- 1Research Center for Environment and Health, Zhongnan University of Economics and Law, Wuhan, China
- 2School of Information and Safety Engineering, Zhongnan University of Economics and Law, Wuhan, China
1 Introduction
Globally, exposure to air pollution is estimated to cause millions of deaths annually as well as loss of healthy years of life (Li et al., 2021). PM2.5 pollution has become a worldwide challenge, especially in developing countries such as China. PM2.5 exposure not only affects the mortality rates of residents as well as outpatient and hospitalization rates for specific diseases but also increases cancer risk and death burden (Tan et al., 2018; Li et al., 2019). In addition, PM2.5 pollution can cause economic losses by increasing health-related expenditures. Hence, evaluating the urban PM2.5-related health economic losses across China is of significance for environmentally sustainable and equitable decision-making developments.
Various scholars have studied PM2.5-related health economic losses in China for different spatial scales. The average PM2.5-related economic loss was 0.3% (amended human capital: AHC) to 1% (value of statistical life: VSL) of the total gross domestic product (GDP) of 190 Chinese cities from 2014 to 2016 (Yang et al., 2018). In the Beijing–Tianjin–Hebei (BTH) region of China, the PM2.5 economic loss was 6,081 million USD in 2017 (Wang et al., 2020). Most of the previous studies on this topic were mainly targeted at limited and individual areas or cities, such as 190 major cities in China (Yang et al., 2018), the BTH region (Wang et al., 2020), and Shanghai (Wu et al., 2017). However, owing to the spatial spillover effects of PM2.5 and regional joint management requirements, it is important to systematically analyze the urban PM2.5-related health economic losses from the national–regional perspectives.
Owing to regional differences in the economic, social, and environmental perspectives, the factors driving PM2.5-related health economic losses in different regions need to be analyzed further. The geographically weighted regression (GWR) is often used to identify such factors because of its flexibility in identifying relationships between different geospatial influences. Fu et al. (2014) used the GWR to analyze the factors influencing PM2.5 health risks; the population, social, and economic factors accounted for about 45% of the PM2.5 health risks. It is therefore helpful to identify the factors driving urban PM2.5-related health economic losses for joint prevention and control at the regional level.
The major aims of this study were as follows: 1) to explore the spatiotemporal variations of PM2.5 in 287 Chinese cities and to identify critical study regions by spatial autocorrelation analysis; 2) to evaluate PM2.5-related health losses in the studied cities based on the monitored data and health loss model; 3) to calculate the PM2.5-related health economic losses according to the VSL; 4) to identify the socioeconomic drivers of PM2.5-related health economic losses based on GWR and to formulate management strategies for key regions.
2 Materials and methods
2.1 Data sources
The daily mean PM2.5 concentration data for 2015–2018 were collected from the website of the China National Environmental Monitoring Centre (CNEMC) (http://www.cnemc.cn/sssj/). Based on the requirements of the ambient air quality standards (GB3095-2012), monitoring sites with valid PM2.5 concentration data for less than 20 h a day, 27 days a month (25 days for February), or 324 days a year were eliminated. The missing data were identified from https://www.aqistudy.cn/historydata/. Because the monitoring data for Sansha and Taiwan were missing and because Lhasa, Urumqi, and Karamay were geographically far away from the other prefecture-level cities, a follow-up analysis of the prefecture-level cities in mainland China was conducted after excluding the abovementioned regions. Vector boundary data of the prefecture-level administrative divisions in China were obtained from the Center for Resources and Environmental Sciences of the Chinese Academy of Sciences (http://www.resdc.cn/). The population data, mortality data, as well as social and economic factors (population density, GDP, urbanization rate, proportion of secondary industry, green coverage rate of built-up areas, industrial sulfur dioxide emissions) were all obtained from the statistical yearbook.
2.2 Health loss model
Some scholars have defined health loss as premature deaths or hospitalizations caused by PM2.5 exposure (Li et al., 2020). PM2.5 health economic loss refers to monetizing the PM2.5 health loss via the willingness to pay (WTP), cost of illness (COI), or AHC method. Three steps were used to quantitatively assess the health impacts of PM2.5: 1) collecting and organizing PM2.5 concentration data from the study areas; 2) identifying the exposed population; 3) calculating the PM2.5-related health effects using the concentration-response coefficient obtained from epidemiological studies. The formula for calculating the health losses from the 287 cities exceeding Grade II standards (35 μg/m3) of the GB 3095-2012 is as follows (Xia, 2019):
where ∆Y (person) is the resulting premature deaths; Y0 (‰) is the baseline rate of all-cause deaths; β is the coefficient between the PM2.5 concentration and health effects (concentration-response coefficient); Pop (person) is the exposed population;
2.3 Health economic loss model
The common methods used to assess the economic impacts of controlling air pollution include the COI, AHC, and WTP approaches. The COI mainly evaluates the medical expenses and other costs incurred by diseases, and it is suitable for assessing the economic impacts caused by air quality changes in small and highly polluted areas (Jo, 2014). The traditional human capital approach emphasizes the damage caused by air pollutants to workers’ capital. The value of premature deaths of young, unemployed, and retired persons is very low, which has ethical and moral defects to a large extent (Qu et al., 2020). The WTP refers to the VSL of the samples calculated using the death risk intention survey value evaluation method. The advantage of the WTP approach is that it can be used to evaluate the use and non-use values of air pollution health losses (Yu et al., 2019). Compared with the three methods noted above, the VSL was selected owing to its flexibility and operability. A model that monetizes the health effects of changes in PM2.5 concentration is shown in the formula below (Ding et al., 2016):
where ∆Y (person) is the resulting premature deaths, VSL (ten thousand RMB/person) is the unit economic value of the health endpoint, and HBE (billion RMB) is the health economic loss. The calculation of the VSL is shown in the Supplementary Material.
2.4 Global autocorrelation
Global autocorrelation is a description of the spatial characteristics of properties throughout a region (Liu et al., 2018). Assuming that the entire study is divided into n units, where the value on the ith unit is xi and mean observed value is
where W is a spatial weight matrix, and
2.5 Local autocorrelation
To study the relativity of the local regions within the entire region, the concept of local autocorrelation is introduced. For unit i, the corresponding local Moran’s I can be defined as follows:
where Ii represents the local Moran’s I coefficient of position i.
2.6 Geographically weighted regression
The GWR is a local statistical method that inserts the spatial locations of the data into the regression parameters to explore the spatial variations between the explained and explanatory variables; it can effectively solve spatial nonstationarity (Duan et al., 2018). The structure of the model is as follows:
where
3 Results and discussion
3.1 Spatiotemporal variation of PM2.5 across China
The distribution of the PM2.5 AM in 287 Chinese cities for 2015–2018 is shown in Figure 1. From 2015 to 2018, the high concentration values were mainly observed in the BTH region and surrounding areas (BTHSA), Fenwei Plain (FWP), and middle reaches of the Yangtze River (MRYR). With the BTH region as the center, the distribution of PM2.5 AM gradually decreased toward the surrounding areas. The areas with low concentrations were mainly observed in the southwest and south regions of China. The PM2.5 AM in northeast China decreased each year, which was consistent with the general trend for the entire country. Overall, the air quality of the Pearl River Delta (PRD) was better than that of the Yangtze River Delta (YRD). The PM2.5 AM in the PRD met the GB 3095-2012 standard (35 µg/m3); however, from 2015 to 2017, Foshan and Zhaoqing exceeded the GB 3095-2012 value, whereas the value of Dongguan decreased first, increased next, and later decreased again. A few cities in the YRD met the GB 3095-2012 expectation, with three, four, four, and eight cities meeting the GB 3095-2012 from 2015 to 2018 in the YRD. Through the analysis of the spatiotemporal variations of the PM2.5 concentrations, we concluded that the PM2.5 pollution was gradually alleviated from 2015 to 2018 across China and that the number of areas with values below 35 μg/m3 continued to increase. The lowest PM2.5 AM was about 15 µg/m3. The area of high concentration decreased gradually, and the corresponding range of the PM2.5 AM also decreased.
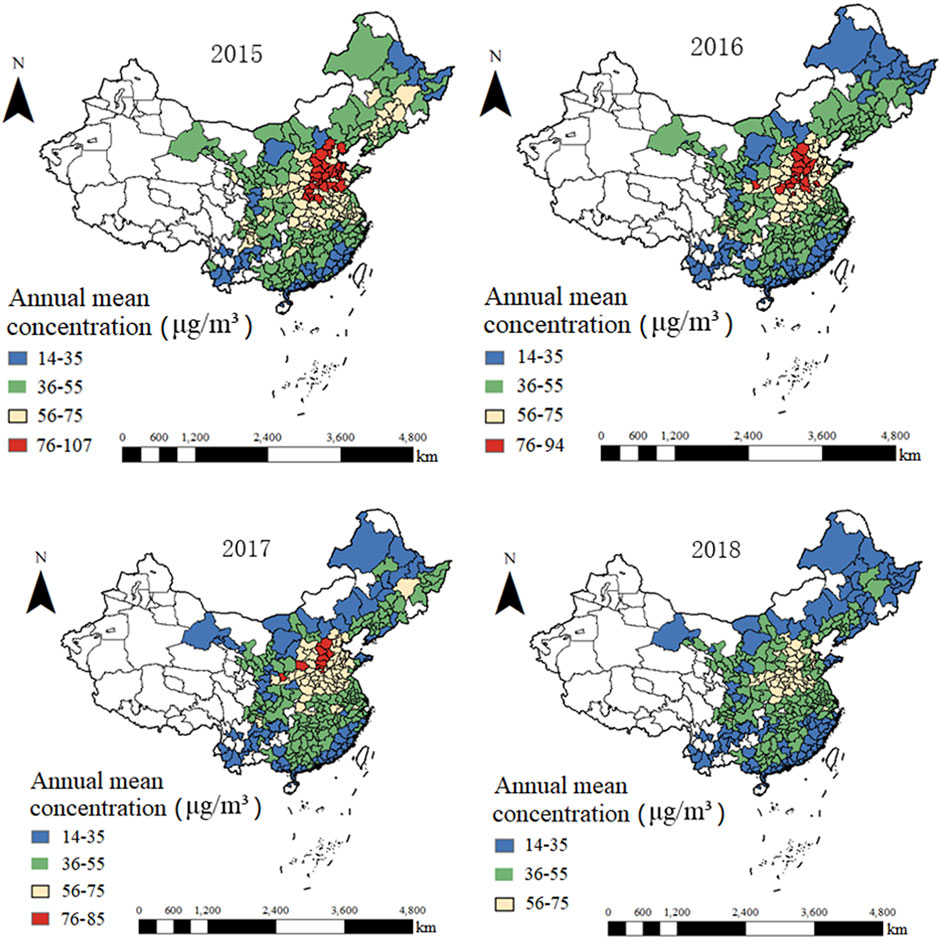
FIGURE 1. Spatiotemporal variation characteristics of the annual mean PM2.5 concentrations in China from 2015 to 2018.
3.2 Evaluating health losses
The results of the PM2.5 health loss assessment in China are shown in Supplementary Table S1. From 2015 to 2018, the number of premature deaths in cities with PM2.5 AM exceeding GB 3095-2012 decreased first, increased next, and decreased again, but the proportion of the total number of deaths decreased annually. The city with the maximum health loss for 2015–2018 was Beijing, the cities with the least health losses for 2015–2016 were Jinchang and Dingxi, and the city with the least health loss for 2017–2018 was Zhongwei.
3.3 Evaluating health economic losses using VSL
By monetizing the health loss results based on the VSL, the health economic loss results for China from 2015 to 2018 were 1,410.626, 1,179.159, 1,295.754, and 772.668 billion RMB (1 RMB = 0.1485 USD as on 18 July 2022), respectively, which account for 1.98%, 1.54%, 1.56%, and 0.85% of the GDPs for the corresponding years for the 287 cities. The assessment results of the PM2.5 health economic losses in key areas for air pollution prevention and control of the Blue Sky Protection Campaign are shown in Table 1. With the exception of the Chengdu-Chongqing (CC) region, the variation trend of the PM2.5 health economic loss was similar to the health losses in other key areas, with inconsistencies in the variation range. The PM2.5 health loss in the CC region decreased, but the health economic losses increased first and then decreased. Except for the PRD, the decline in PM2.5 health economic losses in other key regions were highest from 2017 to 2018, which was consistent with the period of greatest decline in health losses. The PM2.5 health economic losses in the PRD decreased sharply in 2015–2016 and 2017–2018 but increased by 1.7 times in 2017 compared with that in 2016. By comparing the evaluation results of the PM2.5 health and health economic losses, the changes in the PM2.5 health economic losses were influenced by not only the local PM2.5 AM and exposed population but also other factors and mechanisms that remain to be explored.
3.4 Spatial agglomeration effects
The PM2.5 AM, PM2.5-related health losses, and health economic losses in cities exceeding the standard of 35 µg/m3 showed spatial agglomeration effects in China from 2015 to 2018. The Moran’s I index values for the PM2.5 AM, health losses, and health economic losses all showed the increasing–decreasing–increasing trend. ArcGIS was used to test the local autocorrelation. The hotspots of PM2.5 AM from 2015 to 2018 were concentrated mainly in the BTHSA and FWP. In addition, Wuhan, Xiangyang, Jingmen, Xiaogan, and Suizhou of Hubei province showed high–high agglomerations in 2015. The cold spots of PM2.5 AM were concentrated mainly in southwest and south China, with low–low agglomerations in Zhaoqing, Liuzhou, Guilin, Wuzhou, Guigang, and Baiyin for 2015–2018. These cities had relatively lower PM2.5 AM and light pollution.
Areas with high PM2.5 health losses for 2015–2018 were concentrated mainly in the north of the Yangtze River. Although the number of cities with PM2.5 AM exceeding 35 μg/m3 decreased annually, the number of cities with high concentrations did not decrease significantly. The difference between the spatiotemporal variation of the PM2.5 health loss and PM2.5 AM may be related to the difference in the exposed population and baseline mortality rate. Areas with high PM2.5 health economic losses for 2015–2018 were concentrated mainly in the north of the Yangtze River, i.e., most cities in Hebei, Henan, and Shandong provinces. The differences among the spatiotemporal variations of the health economic losses, PM2.5 AM, and health losses may be relevant to the differences in the levels of economic development in these research units.
3.5 Analysis of factors driving health economic losses
As the number of cities with PM2.5 AM values exceeding 35 µg/m3 decreased gradually, the number of cities included from 2015 to 2018 decreased as 235, 214, 204, and 178, respectively. The missing values were mainly replaced with the mean values of the corresponding provinces under which the city is included. The stepwise regression method was used to eliminate linear correlations between variables (Yang et al., 2016). The independent variables included in this study were population density (Den_Pop), GDP, urbanization rate (Urban), proportion of secondary industry (Sec), and industrial sulfur dioxide emissions (SO2). Their VIF values were less than 10. Statistical descriptions of the variables used in this study are shown in Supplementary Tables S2–S5. The results of the GWR model are shown in Supplementary Table S6. Supplementary Figures S2–S4 show the distributions of the influences of various driving factors on health economic losses. The spatial distribution of the regression residuals is shown in Supplementary Figure S1; these residuals are randomly distributed in space, which indicates that the residuals follow a normal distribution. There are spatial differences in the impact degrees of each of the variables on PM2.5-related health economic losses. Because the regression coefficients of the GDP and SO2 were too small, empirical results only show the influences of Den_Pop, Urban, and Sec.
3.5.1 Proportion of secondary industry
Supplementary Figure S2 shows the spatial pattern of the influence of Sec on PM2.5-related health economic loss. The Sec values for BTHSA, YRD, and FWP were inversely proportional to the PM2.5-related health economic losses. The Sec value for PRD is directly proportional to the PM2.5-related health economic loss. For the remaining regions (CC and YRMR), the coefficient directions vary for each year. Thus, the empirical results of the dynamic changes in the different regions serve as a reminder to researchers and decision-makers to further explore the mechanisms of the impact of industrial structure on air pollution, population health, and economy.
3.5.2 Population density
Supplementary Figure S3 shows the spatial pattern of the impact of Den_pop on PM2.5-related health economic loss. From 2015 to 2018, the coefficients of Den_Pop for BTHSA, FWP, and CC were all positive, indicating that the increase in Den_Pop contributed to increase in PM2.5-related health economic losses. In the YRD, only Wenzhou, Quzhou, Chizhou, Jinhua, Anqing, and Hangzhou had negative coefficients for Den_Pop in 2017.
3.5.3 Urbanization rate
Supplementary Figure S4 shows the spatial pattern of the influence of Urban on PM2.5-related health economic loss. From 2015 to 2018, the coefficients of Urban for BTHSA and MRYR were negative. In the YRD, except for Shaoxing, Hangzhou, and Jiaxing, the coefficients for Urban were positive in 2018 and negative for the other years. In the FWP, the coefficient of Urban was negative for all years except in Xi’an, which was positive in 2017 and 2018. In 2015 and 2016, the coefficients of Urban for cities exceeding the 35 µg/m3 standard in CC were negative. The coefficient changes of Urban for the PRD were strongly similar to those of Den_Pop.
The following two anomalies were observed in this study and should be discussed further. First, the increase in the proportion of the secondary industry will reduce PM2.5-related health economic losses. Some studies have shown that the proportion of the secondary industry had a significant positive impact on the PM2.5-related health losses (Fu et al., 2014); this means that the increase in the proportion of the secondary industry will increase the PM2.5-related health economic losses, which is contrary to the results of this study. Therefore, the mechanisms of the impact of the industrial structure on air pollution and health economic loss need to be discussed further. Second, the strategy of “increasing the green coverage rates of built-up areas” has no direct impact on improving the PM2.5 health economic losses. Tong et al. (2015) showed that increasing the green coverage will reduce PM2.5 concentration and improve health benefits. However, in this study, the green coverage rates of the built-up areas did not directly affect the PM2.5-related health economic losses. Hence, it is necessary to conduct in-depth analysis of the influence of the green coverage rates of built-up areas on the health economic levels of the residents in the future.
4 Policies
According to the results of this study, the status quo of PM2.5 control and air pollution control policies in China were combined to propose PM2.5 control suggestions for different regions. In addition to improving the PM2.5 health economic loss assessment system, the following key control areas were screened out according to the geographical environment, economic development level, and other factors. Some of the specific governance suggestions are as follows: For the BTHSA and Fenwei Plain, the urbanization rate can be improved to reduce the health economic losses. The YRD region requires attention on the construction of a regional joint prevention and control system. The CC region needs a balanced relationship between population density and urbanization rate, with additional attention in terms of the impacts of economic development, industrial structure, and human resources on local air quality. Subsequent developments to the MRYR region should be focused on finding a balance between economic development and environmental quality. The PRD region requires attention in terms of maintaining air quality.
5 Conclusion
The PM2.5 AM has gradually decreased from 2015 to 2018, and areas with concentrations below 35 µg/m3 continue to increase; however, the pollution levels still vary in different regions. The Beijing–Tianjin–Hebei and surrounding areas, Yangtze River Delta, Chengdu-Chongqing region, Middle Reaches of the Yangtze River, and Pearl River Delta are some of the key areas for air pollution prevention and control. The number of PM2.5-related premature deaths in 287 cities where exceeded Grade II standards (35 μg/m3) were 872035, 702948, 731110 and 414141, respectively, based on the VSL measure. The population distribution, industrial structure, and economic and social development levels accounted for 83%, 83%, 66%, and 74% of the PM2.5-related health economic losses over 2015 to 2018, respectively, based on the GWR. Therefore, policy makers should consider the impacts of population distribution, industrial structure, and economic and social development levels on health economic losses when formulating air pollution control policies in the future.
Data availability statement
The datasets presented in this study can be found in online repositories. The names of the repository/repositories and accession number(s) can be found in the article/Supplementary Material.
Author contributions
JG and XW structured and wrote the manuscript draft. JY, YZ, and SK analyzed the data and conducted descriptive analyses. FL and ZQ reviewed and polished the manuscript. All authors contributed to manuscript revision, reading, and approval of the submitted version.
Funding
This study was supported by the Hubei Provincial Outstanding Young Science and Technology Innovation Team Project (T2021032) and the “Fundamental Research Funds for the Central Universities” of Zhongnan University of Economics and Law (202211412; 202211413).
Conflict of interest
The authors declare that the research was conducted in the absence of any commercial or financial relationships that could be construed as a potential conflict of interest.
Publisher’s note
All claims expressed in this article are solely those of the authors and do not necessarily represent those of their affiliated organizations, or those of the publisher, the editors, and the reviewers. Any product that may be evaluated in this article or claim that may be made by its manufacturer is not guaranteed or endorsed by the publisher.
Supplementary material
The Supplementary Material for this article can be found online at https://www.frontiersin.org/articles/10.3389/fenvs.2022.951505/full#supplementary-material
References
Ding, D., Zhu, Y., Jang, C., Lin, C. J., Wang, S. X., Fu, J., et al. (2016). Evaluation of health benefit using BenMAP-CE with an integrated scheme of model and monitor data during Guangzhou Asian Games. J. Environ. Sci. 42 (4), 9–18. doi:10.1016/j.jes.2015.06.003
Duan, J. X., Zhai, W. X., Cheng, C. Q., and Chen, B. (2018). Socio-economic factors influencing the spatial distribution of PM2.5 concentrations in China: An exploratory analysis. Environ. Sci. 39 (5), 2498–2504. doi:10.13227/j.hjkx.201709087
Fu, C. H., Wang, W. J., Tang, J., Zeng, X. C., and Feng, Y. (2014). Study on demographic spatial distribution of health risks caused by PM2.5: A case from shenzhen. China Soft Sci. 9, 78–91. doi:10.3969/j.issn.1002-9753.2014.09.008
Hoek, G., Krishnan, R. M., Beelen, R., Peters, A., Ostro, B., Brunekreef, B., et al. (2013). Long-term air pollution exposure and cardio-respiratory mortality: A review. Environ. Health 12 (1), 43. doi:10.1186/1476-069x-12-43
Huang, W., Cao, J. J., Tao, Y. B., Dai, L. Z., Lu, S. E., Hou, B., et al. (2012). Seasonal variation of chemical species associated with short-term mortality effects of PM2.5 in Xi’an, a central city in China. Am. J. Epidemiol. 175 (6), 556–566. doi:10.1093/aje/kwr342
Jo, C. (2014). Cost-of-illness studies: Concepts, scopes, and methods. Clin. Mol. Hepatol. 20, 327–337. doi:10.3350/cmh.2014.20.4.327
Kan, H. D., London, S. J., Chen, G. H., Zhang, Y. H., Song, G. X., Zhao, N. Q., et al. (2007). Differentiating the effects of fine and coarse particles on daily mortality in Shanghai, China. Environ. Int. 33 (3), 376–384. doi:10.1016/j.envint.2006.12.001
Li, T. T., Guo, Y. M., Liu, Y., Wang, J. N., Wang, Q., Sun, Z. Y., et al. (2019). Estimating mortality burden attributable to short-term PM2.5 exposure: A national observational study in China. Environ. Int. 12, 245–251. doi:10.1016/j.envint.2019.01.073
Li, H. J., Zhou, D. Q., and Wei, Y. J. (2020). Review on health economic loss assessment of air pollution. Res. Environ. Sci. 33 (10), 2421–2429. doi:10.13198/j.issn.1001-6929.2020.04.25
Li, F., Liu, J. A., Chen, Z. P., Huang, J. H., Liu, C. Y., and Qu, Z. G. (2021). Navigating to urban environmental health: Professionalized and personalized healthy living assistant based on intelligent health risk management. Urban Clim. 40, 101020. doi:10.1016/j.uclim.2021.101020
Liu, S., Wang, L., Liu, H. X., Su, H., Li, X. L., and Zheng, W. F. (2018). Deriving bathymetry from optical images with a localized neural network algorithm. IEEE Trans. Geosci. Remote Sens. 56 (9), 5334–5342. doi:10.1109/tgrs.2018.2814012
Qu, Z. G., Wang, X. Y., Li, F., Li, Y. N., Chen, X. Y., and Chen, M. (2020). PM2.5-related health economic benefits evaluation based on air improvement action plan in Wuhan city, middle China. Int. J. Environ. Res. Public Health 17 (2), 620. doi:10.3390/ijerph17020620
Tan, C. C., Wang, Y. P., Lin, M. Y., Wang, Z., He, L., Li, Z. Y., et al. (2018). Long-term high air pollution exposure induced metabolic adaptations in traffic policemen. Environ. Toxicol. Pharmacol. 58, 156–162. doi:10.1016/j.etap.2018.01.002
Tong, M. K., Gao, J. X., Tian, M. R., and Ji, P. (2015). Subduction of PM2.5 by road green space in Beijing and its health benefit evaluation. China Environ. Sci. 35 (9), 2861–2867. doi:10.3969/j.issn.1000-6923.2015.09.045
Wang, J. Z., Zhang, L. F., Niu, X. S., and Liu, Z. K. (2020). Effects of PM2.5 on health and economic loss: Evidence from Beijing-Tianjin-Hebei region of China. J. Clean. Prod. 257, 120605. doi:10.1016/j.jclepro.2020.120605
Wu, R., Dai, H. C., Geng, Y., Xie, Y., Masui, T., Liu, Z. Q., et al. (2017). Economic impacts from PM2.5 pollution-related health effects: A case study in Shanghai. Environ. Sci. Technol. 51 (9), 5035–5042. doi:10.1021/acs.est.7b00026
Xia, Z. (2019). Assessment of the health effect of air pollution in Nanjing. Jiangsu China: Nanjing University.
Yang, K., Yang, Y. L., Zhu, Y. H., Li, C., and Meng, C. (2016). Social and economic drivers of PM2.5 and their spatial relationship in China. Geogr. Res. 35 (6), 84–92. doi:10.11821/dlyj201606005
Yang, Y., Luo, L. W., Song, C., Yin, H., and Yang, J. T. (2018). Spatiotemporal assessment of PM2.5-related economic losses from health impacts during 2014-2016 in China. Int. J. Environ. Res. Public Health 15 (6), 1278. doi:10.3390/ijerph15061278
Yu, G. H., Wang, F. F., Hu, J., Liao, Y., and Liu, X. Z. (2019). Value assessment of health losses caused by PM2.5 in Changsha city, China. Int. J. Environ. Res. Public Health 16, 2063. doi:10.3390/ijerph16112063
Keywords: PM2.5, health economic loss, geographically weighted regression, spatiotemporal variation, China
Citation: Guo J, Li F, Qu Z, Wang X, Yan J, Zhou Y and Kong S (2022) Quantitative evaluation of PM2.5-related health economic losses and analysis of their driving factors in Chinese cities. Front. Environ. Sci. 10:951505. doi: 10.3389/fenvs.2022.951505
Received: 24 May 2022; Accepted: 08 August 2022;
Published: 14 September 2022.
Edited by:
Haikun Wang, Nanjing University, ChinaReviewed by:
Jie Liang, Hunan University, ChinaZhijie Zhang, University of Connecticut, United States
Copyright © 2022 Guo, Li, Qu, Wang, Yan, Zhou and Kong. This is an open-access article distributed under the terms of the Creative Commons Attribution License (CC BY). The use, distribution or reproduction in other forums is permitted, provided the original author(s) and the copyright owner(s) are credited and that the original publication in this journal is cited, in accordance with accepted academic practice. No use, distribution or reproduction is permitted which does not comply with these terms.
*Correspondence: Fei Li, bGlmZWlAenVlbC5lZHUuY24=; Zhiguang Qu, cXV6aGlndWFuZ0B6dWVsLmVkdS5jbg==