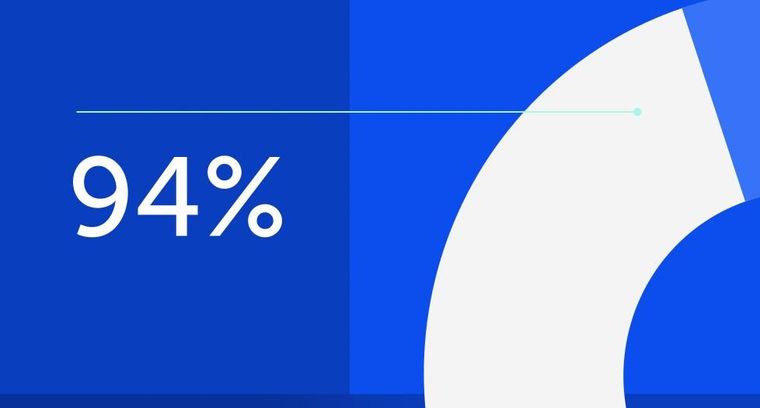
94% of researchers rate our articles as excellent or good
Learn more about the work of our research integrity team to safeguard the quality of each article we publish.
Find out more
ORIGINAL RESEARCH article
Front. Environ. Sci., 15 July 2022
Sec. Environmental Economics and Management
Volume 10 - 2022 | https://doi.org/10.3389/fenvs.2022.947925
This article is part of the Research TopicResource Efficiency and Environmental Impact AssessmentView all 21 articles
The creation of carbon emissions trading markets is a core policy for realizing China’s twin objectives of reaching a peak in CO2 emissions before 2030 and achieving carbon neutrality by 2060. Given that industry is the most significant energy consumer and CO2 emitter, it is imperative to implement carbon reducing initiatives to attain these goals. Following the implementation of carbon emissions trading pilots in China, this article theoretically analyzes the mechanisms of action and paths of influence of China’s carbon trading policies on regional industrial carbon emissions. Then, regarding the trading rights policies launched in 2013 as a quasi-natural experiment, this study uses provincial panel data and industry data from 2003 to 2016 to empirically test the effect of carbon trading on industrial emissions by employing the difference-in-difference and difference-in-difference-in-difference methods. It was found that carbon emissions trading can promote a reduction in regional industrial carbon emissions, achieving the dual aims of reducing total emissions and reducing emission intensity. The reduction effect occurs after the implementation of the carbon trading market policies. The carbon trading policies reduced regional industrial emissions by optimizing regional industrial structures and increasing regional technological innovation. It was also found that reductions in carbon emissions were heterogeneous among industries. These research conclusions will help to improve the top-level design of China’s industrial energy saving and carbon reduction policies and to achieve low-carbon and green industrial development.
Climate change is a global issue that presents a significant challenge to human survival and development. China has emerged as the largest greenhouse gas (GHG) emitter in total annual emissions, to accelerate the pace of GHG emission reduction in China is important to the success of global efforts in addressing climate change (Yang et al., 2022). As an important participant, contributor, and leader in promoting global ecological conservation efforts, China has worked tirelessly to build a “community of human and natural life” through practical action. In 2020, Chinese President Xi Jinping gave an important speech during the general debate of the 75th Session of the United Nations General Assembly in which he emphasized that China will scale up its Intended Nationally Determined Contributions by adopting more effective policies and measures and strive to reach a peak in CO2 emission by 2030 and achieve carbon neutrality by 2060. This This would represent the highest reduction in carbon emission intensity globally and the shortest transition from peak CO2 emission to carbon neutrality in global history. China will need to work very hard to transform its socioeconomic system to achieve these goals. As the most significant contributor to China’s carbon emissions, the industry must fully implement low-carbon and decarbonization strategies, which are key measures of China’s climate governance. Amid this struggle to achieve ambitious emission reductions and reach a peak in CO2 emissions in key industrial sectors as soon as possible, market-based environmental policy tools, such as emissions trading have gained unprecedented importance, especially since China set up carbon emissions trading markets in 2013. The European carbon emission trading system is the largest carbon emission trading market in the world, and the carbon emission trading market based on China, the world’s largest carbon emitter, has great development potential. Therefore, the research on China’s carbon emission trading market is of global significance.
The markets are a major part of a modern environmental governance system. China’s early environmental governance was based on command-and-control environmental policies, but it is widely felt among economists that market-based tools can achieve the internalization of externalities through market transactions and economic incentives. Unlike the existing literature on market-based environmental policies, which mostly looks at emissions trading policies and traditional industrial pollutants, this article focuses more on market-oriented carbon emissions trading policies and industrial carbon emissions reduction, to supplement existing research on market-based tools and emissions reduction. A review of the current literature on market-based environmental policies and carbon emission reduction shows that domestic and foreign scholars have mainly conducted theoretical studies on market-based policies, empirical studies on the effectiveness of carbon emissions trading policies in reducing pollution, and research on the impact of environmental policies on carbon emissions reduction.
Among the theoretical studies on market-based environmental policies, Hahn and Stavins (2011) pointed out that the emissions reduction cost effectiveness of a cap-and-trade system is not impacted by initial allowable allocations, emission source production technology, or the heterogeneity of emission reduction costs, and that it is relatively strong compared to traditional command-and-control environmental policy tools led by the government. Looking at the effectiveness of market-based policies, Schmalensee and Stavins (2019) pointed out that pollution regulation has evolved from sole reliance on command-and-control policies to greater use of emissions trading. Allen et al. (2018). found that market-based environmental policies have significant theoretical advantages, but due to a late start, the current environmental policies of most countries in the world are still command-and-control-based and only supplemented by economic incentive policies. They also note that deficiencies in the economic system and regulatory constraints on enforcement also affect the effectiveness of market-based policy tools in developing countries. Studies, such as those by Bell and Russell (2002) and Kathuria (2006), have pointed out that the effectiveness of economic-incentive environmental regulations is mainly dependent on having a sound market environment and forcibly promoting incentive policies in developing countries may not have the predicted results. Hu et al. (2020) pointed out that China’s relatively weak institutional environment makes the implementation of market-based environmental supervision particularly challenging.
In terms of empirical research on the effectiveness of carbon emissions trading in reducing pollution, Schmalensee and Stavins (2017) pointed out that economic incentives, such as carbon emissions trading, are more effective and economical at controlling pollution than traditional command-and-control methods because of their implementation is more flexible which can encourage enterprises to create innovative emission reduction technologies and processes. Carbon pricing has been hailed as an essential component of any sensible climate policy (Kanamura, 2019). Internalize the externalities, the logic goes, and polluters will change their behavior. Mackellar (2015) suggested that carbon pricing should be a key measure in slowing global warming. Research by Chinese scholars on carbon emissions trading has mainly focused on China’s 2011 proposal to develop carbon markets. According to Tang et al. (2020), the most researched topics in carbon trading literature are the European Union’s Emissions Trading System, the world’s largest carbon market, and China’s regional carbon markets. Most quantitative research on carbon markets discusses price discovery and the effectiveness of carbon markets as financial markets (Joyeux and Milunovich, 2010), price formation and influencing factors (Hammoudeh et al., 2015), and methods of measuring market and policy risk (Blyth and Bunn, 2011), with only a few studies on the effectiveness of carbon trading, as an environmental rights market, at reducing emissions. For example, Anderson and Di Maria (2011) found that the EU’s carbon emissions trading plan reduced emissions by almost 3%, with the majority of the reduction occurring in 15 EU countries. Many empirical analysis results based on G6 countries, BRICs countries and other countries have confirmed that green investment, green technology innovation and renewable energy usage can play a significant role in reducing carbon dioxide emissions (Jia et al., 2021; Su et al., 2021; Xin et al., 2022). Other studies have also focused on the carbon trading market (Ren et al., 2022a; Ren et al., 2022b; Liu et al., 2022; Shi and Xu, 2022).
In contrast, current Chinese literature on the effectiveness of carbon emissions trading in reducing emissions is still relatively small. Most consist of qualitative discussions, empirical discussions, model predictions and simulations, or research on specific industries or regions (Jeris and Nath, 2020; Xu et al., 2022). There are relatively few empirical studies on carbon emissions trading at the national level, but one such study by Li and Lin (2020) pointed out that carbon emissions trading can effectively promote a reduction in carbon emissions as well as reductions in traditional industrial pollutants, such as sulfur dioxide, industrial wastewater, and solid waste, in pilot areas with spillover effects. A study by Cui et al. (2018) found that carbon emissions trading significantly promoted technological innovation among enterprises, especially applications for green patents. Hu et al. (2020) found that compared with other areas, CO2 emissions in carbon trading pilot areas declined by 15.5%, and energy consumption by regulated industries declined by 22.8%.
Regarding research on the impact of environmental policies on carbon emission reduction, because the issue of climate change did not receive widespread attention until the 1990s and because carbon emissions data involves complex calculations (and such data is provided by different international organizations and institutions), many scholars have only begun to pay attention to the role of environmental policies in reducing carbon emissions in recent years. The lack of data means that most existing literature estimates the impact of environmental policies on emissions through models and data simulations. For example, Weng et al. (2018) simulated the impact of different carbon intensity targets on total carbon emissions and economic output. Lin and Jia (2019) established a recursive-dynamic computable general equilibrium (CGE) model to simulate the carbon emission reduction effect of China’s power generation industry emissions trading system.
Very few studies have used carbon emissions data to empirically test the effectiveness of environmental policies in reducing emissions. Zhang et al. (2017) used per capita carbon emissions to measure carbon reduction and found that despite them being relatively new and market mechanisms being imperfect, carbon emissions trading policies are more effective than government command-and-control tools. Other studies have used methods such as decomposition and attribution and scenario simulation to determine the driving factors of carbon intensity, which mainly include energy intensity, emission factors, R&D intensity, and investment intensity.
In summary, Chinese and overseas scholars have conducted many valuable studies on the effectiveness of various environmental policy tools in reducing emissions. However, this author believes that the following two aspects are still urgently in need of research. First, more studies based on the real-life situation in China are required to investigate the effects of China’s carbon trading policies on regional industrial carbon emissions. Much literature ignores the significant differences between China’s emissions trading and its carbon emissions trading policy design. They equate the two and put them both in the basket of market-based environmental policies without distinguishing and analyzing them. In addition, the implementation of market-based environmental policies relies on a sound market environment and incentive mechanisms. In the early stage of using market-based tools in China, the market was not at the level of developed countries, so the results of targeted analysis today may be different from the past. Second, the empirical research on the impact of China’s environmental policies on carbon emission reduction based on scenario simulation and prediction needs to be improved. Due to the lack of official carbon emissions data, simulations and predictions based on theoretical models are more sensitive to minor changes in parameters, which can cause different or even contrary conclusions. Moreover, the economy and government policies are both dynamic, but parameters in simulation models do not capture their dynamism, resulting in model conclusions that do not directly apply to real-life decisions.
Following the research of previous scholars, this article attempts to develop three elements: First is a theoretical and empirical analysis of carbon emissions trading policies, including a summary of the trading policy context, analysis of the role and mechanisms of carbon emissions trading policies in promoting regional industrial carbon emission reductions, and particularly the structural and technical means of reducing emissions. The second is empirical research to identify changes in the regulatory intensity of carbon emissions trading policies and distinguish between the planning and construction period and the formal trading period of the market. This will be more in-depth and specific than previous studies that only looked at regions and years with and without pilot projects. Third, this study uses China’s Carbon Emission Accounts and Datasets (CEADs), which has relatively accurate and up-to-date data on China but which has seldom been used in other studies, to empirically test the effect of carbon emissions trading policies in promoting regional industrial carbon emission reduction and to more comprehensively reflect the level of, and principles behind, carbon emission reduction in China. Analysis in this study covers different provinces and industries and includes the two indicators of total carbon emission reduction and carbon emission intensity reduction. These innovations allow this study to reach conclusions that differ from the existing literature, contributing knowledge to this field. This study uses the most common quasi-natural experiment methods to identify causal effects in evaluating current environmental regulation policies. Therefore, this paper regards China’s carbon emission trading policy launched in 2013 as a quasi-natural experiment to evaluate the effect of environmental regulation policy. In order to comprehensively test the effect of China’s carbon emission trading policy on promoting regional industrial carbon emission reduction, this paper establishes a two-way fixed effect model that uses (DID), difference-in-difference-in-difference (DDD), and other methods to test the impact of carbon emissions trading policies on promoting regional industrial carbon emission reductions, with robustness tests to ensure the reliability of the conclusions.
China began considering establishing carbon emissions trading markets and announced that pilot carbon emissions trading platforms were Shenzhen, Beijing, Tianjin, Shanghai, Chongqing, Guangdong, and Hubei in 2011. Transactions were launched in 2013. According to the China Carbon Emissions Trading Network, the seven provinces and cities involved in the pilot schemes traded 172 million tons of CO2 equivalent between 2013 and 2019, worth a total of 4.3 billion yuan, with both the transaction volume and transaction value increasing year on year. It can be seen from Figure 1 that the trading volume of carbon emission rights in the different cities and provinces was very uneven. As of 2019, most transactions were concentrated in Guangdong (40%) and Hubei (47%). Tianjin and Chongqing, which have the lowest trading volume, only traded 305 and 840 tons of carbon emission rights.
FIGURE 1. Trading Volume of Carbon emission rights in the seven Pilots 2013–2019. Notes: (unit: 10,000 tons of CO2 equivalent). Source: China Carbon Emissions Trading Network.
The total transaction volume across the seven pilot schemes during the first three quarters of 2020 was 23.3 million tons, an increase of 5.77% over the same period the previous year (22.03 million tons). The transaction value was 679 million yuan, a slight decrease (−2.97%) compared to the previous year (700 million yuan). The coronavirus pandemic affected all the pilot schemes, especially in Hubei Province, which resumed trading more than a month (23 March ) after the others. Nevertheless, due to its relatively high daily average transaction volume (9.1 million tons) and value of transactions (250 million yuan), the Hubei pilot scheme retained its leading position among the seven pilot regions, accounting for 38.90% of the total transaction volume and 36.85% of the transaction value. In general, China’s carbon emissions trading markets have not been significantly affected by coronavirus, and they are operating steadily. With the launch of the national carbon market for the power generation industry in June 2021, carbon emissions trading is on the brink of extraordinarily rapid growth.
This paper begins with a statistical analysis of industrial carbon emissions and carbon intensity in pilot and non-pilot areas. The results show that total industrial carbon emissions were higher in both pilot and non-pilot areas during the pilot period (2003–2012) than during the non-pilot period (2013–2016), indicating that economic expansion led to increases in energy consumption and carbon emissions. However, emissions in pilot areas were lower than in non-pilot areas during both the non-pilot period and the pilot period, with a multiplicative relationship between them that expanded from 1.4 times before the pilot to 1.7 times after the pilot. Economic growth in the pilot areas did not bring about a sharp increase in industrial carbon emissions, and industrial carbon intensity in pilot areas declined much faster than non-pilot areas during the pilot period. This tentatively shows that the pilot carbon emissions trading policies may have been effective in reducing regional industrial carbon emissions.
In addition, looking at features and administrative levels of policies and regulations, there are differences in regulatory intensity in different areas. At present, only Beijing, Shenzhen, and Chongqing have formulated local regulations on carbon trading. Other areas mainly manage their carbon markets based on government regulations or departmental documents. This difference in regulatory intensity is transmitted through policies and reflected in the prices and quota-turnover ratio of carbon markets, as shown in Figures 2, 3. The quota-turnover ratio is the ratio of the annual transaction volume of a carbon market to the quota issued for a specific year. It can be seen from the figures that there is a correlation between carbon prices and the quota-turnover ratio. Cities such as Beijing and Shenzhen have relatively high carbon prices and relatively high quota-turnover ratios. According to a 2016 report from the Green Finance Committee’s Carbon Finance Working Group, the criteria for evaluating carbon market development includes resource allocation efficiency (carbon pricing effectiveness) and market operation efficiency (market liquidity) (Yang et al., 2021a; Balezentis et al., 2021; Song et al., 2021; Yao et al., 2021; Zhao et al., 2022).
FIGURE 2. Carbon emissions trading prices of the seven pilots 2013–2020. Source: china carbon emissions trading network.
FIGURE 3. Carbon emissions trading quota-turnover ratios of the seven pilots 2013–2019. Source: china carbon emissions trading network.
Based on the previous, this study proposes the following hypotheses.
Hypothesis 1. Trading carbon emissions rights promotes a reduction in the volume and intensity of regional carbon emissions, and the greater the regulatory intensity, the greater the effectiveness.China’s carbon emissions trading policies can be divided into two time periods: the first is the period of policy planning and local market preparation from 2011 to 2012; the second is the period of formal implementation of the policy from 2013, when trading began. Based on information transmission analysis, it can be inferred that the information received and the expectations formed by the enterprises in these two periods were completely different, and the resulting decisions and behaviors of the enterprises were also different. In the first period, due to China being a large developing country, the value of foreign experience in developing carbon markets was very limited, and policy evolution was relatively slow. As for regulatory intensity, after transactions officially started, companies had to rely on extremely limited information to make judgments and decisions. Some companies adopted a wait-and-see approach and took no action to prevent losses. In the second period, electronic bidding was introduced, which provided real-time changes and announcements of carbon pricing. Companies could obtain timely market supply and demand information such as pricing and transaction volumes in a relatively liquid market. As a result, this study proposes Hypothesis 2.
Hypothesis 2. Carbon emissions trading only reduced industrial carbon emissions following the start of formal market transactions, and reductions increased year on year.The mechanism of action whereby carbon emissions trading policies reduce regional industrial carbon emissions is primarily based on the structural and technical effects of market-based environmental policies.In terms of structural effects, the existence and fluctuation of the market price of carbon emissions trading cause changes in enterprises’ cost-benefit structure, affecting polluters’ behavior. Increases in emission reduction benefits and pollution costs create economic incentives or cost pressures for enterprises. Companies that are expected to emit more than the government quota can choose to purchase the quotas of other companies or seek to limit their emissions through structural adjustments, optimization, and technological innovation. Due to the increased certainty over future costs and benefits, it is possible for companies to conduct effective long-term planning. When companies know that pollution control costs and production costs will rise due to carbon emissions trading policies, they will adjust factors of production based on economic performance and long-term development plans to reduce investment in high-carbon sectors and products while increasing investment in low-carbon sectors and products. This optimizes the efficiency of enterprise resource allocation and the industrial structure of the entire region. The industrial output of pilot industries increases while the output of non-pilot industries decreases, and the output ratio of non-pilot and pilot industries increases. Thus, the industrial structure optimization effect of carbon emissions trading policies promotes a reduction in regional industrial carbon emissions.In terms of technological effects, carbon emissions trading policies enable companies to achieve more effective expectations of the benefits of reducing carbon emissions through green technological innovation and strengthen the economic incentives for enterprises to make technological innovations. Companies can calculate the cost and benefits of reducing emissions and the impact on future economic performance based on their production and operation conditions and the trading volume and price trends of carbon emission rights in the marketplace, which improves the efficiency of corporate decision-making. Therefore, the carbon market encourages enterprises to invest in emission reduction technologies and energy use technologies to achieve technological innovations and low-carbon production and reduce energy consumption and carbon emissions per unit of output value. Moreover, the law of market value plays a fundamental and decisive role in the carbon trading market, which means mandatory supervision by the government is significantly reduced, and the autonomy and flexibility of enterprises in technological innovation are enhanced. Enterprises will also be more willing and motivated to improve production technology and create green innovations to reduce emissions. If the carbon emission rights market operates continuously, effectively, and stably, companies will continue to create technological innovation and achieve regional carbon emission reductions. The mechanisms by which carbon emissions trading policies promote reduced regional industrial carbon emissions are shown in Figure 4.
FIGURE 4. The mechanisms by which carbon emissions trading policies promote reduced regional industrial carbon emissions. This leads to Hypothesis 3.
Hypothesis 3. Carbon emissions trading policies reduce regional industrial carbon emissions by optimizing regional industrial structures and promoting regional technological innovation.In the mechanism of action analysis above, it is stated that carbon emissions trading will optimize local industrial structures, and companies may reduce their investment and production scale in high-carbon industries, investing instead in low-carbon industries, leading to an increase in the output value of low-carbon industries and a decrease in the output value of high-carbon industries. As well as changes to the structures of different industrial sectors, this study also looks at the heterogeneity of reactions to carbon emissions trading policies by industries with different features. In the mechanism of action analysis above, it is stated that carbon emissions trading will optimize local industrial structures, and companies may reduce their investment and production scale in high-carbon industries, investing instead in low-carbon industries, leading to an increase in the output value of low-carbon industries and a decrease in the output value of high-carbon industries. As well as changes to the structures of different industrial sectors, this study also looks at the heterogeneity of reactions to carbon emissions trading policies by industries with different features.Based on this, we propose Hypothesis 4.
Hypothesis 4. The effect of carbon emission rights trading policies in reducing carbon emissions differs by industry, and the effect on high-carbon intensity industries is significantly more significant than for low-carbon intensity industries.
This article regards the carbon emissions trading policy launched in 2013 as a quasi-natural experiment and defines the six provinces (Shenzhen is included in Guangdong Province) in the carbon emission rights pilots as the experiment group, with the non-pilot area as the control group. It uses the difference-in-difference (DID) method to examine the impact of carbon emissions trading in reducing regional industrial carbon emissions. Hu et al. (2020) pointed out that carbon emissions trading pilot areas are geographically spread out in eastern, central, and western regions of China, so they have different economic environments. As the central government determines the pilot areas, they have a top-down nature, so the pilot carbon emissions trading scheme can be regarded as a relatively good quasi-natural experiment.
Based on the above analysis, we constructed a DID two-way fixed effects model:
Where i represents the region, t represents the year, γt represents the fixed effect of the year, μi represents the fixed effect of the area, and εit is the random disturbance term. The explained variable emissionit is the reduction in regional industrial carbon emissions, covering the two indicators of industrial carbon emissions volume and carbon intensity. The key explanatory variable co2t2013 is the DID term. If the coefficient
During the planning and construction period from 2011 to 2012 and the formal implementation period after 2013, there were differences in the regulatory intensity of the carbon emissions trading policies, and there may also have been differences in their effectiveness. To test the impact of different stages and different regulatory intensities, this study identified two stages and selected the annual average carbon trading price indicators and quota-turnover ratio indicators to construct Eq. 2 and Eq. 3.
The data used in this article comes from China Statistical Yearbook and China Statistical Yearbook on Industrial Economy. Carbon emission data comes from China’s Carbon Emission Accounts and Datasets (CEADs). Due to a lack of certain data, industrial sector data runs only to 2016, and data for Tibet is missing. Table 1 shows the descriptive statistics of the main variables. In addition to the variables in the benchmarking and regression, it also shows the descriptive statistics of the two intermediary variables of structural and technical effects in the mechanism verification.
This study used the DID method to establish a two-way fixed effects model for testing the impact of carbon emissions trading policies on regional industrial carbon emissions. The results in Table 2 show that carbon emissions trading led to a significant decrease in regional industrial carbon emissions, simultaneously achieving the dual objectives of reducing carbon volume and carbon intensity. In addition, annual average carbon trading price and allowance turnover are the two indicators of the liquidity and effectiveness of different pilot carbon market The cross-product coefficients of carbon trading, carbon pricing, and the quota-turnover ratio are all significantly negative, which means that the effect of carbon emissions trading is more significant in years when annual average carbon trading prices and the quota-turnover ratio are higher, indicating the importance of increasing regulatory intensity. The coefficients of economic fluctuations indicate that, areas which have higher economic stability enjoy lower carbon intensity while the impact of GDP growth on total emissions are insignificant. This verifies Hypothesis 1.
TABLE 2. Impact of carbon trading on regional industrial carbon emissions: Benchmarking and regression.
We conducted a dynamic effect test to reflect differences in the effects of pilot carbon emissions trading policies in different years. Following the event analysis method framework, a two-way fixed effects model was used in Eq. 4.
Where Dτ is the year dummy variable, ατ is the coefficient being focused on, and the meanings of the other letters are the same as Eq. 1. It can be seen that Eq. 4 sets 2009 as the base year for event analysis, so the specific meaning of the coefficient ατ is whether there is a significant reduction in carbon emissions between pilot provinces and non-pilot provinces in τ compared with 2009. Specifically, if ατ is not significant (the 95% confidence interval includes the null value) before the launch of carbon emissions trading in 2013, a parallel trend is established.
Based on the regression results of Eq. 4 and Figure 5 shows the estimated values of the coefficient ατ from 2010 to 2016 and the 95% confidence intervals. It can be seen that, in the first 3 years of the carbon emissions trading policy, although there were differences in industrial carbon emissions and industrial carbon intensity between pilot and non-pilot areas, these differences are not significant once regional economic factors are controlled, so the parallel trend assumption in the DID method is satisfied. This means that if carbon emissions trading had not started, the carbon emission reduction trends of the two groups (pilot provinces and non-pilot provinces) would be parallel. Moreover, after the launch of carbon emissions trading, reductions in regional industrial carbon emissions grew year on year.
FIGURE 5. The Impact of Carbon Trading on Regional Industrial Carbon Emissions: Dynamic Effect. Note: The vertical lines in the graphs represent the 95% confidence interval of the estimated regression coefficient.
To ensure the reliability of the research results, this paper distinguished between the planning and construction period (2011–2012) and the formal trading period (2013–2016) when examining the impact of the predicted efficacy on the regression results. Prior to the pilot provinces launching formal carbon emissions trading in 2013, the National Development and Reform Commission issued a document in 2011 specifying the seven pilot provinces and cities. This may have enabled companies to form expectations before the official launch of carbon emissions trading and adjust their production and investment behavior accordingly. If there were a systematic difference in their adjusted behavior in pilot provinces and non-pilot provinces, it would lead to bias in the estimation results. To control the impact of enterprise expectations on the research results, this study established Eq. 2 and Eq. 3, and in regression Eq. 1, an interaction term between the pilot provinces and the planning and construction period (2011–2012) is added. The corresponding regression results are shown in Table 3.
Table 3 shows that the regression coefficient of carbon emissions trading barely changed after adding the expectation term during the planned construction period. In addition, the planned construction period coefficient was not significant. This indicates that although the carbon market was in the planning and construction stage in 2011 and 2012, because there were no substantial transactions at this stage and the pilot areas did not issue management documents on the carbon market, it remained unclear which industries and companies would be included in the carbon market and which methods would be used to issue allowances. As a result, at that stage, carbon emissions trading policies did not substantially impact the carbon emissions behavior of companies that adopted a wait-and-see attitude. In 2013, after the carbon emissions trading market was officially launched, relevant policy documents in pilot areas gradually improved. Only then did companies receive information on carbon market pricing and quota-turnover ratios, which they used to adjust their carbon emissions decision-making and behavior accordingly, which led to a reduction in regional industrial carbon emissions. This verifies Hypothesis 2.
In our analysis of the mechanism of action, we stated that carbon emissions trading policies could optimize regional industrial structures (structural effect) and give rise to new energy technologies (technological effect), resulting in a reduction in regional industrial carbon emissions and carbon intensity (Yang et al., 2021b). It can be seen from the results of the benchmarking and regression that carbon emissions trading can significantly reduce regional industrial carbon emissions, but the mechanism of action has not yet been empirically verified. According to Li et al. (2022), there exists some problems in using mediating-effects analysis in causal relationships analysis, however, according to Jiang (2022), we could still use mediating-effects analysis based on the characteristics of our sample and objective of our analysis. Using the intermediary effect method, consisting of a three-step process, this study empirically verified how carbon emissions trading reduces regional industrial carbon emissions.
Tables 4, 5 show the results of the intermediary effect tests. They show that carbon emissions trading can reduce regional industrial carbon emissions by increasing the ratio of the output of non-pilot industries and pilot industries in a region, optimizing the regional industrial structure, and promoting the creation of energy use technologies by industries, thereby reducing energy consumption per unit of output value. Regardless of whether one takes regional industrial carbon emissions or regional industrial carbon intensity as the explanatory variable, after adding the two intermediary variables of structural effect and technological effect, the absolute value and significance of the carbon trading policy coefficient are reduced. The difference is that for the reduction of regional industrial carbon intensity, the structural effect and technological effect both play a role, but for the reduction of regional industrial carbon emissions, the structural effect is not significant. This shows that if the goal is to reduce total regional industrial carbon emissions, the key is to utilize the role of carbon emissions trading to promote regional technological innovation to improve energy efficiency and reduce energy consumption per unit of industrial output value. The result is consistent with Hu et al. (2020). It verifies Hypothesis 3.
TABLE 4. Mechanism verification of the impact of carbon trading on regional industrial carbon emissions.
TABLE 5. Mechanism verification of the impact of carbon trading on regional industrial carbon intensity.
This study used panel data for 35 industries in 30 regions from 2003 to 2016 to analyze industry heterogeneity more accurately to establish a difference-in-difference-in-difference (DDD) fixed effects model.
We first established a DDD model to analyze the impact of carbon emissions trading on industrial carbon emissions and carbon intensity. α1 is the coefficient of the DDD term that this article focused on. If α1 is significantly negative, it means that carbon emission rights trading has effectively promoted a reduction in carbon emissions among industries in pilot provinces. The seven industries covered by the carbon trading pilot are traditional high-energy-consuming and low-efficiency industries in China. Those industries have far more significant difficulties reducing carbon emissions and achieving decarbonization than other industries, which may mean that carbon emissions trading is less effective at reducing carbon emissions in the pilot industries than in average high-carbon industries. Therefore, this article identified two DDD terms: the interaction term of carbon trading DID and pilot industry and the interaction term of carbon trading and high-carbon industry (an industry with carbon intensity above the median). In addition, this study added the individual terms and paired interaction terms of pilot provinces, policy date, and pilot industries/high-carbon industries to the model to control the fixed effects of provinces, industries, and years.
The results of the DDD regression in Table 6 show that carbon emissions trading is significantly more effective at reducing industrial carbon emissions in high-carbon industries than in low-carbon industries. However, there is no evidence that carbon emissions trading reduces carbon emissions more in pilot industries than in other industries.
Could the reason that carbon emissions trading policies had less effect in reducing carbon emissions among pilot industries than among high-carbon industries be because it is easier for non-pilot industries that are high-carbon to reduce their total carbon emissions and carbon intensity? To further analyze the heterogeneity between industries, this study distinguished industries in the carbon trading pilot, high-carbon emitting industries not in the carbon trading pilot, and low-carbon emitting industries and ran a sub-sample regression. The results are shown in Table 7.
Table 7 shows that carbon emissions trading is ineffective at reducing carbon emissions among low-carbon industries. The coefficient is insignificant for reducing the total emission volume of emission intensity. Carbon emissions trading effectively reduced emissions among industries in the carbon trading pilots and high-carbon industries which not in the pilots, but the reduction in industrial carbon emissions among the latter was more significant than among the former. This is consistent with this study’s analysis and the DDD result. During the mechanism verification, we found that both the structural effect and technological effect played a role in reducing industrial carbon intensity, but the structural effect was not significant in reducing the volume of industrial carbon emissions. The structure effect variable in this article is the ratio of the output value of non-pilot industries to the output value of pilot industries. The results of our analysis of industry heterogeneity in this section indicate that carbon emissions trading has a more significant impact on high-carbon industries than low-carbon industries, but the impact on pilot industries is not the most significant among all industries. This verifies Hypothesis 4.
To control environmental pollution, countries use a variety of policy measures in addition to carbon emissions trading. In the benchmarking and regression, this study controlled the influence of government investment in controlling industrial pollution, but the parallel “environmental rights trading” scheme may still influence the estimated results. This study added various regional economic characteristics and the 2007 Chinese government’s policy of expanding pollution trading pilots as a proxy variable for other market-based environmental policies to eliminate the impact of pollution trading on the estimation results. The regression results are shown in columns (1) and (3) of Table 8. It can be seen that after controlling the impact of pollution trading, the carbon emissions trading policy coefficient is still significant, and the absolute value is close to the benchmarking and regression. The impact of pollution trading on reducing regional industrial carbon emissions is insignificant.
TABLE 8. Impact of carbon trading on carbon emissions excluding interference from other environmental policies.
During its 12th Five-Year Plan period (2011–2015), China set a national carbon emission reduction target and assigned carbon intensity reduction targets to 31 regions across the country, and these were continued during the 13th Five-Year Plan period (2016–2020). It should be noted that, in order not to affect overall economic development, China did not set regional targets on total carbon emissions reductions. There were significant differences in carbon intensity reduction targets between regions, ranging from 10% to 19.5% (during the 12th Five-Year Plan period) and 12%–20.5% (during the 13th Five-Year Plan period). Not only did carbon intensity reduction targets become more stringent, but the number of provinces and cities in the highest target level also increased. To control the impact of carbon intensity reduction targets in various regions, this study controlled the reduction target (%) of CO2 emissions per unit of GDP in each region from 2011 to 2016 and regressed the benchmark Eq. 1. The results are shown in columns (2) and (4) of Table 8. They show that after adding carbon intensity reduction targets as a control variable, the absolute value of the carbon emission coefficient does indeed decrease, but it is still significantly negative. In addition, carbon intensity reduction targets significantly reduce regional industrial carbon intensity, but because the targets are mainly aimed at carbon intensity rather than the total volume of regional carbon emissions, the coefficient of the impact of targets on regional industrial carbon emissions is negative with no statistical significance.
To test whether the results of this study are driven by unobservable factors at the province or year level, based on the study by Hu et al. (2020), we conducted a placebo test by randomly assigning provinces as provinces involved in the carbon trading pilots. Specifically, six provinces from 30 were randomly selected as the experimental group. It was assumed that these six provinces implemented the carbon trading pilot and the others were the control group. We performed 400 random samplings, and benchmarking regression was conducted according to Eq. 1. Figure 6 reports the mean value of regression estimates after 400 random allocations. It was found that most estimated coefficients are concentrated near the zero point, the p-values are mostly greater than 0.1, and the regression coefficients are not statistically significant. The true estimated coefficient of the carbon emissions trading policy was an obvious outlier in the placebo test. These results indicate that the probability that the previous estimation results are caused by unobservable province or year factors is very low, so the conclusions of this study are robust.
FIGURE 6. Placebo Test of the Impact of Carbon Trading on Carbon Emissions Excluding Interference. Notes: The X-axis represents the estimated coefficients from 400 randomly assigned carbon trading DID items. The curve is the kernel density distribution of the estimated coefficients. The points are the p-values of the estimated coefficient. The vertical dashed lines are the true estimates, and the horizontal dashed lines represent the 10% significance level.
This study also conducted propensity score matching (PSM)-DID estimation and robustness tests involving a virtual official launch date of carbon emissions trading, the influence of the length of sample interval, the use of substitute variables for industrial carbon emission reduction, the impact of an economic crisis, lagged control variables by one period, DID estimates for two periods, and the influence of space-related factors. The results of all these tests indicate that the conclusions of this study are robust.
This study has produced four main conclusions. First is that carbon emissions trading can significantly promote a reduction in industrial carbon emissions, including the total volume of carbon emissions and carbon emissions per unit of industrial output value (carbon intensity). The empirical results from introducing the annual average price of carbon trading and the quota-turnover ratio show that the higher the intensity of regulation in a given year, the greater the effect of carbon emissions trading policies. Second, during the planning and preparation stage from 2011 to 2012, carbon emissions trading was ineffective, and only after formal trading started in 2013 did regional industrial carbon emissions decline, with reductions growing year on year. Third, carbon emissions trading achieves both environmental and economic benefits by increasing the ratio of the industrial output of non-pilot industries to pilot industries, promoting regional industrial restructuring and energy-related technological innovation, and reducing energy consumption per unit of output value. Moreover, it was found that the structural effect is not statistically significant in reducing regional industrial carbon emissions. Fourth, the effect of carbon emissions trading policies in promoting a reduction in industrial carbon emissions is heterogeneous across industries, as industries with different carbon intensities have different sensitivity to carbon emissions trading policies.
Based on the research conclusions of this study, we propose the following policy recommendations to better utilize the advantages and functions of the market and improve modern environmental governance systems to achieve green, low-carbon development. First, it is recommended to carry out policy innovations in the areas of trading rules, transaction types, participants, and thresholds. This will continuously improve carbon emissions trading policies and create truly effective carbon pricing that will increase the enthusiasm and motivation of enterprises to participate in the market. Our research also shows that ensuring primary market quotas are not exceeded, secondary market prices are effectively transmitted, and there is clear adherence to rules and dispute resolution mechanisms will maximize the efficacy of carbon emissions trading policies. Given the reality that China’s carbon market pricing is far lower than other major international and regional carbon markets, it has a low quota-turnover ratio, and it lacks liquidity, it is important to increase the effectiveness of the carbon market and accelerate the transition from the pilot market to the national unified carbon market by expanding the industry coverage of the national carbon market, formulating a unified system, introducing higher-level and more innovative policies and regulations. The second is to utilize the role of local governments in promoting reductions in regional industrial carbon emissions, particularly focusing on their key role in reducing regional industrial carbon intensity. This study indicates that the structural effect of carbon emissions trading policies is not significant in promoting a reduction in regional industrial carbon emissions and that the reduction effect of carbon trading is slightly greater among high-carbon non-pilot industries than among pilot industries. Therefore, it is even more important to use the power of local governments to introduce supporting systems to maximize the structural effect (structural optimization) and technological effect (energy technology innovations) of carbon emissions trading policies. For example, governments could provide the industries with high carbon intensity and have difficulty decarbonizing with preferential policies, tax incentives, and financial subsidies to guide them to save energy and produce technological innovations. This would fully utilize the government’s synergistic role in reducing industrial carbon emissions. Our third policy recommendation is to use the experience of carbon emissions trading policies to establish an environmental rights trading market. Although emissions trading was introduced relatively early in China, excessive government intervention, strict access, and long compliance periods have meant that companies are not incentivized, so emission reduction effects have been unsatisfactory. Carbon emissions trading policies are based on an independent, enterprise-driven market model with low transaction costs, so to achieve a long-term reduction in regional industrial carbon emissions, government intervention must be gradually reduced, various investment entities should be allowed to participate in transactions, and companies should have clear expectations and economic incentives when joining a carbon emissions trading market. This will ultimately lead to the construction of an environmental rights trading market with a unified framework covering pollution rights and carbon emission rights.
The original contributions presented in the study are included in the article/Supplementary Material, further inquiries can be directed to the corresponding author.
RF, PN, CH, and SJ contributed to conception and design of the study. RF and PN organized the database. CH and SJ performed the statistical analysis. RF, PN, CH, and SJ wrote the first draft of the manuscript. RF, PN, CH, and SJ wrote sections of the manuscript. All authors contributed to manuscript revision, read, and approved the submitted version.
This research was funded by the National Social Science Fund of China (20CJY062).
The authors declare that the research was conducted in the absence of any commercial or financial relationships that could be construed as a potential conflict of interest.
All claims expressed in this article are solely those of the authors and do not necessarily represent those of their affiliated organizations, or those of the publisher, the editors and the reviewers. Any product that may be evaluated in this article, or claim that may be made by its manufacturer, is not guaranteed or endorsed by the publisher.
Allen, B., Zhengyan, L., and Liu, A. A (2018). Efficacy of Command-and-Control and Market-Based Environmental Regulation in Developing Countries[J]. Annu. Rev. Resour. Econ. 10 (1), 381–401. doi:10.1146/annurev-resource-100517-023144
Anderson, B., and Di Maria, C. (2011). Abatement and Allocation in the Pilot Phase of the EU ETS. Environ. Resour. Econ. 48, 83–103. doi:10.1007/s10640-010-9399-9
Baležentis, T., Blancard, S., Shen, Z., and Štreimikienė, D. (2021). Analysis of Environmental Total Factor Productivity Evolution in European Agricultural Sector. Decis. Sci. 52, 483–511. doi:10.1111/deci.12421
Bell, R. G., and Russell, C. (2002). Environmental Policy for Developing Countries. Issues Sci. Technol. 18, 63–70.
Blyth, W., and Bunn, D. (2011). Coevolution of Policy, Market and Technical Price Risks in the EU ETS. Energy Policy 39, 4578–4593. doi:10.1016/j.enpol.2011.04.061
Cui, J. B., Zhang, J. J., and Zheng, Y. (2018). “Carbon Pricing Induces Innovation: Evidence from China's Regional Carbon Market Pilots,” in 130th Annual Meeting of the American-Economic-Association, 453–457. (AEA)).
Hahn, R. W., and Stavins, R. N. (2011). The Effect of Allowance Allocations on Cap-And-Trade System Performance. J. Law Econ. 54, S267–S294. doi:10.1086/661942
Hammoudeh, S., Lahiani, A., Nguyen, D. K., and Sousa, R. M. (2015). An Empirical Analysis of Energy Cost Pass-Through to CO 2 Emission Prices. Energy Econ. 49, 149–156. doi:10.1016/j.eneco.2015.02.013
Hu, Y., Ren, S., Wang, Y., and Chen, X. (2020). Can Carbon Emission Trading Scheme Achieve Energy Conservation and Emission Reduction? Evidence from the Industrial Sector in China. Energy Econ. 85, 104590. doi:10.1016/j.eneco.2019.104590
Jia, S., Qiu, Y., and Yang, C. (2021). Sustainable Development Goals, Financial Inclusion, and Grain Security Efficiency. Agronomy 11, 2542. doi:10.3390/agronomy1109183310.3390/agronomy11122542
Jiang, T. (2022). Mediating Effects and Moderating Effects in Causal Inference. China Ind. Econ. 5, 120–140.
Joyeux, R., and Milunovich, G. (2010). Testing Market Efficiency in the EU Carbon Futures Market. Appl. Financ. Econ. 20, 803–809. doi:10.1080/09603101003636220
Kanamura, T. (2019). Supply-Side Perspective for Carbon Pricing. Quantitative Finance Econ. 3, 109–123. doi:10.3934/qfe.2019.1.109
Kathuria, V. (2006). Controlling Water Pollution in Developing and Transition Countries-Lessons from Three Successful Cases. J. Environ. Manag. 78, 405–426. doi:10.1016/j.jenvman.2005.05.007
Li, S., and Lin, P. (2020). Regional Emission Reduction Promoted by China’s Carbon Emission Trading Scheme: a Difference-In-Differences Analysis with Evidence from Provincial Panel Data. J. Sun Yat-sen Univ. Sci. Ed. 60, 182–194.
Li, W., Yankun, K., Fei, X., and Wei, H. (2022). Comprehensive Framework of Environmental Policy Effects: Empirical Evidence from 16 Pilots Policies (In Chinese). Finance Trade Econ. doi:10.19795/j.cnki.cn11-1166/f.20220408.008
Lin, B., and Jia, Z. (2019). What Will China's Carbon Emission Trading Market Affect with Only Electricity Sector Involvement? A CGE Based Study. Energy Econ. 78, 301–311. doi:10.1016/j.eneco.2018.11.030
Liu, C., Xin, L., and Li, J. (2022). Environmental Regulation and Manufacturing Carbon Emissions in China: a New Perspective on Local Government Competition. Environ. Sci. Pollut. Res. 29, 36351–36375. doi:10.1007/s11356-021-18041-w
Mackellar, L. (2015). Gernot Wagner and Martin L. WeitzmanClimate Shock: The Economic Consequences of a Hotter Planet. Popul. Dev. Rev. 41, 730–731. doi:10.1111/j.1728-4457.2015.00100.x
Ren, X., Duan, K., Tao, L., Shi, Y., and Yan, C. (2022a). Carbon Prices Forecasting in Quantiles. Energy Econ. 108, 105862. doi:10.1016/j.eneco.2022.105862
Ren, X., Li, Y., Yan, C., Wen, F., and Lu, Z. (2022b). The Interrelationship between the Carbon Market and the Green Bonds Market: Evidence from Wavelet Quantile-On-Quantile Method. Technol. Forecast. Soc. Change 179, 121611. doi:10.1016/j.techfore.2022.121611
Sazzad Jeris, S., Nath, R. D., and Deb Nath, R. (2020). Covid-19, Oil Price and UK Economic Policy Uncertainty: Evidence from the ARDL Approach. Quantitative Finance Econ. 4, 503–514. doi:10.3934/qfe.2020023
Schmalensee, R., and Stavins, R. N. (2017). Lessons Learned from Three Decades of Experience with Cap and Trade. Rev. Environ. Econ. Policy 11, 59–79. doi:10.1093/reep/rew017
Schmalensee, R., and Stavins, R. N. (2019). Policy Evolution under the Clean Air Act. J. Econ. Perspect. 33, 27–50. doi:10.1257/jep.33.4.27
Shi, X., and Xu, Y. (2022). Evaluation of China's Pilot Low-Carbon City Program: A Perspective of Industrial Carbon Emission Efficiency. Atmos. Pollut. Res. 13, 101446. doi:10.1016/j.apr.2022.101446
Song, M., Xie, Q., and Shen, Z. (2021). Impact of Green Credit on High-Efficiency Utilization of Energy in China Considering Environmental Constraints. Energy Policy 153, 112267. doi:10.1016/j.enpol.2021.112267
Su, Y., Li, Z., and Yang, C. (2021). Spatial Interaction Spillover Effects between Digital Financial Technology and Urban Ecological Efficiency in China: An Empirical Study Based on Spatial Simultaneous Equations. Ijerph 18, 8535. doi:10.3390/ijerph18168535
Tang, L., Wang, H., Li, L., Yang, K., and Mi, Z. (2020). Quantitative Models in Emission Trading System Research: A Literature Review. Renew. Sustain. Energy Rev. 132, 110052. doi:10.1016/j.rser.2020.110052
Weng, Z., Dai, H., Ma, Z., Xie, Y., and Wang, P. (2018). A General Equilibrium Assessment of Economic Impacts of Provincial Unbalanced Carbon Intensity Targets in China. Resour. Conservation Recycl. 133, 157–168. doi:10.1016/j.resconrec.2018.01.032
Xin, L., Sun, H., Xia, X., Wang, H., Xiao, H., and Yan, X. (2022). How Does Renewable Energy Technology Innovation Affect Manufacturing Carbon Intensity in China? Environ. Sci. Pollut. Res. doi:10.1007/s11356-022-20012-8
Xu, S., Yang, C., Huang, Z., and Failler, P. (2022). Interaction between Digital Economy and Environmental Pollution: New Evidence from a Spatial Perspective. Ijerph 19, 5074. doi:10.3390/ijerph19095074
Yang, C., Li, T., and Albitar, K. (2021a). Does Energy Efficiency Affect Ambient PM2.5? The Moderating Role of Energy Investment. Front. Environ. Sci. 9. doi:10.3389/fenvs.2021.707751
Yang, X., Su, X., Ran, Q., Ren, S., Chen, B., Wang, W., et al. (2022). Assessing the Impact of Energy Internet and Energy Misallocation on Carbon Emissions: New Insights from China. Environ. Sci. Pollut. Res. 29, 23436–23460. doi:10.1007/s11356-021-17217-8
Yang, X., Wang, W., Wu, H., Wang, J., Ran, Q., and Ren, S. (2021b). The Impact of the New Energy Demonstration City Policy on the Green Total Factor Productivity of Resource-Based Cities: Empirical Evidence from a Quasi-Natural Experiment in China. J. Environ. Plan. Manag., 1–34. doi:10.1080/09640568.2021.1988529
Yao, Y., Hu, D., Hu, D., Yang, C., and Tan, Y. (2021). The Impact and Mechanism of Fintech on Green Total Factor Productivity. Green Finance 3, 198–221. doi:10.3934/gf.2021011
Zhang, Y.-J., Peng, Y.-L., Ma, C.-Q., and Shen, B. (2017). Can Environmental Innovation Facilitate Carbon Emissions Reduction? Evidence from China. Energy Policy 100, 18–28. doi:10.1016/j.enpol.2016.10.005
Keywords: carbon emissions trading policies, industrial carbon emission reduction, global climate governance, function mechanism, difference-in-difference
Citation: Feng R, Lin P, Hou C and Jia S (2022) Study of the Effect of China’s Emissions Trading Scheme on Promoting Regional Industrial Carbon Emission Reduction. Front. Environ. Sci. 10:947925. doi: 10.3389/fenvs.2022.947925
Received: 19 May 2022; Accepted: 21 June 2022;
Published: 15 July 2022.
Edited by:
Yin Long, The University of Tokyo, JapanReviewed by:
Gui Jin, China University of Geosciences, ChinaCopyright © 2022 Feng, Lin, Hou and Jia. This is an open-access article distributed under the terms of the Creative Commons Attribution License (CC BY). The use, distribution or reproduction in other forums is permitted, provided the original author(s) and the copyright owner(s) are credited and that the original publication in this journal is cited, in accordance with accepted academic practice. No use, distribution or reproduction is permitted which does not comply with these terms.
*Correspondence: Shuaishuai Jia, dG9uZ2ppamlhQGd6aHUuZWR1LmNu
Disclaimer: All claims expressed in this article are solely those of the authors and do not necessarily represent those of their affiliated organizations, or those of the publisher, the editors and the reviewers. Any product that may be evaluated in this article or claim that may be made by its manufacturer is not guaranteed or endorsed by the publisher.
Research integrity at Frontiers
Learn more about the work of our research integrity team to safeguard the quality of each article we publish.