- 1Department of Computer Science, Huanggang Normal University, Huanggang, China
- 2Balochistan University of Information Technology, Engineering, and Management Sciences (BUITEMS), Quetta, Pakistan
- 3School of Geography, Nanjing Normal University, Nanjing, China
- 4Key Laboratory of Virtual Geographic Environment, Ministry of Education, Nanjing Normal University, Nanjing, China
- 5Department of Computer Science, Muhammad Nawaz Shareef University of Agriculture Multan, Multan, Pakistan
- 6School of Information and Communication Engineering, Hainan Univeristy, Haikou, China
- 7School of Economics, Shandong University of Technology, Zibo, China
In response to the COVID-19 outbreak, severe steps have been taken to control its rapid spread by countries globally. A nationwide lockdown was executed at the end of January 2020 in China, which resulted in a significant change and an improvement in air quality patterns. In this study, the objectives were to assess the spatiotemporal impact of the COVID-19 lockdown on air quality in Nanjing, China. The present study researched the six air pollutant parameters, namely, PM10, PM2.5, SO2, NO2, CO, and O3. The data were divided into six periods, P1–P3: pre-lockdown, during lockdown, and after lockdown periods, P4–P6: 2017–19 (same dates of lockdown). The results reveal that during the COVID-19 control period, a significant drop and an improvement in air quality were observed. According to our findings, the PM10, PM2.5, SO2, NO2, and CO concentrations were reduced by -33.03%, -35.41%, -21.26%, -39.79%, and -20.65%, respectively, while the concentration of O3 significantly increased by an average of 104.85% in Nanjing. From the previous 3 years to lockdown variations, PM10 (-40.60%), PM2.5 (-40.02%), SO2 (-54.19%), NO2 (-33.60%), and CO (23.16%) were also reduced, while O3 increased (10.83%). Moreover, compared with those in the COVID-19 period, the levels of PM10, SO2, NO2, CO, and O3 increased by 2.84%, 28.55%, 4.68%, 16.44%, and 37.36%, respectively, while PM2.5 reduced by up to -14.34% after the lockdown in Nanjing. The outcomes of our study provide a roadmap for the scientific community and local administration to make policies to control air pollution.
1 Introduction
A novel disease later named coronavirus disease 2019 (COVID-19) was first identified at the end of 2019, in Wuhan, China. Due to this contagious disease, a health emergency had been declared by the World Health Organization all around the world (World Health Organization 2020). More than 210 countries had been affected by COVID-19 within 6 months (Wang C et al., 2020). According to a report, about 18,354,342 were affected by the COVID-19 pandemic on 5 August 2020 (World Health Organization 2020). To control the rapid spread of this virus, forced restrictions and control measures were implemented by many countries and nations (McMahon 2020). In this scenario, the air quality significantly improved during the lockdown time. For instance, during the COVID-19 pandemic lockdown period, a sharp drop in air pollution was noticed in several areas and regions all around the world shown by satellite images (Holcombe and O’Key 2020). The lockdown is vital to the public health response to COVID-19, and it has also brought unexpected benefits for the environment (Abdullah et al., 2020; Bhatti et al., 2022a).
Many studies reported that due to these restrictions, a remarkable reduction was found in the concentrations of almost all air pollutants around the globe (Bao and Zhang 2020; Li at al., 2020; Zambrano-Monserrate and Ruano 2020). For instance, according to Berman and Ebisu's study (2020), due to the COVID-19 pandemic lockdown, a significant decline was reported in the concentration of PM2.5 (11%) and NO2 (26%) in the United States. Shakoor et al. (2020) reported that during the COVID-19 pandemic lockdown, the concentrations of CO, NO2, and PM2.5 were decreased by 19%, 37%, and 1.1%, respectively, in the United States. Their results demonstrate that the concentrations of CO, NO2, SO2, PM2.5, and PM10 were decreased by 27%, 39%, 18%, 18%, and 38%, respectively, in China. Another study illustrates that in India and China, the lockdown measures brought positive impacts and decrease in PM2.5 and NO2 concentrations, to be reduced by an average of 65%, 66%, and 45%, 37%, respectively (Agarwal et al., 2020). Meanwhile, it is evident by the study of Sharma et al. (2020) that during the COVID-19 pandemic lockdown period, due to shut down and strict restrictions, an improvement in air quality levels was observed. Their results indicate that during the lockdown period, the concentrations of PM10, PM2.5, NO2, and SO2 decreased by 24–44%, 23–58%, 30–64%, and 35–70%, respectively. According to Zambrano-Monserrate et al. (2020) just after the execution of lockdown, the concentrations of PM2.5 and NO2 were significantly reduced. Another study identified the reduction in pollutant emissions along the Yangtze River Delta using satellite and observed data. The study finds that the water-soluble ions (WSI) were decreased during lockdown and pollutant levels also followed a reduction during the lockdown period (Wang et al., 2021a; Wang et al., 2021b). A similar study is carried out to observe the change of urbanization during COVID-19, and it was observed that pollutant levels in larger cities are more than that in smaller cities (Shen L et al., 2021). Air pollution results in Brescia also show a similar decrease in air pollutants due to lockdown restrictions. A study shows that despite the reduction in emission sources during the lockdown period, which allowed to reach a significant reduction of NOx emissions, the PM levels did not have a similar trend if the annual (not the mean in 3 years) decrease of PM is considered (Bontempi et al., 2022).
To prevent the large-scale spread of the COVID-19 pandemic, China was the first country to carry out a nationwide lockdown to shut down all essential activities such as transportation, educational institutions, markets, and commercial and industrial activities at the end of January 2020 (Tian et al., 2020; Wang Y et al., 2020). Many researchers found that during this period, China found a new pattern of air quality (Chu et al., 2020; Xu et al., 2020; Zheng et al., 2020). Compared to 2017–19, in 366 urban areas and territories across China, the concentrations of particulate matter with an aerodynamic diameter of <10 and 2.5 µm, SO2, NO2, and CO were decreased, while the concentrations of O3 increased (Chen et al., 2020). Due to the reduced economic activities and energy consumption in China during the COVID-19 pandemic, NO2 concentration was significantly reduced, as reported by NASA (NASA 2020). Meanwhile, concentrations of PM10, PM2.5, and NO2 also decreased during the lockdown period, while those of SO2 and O3 increased in Hangzhou, China. During the COVID-19 pandemic lockdown in northern China, reduced economic, social, and industrial activities resulted in substantial improvement in air quality (Wang L et al., 2020).
Our study assessed the six air pollutant parameters (PM10, PM2.5, SO2, NO2, CO, and O3) in Nanjing, using daily average data obtained from nine monitoring sites. During the COVID-19 pandemic, our study determined the impact of lockdown and discovered new findings and substantial changes in the selected air pollutant parameters. Compared with a number of studies related to COVID-19, our study provides better results with a new pattern (Agarwal et al., 2020; Anil and Alagha, 2020; Islam et al., 2020; Kumar et al., 2020; Singh and Chauhan 2020; Aamir et al,. 2021; Borhani et al., 2021; El-Sheekh and Hassan 2021; Shen N et al., 2021). The objective of the current study was to 1) evaluate the spatiotemporal impact of the COVID-19 lockdown measures on air quality in different time periods, 2) determine the variation, and 3) find out the air pollutant concentrations from the previous 3 years to the lockdown period. The study provides valuable materials and new findings for the scholars and local bodies to make policies to improve and control air pollution.
The remainder of the article is organized as follows: section 2 presents the study area, air quality data, study period, data analysis, and the model. Section 3 presents the results and discussion. Section 4 discusses the conclusions.
2 Materials and methods
2.1 Study area
Nanjing, the capital of Jiangsu Province, is one of the most economic hubs of the Yangtze River Delta (YRD) with a large population and well-developed industrial and manufacturing zones. Due to rapid development in different sectors, the city has been facing extreme air pollution in recent years (Zu et al., 2017). Nanjing lies between latitude 31°14′ and 32°37′ and longitude 118°22′ and 119°14′ with 11 districts that can be divided into three regions, urban, rural, and suburban, covering an area of 6596 km2. With a density of 8500 people/km2, the population of Nanjing was approximately 8.34 million according to 2019. The city has four distinct seasons having a subtropical monsoon climate (Nanjing Municipal Government 2018). The main and vital industries in Nanjing are automobile, electronics, steel smelting, and petrochemical.
Presently, a total of nine monitoring stations are working to monitor and record air pollutant concentrations in Nanjing, and their locations are shown in Figure 1.
2.2 Air quality data and study period
To assess the impact of nationwide lockdown on air pollution, daily average concentrations of the six air pollutants, namely, PM10 (particulate matter with diameters of ≤10 µm), PM2.5 (particulate matter with diameters of ≤2.5 µm), SO2 (sulfur dioxide), NO2 (nitrogen dioxide), CO (carbon monoxide), and O3 (ozone) were collected from the Nanjing Environmental Monitoring Centre. A total of nine monitoring stations are working to collect and record air pollutant concentrations in Nanjing. According to the Technical Regulation for Selection of Ambient Air Quality Monitoring Stations of China, these nine monitoring stations belong to different areas that are Xianlin, Pukou, Xuanwuhu, Ruijinlu, Caochangmen, Maigaoqiao, Zhonghuamen, Shanxilu, and Aotizhongxin. In our study, the data were divided into six periods, pre-lockdown: 11 November 2019 to 24 January 2020 (75 days), lockdown period: 25 January 2020 to 8 April 2020 (75 days), post-lockdown: 9 April 2020 to 22 June 2020 (75 days), P4–P6: same dates of lockdown in 2017, 2018, and 2019.
2.3 Data analysis
In this study, summary statistics were calculated during the study period for different variables. This study investigates variations in concentrations of air pollutants in multiple periods as described in the abovementioned lines. In our analysis, the net difference and percentage change were also examined. Box plots were generated to represent the concentrations of these pollutants during the study period. In addition, during the lockdown period, to investigate the relationships between the air pollutants, linear regression analysis was performed.
2.4 HYSPLIT model
The backward trajectories were calculated to study the transport pathways of air mass reaching Nanjing using the NOAA HYSPLIT model (https://ready.arl.noaa.gov/HYSPLIT.php). The model is widely used in atmospheric research and pollution process analysis. The backward trajectories were plotted to identify the sources of air mass in the three periods, pre-LD, active-LD, and post-LD in Nanjing. These trajectories were analyzed for 72 h, starting every 6 h each day. Stein et al. (2015) discussed the details of the HYSPLIT model.
3 Results and discussion
3.1 Understanding the pattern of particulate matter
Due to the large-scale spread of the COVID-19 pandemic, the Government of the People’s Republic of China imposed a lockdown in late January 2020. Consequently, pollution levels during the lockdown period across the country have significantly reduced. According to many studies, a sudden decrease in pollutant concentrations was observed all around the world (Abdullah et al., 2020; Bao and Zhang 2020; Li et al., 2020; Zambrano-Monserrate and Ruano 2020; Bhatti et al., 2022b). The concentration patterns of particulate matter and variations are shown in Figure 2 and Tables 1, 2, 3. Our results demonstrate that compared to the pre-lockdown period, a notable reduction in the concentrations of particulate matter was observed in Nanjing, China. According to the obtained results, the concentrations of PM10 and PM2.5 reduced by −33.03% and −35.41%, respectively, during the COVID-19 lockdown period in Nanjing. According to the study by Shakoor et al. (2020), an approximately 38 and 18% reduction in the concentrations of PM10 and PM2.5 were recorded, respectively, during the lockdown period in China. Another study stated by Berman and Ebisu (2020) revealed that during the COVID-19 pandemic lockdown period in the United States, there was a decline in PM2.5 concentration (11%). In urban areas and regional areas, road dust, transportation, and industrial and construction activities are the main and important sources of particulate matter. The reduction in these sources caused a massive decrease in the concentrations of particulate matter in many areas during the COVID-19 pandemic lockdown (Mor et al., 2021). In addition, the study showed that the concentrations of PM10 and PM2.5 decreased by -15.50% and -32.77%, respectively, from pre- to post-periods in Nanjing. In these findings, the reduction in the concentration of PM2.5 was higher than that of PM10.
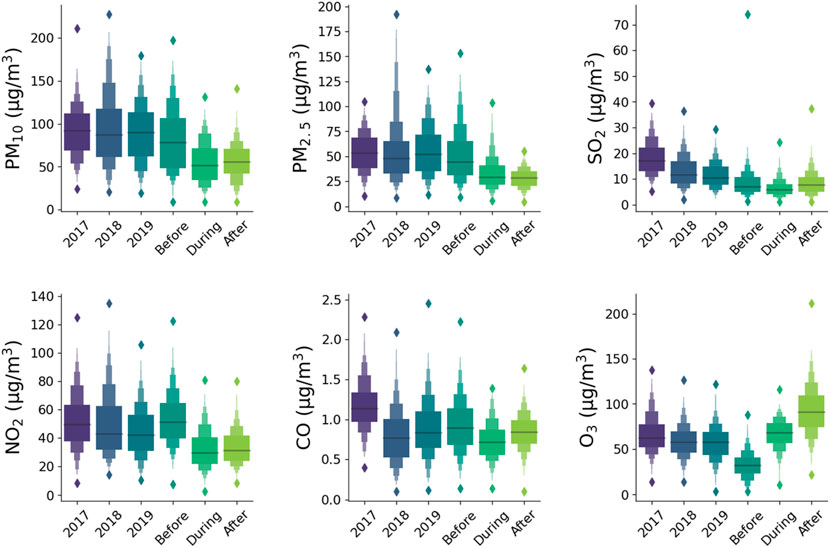
FIGURE 2. Daily average concentrations of PM10, PM2.5, SO2, NO2, CO, and O3 during the all-study periods in Nanjing.
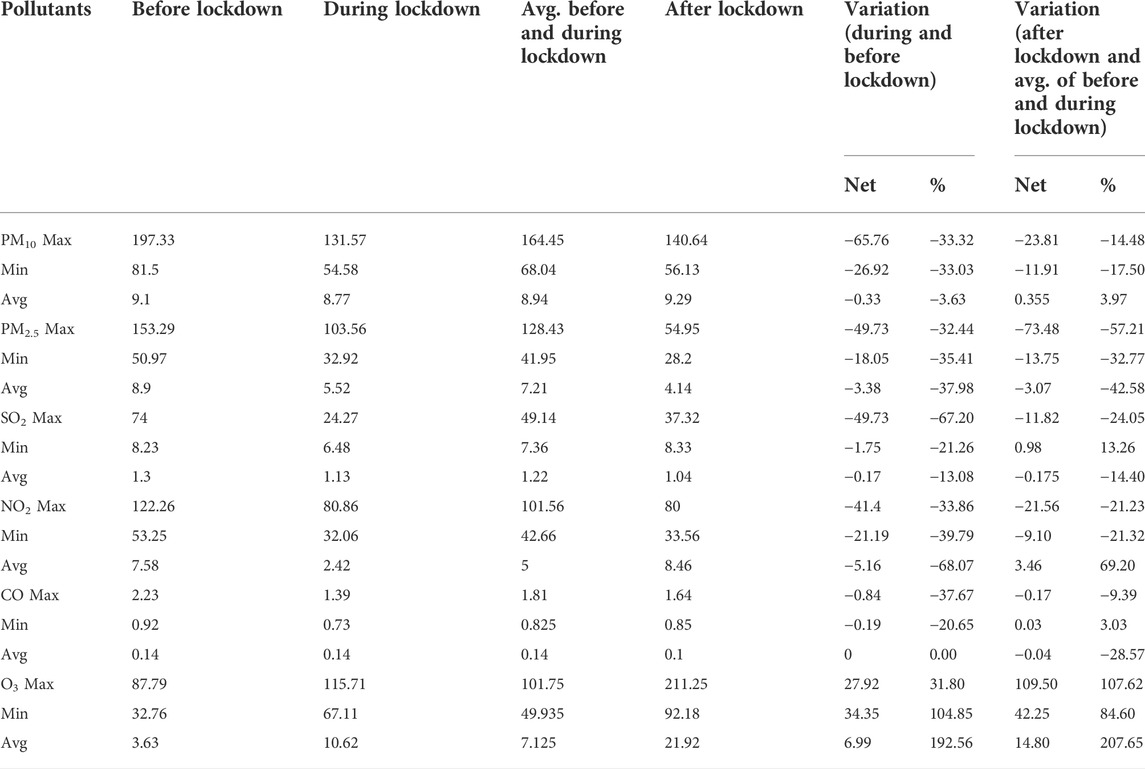
TABLE 1. Daily average concentration and variation of pollutants in different time periods in Nanjing.
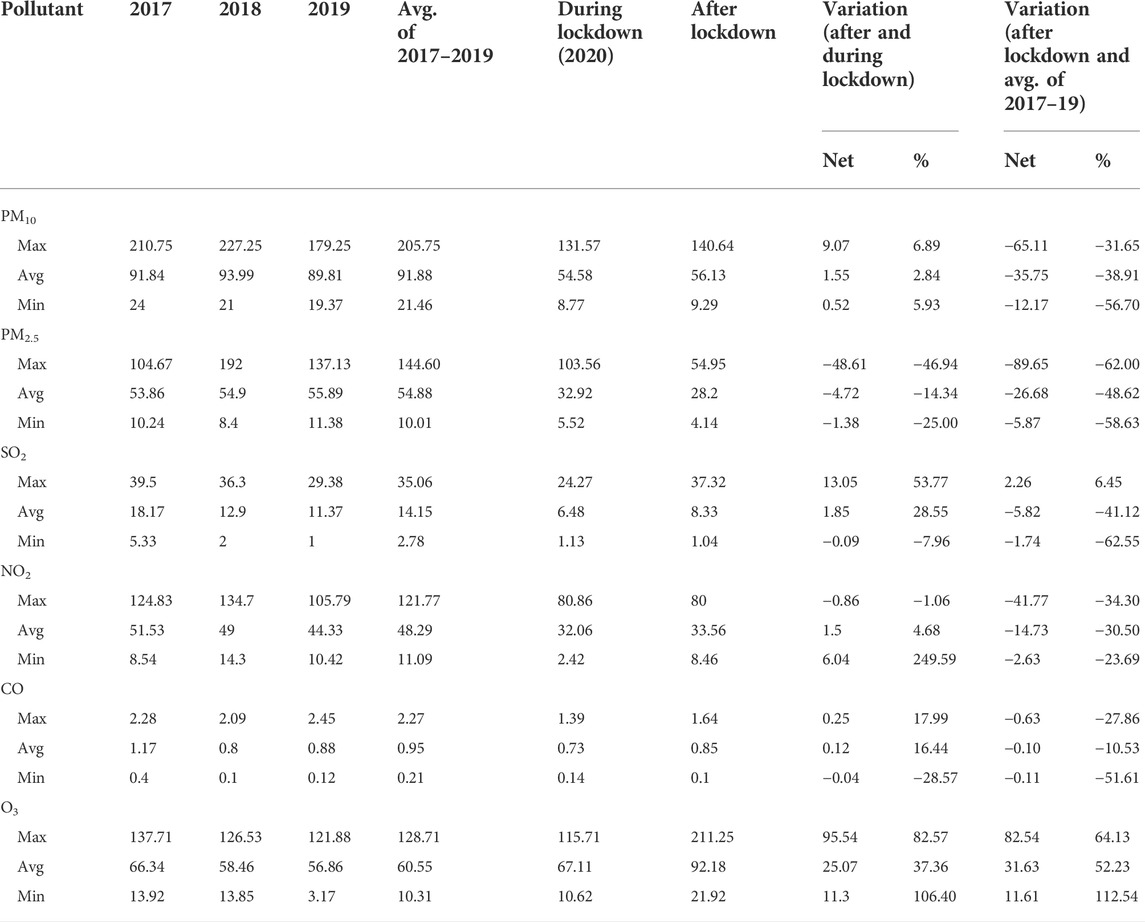
TABLE 3. Daily average concentration and variation of pollutants in different time periods in Nanjing.
Our results demonstrate that in comparison to last year (2019), PM10 and PM2.5 concentrations showed decreasing trends, reduced by an average of -39.23% and -41.10%, respectively. Compared with the last 3 years (2017–19), Nanjing experienced a high reduction in the concentrations of PM10 and PM2.5 by −40.60% and −40.02%, respectively. It can be seen that PM10 and PM2.5 showed similar decreasing trends in this period. A similar pattern was evident by Chen et al. (2020) compared to the corresponding years (2017–19), and the concentrations of particulate matter (PM10, PM2.5) showed a diminishing tendency during the COVID-19 pandemic lockdown period in China. Furthermore, Mahato et al. (2020) reported that during the lockdown period, a significant improvement in the particulate matter was recorded as compared to the previous 3 years (2017–19) in megacity Delhi, India. Islam et al. (2020) found that PM10 and PM2.5 showed a momentous drop from last year (2019) to the lockdown period in Bangladesh. Due to the execution of lockdown in different areas and regions of the world, all essential activities were stopped, such as transportation, construction, and educational, cultural, and industrial activities, which caused a fall in air pollutant levels.
Moreover, compared with the lockdown period, a slight increase in PM10 concentration (2.84%) was found, while in contrast, PM2.5 showed a decreasing trend (−14.34%) after the lockdown period in Nanjing. Compared with the lockdown period, the increase in PM10 concentration can be attributed to the increase in transportation and industrial and construction activities after the lockdown (Mor et al., 2021). In comparison to the preceding 3 years (2017–19), a substantial reduction in PM concentrations was seen after the COVID-19 pandemic lockdown in Nanjing. The results indicate that compared with those in the last 3 years (2017–19), PM10 and PM2.5 showed decreasing trends, reduced by an average of −38.91% and −48.62%, respectively. We can observe that the reduction ratio in PM2.5 concentration is greater than that of PM10. In summary, a drastic drop and an improvement in PM concentration indicate that the COVID-19 pandemic lockdown measures have a considerable impact on changing and improving PM concentration in Nanjing.
3.2 Sulfur dioxide
The results demonstrate that during the lockdown period due to the COVID-19 pandemic, the concentration of SO2 decreased by −21.26% as compared to that of the pre-lockdown period in Nanjing. The SO2 concentration pattern is shown in Figure 2, and variations in SO2 during the study period are presented in Tables 1–3. Coal, diesel, power plants, vehicular traffic, and industrial and manufacturing activities are the main sources of SO2 (Lu et al., 2013). During the lockdown period, these types of activities reduced significantly, which resulted in a decline in SO2 concentration. Shakoor et al. (2020) reported similar findings that the concentration of SO2 decreased by 18% during the lockdown period, compared to the pre-lockdown period in China. Another study documented by Sharma et al. (2020) witnessed that during the lockdown period, SO2 showed a declining trend as compared to that in the pre-lockdown period. Additionally, the results indicated that compared with the pre- and during-lockdown periods, the city found an increasing trend in SO2 concentration (13.26%). Meanwhile, compared with last year (2019) (same dates of lockdown), a significant decreasing trend was observed in SO2 concentration by approximately −43.01%. According to Wang Y et al. (2020) and Islam et al. (2020), compared to last year (2019), SO2 concentration has decreased during the lockdown period in China and Bangladesh. Mahato et al. (2020) also found similar results that in comparison to the last year, SO2 concentration was reduced during the said time period in Delhi, India.
The current study found that in comparison to the previous 3 years (2017–19), SO2 showed a high decreasing trend of approximately −54.19% in Nanjing as shown in Table 2. We can observe that in these findings, the reduction spell in the concentration of SO2 was greater than that of the previous 3 years than last year (2019) in the city. According to Chen et al. (2020), compared to the last 3 years (2017–19), SO2 concentration has reduced in China. Similar results were reported that compared to the last 3 years during the said time period, SO2 concentration was decreased in megacity Delhi, India (Mahato et al., 2020). Our results indicate that the concentration of SO2 increased by 28.55% from the active- to post-lockdown period, while compared with the last 3 years 2019–17 (same time frame of lockdown), the SO2 concentration showed a declining trend (-41.12%) after the lockdown period in Nanjing (Table 3). Overall, the results revealed that the COVID-19 pandemic lockdown has noteworthy impacts on changing and diminishing SO2 concentration during the study period in Nanjing.
3.3 Nitrogen dioxide
The NO2 concentration patterns are shown in Figure 2, and variations in NO2 are presented in Tables 1–3. Generally, NO2 is released from vehicular emissions, fossil fuels, natural fires, lightning, and soils (Reddy et al., 2012). The results of our study demonstrate that compared with the lockdown period, a sizeable decline was observed in NO2 concentration by approximately -39.79 in Nanjing. This reduction in NO2 concentration could be attributed to the reduced industrial and vehicular emissions during the lockdown period in Nanjing. The most momentous similarity with our results is from the study by Shakoor et al. (2020) that the NO2 concentration was reduced by −39% during the lockdown period in China. Another study documented by Agarwal et al. (2020) also supported our results that during the COVID-19 control period, NO2 levels decreased by −37%, in China. According to Bao and Zhang (2020) during the lockdown period, a significant decline was observed in NO2 concentration in many areas and regions across China. All of these findings support our results that NO2 concentration was reduced during the lockdown period in Nanjing. In addition, our findings demonstrate that after the lockdown, NO2 concentration decreased by approximately -21.32% in comparison to the pre- and during-lockdown periods in Nanjing. The reduction ratio in NO2 was quite lower after the lockdown than during the pre- and during-lockdown periods and during the lockdown in comparison to the pre-lockdown period.
The results indicate that compared with the last year (2019), there was a marked decline in NO2 concentration, to -27.68%, during the lockdown period, while in comparison to the previous 3 years (2017–19), NO2 has been reduced by up to -33.60%. Chen et al. (2020) reported that in comparison to the last year (2019), NO2 showed a declining trend during the lockdown period in China. Islam et al. (2020) also found that compared to the last year (2019), an approximately 35–45% reduction in NO2 concentration has been observed in Bangladesh. According to Fu et al. (2020), in comparison to the last 5 years (2015–19), NO2 concentration was at its lowest level during the lockdown period, and while compared with the last year (2019) measurements, NO2 was reduced by approximately 34.7% in North China. Another study revealed that compared to the last 3 years (2017–19), NO2 was reduced significantly during the lockdown period in Delhi (India) (Mahato et al., 2020). Our results demonstrate that compared with the lockdown period, there was a slight increase in NO2 concentration by 4.68% after lockdown while compared to the last 3 years (2017–19), and NO2 has been reduced by an average of -30.50% in Nanjing. The increasing spell in NO2 concentration after lockdown compared with the lockdown period could be attributed to the winter–summer change (Ghosh et al., 2017). In summary, the results show that the COVID-19 pandemic lockdown and forced restrictions have a momentous impact on changing and upgrading Nanjing’s air pollution.
3.4 Carbon monoxide
CO is mainly a colorless gas emanated from biofuel burning, vehicular emission, agricultural waste burning, and combustion of fossil fuels (Hasnain et al., 2021). Figure 2 shows the concentration pattern of CO, and variations in CO are presented in Tables 1–3. According to our findings, from pre to during lockdown, CO levels decreased by approximately -20.65% in Nanjing. This reduction in CO concentration can be attributed to the restriction on transportation and industrial activities during the COVID-19 control period. According to Sulaymon et al. (2021), during the lockdown period, the concentration of CO reduced in Wuhan, China. A significant decrease in CO concentration was also observed from pre to active lockdown in Delhi, India (Mahato et al., 2020). Compared with the pre and lockdown periods, CO showed an increasing trend after the lockdown period. The results reveal that the increase in the concentration of CO was 3.03% after the lockdown. This shows that the increase in CO levels was due to regular actions such as transportation and industrial activities after the lockdown.
The results demonstrate that compared with last year (2019), the city experienced a sizeable decline in the levels of CO. During this window of time, the reduction in CO was −17.05% in Nanjing. Islam et al. (2020) reported that during the lockdown phase, CO exhibited a declining trend compared with the previous year in Bangladesh. Moreover, compared with the last 3 years, the concentration of CO decreased a significant level. We found a remarkable change and decrease in CO concentration by up to −23.16% in Nanjing. It can be noted that the declining value in the concentration of CO was higher in the previous 3 years to the COVID-19 control period than in the last 1 year to the COVID-19 period. A study reported by Hasnain et al. (2021) supports our findings, which indicates that from the previous 3 years to the lockdown period, the concentration level of CO has decreased. Furthermore, the concentration of CO showed an increasing trend from the lockdown period to the post-lockdown period, which increased by approximately 16.44%. Compared with the previous 3 years, CO presented an opposite pattern, to be decreased by up to −10.53% in Nanjing. The results suggest that the COVID-19 lockdown has a large impact on CO concentration.
3.5 Ozone
In the analysis of O3, compared with the pre-lockdown period, we found a significant increase in O3 levels during the COVID-19 period in Nanjing. The results indicate that from pre to during the lockdown periods, the levels of O3 increased by up to 104.85%, and while compared with the pre and during the lockdown periods, the rising value in the concentration of O3 was 84.60% in Nanjing. Figure 2 shows the concentration pattern of O3, and variations in O3 are presented in Tables 1–3. Previously, it has been reported that during the lockdown period, the concentration level of O3 increased in China (Fu et al., 2020; Hasnain et al., 2021). Another study documented by Islam et al. (2020) showed that during the COVID-19 period, O3 concentration decreased in Bangladesh.
O3 is mainly emitted from sunlight, volatile organic compounds (VOCs), and nitrogen oxides (NOx) (Lal et al., 2020). Compared with last year (2019), O3 showed a similar trend, to be increased by an average of 18.03% in Nanjing (Table 2). From the previous 3 years to the lockdown period, our study showed that the increasing value for O3 was 10.83%. According to Fu et al. (2020), during the lockdown period, O3 levels increased in Guangxi, South China compared with that in the previous 4 years. During the lockdown period in Wuhan, Sulaymon et al. (2021) reported that O3 concentration was increased. Compared to last year (2019), the increasing spell in the concentration of O3 was greater than in the previous 3 years to the lockdown period in Nanjing.
Moreover, our results reveal that the concentration of O3 continuously increased during the lockdown period to post-lockdown period in Nanjing. The decreasing value during this period was 37.36% while compared with the last 3 years to the post lockdown period, and O3 levels increased by approximately 52.23%. It should be noted that in opposition to other pollutants, O3 is the only pollutant that showed an increasing trend during the all-study periods in Nanjing. Previously, it was reported by Hasnain et al. (2021) that during the post-lockdown period, the concentration of O3 had increased. The results suggest that from the COVID-19 control period to the post-lockdown period, the increase in the concentration of O3 was due to the winter–summer change.
3.6 Backward trajectory analysis
The backward trajectories were plotted to trace the sources of air masses during the pre-LD, active-LD, and post-LD periods in Nanjing (Figure 3). The backward trajectories were analyzed for 72 h, starting every 6 h each day. We can see that during the pre-LD period, the trajectories originated from Siberia and passed through Mongolia and northwestern China. During the active-LD period, the air mass comes from the northwest of Mongolia and passed through the Liaoning Province before arriving in Nanjing. During the post-LD period, the air mass comes from Inner Mongolia and passed near the Beijing–Tianjin–Hebei (BTH) region and the East China Sea before reaching Nanjing. The air masses that are coming from Mongolia and passing near the Liaoning Province and the BTH region affect the air quality of Nanjing. The HYSPLIT model reveals the influence of long-range transport of air mass over Nanjing.
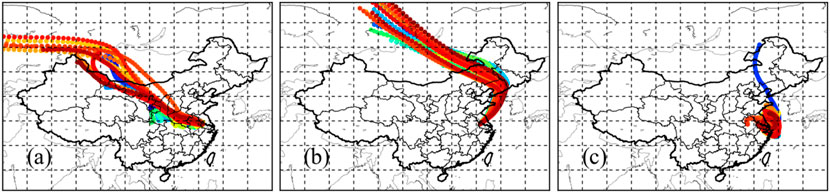
FIGURE 3. HYSPLIT backward trajectories over Nanjing (A) pre-lockdown, (B) during the lockdown, and (C) post-lockdown.
3.7 Co-relationships between air pollutants
The correlations between the daily average concentration of air pollutants in Nanjing during the lockdown period are shown in Figure 4. There was a significant correlation between PM10 and PM2.5 (r = 0.71), while there was also a strong correlation between PM10 and SO2 (r = 0.42), with NO2 (r = 0.30). The results show that the strong correlation between PM10 and PM2.5 can be attributed to mutual pollution sources. The correlation between PM10 and CO was (r = 0.45), and O3 (r = 0.13). In contrast to the correlation between PM10, SO2, and NO2, during the lockdown period, a weak correlation was observed between PM2.5 and SO2 (r = 0.04) with NO2 (r = 0.01). PM2.5 concentration showed a positive correlation with the concentration of CO (r = 0.36), with O3 (r = 0.03). Moreover, SO2 was highly correlated with NO2 (r = 0.70), SO2 with CO (r = 48), and SO2 with O3 (r = 0.03) during the lockdown period in Nanjing. This strong correlation between SO2 and NO2 reveals that it was due to their reduced mutual pollution sources during the lockdown period in the city. The concentration of NO2 was significantly correlated with CO concentration (0 = 0.40), while there was no correlation between NO2 and O3 and CO with O3. The results indicate that the concentration of CO was positively correlated with all other pollutants except with O3, while the concentration of O3 showed weak or no correlation with other pollutants. It suggests that the concentration of O3 increased with the reduction in the concentrations of other pollutants during the lockdown period in Nanjing.
4 Conclusions
In general, a pronounced improvement in air quality was observed during the COVID-19 pandemic lockdown period in Nanjing. In order to evaluate the impact of the COVID-19 pandemic lockdown measures on air pollution, this is an excellent opportunity to work in this direction in different parts of the world. In this study, the effects of the COVID-19 pandemic lockdown on air quality were examined using daily average data for the six air pollutants in Nanjing. The results illustrate that compared to the pre-lockdown period, all the air pollutants showed a remarkable decline during the lockdown period, except for O3 in Nanjing. Among these pollutants, the concentrations of PM10 and PM2.5 were significantly decreased by an average of -33.03% and -35.41%, respectively. Among other pollutants, compared with the pre-lockdown period, SO2 (−21.26%), NO2 (−39.79%), and CO (-20.65%) were also reduced, while O3 levels significantly increased (104.85%) during the lockdown period. Among the selected pollutants, PM10, SO2, NO2, CO, and O3 increased by 2.84%, 28.55%, 4.68%, 16.44%, and 37.36%, respectively, while PM2.5 was the only pollutant that increased by an average of 14.34% after the lockdown period. The study revealed that compared with the last year (2019) (same time frame of lockdown), a significant drop and reduction in all pollutants were observed, while O3 showed an increasing trend. Overall, except for O3, a substantial decrease in all air pollutants was found during the lockdown period in Nanjing. The results of this research will be helpful for the local bodies of the city and the Chinese administration to develop rules and regulations to improve and upgrade air quality in the future.
Data availability statement
The original contributions presented in the study are included in the article/Supplementary Material; further inquiries can be directed to the corresponding authors.
Author contributions
SF: conceptualization, methodology, data curation, writing—original draft, writing—review and editing, visualization, project administration, and funding acquisition; RAW: data curation, writing—original draft, writing—review and editing, and visualization; AHH: conceptualization, methodology, data curation, writing—original draft, writing—review and editing, supervision, resources, project administration, and funding acquisition; AAH: data curation, writing—original draft, and writing—review and editing; UAB: investigation, data curation, writing—original draft, writing—review and editing, supervision, resources, and project administration; EE: investigation, data curation, and writing—review and editing.
Funding
This research was supported by the Key Fund of the National Natural Science Foundation of China (grant No. 41631175). This research is supported by the Youth Foundation of Hubei Educational Committee, 2021, Science and Technology Research Project, Research on the Deep Active Intelligent Interpretation Method of Unbalanced Remote Sensing Image under “Quantity-Spectrum Synergy”, Young and middle-aged talents Project, No. Q20212904 and The Huanggang Normal University High-level Cultivation Project of 2021, Remote sensing data mining with an unbalanced dataset based on deep learning, NO. 202111804.
Conflict of interest
The authors declare that the research was conducted in the absence of any commercial or financial relationships that could be construed as a potential conflict of interest.
Publisher’s note
All claims expressed in this article are solely those of the authors and do not necessarily represent those of their affiliated organizations, or those of the publisher, the editors, and the reviewers. Any product that may be evaluated in this article, or claim that may be made by its manufacturer, is not guaranteed or endorsed by the publisher.
References
Aamir, M., Li, Z., Bazai, S., Wagan, R. A., Bhatti, U. A., Nizamani, M. M., and Akram, S. (2021). Spatiotemporal change of air-quality patterns in Hubei province—a pre-to post-COVID-19 analysis using path analysis and regression. Atmosphere 12 (10), 1338. doi:10.3390/atmos12101338
Abdullah, S., Mansor, A. A., Napi, N. N. L. M., Mansor, W. N. W., Ahmed, A. N., Ismail, M., and Ramly, Z. T. A. (2020). Air quality status during 2020 Malaysia Movement Control Order (MCO) due to 2019 novel coronavirus (2019-nCoV) pandemic. Sci. Total Environ. 729, 139022. doi:10.1016/j.scitotenv.2020.139022
Agarwal, A., Kaushik, A., Kumar, S., and Mishra, R. K. (2020). Comparative study on air quality status in Indian and Chinese cities before and during the COVID-19 lockdown period. Air. Qual. Atmos. heal. 13, 1167. doi:10.1007/s11869-020-00881-z
Anil, I., and Alagha, O. (2020). The impact of COVID-19 lockdown on the air quality of Eastern Province, Saudi Arabia. Air Qual. Atmos. Health 14, 117–128. doi:10.1007/s11869-020-00918-3
Bao, R., and Zhang, A. (2020). Does lockdown reduce air pollution? Evidence from 44 cities in northern China. Sci. Total Environ. 731, 139052. doi:10.1016/j.scitotenv.2020.139052
Berman, J. D., and Ebisu, K. (2020). Changes in U.S. air pollution during the COVID-19 pandemic. Sci. Total Environ. 739, 139864. doi:10.1016/j.scitotenv.2020.139864
Bhatti, U. A., Wu, G., Bazai, S. U., Nawaz, S. A., Baryalai, M., Bhatti, M. A., Nizamani, M. M., et al. (2022a). A pre-to post-COVID-19 change of air quality patterns in anhui Province using path analysis and regression. Pol. J. Environ. Stud. doi:10.15244/pjoes/148065
Bhatti, U. A., Zeeshan, Z., Nizamani, M. M., Bazai, S., Yu, Z., and Yuan, L. (2022b). Assessing the change of ambient air quality patterns in Jiangsu Province of China pre-to post-COVID-19. Chemosphere 288, 132569. doi:10.1016/j.chemosphere.2021.132569
Bontempi, E., Carnevale, C., Cornelio, A., Volta, M., and Zanoletti, A. (2022). Analysis of the lockdown effects due to the COVID-19 on air pollution in Brescia (Lombardy). Environ. Res. 212, 113193. doi:10.1016/j.envres.2022.113193
Borhani, F., Motlagh, M. S., Stohl, A., Rashidi, Y., and Ehsani, A. H. (2021). Changes in short-lived climate pollutants during the COVID-19 pandemic in Tehran, Iran. Environ. Monit. Assess. 193–331. doi:10.1007/s10661-021-09096-w
Chen, Q. X., Huang, C. L., Yuan, Y., and Tan, H. P. (2020). Influence of COVID-19 Event on Air Quality and their Association in Mainland Chinafluence of COVID-19 eventon air quality and their association in mainland China. Aerosol Air Qual. Res. 20, 1541–1551. doi:10.4209/aaqr.2020.05.0224
Chu, B., Zhang, S., Liu, J., Ma, Q., and He, H. (2020). Significant concurrent decrease in PM2.5 and NO2 concentrations in China during COVID-19 epidemic. J. Environ. Sci. 99, 346. doi:10.1016/j.jes.2020.06.031
El-Sheekh, M. M., and Hassan, I. A. (2021). Lockdowns and reduction of economic activities during the COVID-19 pandemic improved air quality in Alexandria, Egypt. Environ. Monit. Assess. 193, 11. doi:10.1007/s10661-020-08780-7
Fu, S., Guo, M., Fan, L., Deng, Q., Han, D., Wei, Y., Luo, J., Qin, G., and Cheng, J. (2020). Ozone pollution mitigation in guangxi (south China) driven by meteorology and anthropogenic emissions during the COVID-19 lockdown. Environ. Pollut. 272, 115927. doi:10.1016/j.envpol.2020.115927
Ghosh, D., Lal, S., and Sarkar, U. (2017). Variability of tropospheric columnar NO2 and SO2 over eastern Indo-Gangetic Plain and impact of meteorology. Air Qual. Atmos. Health 10 (5), 565–574. doi:10.1007/s11869-016-0451-y
Hasnain, A., Hashmi, M. Z., Bhatti, U. A., Nadeem, B., Wei, G., Zha, Y., et al. (2021). Assessment of air pollution before, during and after the COVID-19 pandemic lockdown in nanjing, China. Atmosphere 12, 743. doi:10.3390/atmos12060743
Holcombe, M., and O’Key, S. (2020). Satellite images show less pollution over the US as coronavirus shuts down public places. CNN (23 March 2020), Available at: https://go.nature.com/2VzglPH.
Islam, M. S., Tusher, T. R., Roy, S., and Rahman, M. (2020). Impacts of nationwide lockdown due to COVID-19 outbreak on air quality in Bangladesh: a spatiotemporal analysis. Air Qual. Atmos. Health 14, 351–363. doi:10.1007/s11869-020-00940-5
Kumar, P., Hama, S., Omidvarborna, H., Sharma, A., Sahani, J., Abhijith, K. V., Debele, S. E., Zavala-Reyes, J. C., Bar-wise, Y., and Tiwari, A. (2020). Temporary reduction in fine particulate matter due to ‘anthropogenic emissions switch-off’ during COVID-19 lockdown in Indian cities. Sustain. Cities Soc. 62, 102382. doi:10.1016/j.scs.2020.102382
Lal, S., Naja, M., and Subbaraya, B. (2020). Seasonal variations in surface ozone and its precursors over an urban site in India. Atmos. Environ. X. 34, 2713–2724. doi:10.1016/s1352-2310(99)00510-5
Li, L., Li, Q., Huang, L., Wang, Q., Zhu, A., Xu, J., Liu, Z., Li, H., Shi, L., Li, R., Azari, M., et al. (2020). Air quality changes during the COVID-19 lockdown over the Yangtze River Delta region: an insight into the impact of human activity pattern changes on air pollution variation. Sci. Total Environ. 732, 139282. doi:10.1016/j.scitotenv.2020.139282
Lu, Z., Streets, D. G., Foy, B., and Krotkov, N. A. (2013). Ozone Monitoring Instrument observations of interannual increases in SO2 emissions from Indian coal-fired power plants during 2005e2012. Environ. Sci. Technol. 47 (24), 13993–14000. doi:10.1021/es4039648
Mahato, S., Pal, S., and Ghosh, K. G. (2020). Effect of lockdown amid COVID-19 pandemic on air quality of the megacity Delhi, India. Sci. Total Environ. 730, 139086. doi:10.1016/j.scitotenv.2020.139086
McMahon, J. S. (2020). Coronavirus lockdown likely saved 77,000 lives in China just by reducing pollution. Forbes (16 March 2020). Available at: https://go.nature.com/2BiWBt3.
Mor, S., Kumar, S., Singh, T., Dogra, S., Pandey, V., and Ravindra, K. (2021). Impact of COVID-19 lockdown on air quality in Chandigarh, India: Understanding the emission sources during controlled anthropogenic activities. Chemosphere 263, 127978. doi:10.1016/j.chemosphere.2020.127978
Nanjing Municipal Government (2018). Available at: http://www.nanjing.gov.cn, accessed 12.21.2018.
NASA (2020). Airborne nitrogen dioxide plummets over China. Available at: https://earthobservatory.nasa.gov/images/146362/airborne-nitrogendioxideplummets-over-china.
Reddy, B. S. K., Kumar, K. R., Balakrishnaiah, G., Gopal, K. R., Reddy, R. R., Sivakumar, V., et al. (2012). Analysis of diurnal and seasonal behavior of surface ozone and its precursors (NOx) at a semi-arid rural site in southern India. Aerosol Air Qual. Res. 12, 1081–1094. doi:10.4209/aaqr.2012.03.0055
Shakoor, A., Chen, X., Farooq, T. H., Shahzad, U., Ashraf, F., Rehman, A., Sahar, N., and Yan, W. (2020). Fluctuations in environmental pollutants and air quality during the lockdown in the USA and China: two sides of COVID-19 pandemic. Air Qual. Atmos. Health 13, 1335–1342. doi:10.1007/s11869-020-00888-6
Sharma, M., Jain, S., and Lamba, B. Y. (2020). Epigrammatic study on the effect of lockdown amid Covid-19 pandemic on air quality of most polluted cities of Rajasthan (India). Air Qual. Atmos. Health 13, 1157–1165. doi:10.1007/s11869-020-00879-7
Shen, L., Wang, H., Zhu, B., Zhao, T., Liu, A., Lu, W., and Wang, Y. (2021). Impact of urbanization on air quality in the Yangtze River Delta during the COVID-19 lockdown in China. J. Clean. Prod. 296, 126561. doi:10.1016/j.jclepro.2021.126561
Shen, N., Zhao, X., Li, L., Zhou, B., Duan, F., and Zhao, W. (2021). Spatial and temporal variation characteristics of atmospheric NO2 and SO2 in the Beijing-Tianjin-Hebei region before and after the COVID-19 outbreak. Air Qual. Atmos. Health 14, 1175–1188. doi:10.1007/s11869-021-01016-8
Singh, R. P., and Chauhan, A. (2020). Impact of lockdown on air quality in India during COVID-19 pandemic. Air Qual. Atmos. Health 13, 921–928. doi:10.1007/s11869-020-00863-1
Stein, A. F., Draxler, R. R., Rolph, G. D., Stunder, B. J., Cohen, M. D., Ngan, F., Stein, A. F., Draxler, R. R., Rolph, G. D., Stunder, B. J., and Cohen, M. D. (2015). NOAA’s HYSPLIT atmospheric transport and dispersion modeling system. Bull. Am. Meteorol. Soc. 96, 2059–2077. doi:10.1175/bams-d-14-00110.1
Sulaymon, I. D., Zhang, Y., Hopke, P. K., Zhang, Y., Hua, J., and Mei, X. (2021). COVID-19 pandemic in Wuhan: Ambient air quality and the relationships between criteria air pollutants and meteorological variables before, during, and after lockdown. Atmos. Res. 250, 105362. doi:10.1016/j.atmosres.2020.105362
Tian, H., Liu, Y., Li, Y., Wu, C. H., Chen, B., Kraemer, M. U. G., Li, B., Cai, J., et al. (2020). An investigation of transmission control measures during the first 50 days of the COVID-19 epidemic in China. Science 368 (6491), 638–642. doi:10.1126/science.abb6105
Wang, C., Horby, P. W., Hayden, F. G., and Gao, G. F. (2020). A novel coronavirus outbreak of global health concern. Lancet 395, 470–473. doi:10.1016/s0140-6736(20)30185-9
Wang, H., Miao, Q., Shen, L., Yang, Q., Wu, Y., and Wei, H. (2021b). Air pollutant variations in Suzhou during the 2019 novel coronavirus (COVID-19) lockdown of 2020: High time-resolution measurements of aerosol chemical compositions and source apportionment. Environ. Pollut. 271, 116298. doi:10.1016/j.envpol.2020.116298
Wang, H., Miao, Q., Shen, L., Yang, Q., Wu, Y., Wei, H., and Lu, W. (2021a). Characterization of the aerosol chemical composition during the COVID-19 lockdown period in Suzhou in the Yangtze River Delta, China. J. Environ. Sci. 102, 110–122. doi:10.1016/j.jes.2020.09.019
Wang, L., Li, M., Yu, S., Chen, X., Li, Z., Zhang, Y., et al. (2020). Unexpected rise of ozone in urban and rural areas, and sulfur dioxide in rural areas during the coronavirus city lockdown in Hangzhou, China: implications for air quality. Environ. Chem. Lett. 18, 1713–1723. doi:10.1007/s10311-020-01028-3
Wang, Y., Yuan, Y., Wang, Q., Liu, C. G., Zhi, Q., and Cao, J. (2020). Changes in air quality related to the control of coronavirus in China: implications for traffic and industrial emissions. Sci. Total Environ. 731, 139133. doi:10.1016/j.scitotenv.2020.139133
World Health Organization (WHO). (2020). Corona virus disease (COVID19). Available at: https://www.who.int/docs/defaultsource/coronaviruse/situation-reports/20200805-covid-19-sitrep-198.pdf? sfvrsn=f99d1754_2. Accessed 25 Oct 2020.
Xu, K., Cui, K., Young, L. H., Hsieh, Y. K., Wang, Y. F., Zhang, J., and Wan, S. (2020). Impact of the COVID-19 event on air quality in central China. Aerosol Air Qual. Res. 20, 915–929. doi:10.4209/aaqr.2020.04.0150
Zambrano-Monserrate, M. A., and Ruano, M. A. (2020). Has air quality improved in Ecuador during the COVID-19 pandemic? A parametric analysis. Air Qual. Atmos. Health 13, 929–938. doi:10.1007/s11869020-00866-y
Zambrano-Monserrate, M. A., Ruano, M. A., and Sanchez-Alcalde, L. (2020). Indirect effects of COVID-19 on the environment. Sci. Total Environ. 728, 138813. doi:10.1016/j.scitotenv.2020.138813
Zheng, H., Kong, S., Chen, N., Yan, Y., Liu, D., Zhu, B., Xu, K., et al. (2020). Significant changes in the chemical compositions and sources of PM2.5 in Wuhan since the city lockdown as COVID-19ficant changes in the chemical compositions and sources of PM2.5 in Wuhan since the city lockdown as COVID-19. Sci. Total Environ. 739, 140000. doi:10.1016/j.scitotenv.2020.140000
Keywords: COVID-19, lockdown, air quality, air pollution, Nanjing
Citation: Fei S, Wagan RA, Hasnain A, Hussain A, Bhatti UA and Elahi E (2022) Spatiotemporal impact of the COVID-19 pandemic lockdown on air quality pattern in Nanjing, China. Front. Environ. Sci. 10:952310. doi: 10.3389/fenvs.2022.952310
Received: 24 May 2022; Accepted: 09 August 2022;
Published: 11 October 2022.
Edited by:
Zeeshan Khan, Curtin University Sarawak, MalaysiaReviewed by:
Honglei Wang, Nanjing University of Information Science and Technology, ChinaElza Bontempi, University of Brescia, Italy
Copyright © 2022 Fei, Wagan, Hasnain, Hussain, Bhatti and Elahi. This is an open-access article distributed under the terms of the Creative Commons Attribution License (CC BY). The use, distribution or reproduction in other forums is permitted, provided the original author(s) and the copyright owner(s) are credited and that the original publication in this journal is cited, in accordance with accepted academic practice. No use, distribution or reproduction is permitted which does not comply with these terms.
*Correspondence: Uzair Aslam Bhatti, uzairaslambhatti@hotmail.com; Ahmad Hasnain, 31193024@njnu.edu.cn