- 1Department of Accounting and Taxation, Russian State Agrarian University-Moscow Timiryazev Agricultural Academy (RSAU-MAA named after K. A. Timiryazev), Moscow, Russia
- 2Rector, Moscow Witte University, Moscow, Russia
- 3Department of National Economy, Russian Presidential Academy of National Economy and Public Administration, Moscow, Russia
- 4Department of World Economy, Diplomatic Academy of the Russian Foreign Ministry, Moscow, Russia
Introduction
The era of artificial intelligence (AI) is a period when automation for the first time goes beyond the century-old practice of production and covers a wide range of organizational processes in which intellectual support for managerial decision making is provided (Dudukalov et al., 2021; Ivanov et al., 2022; Popkova, 2022). At the same time, the Sustainable Development Goals (SDGs) have become widespread in the global economic system. In their practical implementation, business practices are reviewed from the point of view of ESG principles and transformed in accordance with them. This process is called ESG investing, including environmental, social, and governance investing on a systemic basis (Gao et al., 2021; Popkova et al., 2021; Popkova and Sergi, 2021; Rehman and Noman, 2022).
ESG is a systemic approach to business management, which covers and reconsiders through the lens of the SDGs (orients toward their support) environmental (E: with the focus on corporate environmental responsibility), social (S: with the focus on corporate social responsibility), and corporate (G: with the focus on financial management, maximization of profit and increase in economic effectiveness of business) management. Thus, ESG investment is a process of financing sustainable development (Aldowaish et al., 2022; Ge et al., 2022).
The concept of “ESG performance” means that a company is evaluated (by shareholders and investors, government, and society) by the ESG criterion when making decisions on management and interaction with the company (Inampudi and Macpherson, 2020). For this purpose, corporate reporting is used, reports on sustainable development, reports on corporate social and environmental responsibility, financial reports, and ESG reports (Breedt et al., 2019). In the works of Fafaliou et al. (2022), Zhang et al. (2022), and Zhang et al. (2021), the scholars note a strong influence of ESG on companies in developed and developing countries: ESG determines the effectiveness of companies, their sustainability to economic crises, investment attractiveness, and strategic perspectives for the development of business.
In the age of AI, ESG practices are based on smart technology automatization means (robots, unmanned transport) under the control of AI, which are united in cyber-physical systems with the help of the Internet of Things (IoT). ESG investment in the age of AI acquires two specific features (Selim, 2020; Sætra, 2021). The first one is that ESG investments imply the financing of smart technologies which systemically improve the environmental, social, and financial characteristics of business activities (Minkkinen et al., 2022a). The second one is that ESG investment is performed based on smart technologies, for example, blockchain (Alkaraan et al., 2022).
The AI era opens up wide opportunities for the development of ESG investments thanks to technological support for improving decision making by all stakeholders (Teichmann et al., 2022). Investors get access to “smart” analytics of investment projects grouped, sorted, and ranked according to the criterion of the degree of compliance with ESG principles, contribution to the implementation of the SDGs, and the correlation between risk and profitability (Aroul et al., 2022; Popkova et al., 2020; Popkova and Sergi, 2022). A business can establish stable AI communications with the external environment, attracting a larger volume of ESG investments and achieving greater payback (Shahzad et al., 2020). Intelligent and automated state–public monitoring of ESG investments is also becoming available to identify and encourage the most responsible market agents (Andersson et al., 2022).
The established approach to the development of ESG investments in the AI era is focused on technology, and therefore it can be called technological. This approach is described in the works of Ielasi et al. (2020), Tong et al. (2022), and Yasmine and Kooli (2022) and involves stimulating scientific and technological progress for AI support of ESG investments. The disadvantage of the existing approach is that it does not take into account the possibility of using advanced “smart” technologies in practice, and the degree of use of their potential.
As practice shows, the availability of advanced technologies is not enough for their application. Based on the technological approach, the works of Li et al. (2022), Minkkinen et al. (2022b), Abdur Rehman Khan et al. (2022), and Sætra (2021) indicate the feasibility of developing “smart” technologies to increase the volume of ESG investments. Focusing on the existing concept of “smart” ESG investments, the AI economy is progressing, but despite the similar level of development of advanced “smart” technologies, there are serious differences in the intensity of automation of ESG investments in developed and developing countries (Jonsdottir et al., 2022). The available publications (Chen et al., 2022; Sharma et al., 2022) do not explain these differences, which is a gap in the literature. The set of conditions necessary and sufficient for the development of the AI economy is more fully reflected in UNCTAD (2022), which highlights the following:
− ICT ranking, showing the level of development, availability, and quality of advanced telecommunications infrastructure required for the application of “smart” technologies
− Skills ranking, demonstrating the availability of highly qualified personnel with digital competencies necessary for the use of “smart” technologies
− R and D ranking, characterizing the direct accessibility of “smart” technologies and the degree of their innovation
− Industry ranking, revealing the degree of high-tech production and international trade
− Finance ranking, demonstrating the adequacy of funding allocations to “smart” technologies and flexibility of financial instruments to achieve this goal
Systematic consideration of the abovementioned conditions reveals the AI economy in a new light–from the standpoint of social institutes. Since it is the differences in institutional support that form the basis for categorizing the countries of the world with the division of developed and developing countries, this article, based on the works of Shkalenko et al. (2022), Yankovskaya et al. (2021), hypothesizes that the AI economy institutes to determine the features of ESG investments in developed and developing countries.
The purpose of this article is to identify prospects and offer recommendations for the development of ESG investing in the AI era, taking into account the characteristics of developed and developing countries. To achieve this goal, the following tasks are set and solved:
− To identify the features of the impact of digital technologies of the AI era on the existing ESG investing practices in developed and developing countries
− To identify the prospects for the development of ESG investing in the AI era, as well as to offer authors’ recommendations, separately for developed and developing countries
A theory for the relationship between ESG and artificial intelligence: A literature review and gap analysis
The central scientific category of this article is the age of artificial intelligence (AI). It is treated as a new, modern stage of the development of society and economy, in which smart technologies, which are based on AI, are widely applied in practice (Kukushkina et al., 2022; Ragulina et al., 2022).
The age of AI began due to the fourth Industrial Revolution, the essential difference of which from previous industrial revolutions is the systemic coverage of technological modernization (Wilson et al., 2022). While in the past, industrial revolutions improved only production technologies, now–under the conditions of the fourth Industrial Revolution–management technologies are also improved. AI ensures the intellectual support for decision making, in particular investment decisions (Luitse and Denkena, 2021; Som, 2021).
This article is based on the theory of the relationship between ESG and artificial intelligence (AI). ESG performance is the evaluation of the company’s effectiveness from the position of sustainable development with the systemic coverage of environmental (E: with the focus on corporate environmental responsibility), social (S: with the focus on corporate social responsibility) effectiveness, and effectiveness of corporate governance (G: with the focus on profitability) (Avramov et al., 2022; Pedersen et al., 2021). ESG seriously influences companies in developed and developing countries (Cardillo et al., 2022; Chen et al., 2022; Lööf et al., 2022).
Fritz-Morgenthal et al. (2022), Minkkinen et al. (2022b), and Nauck (2019) point out in their works that AI allows (based on the Internet of Things, IoT) to collecting and analyzing Big Data on the topic of green innovation and corporate social and environmental responsibility. This data can be used as a basis for drawing much more complete and reliable internal and external corporate sustainability reporting (Maas, 2018). In addition, smart reporting on the implementation of ESG investment projects can be drawn (Vetrò et al., 2019).
Viriato (2019) and Wang et al. (2021) in their works hold that AI allows investors to make the most justified (coordinated) decisions on the location of ESG investments thanks to automated market analysis and intelligent support for decision making. In particular, corporate reporting can be processed by AI at a rapid pace and serve as a milestone for investment decisions (Cornforth, 2018). Furthermore, AI allows for building up the most efficient investment portfolios with a consistent risk-return ratio, as well as the ratio of economic, social, and environmental efficiency of capital investment projects (Auer and Schuhmacher, 2016; In et al., 2019).
Thus, the literature review has shown that both from the standpoint of demand (ESG investors) and the standpoint of supply (companies implementing ESG investment projects), AI contributes to benchmarking and ensures the balance of ESG investment markets. On the other hand, the gap analysis has revealed somewhat poor elaboration of the causal relationships of the development of ESG investments in the AI era, which is a gap in the literature. Since market patterns have their specific character in developed and developing countries, the causal relationships of the development of ESG investments in the AI era are studied and modeled individually in these categories of countries to fill the identified gap in this article.
Materials and methods
The initial point of this research is the following proposed hypothesis: institutes of the AI economy determine the specifics of ESG investment in developed and developing countries. The logic of the research consists of the following: at the qualitative level of the research, a potential connection between ESG analyses and principles of responsible AI is seen. This connection is manifested in the fact that responsible AI allows for high precision forecasting of the environmental consequences of the activities of companies and planning of the projects of corporate environmental responsibility (E).
Responsible AI ensures the growth of corporate social responsibility through smart monitoring of the safety of workplaces, remote execution of manipulations that are dangerous for employees’ health, creation of knowledge-intensive jobs, and expansion of opportunities for advanced training based on remote corporate training (S); responsible AI also stimulates the rationalization of the use of resources, optimization of all business processes, especially production and logistics, and increase in the scale effect and profitability of the business (G).
The methodology of testing the hypothesis is based on regression analysis, as a reliable method of economic statistics. The research is performed in two successive stages. In the first stage, we determine the specifics of the influence of digital technologies of the age of AI on the existing practices of ESG investing in developed and developing countries. For this, we perform the economic and mathematical modeling of the influence of the factors of Readiness for frontier technologies according to the UNCTAD (2022) on ESG score according to Morningstar (2021). The research model has the following form:
where ESG: ESG score, the rest: factors of readiness for frontier technologies; skl: skill ranking; r&d: R&D ranking; ind: industry ranking; fin: finance ranking; ict: ICT ranking.
To check the reliability of the econometric models, we use significance F and t-Stat. We formed a sample of developed countries (Denmark, Italy, the United States, Canada, Austria, Belgium, Australia, Norway, New Zealand, and the Czech Republic) and a sample of developing countries (China, India, Colombia, Malaysia, Chile, Brazil, Indonesia, Qatar, Russia, and Saudi Arabia). The samples contain countries with the highest level of development of ESG investment, according to the ranking by Morningstar (2021).
In the second stage, we determine the prospects and offer recommendations for the development of ESG investment in the age of AI in developed and developing countries. For this, we use the least squares method based on the obtained regression models. We also use the method of SWOT analysis to perform a quantitative-qualitative study of strengths and weaknesses, opportunities, and threats to the development of ESG investment in the age of AI in developed and developing countries.
Features of the impact of digital technologies of the AI era on the existing ESG investing practices in developed and developing countries
To determine the specifics of the development of ESG investments in the AI era in developed and developing countries (to test the hypothesis put forward in the article), an empirical study based on the available official statistics of Morningstar (2021), reflects the level of development of ESG investments in various countries was conducted. Readiness for frontier technologies is also taken into account, reflecting the quantitative measurement of the level of development of the institutes of the AI economy. The study was conducted based on 10 developed and 10 developing countries from different parts of the world with the highest level of development of ESG investment in 2021 (Table 1).
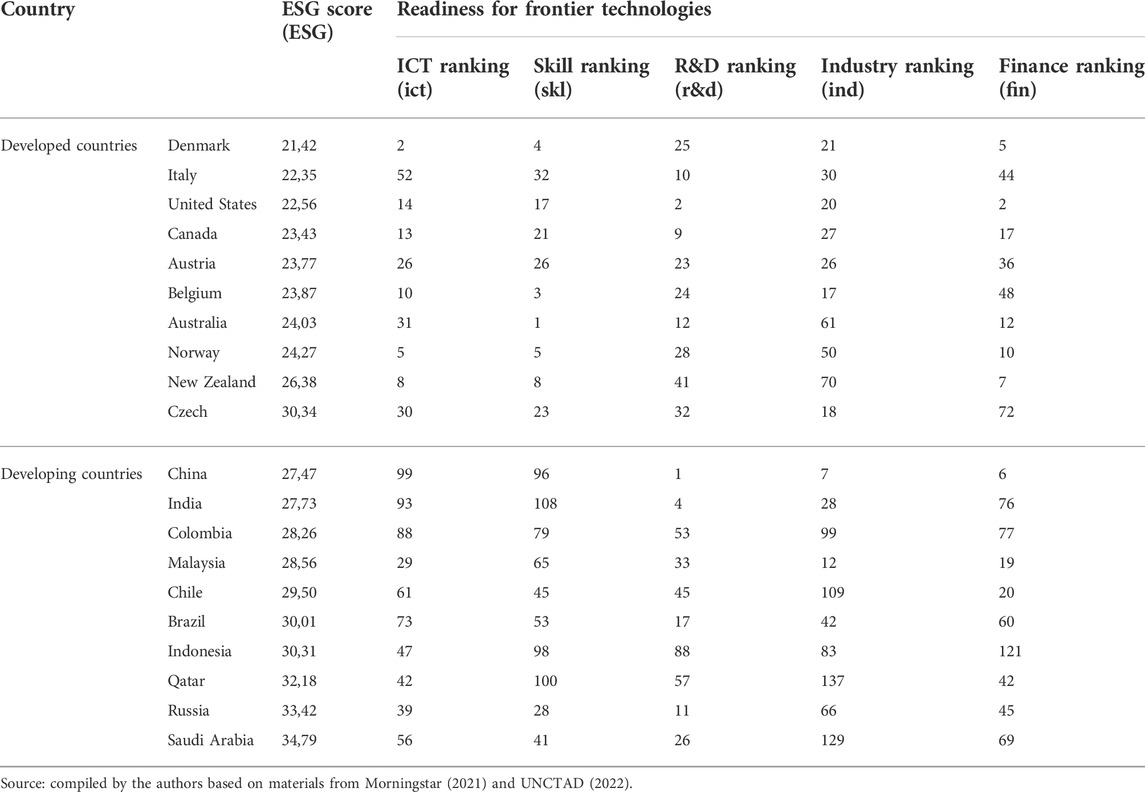
TABLE 1. ESG investments and readiness for frontier technologies in developed and developing countries in 2021.
The benefit of the ranking of developing and developed countries shown in Table 1 is that it has revealed a significant discrepancy between the existing boundaries of these categories of countries based on the criterion of market freedom and the efficiency of institutes (the integration of countries into the OECD defines them as developed countries) and on the criterion of income level (according to the World Bank classification, countries with a high level of income are considered as developed countries, while all other countries are considered as developing countries) with the criteria of ESG investments and readiness for frontier technologies.
For example, China is one of the most active users of artificial intelligence (AI) on the international scale, although it ranks among the developing countries. On the other hand, Austria is classified as a developed country by the OECD and the World Bank, while Table 1 shows that this country is classified as a country that is lagging behind in many respects. Standard boundaries of categories of countries have been stored in Table 1 to make the results comprehensible, easily interpretable, reproducible, and comparable with other studies on the topic of distinctions between developed and developing countries.
As a result of processing the data from Table 1 using regression and correlation analysis methods, the following two economic and mathematical models of the contribution of the institutes of the AI economy to the development of ESG investments were obtained:
− Model for developed countries: ESG = 18.91 – 0.12ict + 0.07skl-0.02r&d + 0.11ind + 0.13fin. The resulting model means that in developed countries, the development of ESG investments is positively influenced by such institutes of the AI economy as ICT and R&D. The cumulative correlation of ESG investments with the institutes of the AI economy is estimated at 83.86% (high). Because of the mentioned inconsistency of the sample of developed countries (reduced volume of ESG investments and readiness for frontier technologies despite the high-income level, high market freedom, and high efficiency of institutes), the model for developed countries is only reliable at a significance level of 0.3 (Significance F = 0 .27746), although the standard error is relatively small and is equal to 2.06. The t-statistics for the factor variables were as follows: for ICT ranking (ict): −1.04, for Skills ranking (skl): 0.73, for R&D ranking (r&d): −0.16, for Industry ranking (ind): 1.38, for Finance ranking (fin): 1.76;
− Model for developing countries: ESG = 32.86–0.07ict-0.01skl-0.07r&d + 0.04ind + 0.03fin. The resulting model means that in developed countries, the development of ESG investments is positively influenced by such institutes of the AI economy as ICT, Skills, and R&D. The cumulative correlation of ESG investments with the institutes of the AI economy is estimated at 94.56% (very high). The model for developing countries proved to be more reliable–it is reliable at a significance level of 0.05 (Significance F = 0.0440), and the standard error is small and equals 1.22. The t-statistics for the factor variables were as follows: for ICT ranking (ict): −2.91, for Skills ranking (skl): −0.32, for R&D ranking (r&d): −2.73, for Industry ranking (ind): 4.49, for Finance ranking (fin): 1.91.
Prospects and recommendations for the development of ESG investments in the AI era in developed and developing countries
To determine the prospects for the development of ESG investments in the AI era in developed and developing countries, based on the obtained economic and mathematical models, it was found that in developed countries, due to the progress of ICT institutes (+94.76% compared to the level of 2021) and R&D (+95.15%), the level of development of ESG investments may increase up to 26.80 points, that is, by 10.55% compared to 2021 (24.24 points).
To clarify the quantitative results, they were supplemented with a qualitative study using the SWOT analysis method, as a result of which (based on statistics from Table. 1) it was revealed that the strengths (S) of the AI economy in developed countries are the high level of development of such institutes as ICT (on average, in the sample of developed countries, 19.10 position) and R&D (20.60 positions).
The weaknesses (W) are the small contribution of skills to the development of ESG investments, despite the high level of development of this institute of the AI economy (14th place), as well as the moderate level of development and a small contribution to the development of ESG investments of such institutes as Industry (34th position) and Finance (25.30 positions). Opportunities (O) are associated with the further development of ICT and R&D institutes, as well as with the transformation of skills, industry, and finance institutes towards greater support for the SDGs and achieving their more significant contribution to the development of ESG investments. Threats (T) consist of the slow pace of development of ICT and R&D institutes as well as difficulties in the transformation of the Skills institute.
In developing countries, due to the progress of ICT institutes (+98.41%), Skills (+98.60%) and R&D (+97.01%), the level of development of ESG investment may increase up to 37.05 points, that is, by 22.57% compared to 2021 (30.22 points). The SWOT analysis showed that the strength (S) of the AI economy in developing countries is a moderate level of development of R&D institute (33.50 positions).
Weaknesses (W) is the low level of development of such institutes as ICT (62.70 positions) and Skills (71.30 positions), as well as a small contribution to the development of ESG investments in Industry and Finance institutes. Opportunities (O) are associated with the further development of R&D institutes, with accelerated progress in the development of ICT and Skills institutes, as well as with the transformation of Industry and Finance institutes towards greater support for the SDGs and achieving their more significant contribution to the development of ESG investments. Threats (T) consist in the slow pace of development of the R&D institute, as well as in difficulties in the transformation of the finance institute.
Discussion
The article contributed to the development of the concept of “smart” ESG investments by clarifying the causal relationships of the development of ESG investments in the AI economy. In contrast to Ielasi et al. (2020), Tong et al. (2022); Yasmine and Kooli (2022), it has been proved that the contribution of the AI economy to the development of ESG investments is related not so much to institutes but technologies. This allows us to propose a new (alternative) approach to the development of ESG investments in the AI era, involving the systematic development of institutes of the AI economy.
However, standard institutes (freedom of international trade, protection of investors, common “rules of the game” in industry markets) are not enough. Special institutes are required and are coming to the fore to ensure the movement of ESG investment flows in the AI era. Corporate social and environmental responsibility, green finance, corporate management, etc. serve as these special institutes. This is because ESG investments in the AI era, despite the traditional leadership of developed countries in most rankings, are more pronounced in developing countries.
It is suggested that a new classification of countries, in which the boundaries of developed and developing countries will be determined with due account for the level of development of the mentioned institutes of ESG investments in the AI era, could serve as a basis for the proposed approach. This will provide a means for a more reliable definition of the positions of countries in the world economic system from the standpoint of environmental economics and management, as well as for a more objective and accurate assessment of their progress in the development of ESG investments in the AI era.
The advantage of the new approach is, first, that it more accurately and reliably describes the regularities of development of ESG investments in the AI era. Second, the new approach explains the differences in the development of ESG investments in the AI economy of developed and developing countries, and also allows us to find unique application solutions for them, taking into account their specifics.
Also, as a result of the study, unlike Li et al. (2022), Minkkinen et al. (2022b), Rehman Khan et al. (2022); Sætra (2021), it has been proved that the development of “smart” technologies alone are not enough to increase the volume of ESG investments - the development of institutes of the AI economy is also required. This served as an argument for a clear division of the AI economies of developed and developing countries, whose institutes contribute differently (among institutes and categories of countries) to the development of ESG investments.
Conclusion
The result of the study is proof of the hypothesis put forward in the article. A review of international experience in 2021 showed that the institutes of the AI economy determine the features of ESG investments in developed (where ICT and R&D institutes are the most significant and highly developed) and developing countries (where ICT, Skills, and R&D institutes are the most significant, but only R&D institute is moderately developed). The obtained models, reasonable prospects, and proposed recommendations outlined the priorities for the development of institutes in the AI economy for the most effective support for ESG investments, taking into account the characteristics of each category of countries.
The contribution of the article to the improvement of scientific knowledge consists of the fact that the article has provided a new basis for the classification of countries, which enables a more exact definition of their modern boundaries in the AI era. It is suggested that the level of development and institutes of ESG investments could serve as this new basis. The article has made its contribution to the literature through the development of the theory for the relationship between ESG and artificial intelligence (AI), showing that this relationship can be observed at the level of institutes, not technologies, as in the previous opinion.
The theoretical significance of the results obtained in the article is related to the fact that the proposed new institutional approach to the development of ESG investments in the AI era takes into account the possibilities of using advanced “smart” technologies in practice, as well as the degree of use of their potential, and therefore bridges the gap between theory and practice. The applied significance of the authors’ conclusions and recommendations is that they take into consideration the characteristics of developed and developing countries and allow for the most effective management of the AI economy (through the development of its target institutes proposed in the article) in support of the development of ESG investments.
Recommendation and solution
As a prospective solution to the problem of the development of ESG investing in the age of AI, we propose a transition from the isolated development of technologies to the systemic development of institutes. Due to this, the process of development of ESG investing in the age of AI transforms from linear into cyclical, for institutes in society and the economy initiate the creation and implementation of new technologies, which, in its turn, strengthens the institutes and causes repetition of the cycle.
In developed countries, for the development of ESG investment in the age of AI, it is recommended to focus on the development of the institute of ICT and institute of R&D, and in developing countries–also the institute of Skills. That is, technologies play an important role, and for this, it is recommended to improve the telecommunication infrastructure and disseminate ICT. However, the progress of technologies only is not enough in both categories of countries.
A mandatory condition for the development of ESG investment in the age of AI is the growth of innovative activity in the economy. For this, it is recommended to increase the volume of financing of R&D and the share of science-intensive, high-tech, and innovative products. In developing countries, an important role belongs to social adaptation. For this, it is recommended to fill in the gaps in competencies, train digital personnel, create knowledge-intensive jobs and implement corporate training.
The advantage of the proposed solution is the comprehensive development of society, economy, and technologies, as well as consideration of the specifics and offering of the authors’ applied recommendations for developed and developing countries. This will allow reaching the mass character of ESG investing and an increase in scale in the age of AI, as well as ensure the reliable support for the implementation of the SDGs in the Decade of Action from society and business based on the technologies of the age of AI and ESG investing.
Economic policy implications
The results obtained allow concluding that the models of development of ESG investment in the age of AI are different in developed and developing countries. Based on the compiled econometric models and results of the SWOT analysis, the following recommendations for economic policy are offered for developed countries: 1) supporting the achieved high level of development of the institute of ICT and the institute of R&D through the improvement of the legal regulation of these institutes; 2) stimulating the growth of the contribution of skills to ESG investments through the development of the “knowledge economy”; 3) raising the level of the development of the institutes of industry and finance and increasing their contribution to ESG investments through the stimulation of support for the SDGs.
The following recommendations for economic policy are offered for developing countries: 1) supporting the achieved high level of the development of the institute of R&D through further development of the innovative economy; 2) raising the level of the development of the institutes of ICT and skills through accelerated digital modernization of society and economy; 3) stimulating the growth of the contribution to ESG investments by the institutes of industry and finance through stimulating corporate social responsibility. The proposed recommendations allow focusing the national economic policy on the key spheres, thus facilitating the increase in its effectiveness.
Limitations and prospects for future research
Summing up the research, it should be noted that the institutional approach to studying the influence of the AI economy on ESG investments, which is offered and used in this article, has demonstrated its high effectiveness. The advantage of the institutional approach is the possibility to combine qualitative and quantitative research methods, as well as to take into account the specifics of developed and developing countries.
A limitation of this research is the generalized consideration of developed and developing countries at the level of categories, while in each country, institutes of the AI economy are specific, similar to the practices of ESG investment. To deal with this limitation, it is recommended to perform a range of case studies, to identify the national models of development of ESG investment based on the institutes of the AI economy and with the use of the institutional approach.
Author contributions
All authors listed have made a substantial, direct, and intellectual contribution to the work and approved it for publication.
Conflict of interest
The authors declare that the research was conducted in the absence of any commercial or financial relationships that could be construed as a potential conflict of interest.
Publisher’s note
All claims expressed in this article are solely those of the authors and do not necessarily represent those of their affiliated organizations, or those of the publisher, the editors, and the reviewers. Any product that may be evaluated in this article, or claim that may be made by its manufacturer, is not guaranteed or endorsed by the publisher.
References
Aldowaish, A., Kokuryo, J., Almazyad, O., and Goi, H. C. (2022). Environmental, social, and governance integration into the business model: Literature review and research agenda. Sustainability 14, 2959. doi:10.3390/su14052959
Alkaraan, F., Albitar, K., Hussainey, K., and Venkatesh, V. G. (2022). Corporate transformation toward Industry 4.0 and financial performance: The influence of environmental, social, and governance (ESG). Technol. Forecast. Soc. Change 175, 121423. doi:10.1016/j.techfore.2021.121423
Andersson, E., Hoque, M., Rahman, M. L., Uddin, G. S., and Jayasekera, R. (2022). ESG investment: What do we learn from its interaction with stock, currency and commodity markets? Int. J. Fin. Econ. 27 (3), 3623–3639. doi:10.1002/ijfe.2341
Aroul, R. R., Sabherwal, S., and Villupuram, S. V. (2022). ESG, operational efficiency and operational performance: evidence from real estate investment trusts. Manag. Finance 48 (8), 1206–1220. doi:10.1108/MF-12-2021-0593
Auer, B. R., and Schuhmacher, F. (2016). Do socially (ir)responsible investments pay? New evidence from international ESG data. Q. Rev. Econ. Finance 59, 51–62. doi:10.1016/j.qref.2015.07.002
Avramov, D., Cheng, S., Lioui, A., and Tarelli, A. (2022). Sustainable investing with ESG rating uncertainty. J. Financial Econ. 145 (2), 642–664. doi:10.1016/j.jfineco.2021.09.009
Breedt, A., Ciliberti, S., Gualdi, S., and Seager, P. H. (2019). Is ESG an equity factor or just an investment guide? J. Invest. 28 (2), 32–42. doi:10.3905/joi.2019.28.2.032
Cardillo, G., Bendinelli, E., and Torluccio, G. (2022). COVID-19, ESG investing, and the resilience of more sustainable stocks: Evidence from European firms. Bus. Strategy Environ., 10.1002/bse.3163. doi:10.1002/bse.3163
Chen, G., Wei, B., and Dai, L. (2022). Can ESG-responsible investing attract sovereign wealth funds’ investments? Evidence from Chinese listed firms. Front. Environ. Sci. 10, 935466. doi:10.3389/fenvs.2022.935466
Dudukalov, E. V., Terenina, I. V., Perova, M. V., and Ushakov, D. (2021). Industry 4.0 readiness: The impact of digital transformation on supply chain performance. E3S Web Conf. 244, 08020. doi:10.1051/e3sconf/202124408020
Fafaliou, I., Giaka, M., Konstantios, D., and Polemis, M. (2022). Firms’ ESG reputational risk and market longevity: A firm-level analysis for the United States. J. Bus. Res. 149, 161–177. doi:10.1016/j.jbusres.2022.05.010
Fritz-Morgenthal, S., Hein, B., and Papenbrock, J. (2022). Financial risk management and explainable, trustworthy, responsible AI. Front. Artif. Intell. 5, 779799. doi:10.3389/frai.2022.779799
Gao, Y., Li, Y., and Wang, Y. (2021). The dynamic interaction between investor attention and green security market: an empirical study based on baidu index. China Finance Rev. Int. doi:10.1108/CFRI-06-2021-0136
Ge, G., Xiao, X., Li, Z., and Dai, Q. (2022). Does ESG performance promote high-quality development of enterprises in China? The mediating role of innovation input. Sustainability 14, 3843. doi:10.3390/su14073843
Ielasi, F., Ceccherini, P., and Zito, P. (2020). Integrating ESG analysis into smart beta strategies. Sustain. Switz. 12 (22), 93511–93522. doi:10.3390/su12229351
In, S. Y., Rook, D., and Monk, A. (2019). Integrating alternative data (also known as ESG data) in investment decision making. Glob. Econ. Rev. 48 (3), 237–260. doi:10.1080/1226508X.2019.1643059
Inampudi, K., and Macpherson, M. (2020). “The impact of AI on environmental, social and governance (ESG) investing: Implications for the investment value chain,” in The artificial intelligence handbook for investors, entrepreneurs and FinTech visionaries. Editors S. Chishti, I. Bartoletti, A. Leslie, and M. M. Shân (New Jersey: John Wiley & Sons). doi:10.1002/9781119551966.ch35
Ivanov, A. A., Ivanov, A. A., and Ivashchenko, Y. S. (2022). Digital technologies of the self: Instrumental rationality or creative integrity? Lect. Notes Netw. Syst. 345, 139–147.
Jonsdottir, B., Sigurjonsson, T. O., Johannsdottir, L., and Wendt, S. (2022). Barriers to using ESG data for investment decisions. Sustain. Switz. 14 (9), 5157. doi:10.3390/su14095157
Kukushkina, A. V., Mursaliev, A. O., Krupnov, Y. A., and Alekseev, A. N. (2022). Environmental competitiveness of the economy: Opportunities for its improvement with the help of AI. Front. Environ. Sci. 10, 953111. doi:10.3389/fenvs.2022.953111
Li, S., Yu, Y., Jahanger, A., Usman, M., and Ning, Y. (2022). The impact of green investment, technological innovation, and globalization on CO2 emissions: Evidence from MINT countries. Front. Environ. Sci. 10, 868704. doi:10.3389/fenvs.2022.868704
Lööf, H., Sahamkhadam, M., and Stephan, A. (2022). Is corporate social responsibility investing a free lunch? The relationship between ESG, tail risk, and upside potential of stocks before and during the COVID-19 crisis. Finance Res. Lett. 46, 102499. doi:10.1016/j.frl.2021.102499
Luitse, D., and Denkena, W. (2021). The great transformer: Examining the role of large language models in the political economy of AI. Big Data Soc. 8 (2), 205395172110477. doi:10.1177/20539517211047734
Maas, M. M. (2018). “Regulating for 'normal AI accidents': Operational lessons for the responsible governance of artificial intelligence deployment,” in AIES - Proceedings of the 2018 AAAI/ACM Conference on AI, Ethics, and Society, New Orleans La, USA, Feb 2-3, 223–228.
Minkkinen, M., Niukkanen, A., and Mäntymäki, M. (2022a). What about investors? ESG analyses as tools for ethics-based AI auditing. AI Soc. doi:10.1007/s00146-022-01415-0
Minkkinen, M., Zimmer, M. P., and Mäntymäki, M. (2022b). Co-shaping an ecosystem for responsible AI: Five types of expectation work in response to a technological frame. Inf. Syst. Front. doi:10.1007/s10796-022-10269-2
Morningstar (2021). Sustainability atlas assesses the ESG profile of countries across the globe. URL: https://www.morningstar.co.uk/uk/news/211424/which-countries-lead-on-esg.aspx (data accessed: 13.05.2022).
Pedersen, L. H., Fitzgibbons, S., and Pomorski, L. (2021). Responsible investing: The ESG-efficient frontier. J. Financial Econ. 142 (2), 572–597. doi:10.1016/j.jfineco.2020.11.001
Popkova, E. (2022). Advanced issues in the green economy and sustainable development in emerging market economies (elements in the economics of emerging markets). Cambridge: Cambridge University Press.
Popkova, E., Alekseev, A. N., Lobova, S. V., and Sergi, B. S. (2020). The theory of innovation and innovative development. AI scenarios in Russia. Technol. Soc. 63, 101390. doi:10.1016/j.techsoc.2020.101390
Popkova, E. G., Oudah, A.-M. M. Y., Ermolina, L. V., and Sergi, B. S. (2021). Financing sustainable development amid the crisis of 2020. A research note. Lect. Notes Netw. Syst. 198, 773–780. doi:10.1007/978-3-030-69415-9_88
Popkova, E. G., and Sergi, B. S. (2021). Dataset modelling of the financial risk management of social entrepreneurship in emerging economies. Risks 9 (12), 211. doi:10.3390/risks9120211
Popkova, E. G., and Sergi, B. S. (2022). High-tech economic growth from the standpoint of the theory of economic time: Modelling and reducing space–time inequality. Smart Innovation, Syst. Technol. 287, 15–22. doi:10.1007/978-981-16-9804-0_2
Ragulina, Y. V., Dubova, Y. I., Litvinova, T. N., and Balashova, N. N. (2022). The environmental AI economy and its contribution to decarbonization and waste reduction. Front. Environ. Sci. 10, 914003. doi:10.3389/fenvs.2022.914003
Rehman, F. U., and Noman, A. A. (2022). China's outward foreign direct investment and bilateral export sophistication: a cross countries panel data analysis. China Finance Rev. Int. 12 (1), 180–197. doi:10.1108/cfri-04-2020-0040
Rehman Khan, S. A., Hassan, S., Khan, M. A., Godil, D. I., and Tanveer, M. (2022). Nexuses between energy efficiency, renewable energy consumption, foreign direct investment, energy consumption, global trade, logistics and manufacturing industries of emerging economies: In the era of COVID-19 pandemic. Front. Environ. Sci. 10, 880200. doi:10.3389/fenvs.2022.880200
Sætra, H. S. (2021). A framework for evaluating and disclosing the esg related impacts of ai with the SDGs. Sustain. Switz. 13 (15), 8503. doi:10.3390/su13158503
Selim, O. (2020). “ESG and AI: The beauty and the beast of sustainable investing,” in Sustainable investing: A path to a new horizon. Editors Herman Bril, Georg Kell, and Andreas Rasche (England: Routledge), 227–243. doi:10.4324/9780429351044-12
Shahzad, F., Hussain Baig, M., Rehman, I. U., Latif, F., and Sergi, B. S. (2020). What drives the impact of women directors on firm performance? Evidence from intellectual capital efficiency of US listed firms. J. Intellect. Cap. 21 (4), 513–530. doi:10.1108/JIC-09-2019-0222
Sharma, U., Gupta, A., and Gupta, S. K. (2022). The pertinence of incorporating ESG ratings to make investment decisions: a quantitative analysis using machine learning. J. Sustain. Finance Invest., 1–15. doi:10.1080/20430795.2021.2013151
Shkalenko, A. V., Inshakova, A. O., and Inshakova, E. I. (2022). “The digital economy in action: Integration of institutional economy paradigms and law in the neo-industrialization period,” in Advances in research on Russian business and management (North Carolina: Information Age Publishing), 99–116.
Som, T. (2021). Sustainability in energy economy and environment: Role of AI based techniques. Model. Optim. Sci. Technol. 18, 647–682. doi:10.1007/978-3-030-72929-5_31
Teichmann, F., Boticiu, S. R., and Sergi, B. S. (2022). Compliance risks for crowdfunding. A neglected aspect of money laundering, terrorist financing and fraud. J. Financ. Crime. doi:10.1108/JFC-05-2022-0116
Tong, L., Yan, W., and Manta, O. (2022). Artificial intelligence influences intelligent automation in tourism: A mediating role of Internet of Things and environmental, social, and governance investment. Front. Environ. Sci. 10, 853302. doi:10.3389/fenvs.2022.853302
UNCTAD (2022). Technology and innovation report 2021. URL: https://unctad.org/page/technology-and-innovation-report-2021 (data accessed: 13.05.2022).
Vetrò, A., Santangelo, A., Beretta, E., and De Martin, J. C. (2019). AI: from rational agents to socially responsible agents. Digital Policy, Regul. Gov. 21 (3), 291–304. doi:10.1108/DPRG-08-2018-0049
Viriato, J. C. (2019). AI and machine learning in real estate investment. J. Portfolio Manag. 45 (7), 43–54. doi:10.3905/jpm.2019.45.7.043
Wang, X., Butt, A. H., Zhang, Q., Ahmad, H., and Shafique, M. N. (2021). Intention to use AI-powered financial investment robo-advisors in the M-banking sector of Pakistan. Inf. Resour. Manag. J. 34 (4), 1–27. doi:10.4018/IRMJ.2021100101
Wilson, M., Paschen, J., and Pitt, L. (2022). The circular economy meets artificial intelligence (AI): understanding the opportunities of AI for reverse logistics. Manag. Environ. Qual. Int. J. 33 (1), 9–25. doi:10.1108/MEQ-10-2020-0222
Yankovskaya, V. V., Osipov, V. S., Zeldner, A. G., Panova, T. V., and Mishchenko, V. V. (2021). Institutional matrix of social management in region's economy: stability and sustainability vs innovations and digitalization. Int. J. Sociol. Soc. Policy 41 (1-2), 178–191. doi:10.1108/IJSSP-03-2020-0088
Yasmine, B., and Kooli, M. (2022). Smart beta ESG disclosure. J. Asset Manag. doi:10.1057/s41260-022-00257-1
Zhang, D., Zhao, Z., and Lau, C. K. M. (2022). Sovereign ESG and corporate investment: New insights from the United Kingdom. Technol. Forecast. Soc. Change 183, 121899. doi:10.1016/j.techfore.2022.121899
Keywords: ESG investing, AI era, developed countries, developing countries, institutions, smart technologies, automation, AI economy
Citation: Khoruzhy LI, Semenov AV, Averin AV and Mustafin TA (2022) ESG investing in the AI era: Features of developed and developing countries. Front. Environ. Sci. 10:951646. doi: 10.3389/fenvs.2022.951646
Received: 24 May 2022; Accepted: 15 August 2022;
Published: 03 October 2022.
Edited by:
Elena G. Popkova, Moscow State Institute of International Relations, RussiaReviewed by:
Aidarbek T. Giyazov, Batken State University, KyrgyzstanLadislav Zak, Prague, Czech Republic
Nibedita Saha, Tomas Bata University in Zlín, Czechia
Copyright © 2022 Khoruzhy, Semenov, Averin and Mustafin. This is an open-access article distributed under the terms of the Creative Commons Attribution License (CC BY). The use, distribution or reproduction in other forums is permitted, provided the original author(s) and the copyright owner(s) are credited and that the original publication in this journal is cited, in accordance with accepted academic practice. No use, distribution or reproduction is permitted which does not comply with these terms.
*Correspondence: Liudmila I. Khoruzhy, ZGthMTk1NUBtYWlsLnJ1