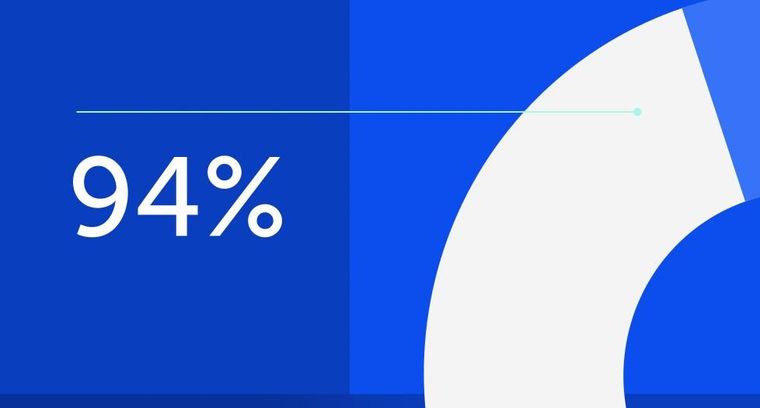
94% of researchers rate our articles as excellent or good
Learn more about the work of our research integrity team to safeguard the quality of each article we publish.
Find out more
ORIGINAL RESEARCH article
Front. Environ. Sci., 17 August 2022
Sec. Environmental Economics and Management
Volume 10 - 2022 | https://doi.org/10.3389/fenvs.2022.945968
This article is part of the Research TopicClimate Change Information for Regional Impact and Risk AssessmentView all 10 articles
Climate change, which is mainly caused by carbon emissions, has attracted attention worldwide. With the continuous increase in temperature, the urban heat island effect, extreme weather, and water shortages have seriously affected the urbanization process. Through an empirical analysis of panel data from 28 provinces in China from 2006 to 2018, this study examines the impact of climate change-induced temperature changes on the urbanization of China’s population. The results show that the urbanization level has a significant double-threshold effect on the impact of temperature on urbanization. When the urbanization level crosses the corresponding threshold value, the negative impact of temperature on urbanization is relatively weak. Understanding the impact of climate change on urbanization has become increasingly important as climate warming increases. On the one hand, the climate issue has always been a topic of common concern around the world. On the other hand, studying how climate change affects population urbanization is conducive to identifying the human factors that affect climate change and proposing corresponding solutions. Simultaneously, it also provides a reference for optimizing the distribution of urban and rural populations, and can comprehensively consider the relationship between climate change and urbanization in the formulation and implementation of policies. On this basis, the Chinese government should strengthen financial support for climate change, play a leading role in policies, improve the urban layout and structure, and increase the resilience of cities to climate change.
Under global warming, extreme weather conditions have become more and more frequent. By 2010, the area affected by extreme heat waves in the world had tripled since 2000 (Zampieri et al., 2016), and by 2018, the frequency and magnitude of extreme high-temperature weather in parts of China had almost doubled (He et al., 2022). Since its reform and opening up, large-scale industrialization and sustained and rapid economic growth have enabled China to experience the largest and fastest urbanization process in the world. According to data from the National Bureau of Statistics of China, China’s urban population accounted for 60.9% of the total population as of 2019. According to the 2018 United Nations Urbanization Development Outlook, 55% of the global population currently lives in urban areas, and it is estimated that this ratio will reach 68% by 2050. It is indisputable that large-scale climate change and urbanization on a global scale are prominent issues that the global population must face. With the vigorous development of the economy and the promotion of urbanization, the pursuit of living standards is no longer limited to the satisfaction of basic conditions, such as food, clothing, and warmth, but more to the common development of material conditions and the spiritual world. As the most populous country in the world, and in the process of continuously improving the level of urbanization, various environmental problems are more serious than those in Western countries, particularly the lack of green space per capita caused by a large population density. Climate change caused by human activities has also brought multiple problems to natural systems and urban development (Field et al., 2014). China’s carbon dioxide emissions rank first in the world, accounting for 29% of global added value. Natural disasters caused by climate change are becoming increasingly serious. Global warming has caused the melting of glaciers, accelerated evaporation of water resources, and destruction of ecological balance. At present, due to the rising temperature, environmental pollution and deterioration of air quality in certain urban areas of China, there has been a phenomenon of urban population transfer to suburbs, small and medium-sized cities, or rural areas.
Under the influence of climate change, the temperature in Northeast China is increasing annually in winter (Zhou et al., 2020). The most direct reason for the increase in temperature is the increase in greenhouse gases, particularly carbon dioxide emissions. Some scholars believe that urbanization promotes carbon emissions (Huo et al., 2020), but while human socioeconomic activities lead to climate change, climate change also affects human socioeconomic activities, and there is considerable interaction between socioeconomic and climate systems (Karl et al., 1988). The heat island effect brought about by the process of urbanization (Shepherd and Burian, 2003) and the uneven distribution of precipitation will have a severe impact on the local climate (Matsumoto, 2019). The impact of climate change on people’s lives is also large, which may lead to nonlinear changes in crop yield (Chen et al., 2016), affect electricity demand (Fan et al., 2019), reduce labor productivity in artificial production caused by changes in ambient temperature, and cause substantial economic losses (Cai et al., 2018). According to reports by the International Energy Agency and the Environment Agency, under the influence of COVID-19, carbon emissions worldwide have been significantly reduced in a short period of time, and China’s air quality has significantly improved (Wang et al., 2021). As various industries have been severely impacted by the epidemic, multiple companies face the risk of bankruptcy. When the new crown epidemic has eased, its energy use and greenhouse gas emissions in the process of recovery of industrial production and manufacturing will very likely exceed that before the outbreak (Wang and Su, 2020). If a city’s system to deal with climate change is imperfect, it is likely to lead to a sharp decline in air quality, affecting the physical and mental health of residents, thus affecting the level of urbanization. Frameworks for understanding the resilience of cities to the impacts of climate change are still developing (Davoudi et al., 2012; Silva et al., 2012), and cities are still struggling to adapt their policies and institutions to mitigate the adverse impacts of climate change. The spatial distribution pattern of the population will be deeply affected by factors such as resource and environmental constraints and economic and industrial guidance. Currently, China’s urbanization process and population density are showing serious regional imbalances, and the eastern coastal areas have formed a large-scale economy. However, urbanization levels in the central and western regions were relatively low. Although regional differences in population urbanization are closely related to regional economic development, differences in climate and the environment also have an important impact on the process of regional urbanization. China should attach great importance to the environment and air quality in the economic development process in order to achieve sustainable development and create a green economy (Chai et al., 2021).
Most studies on climate change have focused on agricultural production and carbon emissions. Few scholars have explored the impact of climate on labor mobility and urbanization. Therefore, this study conducts an empirical analysis on whether climate change can affect China’s urbanization, which is expanding perspectives in the field of climate change, and how rational urban planning can improve resilience to climate change. On the one hand, this paper discusses the impact of climate driving on the urbanization level of different Chinese provinces from the perspective of temperature change, which further enriches the research content of climate change. On the other hand, this study first uses the maximum and minimum temperatures as independent variables to improve robustness, and then constructs a GMM regression model, which effectively solves the problem of endogenous variables. In addition, a threshold regression method was used to study the possible nonlinear relationship between climate change and urbanization levels.
The effects of climate change are manifold. Climate change is an important topic worldwide, and some recent studies have considered the interaction between socioeconomic and climate systems in climate change research (Monier et al., 2018). At the macro level, Nordhaus summarized a large number of macroeconomic studies on the different impacts of climate change, such as damage to agriculture, coastal areas, amenity values, biodiversity, and human health (Nordhaus, 1997). A city is a whole of “society-ecology-technology,” composed of closely connected parts, driven and contributed by social, ecological, and technological forces (Monstadt, 2009). When any of these factors change, it will have an impact on urban development and even economic growth. For example, high temperatures will cause economic losses (Rezai et al., 2017; Nagy et al., 2018), and the relationship between GDP loss and global average temperature increase is roughly linear (Jun’Ya et al., 2017). The adverse effects of climate change on urbanization mainly manifest as changes in energy consumption (Giannakopoulos and Psiloglou, 2006), increased mortality and spread of infectious diseases (Alcoforado et al., 2015), coastal city safety issues (Takagi et al., 2016), infrastructure damage (Huong and Pathirana, 2013), and water scarcity (Kummu et al., 2010). At the micro level, climate change will impact the health and mentality of the individual labor force, thus affecting working hours and efficiency (Xiang et al., 2014; Li et al., 2016). Notably, the impact on outdoor workers, such as agricultural workers, is greater than that of indoor workers (Kjellstrom et al., 2009), because the most direct impact of climate change on human health is through the thermal effect of carbon emissions. According to the Intergovernmental Panel on Climate Change forecast, the global average temperature is expected to increase by 2°C by 2100. In the report “Cities and Climate Change” published by the Organization for Economic Co-operation and Development, it is pointed out that compared with rural areas, there is a large amount of concrete and asphalt in cities, as well as equipment such as factory machines, household appliances and urban lighting of residual heat. At the same time, the large area of urban buildings destroys the urban greenspace ecosystem and further aggravates the urban heat island effect. Climate change alters the composition of chemical pollutants in the atmosphere, potentially causing air pollution, particularly in densely populated areas, and adversely affecting urbanization. Based on this, Hypothesis 1 was proposed.
H1. Climate change has a negative impact on population urbanization.The current political environment in the majority of countries emphasizes economic growth, and cities are under pressure to contribute to national development and innovation processes (Bettencourt et al., 2007; Shearmur, 2012). The level of urban development is crucial for a country’s economic growth. In addition to industrialization and policy factors, economic growth and structural adjustment, especially trade opening, are the main drivers of urbanization in China (Zhang, 2002). According to the Paris Agreement, countries have made commitments to control carbon emissions. However, if reducing carbon emissions requires sacrificing economic growth, the motivation and efforts of countries to commit to reducing emissions will be greatly reduced (Wang and Zhang, 2021). Simultaneously, to reduce the spread of COVID-19, many countries have implemented foreign trade restrictions. Therefore, the rise of trade protectionism has brought new challenges to carbon emission reduction in various countries. The world is undergoing a significant urbanization process, while China has made great achievements in urbanization since the reform and opening up (Yy et al., 2019), whose speed and scale are much higher than those of other countries in the same period (Wang and Li, 2019). However, the environmental problems brought about by the extensive economy have gradually emerged, and China is facing enormous pressure to control environmental pollution. Although the government has strengthened its control of environmental pollution by promulgating a series of policies and regulations, there is still a long way to go to control environmental pollution in China (Song et al., 2020). With the further improvement of the urbanization level and the rise of temperature, environmental pollution is bound to become an important factor affecting the health of urban residents (Reiner et al., 2015), which will have an adverse impact on urbanization. In recent years, urbanization in China has been increasing rapidly, however, the problem of the urban population not increasing simultaneously has received a lot of attention. Previous studies have examined the relationship between urbanization, environmental pollution, and residents’ health. The impacts of climate change on urbanization are increasingly evident, as urban liveability is becoming vulnerable to extreme weather conditions, such as persistent heatwaves and flooding. Cities provide 80% of jobs worldwide and have long been the main drivers of social innovation and wealth creation, however, cities consume more than 3% of the planet’s total resources. Half and three-quarters of total greenhouse gas emissions (Xu et al., 2021). Air pollution and anthropogenic heat from urbanization adversely affect physical and mental health (Michail et al., 2013; Mueller et al., 2017). Because the income level of urban residents is generally higher than that of rural areas, they can more easily choose to live in other areas with a better natural environment when they suffer from environmental problems caused by climate change. The cost of relocation leads to the creation of an environmental poverty trap, which in turn affects the promotion of urbanization. Concurrently, due to the vast territory of China, various climatic and environmental problems caused by urbanization will not be effectively solved due to differences in the regional economy and natural environment, resulting in the emergence of urban populations in some areas, to suburbs, small and medium-sized cities, and rural areas. This phenomenon of transfer is called “counter-urbanization”. Chinese researchers have explored “counter-urbanization” according to the specific situation in China’s urbanization process. Their results show that, although counter-urbanization has not yet fully arrived in China, residents’ concerns about their physical health caused by external adverse conditions, such as environmental pollution and traffic congestion, may affect the influx of the labor force into cities (Wang et al., 2019). For China, which is still in the development stage, the slowdown of urbanization will weaken the driving role of its political and cultural center, which is detrimental to the overall development of the country (De Matteis, 1986). With the increasing pressure of climate change and urban problems, urban functions may be decomposed into livable small- and medium-sized towns and villages, and the urban population will flow to the suburbs and rural areas. However, as provinces with a high level of urbanization have a more complete infrastructure, urban planning may be relatively reasonable, and the scale of the tertiary industry that can attract talent is growing on this basis, therefore, the impact of climate may be different. Simultaneously, owing to temperature differences in different regions, not all regions suffer economic losses due to climate change. Owing to the relative advantages brought about by differences in labor productivity changes between different regions, some regions in medium- and low-temperature areas have achieved positive economic benefits (Matsumoto, 2019). According to the EKC curve hypothesis, environmental quality first declines with economic growth and then gradually improves after economic growth reaches a certain level, showing an inverted U-shaped trend (Renzhi and Baek, 2020; Liu and Lai, 2021). Economic growth and climate change may lead to changes in urbanization, the effects of which may be nonlinear. The three dimensions of sustainable development mentioned in the “2030 Agenda for Sustainable Development” are social, economic, and environmental (Nations, 2015), and they interact with each other. Urbanization, as an important factor at the social level (Yao et al., 2021), will inevitably have an impact on urbanization when climate changes, and ultimately affect the relationship between economic and ecological footprints (Wang et al., 2022). Therefore, Hypothesis 2 is proposed.
H2. Climate change has a nonlinear effect on urbanization. When the urbanization level is greater than the threshold, the negative impact of climate change on urbanization weakens.Although the scope of this study is similar to that of the existing literature, it contributes to the existing literature in several respects. First, the existing literature mainly studies the relationship between the economy and the environment and seldom examines social factors. This study examines urbanization, an important indicator of the social dimension, and improves relevant research in the field of sustainable development. Second, as temperature is the most intuitive feeling of climate change, this study adds the highest and lowest temperatures on the basis of average temperature, which improves the robustness of the research; alternatively, it can enable the government to utilize more ways to analyze the specific causes of climate change and provide a basis for formulating policies related to environmental protection and urbanization development.
Urbanization is the phenomenon or process of population concentration in cities or urban areas and an increase in the density of urban settlements within a given territory. As a process of population migration, first, there is an increase in the proportion of the population living in all urban areas. Second, this proportion of the population is increasingly concentrated in larger urban settlements (Gu, 2019). This is accompanied by migration of the population from rural to urban areas, and rural areas gradually evolve into urban areas. China is the most populous country in the world, and there is a very significant gap between the rich and the poor in the East and the West, which will lead to greater population mobility. Due to the distribution of climate stations and lack of data, this study used panel data of 28 Chinese provinces from 2006 to 2018. Climate data from 28 provincial-level weather stations in China, and other data were obtained from China’s National Bureau of Statistics. A base climate station was established with the approval of the local land administration department, the urban and rural construction and environmental protection department, and the National Meteorological Bureau after surveying the site and proposing a plan by the provincial meteorological bureau. The distribution of the base climate stations is based on the distribution of climate zones in China and is determined by the size of the climate zones and spacing requirements of the base climate stations. There is a certain distance requirement according to China’s terrain, climate, and other circumstances. The base climate station makes hourly climate observations and submits meteorological records to national and provincial meteorological administrations. Therefore, we used the mean temperature of the climate station located in the province to represent the annual mean, maximum, and minimum temperatures of the province.
The annual mean, maximum, and minimum temperatures of the climate station located in the province were used to represent climate change.
In this study, the ratio of the permanent urban population to the total resident population of the region, namely the urbanization rate, was used as a substitute index of the urbanization level as the dependent variable.
According to Wu et al. (2020), the control variables are the average wage of urban workers, GDP growth rate, natural population growth rate, foreign direct investment, proportion of tertiary industry, and real estate development investment. Table 1 presents a more intuitive description of the variables.
Before panel threshold regression, random- and fixed-effect models were used for regression analysis. To ensure the robustness of the equation, the annual maximum and minimum temperatures were added to the regression.
Because of inertia or partial adjustment, an individual’s current behavior depends on past behavior. In the panel model, the dynamic panel model takes the lag of the dependent variable as the independent variable and considers the lag effect of the dependent variable (Alvarez and Arellano, 2003). That is, the impact of the urbanization process is temporal, and the climate impact of the current urbanization process is at the beginning of the next stage; therefore, this study adopts a dynamic panel model. To prevent the endogeneity problem, the SYS-GMM model was constructed to investigate whether there is a linear relationship between climate change and urbanization level. According to Wu et al. (2019), urbanization may be affected in the early stages. Therefore, Ur-1 was added to the model as an independent variable to obtain the benchmark model for this study.
Threshold regression refers to selecting a variable as the threshold variable, dividing the regression model into multiple intervals according to the threshold, classifying the samples after regression, and comparing the coefficients of different intervals (Wang and Wang, 2020). The panel threshold data model proposed by Hansen. (2000) implements parameter estimation and hypothesis testing of the threshold values using strict statistical inference methods. When the climate changes, particularly when the temperature increases due to greenhouse gas emissions, weather problems such as the heat island effect and uneven spatial distribution of precipitation will have adverse effects on urbanization. When urbanization reaches a certain height, the ability of cities to deal with the harm caused by climate change gradually improves, therefore, the adverse impact of climate change on the urbanization process will be alleviated. Therefore, in this study, a panel threshold data model was used to reveal the nonlinear influence of temperature on urbanization. In contrast to the past, this study considers urbanization as the threshold variable, which is also the explained variable in the model. The panel threshold model of the nonlinear correlation between temperature and urbanization was constructed as follows:
where i represents province; t represents year;
Table 2 presents the descriptive statistics. It can be seen from the table that the maximum urbanization level of the sample provinces is 89.600 and the minimum is 53.010, indicating a large gap in urbanization level across the country. The maximum annual average temperature is 25.380, the minimum is −3.060, the maximum annual maximum temperature is 44, and the minimum temperature is −42.800. Thus, owing to the large latitude in China, the annual average temperature of each province is very different.
The GMM is a common parameter estimation model. Arellano proposed the first-order difference GMM method and has continuously improved it to form a system GMM, which can solve the shortcomings of the estimation of the endogeneity problem of the original variables. By combining the horizontal and difference regression equations, the lag level was used as the instrumental variable of the first-order difference, and the first-order difference was used as the instrumental variable of the horizontal variable to obtain an unbiased estimation result. In this study, a systematic GMM with a lag term was established to study the impact of temperature change on urbanization. The unit root test is an important method for determining the stationarity of variables, which is the premise of time series modeling. Since most macroeconomic variables are non-stationary, their stability of economic variables must be tested before further analysis (Wu et al., 2019). As a test method for panel data, the unit root test was proposed by Levin and Lin (Andrew et al., 2002), who relaxed the original assumption of independence and the same distribution, proposed a test method of independence and different distributions, and allowed the existence of heteroscedasticity.
LLC and IPS tests were used to test the stability of the relevant variables. From Table 3, we can see that all relevant variables reject the 1% unit root hypothesis.
From Table 4, it can be concluded that the VIF test is performed on the variables, and there is no multicollinearity among the variables, indicating that the regression results are reasonable and valid.
At the same time, in order to test the possible cross-sectional correlation problems in the process, this paper adopts the CD test to analyze the data.
From the data results in Table 5, it can be seen that each CD test statistic rejects the null hypothesis that the cross-sectional units are independent of each other at the 1% significant level. CIPS (Second Generation Panel Unit Root Test) test. It can be seen from Table 6 that each variable rejects the 1% unit root hypothesis, indicating that the experimental results are stable.
Based on the results in Table 7, we used the fixed- and random-effect models to conduct regression analysis on average, annual maximum, and annual minimum temperatures, and concluded that air temperature was negatively correlated with urbanization, which was significant at the 1% level, which improved the robustness to a certain extent. According to the model R-squared, it can be judged that the interpretation results of the fixed effect model and the random effect model are valid, the results of the fixed effect are stronger than the random effect, and the fitting effect is better. In the subsequent threshold regression results, the R-squared value is also relatively high.
As shown in Table 8, regarding the validity of the tool variables, the model selected the Hansen test as the over-identification constraint test. The model utilizes the observed values of the threshold variables to estimate the appropriate threshold, thus avoiding the insufficiency of subjective judgment partitions and yielding more accurate results (Shao et al., 2022). The model also verifies whether the residual term is autocorrelated with the first- and second-order sequences by judging the p-value of AR (He et al., 2022). It can be seen from the results in Table 5 that the Hansen test cannot reject the null hypothesis and the p-value of AR (He et al., 2022) is large, indicating that the tool variables are not over-identified and there is no second-order sequence correlation between the correlation estimation equation. Therefore, the use of the Hansen test in this study is sufficient to demonstrate the reliability of the results, indicating that the regression results of the system GMM estimation method adopted in this study are reliable and effective.
The regression coefficients of the explained variables are significantly negative during the lag period, indicating that urbanization is significantly affected by the lag period. From the regression model of the national sample, the average, maximum, and minimum temperatures all have a significant negative correlation effect on urbanization level, which proves that the result is robust, indicating that temperature will hurt China’s urbanization in China. This shows that, in the face of climate change, China’s urban problems need to be further rationally planned to reduce the potential urban problems caused by climate or environment, thus limiting economic growth. Thus, Hypothesis 1 is verified.
According to the results in Table 9, urbanization threshold variables all pass the single and double thresholds at a significance level of 1%.
It can be seen from Table 10 that the thresholds of urbanization for the average temperature are 38.500 and 49.380.
As shown in Table 11, when the urbanization level was <38.50, the increase in average temperature had the greatest negative effect on urbanization, with a correlation coefficient of −0.906. When the urbanization level was >38.50 and ≤49.38, the negative impact degree decreased to −0.496. When the urbanization level was >49.38, the influence intensity further decreased to -0.119. All these passed the 1% significance level test. It can be seen that increasing temperatures will promote the occurrence of de-urbanization; however, with the increase in urbanization level, the impact of temperature decreases. This may be because places with high levels of urbanization have relatively complete infrastructure and are less affected by climate. However, when urbanization is less than a certain level, the cost of leaving the city may be relatively low; therefore, people are more willing to flee the city. Therefore, temperature had a greater negative impact on urbanization in this case. Simultaneously, climate change has also had a great effect on the development of the economy; on the one hand, China’s carbon dioxide emissions increased by 29% of the world’s increase, although on the issue of greenhouse gas emissions, China presented a highly responsible attitude to the world, in the international community, particularly in developed countries. The highly developed economies that developed countries enjoy today have gone through a process of high carbon dioxide emissions. China’s PER capita emissions of carbon dioxide are now at the level of Britain and the US at the beginning of the 20th century; China’s PER capita GDP only reached the level of some developed countries in 1960. These numbers indicate that emissions are a developmental problem. If emissions are cut on a large scale, China faces a large-scale reform of its energy structure, and it is doomed to spend a lot of manpower material resources; on the other hand, climate change leads to frequent extreme weather to a certain extent, the temperature increase will warm the ocean, resulting in a large amount of water vapor, which will result in more frequent rainfall and snowfall, and an increase in rainfall will result in flooding. As a result, buildings and infrastructure will be damaged, as floods also cause great disturbance to economic activities, and thus adversely affect economic development.
First, the stochastic effect model, fixed effect model, and systematic GMM regression analysis show that temperature will negatively affect urbanization, and the same conclusion is reached by adding annual maximum and minimum temperatures to improve robustness. Second, by introducing urbanization as a threshold variable, the panel threshold model was used to study the impact of temperature on urbanization. We concluded that Hypothesis 2 is satisfied. When the urbanization level was lower than the first threshold of 38.50, the increase in average temperature had a significant negative effect on urbanization. When the urbanization level between the two thresholds was >38.50 and ≤49.38, the negative impact degree decreased. When the urbanization level was greater than the second threshold value of 49.38, the negative impact caused by temperature further decreased, and all of the above passed the significance level test of 1%. A possible reason for this phenomenon is that, when the level of urbanization is low, the infrastructure construction is not suitable, and the city does not have sufficient strength to quickly and effectively formulate countermeasures in the face of environmental damage caused by temperature changes. When the level of urbanization reaches a certain level, the economic strength of the city itself is relatively strong, and the ability of the city to cope with climate change has been strengthened, resulting in a decrease in the negative impact of temperature change on urbanization. Increasingly high, the city’s ability to deal with risks and related system construction will be further improved, therefore, the negative impact of temperature on urbanization will be further reduced.
The current understanding of the relationship between climate change and urbanization is still in the exploratory stage. Climate change is continuously affected by urbanization and other human activities, and climate change impacts urbanization and human activities. The timetable for China to achieve its carbon neutrality goal by 2060 has been clarified, and it is necessary to formulate a roadmap for a set of concrete plans to achieve this goal. In-depth exploration of the interaction, impact mechanism, and implementation path of climate change, carbon emissions, and urbanization are important aspects for realizing a green economy and sustainable development. This study examined the relationship between climate change and population urbanization in China. On the one hand, the average, maximum, and minimum temperatures were selected for research on the measurement of temperature, and economic factors were considered in the selection of control variables, which enriched the research content of the sustainable development agenda. On the other hand, it can make the government pay more attention to observing the most intuitive manifestations of climate change, explore its causes, and take relevant measures to solve environmental problems in a timely manner.
Urbanization is an inevitable choice for society to deal with climate change, and is also an important stage of economic development. Establishing environmental awareness, building green communities, developing a low-carbon and innovation-driven economy, and advocating sustainable social concepts such as moderate consumption are ways to achieve climate-resilient cities and sustainable human development goals. This study makes recommendations from three aspects: urban planning, innovative development, and narrowing the urban-rural gap.
First, optimize urban planning and promote a combination of ecology and urban construction. China’s urban planning to address climate change focuses mainly on the systematic and hierarchical construction of low-carbon urban planning frameworks to explore mitigation and adaptation technologies to address climate change. Given the increasingly prominent contradiction between climate change, human activities and environment, ecology, and resource carrying capacity, megacities and urban agglomerations need to realize harmonious coexistence between nature and humans and sustainable development of the regional economy through urban planning and construction. It is necessary to evaluate the climate and environmental effects of urban planning implementation, provide scientific support for the construction of a green and livable environment, adjust the relationship between urban development and the ecological environment, and maximize the social and economic benefits. Second, strengthen innovation capacity. Technological innovation forms the basis for developing zero-carbon energy and improving energy efficiency. Therefore, technological progress can fundamentally solve the problems of emissions and climate change. Third, pay attention to balanced development between urban and rural areas and narrow the income gap between urban and rural areas. The government can release preferential measures to encourage the development of township enterprises, encourage farmers to work in cities, and promote the construction of small towns. Finally, popularize energy conservation education. The state should vigorously increase publicity and education in energy conservation, incorporate energy conservation knowledge into the national education and training system, popularize scientific knowledge of energy conservation, arouse the public’s sense of crisis and urgency in energy conservation and environmental protection, create an atmosphere of public opinion to save resources and slow global warming, attach great importance to energy conservation and emission reduction, and make the public truly realize the importance of energy conservation and emission reduction.
Although this study uses an econometric model based on panel data to explore the impact of climate change on urbanization in China, it still has certain limitations. For example, annual data do not fully reflect the impact of seasonal climate fluctuations on urbanization. Therefore, we will attempt to collect more detailed data in the future to address these issues. Simultaneously, there are multiple factors that affect the urbanization process, and this study only considers the climate change factor. Climate change can affect urbanization in several ways. In addition to the most obvious temperature changes, it also affects the urbanization process by changing the energy structure, affecting the spatial distribution of precipitation, and causing natural disasters. In the follow-up, we will investigate the internal mechanisms of climate impact on urbanization from multiple perspectives and propose policies. In addition, the results only reflect the direction of the influence of temperature on urbanization; cities are a system, and further research on more microscopic aspects of the system should be considered.
The original contributions presented in the study are included in the article/Supplementary Material, further inquiries can be directed to the corresponding author.
Ku-CC: project administration. X-RM, YY and Y-JL: investigation and validation. Ku-CC, X-RM, YY, Y-JL: formal analysis. Ke-CC: conceptualization and supervision. All authors designed and conducted the study, analyzed the data, and wrote the draft. All authors contributed to the interpretation of results, critically reviewed draft, and approved the final manuscript.
The authors declare that the research was conducted in the absence of any commercial or financial relationships that could be construed as a potential conflict of interest.
All claims expressed in this article are solely those of the authors and do not necessarily represent those of their affiliated organizations, or those of the publisher, the editors and the reviewers. Any product that may be evaluated in this article, or claim that may be made by its manufacturer, is not guaranteed or endorsed by the publisher.
Alcoforado, M. J., Marques, D., Garcia, R. A., Canário, P., de Fátima Nunes, M., Nogueira, H., et al. (2015). Weather and climate versus mortality in Lisbon (Portugal) since the 19th century. Appl. Geogr. 57, 133–141. doi:10.1016/j.apgeog.2014.12.017
Alvarez, J., and Arellano, M. (2003). The time series and cross-section asymptotics of dynamic panel data estimators. Econometrica 71 (4), 1121–1159. doi:10.1111/1468-0262.00441
Andrew, L, Chien-Fu, L, and Chu, C. J. (2002). Unit root tests in panel data: Asymptotic and finite-sample properties. J. Econ. 108 (1), 1–24. doi:10.1016/s0304-4076(01)00098-7
Bettencourt, L. M., Lobo, J., Helbing, D., Kühnert, C., and West, G. B. (2007). Growth, innovation, scaling, and the pace of life in cities. Proc. Natl. Acad. Sci. U. S. A. 104 (17), 7301–7306. doi:10.1073/pnas.0610172104
Cai, X., Yi, L., and Jin, W. (2018). The impact of temperature on manufacturing worker productivity: Evidence from personnel data. J. Comp. Econ. 46 (4), 889–905. doi:10.1016/j.jce.2018.06.003
Chai, K.-C., Li, Q., Bao, X.-L., Zhu, J., and He, X.-X. (2021). An empirical study of economic cycle, air quality, and national health since reform and opening up. Front. Public Health 9, 706955. doi:10.3389/fpubh.2021.706955
Chen, S., Chen, X., and Xu, J. (2016). Impacts of climate change on agriculture: Evidence from China. J. Environ. Econ. Manage. 76, 105–124. doi:10.1016/j.jeem.2015.01.005
Davoudi, S., Shaw, K., Haider, L. J., Quinlan, A. E., Peterson, G. D., and Wilkinson, C. (2012). Resilience: A bridging concept or a dead end? “Reframing” resilience: Challenges for planning theory and practice interacting traps: Resilience assessment of a pasture management system in Northern Afghanistan Urban resilience: What does it mean in planning practice? Resilience as a useful concept for climate change adaptation? The politics of resilience for planning: A cautionary note Plan. Theory Pract. 13 (2), 299–333. doi:10.1080/14649357.2012.677124
De Matteis, G. (1986). Urbanization and counter-urbanization in Italy. Ekistics. 53 (316-317), 26–33.
Fan, J. L., Hu, J. W., and Zhang, X. (2019). Impacts of climate change on electricity demand in China: An empirical estimation based on panel data. Energy 170, 880–888. doi:10.1016/j.energy.2018.12.044
Field, C. B., Barros, V. R., and Change, I. P. (2014). IPCC. Climate change 2014: Impacts, adaptation, and vulnerability. Part A: Global and sectoral aspects. Contribution of working group II to the fifth assessment report of the intergovernmental panel on climate change. guangdong agricultural sciences. Cambridge, United Kingdom: Cambridge University Press, 1–1101.
Giannakopoulos, C., and Psiloglou, B. E. (2006). Trends in energy load demand for athens, Greece: Weather and non-weather related factors. Clim. Res. 31 (1), 97–108. doi:10.3354/cr031097
Gu, C. (2019). Urbanization: Processes and driving forces. Sci. China Earth Sci. 62 (9), 1351–1360. doi:10.1007/s11430-018-9359-y
Hansen, B. E. (2000). Sample splitting and threshold estimation. Econometrica 68 (3), 575–603. doi:10.1111/1468-0262.00124
He, B.-J., Wang, J., Zhu, J., and Qi, J. (2022). Beating the urban heat: Situation, background, impacts and the way forward in China. Renew. Sustain. Energy Rev. 161, 112350. doi:10.1016/j.rser.2022.112350
Huo, T., Li, X., Cai, W. G., Zuo, J., and Wei, H. (2020). Exploring the impact of urbanization on urban building carbon emissions in China: Evidence from a provincial panel data model. Sustain. Cities Soc. 56, 102068. doi:10.1016/j.scs.2020.102068
Huong, H. T. L., and Pathirana, A. (2013). Urbanization and climate change impacts on future urban flooding in Can Tho city, Vietnam. Hydrol. Earth Syst. Sci. 17 (1), 379–394. doi:10.5194/hess-17-379-2013
Jun’Ya, T., Shinichiro, F., Kiyoshi, T., Yasuaki, H., Tomoko, H., Yasushi, H., et al. (2017). Cost of preventing workplace heat-related illness through worker breaks and the benefit of climate-change mitigation. Environ. Res. Lett. 12 (6), 064010. doi:10.1088/1748-9326/aa72cc
Karl, T. R., Diaz, H. F., and Kukla, G. (1988). Urbanization: Its detection and effect in the United States climate record. J. Clim. 1 (11), 1099–1123. doi:10.1175/1520-0442(1988)001<1099:UIDAEI>2.0.CO;2
Kjellstrom, T., Kovats, R. S., Lloyd, S. J., Holt, T., and Tol, R. (2009). The direct impact of climate change on regional labor productivity. Archives Environ. Occup. Health 64 (4), 217–227. doi:10.1080/19338240903352776
Kummu, M., Ward, P. J., de Moel, H., and Varis, O. (2010). Is physical water scarcity a new phenomenon? Global assessment of water shortage over the last two millennia. Environ. Res. Lett. 5 (3), 034006. doi:10.1088/1748-9326/5/3/034006
Li, X., Chow, K. H., Zhu, Y., and Lin, Y. (2016). Evaluating the impacts of high-temperature outdoor working environments on construction labor productivity in China: A case study of rebar workers. Build. Environ. 95, 42–52. doi:10.1016/j.buildenv.2015.09.005
Liu, Y., and Lai, X. (2021). EKC and carbon footprint of cross-border waste transfer: Evidence from 134 countries. Ecol. Indic. 129, 107961. doi:10.1016/j.ecolind.2021.107961
Matsumoto, K. (2019). Climate change impacts on socioeconomic activities through labor productivity changes considering interactions between socioeconomic and climate systems. J. Clean. Prod. 216, 528–541. doi:10.1016/j.jclepro.2018.12.127
Michail, F., José, L., Deborah, S., and Seto, K. C. (2013). Does size matter? Scaling of CO2 emissions and U.S. Urban areas. Plos One 8 (6), e64727. doi:10.1371/journal.pone.0064727
Monier, E., Paltsev, S., Sokolov, A., Chen, Y., Gao, X., Ejaz, Q., et al. (2018). Toward a consistent modeling framework to assess multi-sectoral climate impacts. Nat. Commun. 9 (1), 660. doi:10.1038/s41467-018-02984-9
Monstadt, J. (2009). Conceptualizing the political ecology of urban infrastructures: Insights from technology and urban studies. ENnviron Plan. A 41 (8), 1924–1942. doi:10.1068/a4145
Mueller, N., Rojas-Rueda, D., Basagaña, X., Cirach, M., Cole-Hunter, T., D Advand, P., et al. (2017). Urban and transport planning related exposures and mortality: A health impact assessment for cities. Environ. Health Perspect. 125 (1), 89–96. doi:10.1289/ehp220
Nagy, G. J., Leal, F. W., Azeiteiro, U. M., Johanna, H., and Chunlan, L. (2018). An assessment of the relationships between extreme weather events, vulnerability, and the impacts on human wellbeing in Latin America. Int. J. Environ. Res. Public Health 15 (9), 1802. doi:10.3390/ijerph15091802
Nations, U. Sustainable development goals. (2015). Retrieved from: https://www.un.org/sustainabledevelopment/zh/sustainable-development-goals/.
Nordhaus, W. D. (1997). Managing the global commons: The economics of climate change. J. Econ. Literature (4), 26.
Reiner, R. C., Smith, D. L., and Gething, P. W. (2015). Climate change, urbanization and disease: Summer in the city. Trans. R. Soc. Trop. Med. Hyg. 109 (3), 171–172. doi:10.1093/trstmh/tru194
Renzhi, N., and Baek, Y. J. (2020). Can financial inclusion be an effective mitigation measure? Evidence from panel data analysis of the environmental kuznets curve. Finance Res. Lett. 37, 101725. doi:10.1016/j.frl.2020.101725
Rezai, A., Taylor, L., and Foley, D. (2017). Economic growth, income distribution, and climate change. Ecol. Econ. 146, 164–172. doi:10.1016/j.ecolecon.2017.10.020
Shao, Q., Tao, R., and Luca, M. M. (2022). The effect of urbanization on health care expenditure: Evidence from China. Front. Public Health 10, 850872. doi:10.3389/fpubh.2022.850872
Shearmur, R. (2012). Are cities the font of innovation? A critical review of the literature on cities and innovation. Cities 29 (S2), S9–S18. doi:10.1016/j.cities.2012.06.008
Shepherd, J. M., and Burian, S. J. (2003). Detection of urban-induced rainfall anomalies in a major coastal city. Earth Interact. 7 (4), 1–17. doi:10.1175/1087-3562(2003)007<0001:douira>2.0.co;2
Silva, J. D., Kernaghan, S., and Luque, A. (2012). A systems approach to meeting the challenges of urban climate change. Int. J. Urban Sustain. Dev. 4 (2), 125–145. doi:10.1080/19463138.2012.718279
Song, Y., Yang, T., Li, Z., Zhang, X., and Zhang, M. (2020). Research on the direct and indirect effects of environmental regulation on environmental pollution: Empirical evidence from 253 prefecture-level cities in China. J. Clean. Prod. 269, 122425. doi:10.1016/j.jclepro.2020.122425
Takagi, H., Thao, N. D., and Anh, L. T. (2016). Sea-level rise and land subsidence: Impacts on flood projections for the mekong delta’s largest city. Sustainability 8 (9), 959. doi:10.3390/su8090959
Wang, Q., and Su, M. (2020). A preliminary assessment of the impact of COVID-19 on environment–A case study of China. Sci. Total Environ. 728, 138915. doi:10.1016/j.scitotenv.2020.138915
Wang, Q., and Wang, L. (2020). Renewable energy consumption and economic growth in OECD countries: A nonlinear panel data analysis. Energy 207, 118200. doi:10.1016/j.energy.2020.118200
Wang, Q., Wang, S., and Jiang, X.-t. (2021). Preventing a rebound in carbon intensity post-COVID-19–lessons learned from the change in carbon intensity before and after the 2008 financial crisis. Sustain. Prod. Consum. 27, 1841–1856. doi:10.1016/j.spc.2021.04.024
Wang, Q., Wang, X., and Li, R. (2022). Does urbanization redefine the environmental kuznets curve? An empirical analysis of 134 countries. Sustain. Cities Soc. 76, 103382. doi:10.1016/j.scs.2021.103382
Wang, Q., and Zhang, F. (2021). The effects of trade openness on decoupling carbon emissions from economic growth–evidence from 182 countries. J. Clean. Prod. 279, 123838. doi:10.1016/j.jclepro.2020.123838
Wang, X., Shao, S., and Li, L. (2019). Agricultural inputs, urbanization, and urban-rural income disparity: Evidence from China. China Econ. Rev. 55, 67–84. doi:10.1016/j.chieco.2019.03.009
Wang, Z., and Li, J. (2019). The effect of urbanization on environmental pollution in rapidly developing urban agglomerations. J. Clean. Prod. 237, 117649. doi:10.1016/j.jclepro.2019.117649
Wu, H., Gai, Z., Guo, Y., Li, Y., Hao, Y., and Lu, Z. N. (2020). Does environmental pollution inhibit urbanization in China? A new perspective through residents' medical and health costs. Environ. Res. 182, 109128. doi:10.1016/j.envres.2020.109128
Wu, H., Hao, Y., and Weng, J. H. (2019). How does energy consumption affect China's urbanization? New evidence from dynamic threshold panel models. Energy Policy 127, 24–38. doi:10.1016/j.enpol.2018.11.057
Xiang, J., Peng, B., Pisaniello, D., and Hansen, A. (2014). Health impacts of workplace heat exposure: An epidemiological review. Ind. Health 52 (2), 91–101. doi:10.2486/indhealth.2012-0145
Xu, D., Gao, J., Lin, W. P., and Zhou, W. Y. (2021). Differences in the ecological impact of climate change and urbanization. Urban Clim. 38, 100891. doi:10.1016/j.uclim.2021.100891
Yao, J., Xu, P., and Huang, Z. (2021). Impact of urbanization on ecological efficiency in China: An empirical analysis based on provincial panel data. Ecol. Indic. 129, 107827. doi:10.1016/j.ecolind.2021.107827
Yy, A., Jl, B., Yl, B., and Ql, A. (2019). The impact of urbanization on China's residential energy consumption. Struct. Change Econ. Dyn. 49, 170–182. doi:10.1016/j.strueco.2018.09.002
Zampieri, M., Russo, S., di Sabatino, S., Michetti, M., Scoccimarro, E., and Gualdi, S. (2016). Global assessment of heat wave magnitudes from 1901 to 2010 and implications for the river discharge of the Alps. Sci. Total Environ. 571, 1330–1339. doi:10.1016/j.scitotenv.2016.07.008
Zhang, K. H. (2002). What explains China's rising urbanisation in the reform era? Urban Stud. 39 (12), 2301–2315. doi:10.1080/0042098022000033881
Keywords: climate change, economic development, environmental protection, threshold regression, urbanization
Citation: Chai K-C, Ma X-R, Yang Y, Lu Y-J and Chang K-C (2022) The impact of climate change on population urbanization: Evidence from china. Front. Environ. Sci. 10:945968. doi: 10.3389/fenvs.2022.945968
Received: 17 May 2022; Accepted: 27 July 2022;
Published: 17 August 2022.
Edited by:
Benjamin Lamptey, University of Leeds, United KingdomReviewed by:
Qiang Wang, China University of Petroleum, Huadong, ChinaCopyright © 2022 Chai, Ma, Yang, Lu and Chang. This is an open-access article distributed under the terms of the Creative Commons Attribution License (CC BY). The use, distribution or reproduction in other forums is permitted, provided the original author(s) and the copyright owner(s) are credited and that the original publication in this journal is cited, in accordance with accepted academic practice. No use, distribution or reproduction is permitted which does not comply with these terms.
*Correspondence: Ke-Chiun Chang, a2VjaGl1bkBnbWFpbC5jb20=
Disclaimer: All claims expressed in this article are solely those of the authors and do not necessarily represent those of their affiliated organizations, or those of the publisher, the editors and the reviewers. Any product that may be evaluated in this article or claim that may be made by its manufacturer is not guaranteed or endorsed by the publisher.
Research integrity at Frontiers
Learn more about the work of our research integrity team to safeguard the quality of each article we publish.