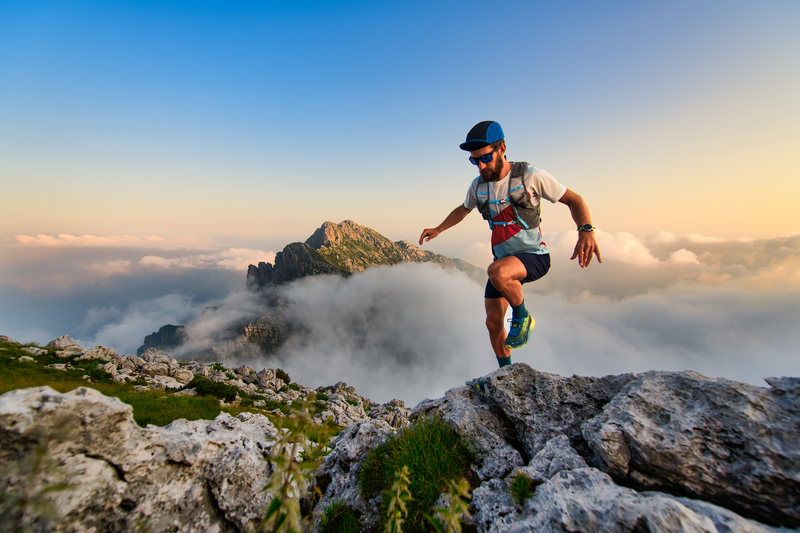
94% of researchers rate our articles as excellent or good
Learn more about the work of our research integrity team to safeguard the quality of each article we publish.
Find out more
ORIGINAL RESEARCH article
Front. Environ. Sci. , 05 July 2022
Sec. Environmental Economics and Management
Volume 10 - 2022 | https://doi.org/10.3389/fenvs.2022.949164
This article is part of the Research Topic Financial and Trade Globalization, Greener Technologies and Energy Transition View all 45 articles
Due to the impact of COVID-19 and other factors, SMEs are increasingly facing the contradiction of financing constraints. In order to explore feasible ways to ease the financing constraints of SMEs, we further incorporate digital inclusive finance into the analytical framework of financing constraints of SMEs, and test the causal relationship between them by using models such as two-way fixed effects model and moderated intermediary effect model. We find that digital inclusive finance can effectively alleviate financing constraints of SMEs, and this phenomenon is particularly significant in private enterprises and family enterprises. In addition, the mitigation effect of digital financial inclusion is more like icing on the cake, and it cannot provide practical assistance to small and medium-sized enterprises with poor business conditions. Further research also finds that commercial credit seems to be an effective channel for digital financial inclusion to alleviate financing constraints of SMEs, but corporate leverage also plays an important role in this process, playing a negative moderating role. In general, our study strengthens the effectiveness of digital inclusive finance in alleviating financing constraints of SMEs, at the same time, confirming the existence of commercial credit channels and the moderating effect of enterprises’ lever ratio and providing a feasible direction for alleviating financing constraints of enterprises.
The Central Economic Work Conference of China in December 2020 emphasized the need to continuously stimulate the vitality of market players, improve policies to reduce fees and taxes, strengthen inclusive financial services, make greater efforts to promote reform and innovation, and increase the vitality of market players, especially small and medium-sized enterprises and individual entrepreneurs. According to data from the Ministry of Industry and Information Technology, by the end of 2018, the number of SMEs in China exceeded 30 million, a huge base contributes more than half of China’s tax revenue and gross product accounts for more than 60% of the annual GDP, while the life cycle of most SMEs is only 3 years, and less than 7% can exceed 5 years. The financing constraint faced by SMEs, however, is an important reason for their short life cycle, which greatly limits the sustainability of their contribution to our economic development.
As the integration of digital technology and the financial industry continues to deepen, the trend of digitalization of financial inclusion is also coming to the fore (Nuanmeesri, 2021; Park and Kim, 2022). A report by Peking University’s Digital Inclusive Finance Index shows that China’s digital inclusive finance business has made substantial progress from 2011 to 2018. The median value of the Digital Inclusion Index for each province in China was only 33.6 in 2011, but grew to 294.3 in 2018, with an average annual increase of 36.4% in the index (Li et al., 2020; Chen and Zhang, 2021). Digital inclusive finance has become the mainstream of the current development of inclusive finance because of its characteristics such as extending the service boundary of inclusive finance (Buchak et al., 2018; Lee and Shin, 2018; Bollaert et al., 2021). What is the role of digital inclusion when SMEs, which are typically weak economy individuals, face financing constraints? If digital inclusion works for SMEs, is there any significant difference in the results? What is the path of action?
Most studies show that digital inclusive finance can significantly alleviate the degree of information asymmetry between the supply and demand of funds, provide diversified financial services, improve the financial accessibility and convenience of real enterprises and effectively alleviate the financing constraints faced by SMEs (Demertzis et al., 2018; Yin et al., 2019; Chen and Yoon, 2021). Nevertheless, the effect of digital inclusive finance on alleviating the financing constraints of enterprises shows significant inconsistency across different enterprises. In terms of its transmission path, digital inclusive finance may achieve the effect of alleviating corporate financing constraints by reducing corporate information asymmetry and enhancing corporate transparency (Heiskanen, 2017). Through the review of the existing literature, it is not difficult to find that although the “black box” of digital inclusive finance on corporate financing constraints has been widely concerned by scholars, most of the existing studies focus on a single channel, without in-depth analysis on whether its intermediary mechanism is regulated by other factors. In order to further explain the influence mechanism of digital inclusive finance and corporate financing constraints, we tentatively include business credit into the analysis framework of digital inclusive finance and corporate financing constraints, and further investigate whether this path will be adjusted by corporate leverage level, hoping to fully analyze the channels of digital inclusive finance on corporate financing constraints.
We have made efforts to explore the mechanism of digital inclusive finance on the financing constraints of small and medium-sized enterprises. However, due to the availability of data, our research samples are mostly limited to listed small and medium-sized enterprises, and we have not conducted in-depth excavation for smaller micro enterprises. But one flaw cannot obscure the splendor of the jade, compared with the existing literature, we may have the following marginal contributions: first, on the basis of analyzing the static impact of digital inclusive finance on the financing constraints of SMEs from multiple perspectives, we further analyze its dynamic characteristics and clarify the characteristics of the impact of digital inclusive finance on the financing constraints of SMEs in the time dimension; second, we explore the heterogeneity of digital inclusive finance on the financing constraints of SMEs through multiple channels. Based on sub-sample regression and quantile regression models, we explore the heterogeneity of digital inclusive finance to alleviate corporate financing constraints under different enterprise property rights nature and different levels of financing constraints, broadening the heterogeneity of the inhibiting effect of digital inclusive finance on financing constraints of SMEs; third, we further identify the mechanism of the effect of digital inclusive finance on the financing constraints of SMEs, and adopt a model with moderating mediating effects to confirm the survival of the commercial credit channel and the moderating path of corporate leverage on the commercial credit channel, which has certain implications for subsequent studies.
Although the theory of MM, proposed by Professors Modigliani and Miller, argues that the value of a company is independent of its capital structure. However, in reality, due to the changes in the value of risk during external financing, investors will lower their expectations on the solvency of the enterprise and refuse to inject funds, thus resulting in financing constraints.
The financing constraints of SMEs in China have been a long-standing problem and the formal introduction of the concept of digital inclusive finance can be traced back to the G20 Summit held in 2016. Since the introduction of digital inclusive finance, its effect on the financing constraints of SMEs has received wide attention. Generally speaking, digital inclusive finance may affect the financing constraints of SMEs through the following ways. First, improving enterprise credit evaluation information. The root cause of the financing constraints of SMEs is the information asymmetry between borrowers and lenders, and the development of digital inclusive finance will effectively promote the combination of digital technology and financial institutions to help financial institutions fully grasp the current business situation and credit level of enterprises and make scientific decisions (Chun and Cho, 2022; Lee, 2022). Second, improving the efficiency of enterprise financing. Unlike traditional inclusive finance, digital inclusive finance based on the Internet platform will extend to a wider group of SMEs, and the online peer-to-peer business model will optimize interactivity and reduce the operational aspects of the enterprise financing process, thus improving the efficiency of enterprise financing. Existing literature has demonstrated that digital inclusive finance has a significant mitigating effect on financing constraints of SMEs through cash-cash flow sensitivity models, two-way fixed effects models and Eulerian investment equation models (Zhang et al., 2020). However, they are more focused on analyzing the static impact mechanism of digital inclusive finance on financing constraints of SMEs, while relatively little research has been conducted on its dynamic mechanism. In fact, we can not only discuss the lagged effect of digital inclusion to some extent, but also more effectively mitigate the endogeneity problem caused by two-way causality by including first-order lags of digital inclusion indicators in the benchmark study (Li et al., 2021a). Although much of the correlation between digital inclusive finance and the financing constraints of SMEs can be attributed to the inhibiting effect of digital inclusive finance on the financing constraints of SMEs, the driving effect of the financing constraints of SMEs on the development of digital inclusive finance cannot be ignored. When SMEs face greater financing constraints, they will be more motivated to develop digital inclusive finance in order to optimize their information disclosure capabilities and enhance their operational capabilities. The use of first-order lags can observe the lagged effect of digital inclusive finance, but it is difficult to study its dynamic long-term mechanism. The widespread nature of digital financial inclusion radiation leads to a more complex action path, and therefore its action results may reflect a time lag effect and show a longer dynamic characteristic in the time dimension.
Based on the above analysis, we propose the hypothesis1 that digital inclusive finance has a suppressive effect on the financing constraints of SMEs and is characterized by a long-term mechanism.
Despite the continuous development of digital inclusive finance, it can be found from the report of Peking University Digital Inclusive Finance Index (2011-2020) that the development of digital inclusive finance in different regions of China is obviously uncoordinated, and the development degree of digital inclusive finance in the eastern region is significantly higher than that in the central and western regions. The problem of heterogeneity is often prevalent in the study of digital inclusive finance constraints on SMEs due to various factors. The results of the study on the financing constraints of SMEs under different levels of economic development of inclusive finance show that the development of inclusive finance will improve the financing constraints of SMEs in regions with higher levels of economic development, but the mitigation effect is not significant for the more economically backward regions, which can hardly afford the costs necessary for financial development on their own and are less attractive to external funds, while the result cannot be shown in the overall regression. In addition, the impact of digital inclusive finance on corporate financing constraints will also differ under different property rights subjects. On the one hand, compared with private enterprises that lack high-quality collateral assets, state-owned enterprises tend to have stronger financial strength and abundant high-quality assets, which make them more likely to obtain financial support from financial institutions, and the implicit guarantee unique to state-owned enterprises also makes them more likely to be favored by financial institutions, and the function of digital finance to improve information evaluation will be greatly discounted to state-owned enterprises under this condition, causing the difference in the impact of digital inclusive finance on SMEs between state-owned enterprises and private enterprises (Acharya et al., 2016). Therefore, it is necessary to consider the differences in financing constraint mitigation due to the heterogeneity problem of corporate subjects. On the other hand, in the known studies on heterogeneity, most scholars have studied the heterogeneity of different types of firms, regions, etc. from the perspective of explanatory variables, i.e., by adding interaction terms or group regressions to the model, but there is relatively little literature that considers heterogeneity from the perspective of explanatory variables. In fact, the financing constraints faced by SMEs largely reflect the evaluation of financial institutions on their own operating conditions and credit levels, and the two often show a tendency to move in the same direction. Digital financial inclusion dividends enjoyed by enterprises with different degrees of financing constraints will vary in this condition. In the case of extreme financing constraints, SMEs may have multiple default records and high business risks, facing bankruptcy crises, so the role of digital inclusive finance in helping them will be minimal; while in the case of lighter financing constraints, the optimized channels of digital inclusive finance will work directly for SMEs, effectively alleviating the financing constraints faced by them. Previous studies have shown that quantile regression can effectively identify the influence of independent variables on dependent variables under different conditional distributions (Maiti, 2021). In view of this, for the subject of this paper, different enterprises face great differences in financing constraints, which cannot be reflected in the least square estimation based on mean regression.
Based on the above analysis, we propose the hypothesis2 that the inhibitory effect of digital inclusive finance on SMEs’ financing constraints is heterogeneous under different property right subjects and different financing constraints.
Digital inclusive finance can significantly reduce the information asymmetry between financiers and investors through the combination of scenarios and data. In the case of complete information, the substitution of external financing and internal financing will be strengthened, and the problem of corporate financing constraints will be alleviated, but the actual mechanism of action is still not systematically clear. With the development of digital inclusive finance, the transparency of corporate information will improve, the market will get more information about the business status of enterprises (Demertzis et al., 2018), and the commissioned accounting firms will give reasonable audit opinions based on public data, at which time the business reports of listed SMEs will be more authentic, and better performing enterprises will get better commercial credit in their own industry chain, releasing positive credit signals to the market, reducing their own credit risk, increasing creditors’ expectations of their debt repayment, and lowering debt financing costs, thus alleviating corporate financing constraints (Lin et al., 2011; Duchin et al., 2017). It is not difficult to find that digital inclusive finance can alleviate the financing constraints of enterprises in a wide range of ways. Although scholars have conducted in-depth studies on the ways of digital inclusive finance to alleviate the financing constraints of enterprises, most of them focus on a single mediating effect or moderating effect, and few scholars have analyzed the mechanism of this effect by considering the moderating mediating effect. As a matter of fact, the relationship between digital inclusive finance and financing constraints of SMEs is complex, and it is often biased to analyze the mechanism of digital inclusive finance on financing constraints of SMEs from a single perspective, while the moderated mediated effects model can clarify the complex relationship between them to a certain extent. It has been shown that the improvement of corporate business credit will significantly alleviate the financing constraint of enterprises, but the way in which digital inclusive finance acts on business credit has not been further analyzed (Biais and Gollier, 1997). In most cases, corporate leverage will also affect the business credit of firms and thus play a moderating role. The operating leverage ratio of a company can visually reflect the ratio of assets and liabilities of the company and its own operating risks. When a company’s leverage ratio is higher than the generally acceptable level in the industry, the related upstream and downstream enterprises will question its solvency, which will reduce its commercial credit.
Based on the above analysis, we propose the hypothesis3 that the development of digital inclusive finance will have a significant inhibitory effect on corporate financing constraints, and this mechanism of action will be achieved in part by increasing commercial credit and moderated by corporate leverage.
This paper makes an empirical analysis based on the data from 2011 to 2018 of Chinese listed companies in the former small and medium-sized enterprises board which merged with the main board of Shenzhen Stock Exchange in April 2021. The SMEs financial data is obtained from the CSMAR database as well as the RESSET database. In order to improve the robustness of the regression results, we processed the data as follows: first, We apply a 1% bilateral tailing to the main variables in the model to eliminate regression bias due to outliers; second, ST and *ST enterprises are considered to be excluded due to their poor business performance, low credibility of data values, high abnormality rate, and risk of delisting; third, considering that the financial industry is a highly indebted industry with low reference of financial indicators, therefore, financial SMEs are excluded based on the SEC 2012 edition industry classification standards.
With the current status of research on the measure of financing constraint, it can be broadly divided into two categories. One is the adoption of a single financial indicator as a proxy variable for a firm’s financing constraint, e.g., Li et al. (2021b) used 30% of the sample enterprise’s asset scale as the critical point of whether the enterprise has financing constraints to describe the financing constraints of the enterprise. The second is to construct a comprehensive financing constraint index by selecting different dimensional indicators. In the existing studies, the comprehensive financing constraint index can better overcome the endogeneity problem in the model and is therefore more widely used. Although the KZ index constructed by Kaplan and Zingales (1997) using Logit regression based on the investment-cash flow sensitivity approach is able to portray to some extent the financing constraints faced by firms, there are certain shortcomings due to the measurement bias problem of Tobin’Q in China, which may lead to some measurement bias in the KZ index (Whited and Erickson, 2001). Using a similar approach, Mulier et al. (2016) added external industry characteristics to the KZ index and used it to construct the WW index, but in terms of the research subjects in this paper, there are shortcomings such as size constraints and imperfect statistics of the financial sector in SMEs, so the financial indicators used in the construction of the WW index may have statistical errors. In contrast, the SA index constructed by Hadlock and Pierce (2010) by selecting firm asset size and firm age is able to better portray the financing constraints faced by firms while being more exogenous. Considering the endogeneity of the regression results, the SA index with stronger exogeneity characteristics is finally selected as a measure of the financing constraints of enterprises for analysis.
The core explanatory variable of this paper is the digital inclusive finance index. In view of the existing literature, the widely used Peking University Digital Inclusive Finance Index is selected to measure the index, which provides a more comprehensive picture of the current development of digital inclusive finance in China. In the course of the study, an analytical path from high-dimensional to low-dimensional and micro to macro is adopted to test the model results. We scaled the digital financial inclusion and its sub-indexes by 100 times to facilitate the analysis of the results.
The main variables incorporated in the baseline model are shown in Table 1. Among them, return on assets, return on invested capital, net profit rate of total assets, operating profit rate and so on mainly reflect the operating capacity of enterprises. Return on assets is expressed using total profit divided by total assets; return on invested capital is defined as (net profit + finance costs)/(total assets − current liabilities). Due to the measurement bias problem of Tobin’Q, the growth rate of main business is selected to measure the growth ability of the company. In addition, the dividend distribution rate was chosen to measure the payout capacity because it can reflect the operating condition within the company to some extent. Considering the main impact of corporate liquidity on corporate financing constraints, we selected the cash recovery rate to control for it, which can be expressed as the net cash flow generated from corporate operating activities divided by total assets at the end of the period. In this paper, we refer to Lannelongue et al. by including the firm’s capital intensity in the model to control for differences in financing constraints due to different types of firms, (Lannelongue et al., 2017). Referring to Tsipouridou and Spathis (2014), a dichotomous variable of the audit opinion of the auditor firm is included, which is taken as 0 when the auditor issues a standard unqualified opinion and one for the others. In addition, time dummy variables for time effects and industry dummy variables for industry effects are included in the model to control those factors that vary over time and individually and to reduce the correlation between the disturbance terms and the core explanatory variables.
The statistics in the model are described as shown in Table 2. As can be seen from the results in Table 2, there is a significant difference between the maximum and minimum values with respect to |SA|, indicating a large variation in the internal financing constraints of SMEs, thus reflecting the need for heterogeneity analysis at different degrees of financing constraints, i.e., at different quartiles of |SA|. In addition, the standard deviation of the Digital Inclusion Index shows that the degree of fluctuation of digital inclusion in China is large across geographic regions and years, and it can be found that the difference between the minimum and maximum values of digital inclusive finance index in each city is close to 300 by observing the city digital inclusive finance index Cia. By looking at the other financial indicators in Table 2, it is found that the level of operation varies significantly among different companies, and this finding also reflects to some extent the need for heterogeneity analysis by sub-sample regression.
This paper refers to the method of Fernandes and Ferreira (2021) to construct SA index. Its formula is shown in Eq. 1.
SA denotes the financing constraint index,
Based on the SA index obtained in Eq. 1, the following benchmark model is considered using the LSDV method:
Where
In addition, due to the specificity of the financing constraints faced by SMEs, i.e., SMEs themselves face two types of financing constraints—difficulty in raising funds and failure to raise funds. We treat |SA| based on this perspective, considering that groups with larger |SA| indices are themselves in poor operating conditions, unable to gain a competitive advantage in their industries, and that information transmission leads to negative external expectations, and therefore are unable to obtain internal and external financing. Therefore, the |SA| index above the 90% quantile is assigned to the |SA| index at the 90% quantile as a way to portray the situation where a firm is unable to raise capital. At this point, the model faces right-censored loss, and to solve the selective bias problem, the panel Tobit model is used for re-estimation, and the equations are constructed as shown in Eq. 3.
Based on the baseline regression, this paper replaces the digital inclusive finance index with the lagged term of the core explanatory variables to re-regress, on the one hand, to corroborate the robustness of the regression results, and on the other hand, to analyze the time lag effect of the digital inclusive finance index in alleviating financing of SMEs and its long-term mechanism. Based on this analysis, Eq. 4 is constructed.
In Eq. 4, the
In the heterogeneity analysis, we will approach it from two perspectives. First, from the perspective of explanatory variables, corporate subjects are analyzed according to the nature of enterprises into state-owned and private, family and non-family; second, from the perspective of explanatory variables, the heterogeneity of the coefficients of regression results of core explanatory variables at different degrees of explanatory variables is explored, i.e., the heterogeneity of the inhibitory effect of digital inclusive finance on SMEs’ financing constraints at different financing constraints quantile points is considered. We model the quantile regression for this purpose as shown in Eq. 5.
In the Eq. 5, the
We use the Least Squares Dummy Variable method (LSDV) to fix the effects of time and industry effects on the model analysis. And based on this, we analyze the effect of digital financial inclusion index Cia on the explanatory variable financing constraints |SA|. The results of the baseline regression are shown in Table 3.
Firstly, the regression analysis of the benchmark Eq. 2 is performed on the basis of fixed time and industry effects and the addition of control variables; Secondly, considering the characteristic facts of the development of digital inclusive finance, the digital inclusive finance index is downscaled to analyze the impact of the breadth of coverage and depth of use of the digital inclusive finance index on the financing constraints of SMEs; finally, considering the specificity of the financing constraints faced by SMEs, the |SA| index is censored and a mixed panel Tobit model is used for the analysis. In addition, to alleviate the problem of invalid t-statistics caused by heteroskedasticity, the regression results are corrected by robust standard errors with industry as clusters.
The results in Table 3 show that there is a negative correlation between the digital inclusive finance index and |SA|. Which means that a larger digital inclusive finance index will lead to a smaller |SA| and the financing constraint faced by enterprises can be alleviated, i.e., the development of digital inclusive finance has a significant contribution to improving the financing constraint of SMEs. In addition, M(2) and M(3) in Table 3 illustrate that there is also a negative and significant relationship between the breadth of coverage and depth of use of digital inclusive finance and the |SA| index at the 1% level of significance, which further supports the results of M(1). According to the empirical results in Table 3, it can be concluded that the development of digital inclusive finance has a significant inhibitory effect on the financing constraints of SMEs, and this inhibitory effect remains robust to its sub-index.
Based on the benchmark regression, the direction of the role of digital financial inclusion index on corporate financing constraints in the case of deletion is further analyzed. The regression results are shown in Table 4.
In Table 4, it can be found that the LR tests all strongly reject the original hypothesis that a mixed Tobit model should be used, therefore, it is reasonable to use a random effects panel Tobit model. On this basis, as listed in the Table 4, observing the coefficients of the total index and each digital financial inclusion dimension index, both the total digital financial inclusion index and its sub-indexes show a significant negative correlation with the corporate financing constraints index
The above analysis only considers the impact of digital inclusive finance index on SMEs’ financing constraints from a static perspective. To further analyze the dynamic impact mechanism of digital inclusive finance on SMEs’ financing constraints, we replace the total index lagged term of the digital inclusive finance index with the original model’s digital inclusive finance index for analysis and the regression results are shown in Table 5.
In the Table 5, the first-order lagged coefficient of the core explanatory variable Cia is −0.012 and significant at the 1% significance level. This lagged coefficient not only verifies the robustness of the effect of digital inclusive finance on the alleviation of corporate financing constraints under the condition of considering reverse causality, but also indicates that there is a time lag characteristic of the inhibitory effect of digital financial inclusion index on the financing constraints of SMEs. This feature may be mainly caused by a combination of both internal and external time lag pathways. On the one hand, the development of digital inclusive finance is a gradual process, and its alleviation of enterprise financing constraints needs to act on the external environment, such as access to credit information and business status through the Internet and other means, so as to alleviate the asymmetry of external information and reduce the possibility of adverse selection and moral hazard. This process then manifests itself as an external time lag. On the other hand, digital financial inclusion acts on the internal aspects of the company, mainly in the reduction of finance costs and optimization of decision-making processes, and these improvements do not manifest themselves in a short period of time, thus creating an internal time lag. It also partly explains the fact that the coefficients of the first-order lagged and second-order lagged digital inclusion indices are larger than those of the current period digital inclusion index. In the long run, the dynamic characteristics of the digital financial inclusion index on corporate financing constraints exhibit a trend that rises and then falls, and the trend peaks at first-order lags and second-order lags. Table 5 indicates that there is a long-term mechanism for the alleviation effect of digital inclusive finance on enterprise financing constraints, and the strength of this long-term mechanism shows a lagged distribution feature. Comprehensive analysis of this part of the empirical results shows that the development of digital inclusive finance can alleviate the financing constraints faced by enterprises, and there is a dynamic lagged distribution feature, and this conclusion can prove the hypothesis1 of this paper is valid.
We conduct the following robustness tests. First, the regression is re-run using KZ index instead of SA index for robustness testing. KZ index is constructed to measure operating cash flow, cash payout level, cash holdings, degree of indebtedness, and growth, respectively, referring to the method of Castro et al. (2015). Among them, operating cash flow is expressed using net cash flow from operating activities divided by total assets at the beginning of the period; cash payout level is expressed using cash dividends divided by total assets at the beginning of the period; cash holdings are expressed using cash and cash equivalents divided by total assets at the beginning of the period, and the degree of debt is expressed using gearing ratio; and growth is measured by Tobin’s Q. They are divided into binary dummy variables by their means in turn, and logistic regressions are re-run with each variable through a logit regression model, and the fitted values of the regression results are considered as KZ indices. According to the definition of KZ index, KZ index shows a positive correlation with the degree of corporate financing constraints. Second, the general characteristics of the digital financial inclusion index to alleviate the financing constraints of enterprises are tested by replacing the digital financial inclusion index with a province-based one by up-dimensioning the core explanatory variables. Third, in order to exclude the bias of the model estimation by firms with a short establishment period, the sample of SMEs with an establishment period of not more than 3 years within the sample period is excluded for re-testing. Fourth, the control variables in this paper are mostly micro variables selected to measure the internal characteristics of firms, while the macroeconomic environment may be missed to have an impact on the profitability of SMEs, which in turn affects the credit assessment of SMEs by financial institutions. Therefore, we expect that macroeconomic development may have a positive impact on corporate financing constraints and select GDP per capita for testing. The results of the robustness test with various regressions are shown in the Table 6.
It is observed from the regression results M(12) in Table 6 that the coefficient of Cia is still significantly negative when the KZ index is adopted to replace the explanatory variables, indicating that there is a significant inhibitory effect of digital financial inclusion on corporate financing constraints, thus further confirming the results of the benchmark model. In the regression results from M(13) to M(15), the coefficients of the explanatory variables after the dimensional upgrade are still consistent with the baseline regression results, which indicates that the inhibitory effect of digital inclusion remains obvious even when the scope is expanded to the provincial level, reflecting to some extent the characteristics of inclusion in digital inclusion. The estimated results after excluding samples with shorter establishment time are shown in M(16), and the coefficients of the core explanatory variables do not show a fundamental shift. M(17) shows the estimation results after adding the regional economic development GDP per capita variable, and it can be found that the coefficient of the regional economic development variable Pgdp is not statistically significant. Therefore, it can be concluded that regional economic development has no significant effect on local SMEs’ financing constraints, negating the previous expectation. Taken together, the conclusion that digital financial inclusion has a significant inhibitory effect on SMEs’ financing constraints in the benchmark regression can be considered robust.
Considering that the model may have endogeneity problems caused by observable omitted variables, we intend to adopt the instrumental variable method for endogeneity testing. Referring to the method used by Luo et al. (2022) to construct the FinTech index, the text mining method was used to dig out the frequency of words about digital inclusive finance appearing in the Baidu search engine in each province each year and take the logarithmic value as an instrumental variable. The instrumental variable constructed by the text mining method can better reflect the characteristics of digital inclusive finance and meet the requirements related to digital inclusive finance. After controlling for enterprise micro variables, year effects and industry effects, there is no direct correlation between the constructed digital inclusive finance index and micro enterprises, so the word frequencies constructed by using text mining to measure digital inclusive finance can be used as a valid instrumental variable. In the regression process, the instrumental variable is first added to the model to derive the fitted value of the core explanatory variable, and then the fitted value of this core explanatory variable is brought into the model for a two-stage regression. Considering the possible endogeneity problem of the censored data, a two-stage least squares regression was performed in the endogeneity test by replacing the explanatory variables with the censored |SA|. The regression results are shown in Table 7.
Step2 in the Table 7 shows the results of the two-stage regression, and it can be found that the results obtained after using the digital financial inclusion index constructed by the text mining method as an instrumental variable do not change substantially in direction from the baseline regression results. Considering that instrumental variables may have weak instrumental variables and unidentifiable problems, weak instrumental variable test and unidentifiable test are conducted in this paper in turn, and the test results all indicate that the selected instrumental variables are valid. Among them, the p-value of Kleibergen-Paap rk LM statistic indicates that the original hypothesis of unidentifiability is strongly rejected and the instrumental variables are considered to be identifiable, i.e., the instrumental variables are correlated with the endogenous explanatory variables; the F-value of Cragg-Donald Wald indicates that the original hypothesis of validity of the instrumental variables is accepted, i.e., there is no problem of weak instrumental variables. Based on the above endogeneity test analysis, it can be concluded that the conclusion that the development of digital inclusive finance helps to alleviate the financing constraints of SMEs is robust and further supports the rationality of the hypothesis1.
Research has found that SMEs themselves are the main bearers of the resource mismatch problem, and that the financing mismatch faced by SMEs is more pronounced than that faced by large enterprises. However, there is still a large heterogeneity in the internal financing constraints of SMEs even in this context. In view of this, we divided the SMEs subjects into state-owned enterprises as well as private enterprises, family enterprises and non-family enterprises for heterogeneity analysis, respectively. Among them, multi-person family enterprises, i.e., in addition to the actual controller, at least one related family member holding, managing or controlling a listed company is regarded as family enterprises; a single natural person entrepreneur enterprise as well as multiple natural person entrepreneur enterprises are regarded as non-family enterprises. The results of the regression of heterogeneity in the classification of business subjects are shown in Table 8.
As can be found from Table 8, the seemingly uncorrelated difference test of the digital financial inclusion index indicates that its inhibitory effect on financing constraints of SMEs is significantly different between state-owned and private, family and non-family. Digital inclusive finance has a significant inhibitory effect on the financing constraints of private SMEs, but it does not significantly alleviate the financing constraints of state-owned SMEs. This could be due to the fact that private SMEs may be subject to credit bias in the credit process compared to state-owned SMEs, resulting in the greater financing constraints they face. Therefore, when digital inclusive finance mitigates adverse selection and moral hazard, it can effectively soothe the financing constraints faced by private SMEs. The degree of financing constraints faced by state-owned SMEs is relatively low due to the nature of their state-owned property rights and the implicit government guarantee, so the degree to which digital inclusive finance can alleviate the financing constraints of state-owned SMEs is not obvious. In the heterogeneity analysis between family and non-family firms, digital inclusive finance alleviates the financing constraints of family firms to a significantly greater extent than non-family firms. It could be due to the fact that family firms have their own management system deficiencies and poor information transparency, which leads to their generally greater financing constraints than non-family firms. The continuous development of digital inclusive finance can effectively optimize the internal decision-making mechanism of family firms and improve the efficiency of internal and external information transfer, thus alleviating their financing constraints to a greater extent than non-family firms.
In the above discussion of heterogeneity, it can be found that there are differences in the degree of alleviation of financing constraints among different enterprise subjects, but there are also intersections of financing constraints among different enterprise subjects, and there may be some private SMEs with weaker financing constraints than some state-owned SMEs and some family enterprises with weaker financing constraints than some non-family enterprises, while this situation is often prevalent in reality. We consider using quantile regressions to analyze the impact of digital inclusion on financing constraints of SMEs at different quantile levels in order to analyze such financing constraints to further capture the problem of heterogeneity caused by differences in financing constraints. In addition, 2,000 times of bootstrap self-sampling were used to estimate its parameters to improve the robustness of the estimation results (Janekova et al., 2019; Janekova et al., 2021). In this paper, regression analyses are conducted from 0.1 to 0.9 quartiles respectively. And for space constraints, the regression results are presented in 0.2 quartile intervals as shown in Table 9.
Table 9 shows some of the regression results from 0.1 to 0.9 quartiles respectively. To test the variability of the estimation results, the regression coefficients of the digital finance index at different quantile levels are subjected to the Wald test with the estimated coefficients at the 0.5 quantile level. The results show that the estimates are significantly different from those at the 0.5 quantile at the extreme quantile represented by 0.9, confirming the existence of the heterogeneity problem caused by differences in financing constraints. By observing the coefficient of Cia, the total index of digital financial inclusion, it can be found that although the coefficient at 0.2 quantile cannot be significant on the 95% confidence interval, as for the overall trend, the inhibitory effect of digital financial inclusion is weaker with the increase of financing constraint degree of enterprises. In particular, the mitigating effect of the digital financial inclusion index is significantly weaker and at a lower level of significance when the financing constraints are extreme, e.g., at the 0.9 quartile, which may be due to the fact that SMEs under this financing constraint have their own poor business conditions and lack the necessary sources of profitability and core competitiveness to continue to sustain in the market. The role of digital financial inclusion, however, is more mainly reflected in reducing the degree of information asymmetry between the two parties, thus enabling those customers who are unable to lend but have good credit and repayment ability to obtain financing. Therefore, market exit mechanisms should be improved as soon as possible for enterprises in this financing constraint segment and enterprises with poor operating conditions should be encouraged to undergo bankruptcy and restructuring to enhance market vitality. This finding also further confirms the reasonableness of taking 90% for the |SA| index above as the threshold for its existence of difficulty and inaccessibility to financing. Figure 1 portrays the coefficient line graph of the digital inclusion index Cia at each quantile level in order to show more visually the change in the strength of the inhibitory effect of the digital inclusion index, with the shaded area representing the 95% confidence interval.
The above results show that the heterogeneity of the inhibitory effect of digital inclusive finance on corporate financing constraints is not only reflected in the differences of corporate subjects but also in the differences of financing constraints, which supports the validity of hypothesis2 in this paper.
In the above pages, it has been concluded through empirical studies that the development of digital inclusive finance will be effective in curbing the degree of financing constraints of SMEs, but its mechanism of action is still unclear. Most studies have confirmed that digital inclusive finance will achieve the effect of alleviating corporate financing constraints by reducing corporate financing costs, improving corporate transparency, and reducing corporate leverage, but will this path of action be moderated by other paths? Based on the moderated mediating effect model of Cheung and To (2021), we explore the impact mechanism of digital inclusive finance index on financing constraints of SMEs from the perspective of business credit. In the quantification of business credit, the accounts payable minus accounts receivable of the firm divided by total assets is used as a variable to measure business credit with reference to Choi and Kim (2005). A firm’s net business credit can be understood as the difference between accounts payable and accounts receivable, and the larger this difference is, the better the firm’s business credit is. However, this business credit may be influenced by the firm’s own leverage ratio. When the leverage of a company is high, the business risks are higher and business affiliates will lower the credit expectations of that company, resulting in a decline in the business credit of that company. Based on the above analysis, it is necessary for this section to explore whether the way of digital inclusive finance to alleviate financing constraints by improving business credit is moderated by the leverage ratio of enterprises.
By observing M(18) in Table 10, it can be found that the interaction term of Cia and Leverage is significant and has a significantly positive sign at the 5% level of significance, which implies that the effect of digital inclusion finance in alleviating corporate financing constraints will be moderated by total corporate leverage, and the increase in total corporate leverage will reduce the inhibitory effect of digital inclusion finance, which also reflects the importance of corporate deleveraging. In addition, the interaction term between Cia and Leverage is significantly negative at the 10% confidence interval as shown by M(19), suggesting that the contribution of digital inclusion to corporate business credit will diminish as corporate leverage increases. Finally, it can be found that the digital inclusion index still has a significant inhibitory effect on corporate financing constraints in the regression results of M(20), which indicates that the path of digital inclusion index to alleviate financing constraints consists from at least two routes. One is that the development of digital inclusive finance index will directly alleviate the financing constraint of enterprises, and the other is that the development of digital inclusive finance will improve the business credit of enterprises, thus indirectly alleviating the financing constraint of enterprises. In addition, the coefficient of Pay is negative, but the interaction of Leverage and Pay is significantly positive, which implies that the improvement of firms’ business credit can also alleviate firms’ financing constraints to some extent, but the effect diminishes with the increase of firms’ total leverage. Overall, corporate leverage will cut the extent to which digital inclusion inhibits corporate financing constraints by moderating the direct path and the first half of the intermediation effect path. Based on the above regression, the value of this intermediation effect can be calculated as (0.0443 − 0.00063 * Leverage) (-0.00297 + 0.00116 * Leverage), however, since the coefficient
We analyze the heterogeneous impact of digital inclusion on corporate financing constraints and explore its transmission mechanism based on the 2011-2018 data set of listed SMEs and the Peking University Digital Inclusion Index, and conclude the following.
First, our analysis reveals that digital inclusive finance will significantly alleviate the degree of financing constraints of SMEs by building a two-way fixed effects model and a panel Tobit model. The study finds that digital inclusive finance will have a positive shock with a significant dampening effect on financing constraints of SMEs conditional on the imposition of control variables. In addition, the results of dynamic analysis in the time dimension show that there is a long-lasting mechanism and hesitation in the inhibitory effect of digital inclusive finance on corporate financing constraints, which is most significant at the first-order lag or second-order lag. Second, there is significant heterogeneity in the alleviation of SMEs’ financing constraints by digital inclusive finance under the analysis of multiple perspectives. In terms of differences in corporate entities, the development of digital inclusive finance will significantly alleviate the financing constraints of private SMEs, while the alleviation effect on state-owned SMEs is not significant. In addition, the analysis of family firms and non-family firms reveals that digital inclusive finance has a more significant inhibitory effect on the financing constraints of family firms. In terms of differences in firm financing constraints, the quantile regression finds that the inhibitory effect of digital inclusion finance on financing constraints of SMEs decreases with the increase of SMEs’ financing constraints, which is significantly heterogeneous. Third, in the analysis of the paths of digital inclusion finance to alleviate financing constraints, we analyze the paths of action of digital inclusion finance based on a moderated mediating effects model and find that digital inclusion finance will have an impact on corporate financing constraints through at least two types of paths. The development of digital inclusive finance will directly alleviate the financing constraints of enterprises, and this dampening effect will be reduced by the positive regulation of corporate leverage. In addition, the development of digital inclusive finance will significantly improve the business credit of SMEs, thus indirectly alleviating the financing constraint of enterprises, and it is also moderated by the total corporate leverage in raising corporate business credit. The higher the total corporate leverage, the lower the magnitude of raising corporate business credit.
Based on the above findings, we put forward the following policy recommendations. First, on the basis of clarifying the inhibiting effect of digital inclusive finance on the financing constraints of SMEs, we promote the combination of digitalization and inclusive finance, bring into play the driving role of digital inclusive finance, further extend the reach of inclusive finance, reduce the cost of inclusive finance, and help the development of the real economy. Second, it should be fully aware of the heterogeneous characteristics of the inhibiting effect of digital inclusive finance on the financing constraints of SMEs. Reasonable deployment of financial resources and differentiated allocation should be implemented. Specifically, the differences of enterprise subjects should be fully grasped, financial resources should be injected precisely, the focus should be on supporting high-quality private SMEs and family enterprises in China. Furthermore, it is also necessary to use digital means to accurately identify business conditions, especially unsustainable business groups, improving the market exit mechanism and stimulating the market vigor. Third, our findings suggest that corporate leverage will negatively moderate the digital inclusive finance to alleviate corporate financing constraints. Although the deleveraging process in China has achieved remarkable results in recent years, the corporate leverage ratio has shown an upward trend again due to the impact of the epidemic, so the corporate leverage operation should be controlled within a reasonable range to prevent and mitigate systemic risks. In addition, we should also pay attention to the important role of commercial credit in digital finance in restraining the expansion of corporate financing constraints, further improve the commercial credit evaluation system, and open the commercial credit evaluation rules.
The raw data supporting the conclusion of this article will be made available by the authors, without undue reservation.
JL: Conceptualization, methodology, software, investigation, Writing—Original draft; RW: Data curation, Writing—Original draft, Formal Analysis; YG: Writing—Review and editing.
This research is supported by the National Natural Science Foundation of China (Grant No. 71804096), the Shandong Provincial Natural Science Foundation of China (Grant No. ZR2021MG028), the Shandong Provincial Natural Science Foundation of China (Grant No. ZR2019BG017).
The authors declare that the research was conducted in the absence of any commercial or financial relationships that could be construed as a potential conflict of interest.
All claims expressed in this article are solely those of the authors and do not necessarily represent those of their affiliated organizations, or those of the publisher, the editors and the reviewers. Any product that may be evaluated in this article, or claim that may be made by its manufacturer, is not guaranteed or endorsed by the publisher.
Acharya, V. V., Anginer, D., and Warburton, A. J. (2016). The End of Market Discipline? Investor Expectations of Implicit State Guarantees. SSRN J. doi:10.2139/ssrn.1961656
Biais, B., and Gollier, C. (1997). Trade Credit and Credit Rationing. Rev. Financ. Stud. 10 (4), 903–937. doi:10.1093/rfs/10.4.903
Bollaert, H., Lopez-de-Silanes, F., and Schwienbacher, A. (2021). Fintech and Access to Finance. J. Corp. Finance 68, 101941. doi:10.1016/j.jcorpfin.2021.101941
Buchak, G., Matvos, G., Piskorski, T., and Seru, A. (2018). Fintech, Regulatory Arbitrage, and the Rise of Shadow Banks. J. Financial Econ. 130 (3), 453–483. doi:10.1016/j.jfineco.2018.03.011
Castro, F., Kalatzis, A. E. G., and Martins-Filho, C. (2015). Financing in an Emerging Economy: Does Financial Development or Financial Structure Matter? Emerg. Mark. Rev. 23 (5), 96–123. doi:10.1016/j.ememar.2015.04.012
Chen, H., and Yoon, S. S. (2021). Does Technology Innovation in Finance Alleviate Financing Constraints and Reduce Debt-Financing Costs? Evidence from China. Asia Pac. Bus. Rev. 1, 1–26. doi:10.1080/13602381.2021.1874665
Chen, S., and Zhang, H. (2021). Does Digital Finance Promote Manufacturing Servitization: Micro Evidence from China. Int. Rev. Econ. Finance 76, 856–869. doi:10.1016/j.iref.2021.07.018
Cheung, M. F. Y., and To, W. M. (2021). Effect of Customer Involvement on Co-creation of Services: A Moderated Mediation Model. J. Retail. Consumer Serv. 63, 102660. doi:10.1016/j.jretconser.2021.102660
Choi, W. G., and Kim, Y. (2005). Trade Credit and the Effect of Macro-Financial Shocks: Evidence from U.S. Panel Data. J. Financ. Quant. Anal. 40 (4), 897–925. doi:10.1017/s0022109000002027
Chun, Y. H., and Cho, M. K. (2022). An Empirical Study of Intelligent Security Analysis Methods Utilizing Big Data. J. Logist. Inf. Serv. Sci. 9 (1), 26–35. doi:10.14704/web/v19i1/web19311
Demertzis, M., Merler, S., and Wolff, G. B. (2018). Capital Markets Union and the Fintech Opportunity. J. Financial Regul. 4 (1), 157–165. doi:10.1093/jfr/fjx012
Duchin, R., Gilbert, T., Harford, J., and Hrdlicka, C. (2017). Precautionary Savings with Risky Assets: When Cash Is Not Cash. J. Finance 72 (7), 793–852. doi:10.1111/jofi.12490
Fernandes, A. P., and Ferreira, P. (2021). Executives' Gender Pay Gap and Financing Constraints. J. Econ. Behav. Organ. 192, 381–404. doi:10.1016/j.jebo.2021.10.009
Hadlock, C. J., and Pierce, J. R. (2010). New Evidence on Measuring Financial Constraints: Moving beyond the KZ Index. Rev. Financ. Stud. 23 (5), 1909–1940. doi:10.1093/rfs/hhq009
Heiskanen, A. (2017). The Technology of Trust: How the Internet of Things and Blockchain Could Usher in a New Era of Construction Productivity. Constr. Res. Innovation 8, 66–70. doi:10.1080/20450249.2017.1337349
Janekova, J., Fabianova, J., and Fabian, M. (2019). Assessment of Economic Efficiency and Risk of the Project Using Simulation. Int. J. Simul. Model. 18 (2), 242–253. doi:10.2507/IJSIMM18(2)467
Janekova, J., Fabianova, J., and Kadarova, J. (2021). Selection of Optimal Investment Variant Based on Monte Carlo Simulations. Int. J. Simul. Model. 20 (2), 279–290. doi:10.2507/IJSIMM20-2-557
Kaplan, S. N., and Zingales, L. (1997). Do Investment-Cash Flow Sensitivities Provide Useful Measures of Financing Constraints? Q. J. Econ. 112 (1), 169–215. doi:10.1162/003355397555163
Lannelongue, G., Gonzalez-Benito, J., and Quiroz, I. (2017). Environmental Management and Labour Productivity: The Moderating Role of Capital Intensity. J. Environ. Manag. 190, 158–169. doi:10.1016/j.jenvman.2016.11.051
Lee, I., and Shin, Y. J. (2018). Fintech: Ecosystem, Business Models, Investment Decisions, and Challenges. Bus. Horizons 61 (1), 35–46. doi:10.1016/j.bushor.2017.09.003
Lee, S. Y. (2022). Mobile Digital Forensics Framework to Increase Security Level of for Smartphone User. J. Logist. Inf. Serv. Sci. 9 (1), 68–84. doi:10.33168/LISS.2022.0106
Li, J., Wu, Y., and Xiao, J. J. (2020). The Impact of Digital Finance on Household Consumption: Evidence from China. Econ. Model. 86, 317–326. doi:10.1016/j.econmod.2019.09.027
Li, Y., Tan, J., Wu, B., and Yu, J. (2021). Does Digital Finance Promote Entrepreneurship of Migrant? Evidence from China. Appl. Econ. Lett. 4, 1–4. doi:10.1080/13504851.2021.1963404
Li, K., Xia, B., Chen, Y., Ding, N., and Wang, J. (2021). Environmental Uncertainty, Financing Constraints and Corporate Investment: Evidence from China. Pacific-Basin Finance J. 70, 101665. doi:10.1016/j.pacfin.2021.101665
Lin, Z., Jiang, Y., and Xu, Y. (2011). Do modified Audit Opinions Have Economic Consequences? Empirical Evidence Based on Financial Constraints. China J. Account. Res. 4 (3), 135–154. doi:10.1016/j.cjar.2011.06.004
Luo, S., Sun, Y., Yang, F., and Zhou, G. (2022). Does Fintech Innovation Promote Enterprise Transformation? Evidence from China. Technol. Soc. 68, 101821. doi:10.1016/j.techsoc.2021.101821
Maiti, M. (2021). Quantile Regression, Asset Pricing and Investment Decision. IIMB Manag. Rev. 33 (1), 28–37. doi:10.1016/j.iimb.2021.03.005
Mulier, K., Schoors, K., and Merlevede, B. (2016). Investment-cash Flow Sensitivity and Financial Constraints: Evidence from Unquoted European SMEs. J. Bank. Finance 73, 182–197. doi:10.1016/j.jbankfin.2016.09.002
Nuanmeesri, S. (2021). The Efficiency of Elderly Community Product Using Digital Marketing through Unfolding of Practice during COVID19 Pandemic. J. Syst. Manag. Sci. 11 (4), 65–86. doi:10.33168/JSMS.2021.0404
Park, J., and Kim, S. (2022). Building a Sustainable Digital Supply Chain: The Case of Grab. J. Syst. Manag. Sci. 12 (1), 254–272. doi:10.33168/JSMS.2022.0118
Tsipouridou, M., and Spathis, C. (2014). Audit Opinion and Earnings Management: Evidence from Greece. Account. Forum 38, 38–54. doi:10.1016/j.accfor.2013.09.002
Whited, T. M., and Erickson, T. (2001). On the Information Content of Different Measures of Q. SSRN J. doi:10.2139/ssrn.279315
Yin, Z., Gong, X., Guo, P., and Wu, T. (2019). What Drives Entrepreneurship in Digital Economy? Evidence from China. Econ. Model. 82, 66–73. doi:10.1016/j.econmod.2019.09.026
Keywords: digital inclusive finance, financing constraints, firm heterogeneity, moderated intermediation effect, sustainable development of SMEs
Citation: Li J, Wei R and Guo Y (2022) How Can the Financing Constraints of SMEs Be Eased in China?-Effect Analysis, Heterogeneity Test and Mechanism Identification Based on Digital Inclusive Finance. Front. Environ. Sci. 10:949164. doi: 10.3389/fenvs.2022.949164
Received: 20 May 2022; Accepted: 14 June 2022;
Published: 05 July 2022.
Edited by:
Umer Shahzad, Anhui University of Finance and Economics, ChinaReviewed by:
Zhenji Zhang, Beijing Jiaotong University, ChinaCopyright © 2022 Li, Wei and Guo. This is an open-access article distributed under the terms of the Creative Commons Attribution License (CC BY). The use, distribution or reproduction in other forums is permitted, provided the original author(s) and the copyright owner(s) are credited and that the original publication in this journal is cited, in accordance with accepted academic practice. No use, distribution or reproduction is permitted which does not comply with these terms.
*Correspondence: Jianwei Li, MjAxNTEzMjg3QHNkdGJ1LmVkdS5jbg==
Disclaimer: All claims expressed in this article are solely those of the authors and do not necessarily represent those of their affiliated organizations, or those of the publisher, the editors and the reviewers. Any product that may be evaluated in this article or claim that may be made by its manufacturer is not guaranteed or endorsed by the publisher.
Research integrity at Frontiers
Learn more about the work of our research integrity team to safeguard the quality of each article we publish.