. Environ. Sci. 10, 914003. doi:10.3389/fenvs.2022.914003
Ram, B., and Webler, T. (2022). Social amplification of risks and the clean energy transformation: Elaborating on the four attributes of information. Risk Anal. 42, 1423–1439. doi:10.1111/risa.13902
Ratner, S., Berezin, A., Gomonov, K., Serletis, A., and Sergi, B. S. (2022). What is stopping energy efficiency in Russia? Exploring the confluence of knowledge, negligence, and other social barriers in the krasnodar region. Energy Res. Soc. Sci. 85, 102412. doi:10.1016/j.erss.2021.102412
Ratner, S., Berezin, A., and Sergi, B. S. (2021). Energy efficiency improvements under conditions of low energy prices: The evidence from Russian regions. Energy Sources, Part B Econ. Plan. Policy, 1–20. doi:10.1080/15567249.2021.1966134
Reshetnikova, M. S., and Pugacheva, I. A. (2022). The global industrial robotics market: Development trends and volume forecast. Current problems of the world economy and international trade. Emerald Publishing Limited, 42.
Sergi, B. S., and Popkova, E. G. (2022). Towards a ‘wide’ role for venture capital in OECD countries' Industry 4.0. Heliyon 8 (1), e08700. doi:10.1016/j.heliyon.2021.e08700
Sisodia, G. S., Awad, E., Alkhoja, H., and Sergi, B. S. (2020). Strategic business risk evaluation for sustainable energy investment and stakeholder engagement: A proposal for energy policy development in the Middle East through khalifa funding and land subsidies. Bus. Strategy Environ. 29 (6), 2789–2802. doi:10.1002/bse.2543
Štreimikienė, D., Samusevych, Y., Bilan, Y., Vysochyna, A., and Sergi, B. S. (2022). Multiplexing efficiency of environmental taxes in ensuring environmental, energy, and economic security. Environ. Sci. Pollut. Res. 29 (5), 7917–7935. doi:10.1007/s11356-021-16239-6
Tan, X., Geng, Y., Vivian, A., and Wang, X. (2021). Measuring risk spillovers between oil and clean energy stocks: Evidence from a systematic framework. Resour. Policy 74, 102406. doi:10.1016/j.resourpol.2021.102406
Teichmann, F., Falker, M.-C., and Sergi, B. S. (2020). Gaming environmental governance? Bribery, abuse of subsidies, and corruption in European union programs. Energy Res. Soc. Sci. 66, 101481. doi:10.1016/j.erss.2020.101481
UN (2022). UN climate change conference in Glasgow (COP-26). Available at: https://www.un.org/ru/climatechange/cop26 (Accessed 06 29, 2022).
UNDP (2022). Sustainable development report 2021: Explore data. Available at: https://dashboards.sdgindex.org/(Accessed 05 15, 2022).
Vatsa, P., and Miljkovic, D. (2022). Energy and crop price cycles before and after the global financial crisis: A new approach. J. Agric. Econ. 73 (1), 220–233. doi:10.1111/1477-9552.12454
Vitiello, S., Andreadou, N., Ardelean, M., and Fulli, G. (2022). Smart metering roll-out in europe: Where do we stand? Cost benefit analyses in the clean energy package and research trends in the green deal. Energies 15 (7), 2340. doi:10.3390/en15072340
Wachnik, B., Kłodawski, M., and Kardas-Cinal, E. (2022). Reduction of the information gap problem in industry 4.0 projects as a way to reduce energy consumption by the industrial sector. Energies 15 (3), 1108. doi:10.3390/en15031108
Wang, J., Hui, W., Liu, L., Du, Y., and Li, J. (2022). Estimation and influencing factor Analysis of carbon emissions from the entire production cycle for household consumption: Evidence from the urban communities in beijing, China. Front. Environ. Sci. 10, 843920. doi:10.3389/fenvs.2022.843920
Xuan, J., and Ocone, R. (2022). The equality, diversity and inclusion in energy and AI: Call for actions. Energy AI 8, 100152. doi:10.1016/j.egyai.2022.100152
Zhang, L., Chen, Z., Yang, C., and Xu, Z. (2022). Global supply risk assessment of the metals used in clean energy technologies. J. Clean. Prod. 331, 129602. doi:10.1016/j.jclepro.2021.129602
Keywords: reduction of carbon emissions, decarbonization, “clean” energy, artificial intelligence (AI), disruptive technological innovations, industry 4.0, environmental AI economics and management
Citation: Vorozheykina TM (2022) Challenges and prospects of decarbonization of the economy in the age of AI. Front. Environ. Sci. 10:952821. doi: 10.3389/fenvs.2022.952821
Received: 25 May 2022; Accepted: 08 August 2022;
Published: 14 September 2022.
Edited by:
Bruno Sergi, Harvard University, United StatesReviewed by:
Ishfaq Hamid, Shri Mata Vaishno Devi University, IndiaMd Shabbir Alam, University of Bahrain, Bahrain
Copyright © 2022 Vorozheykina. This is an open-access article distributed under the terms of the Creative Commons Attribution License (CC BY). The use, distribution or reproduction in other forums is permitted, provided the original author(s) and the copyright owner(s) are credited and that the original publication in this journal is cited, in accordance with accepted academic practice. No use, distribution or reproduction is permitted which does not comply with these terms.
*Correspondence: Tatiana M. Vorozheykina, dm9yb3poZXlraW5hQGdtYWlsLmNvbQ==