- Nanjing Meteorology Bureau, Nanjing, China
Jiangsu Province is located in the middle coastal area of East China, and has a relatively developed economy and a large population density. Severe convection often occurs in Jiangsu Province, and the lightning activities may cause great economic losses and social impact in this region. Based on the theory of natural disaster risk assessment, this study investigates the risk of hazard factors, the sensitivity of hazard-pregnant environment, and the frangibility of a hazard-bearing body in Jiangsu Province. Then, we select thunderstorm days, cloud-to-ground (CG) lightning density, CG lightning current intensity, altitude, topographic relief, soil-electric conductivity, drainage density, population density, gross domestic product (GDP) per land area, and soil utilization type as the influencing factors, and then we use the analytic hierarchy process and entropy weight method to calculate the weights of the influencing factors. Furthermore, all the factors are weighted stacked in ArcGIS, and finally the lightning disaster risk zoning map (1 × 1 km of Jiangsu Province is presented. The results show that the risk of lightning disaster in Jiangsu is low in the north and high in the south. The area of different risk level increases with the decrease in risk level. High-risk areas are mainly in the middle of Nanjing, eastern Changzhou, eastern Wuxi, and the middle of Suzhou. Medium-risk areas are mainly in northern Nanjing, eastern Changzhou, eastern Suzhou, the Tongshan District of Xuzhou City, the Chongchuan District of Nantong City, Lianyun District of Lianyungang City, and the Xiangshui County, Sheyang County, and Dafeng District of Yancheng City. The sub–low-risk areas are mainly in Xuzhou, Suqian, Huai’an, and the middle of Yangzhou City. The low-risk areas are mainly in Lianyungang, Yancheng, northern Nantong, Taizhou, Zhenjiang, southern Nanjing, western Changzhou, western Wuxi, and western Suzhou. We hope this study could provide some references for the lightning disaster prevention and mitigation in Jiangsu Province and East China.
1 Introduction
Lightning often occurs during a strong atmospheric convection. The physical effects of lightning, such as high current, high voltage, and strong electromagnetic radiation, have a serious impact on transportation, electric power, broadcasting, communication, petroleum, chemical industry, aviation, and aerospace industries (Christian et al., 2003; Zhang et al., 2006; Qie et al., 2013). Therefore, it is called “a major public hazard in the electronic age” by the International Electrotechnical Commission (Xu, 2004; Li et al., 2009). Lightning not only greatly affects mobile communication, but also seriously threatens people’s lives and property Zhang et al. (2011). As one of the important means of risk management, risk zoning can effectively avoid and prevent risks (Zhang, 2014). Therefore, carrying out a regional lightning disaster risk assessment and zoning is of great significance in disaster prevention and reduction.
In recent years, some scholars have conducted in-depth research on lightning disaster risk in different regions and have achieved some meaningful results (Black et al., 2015; Miller et al., 2018; Mahdariza, 2018; Zhang K. et al., 2021). The risk assessment of lightning disaster mainly has two technical problems. The first one is to determine the influencing factors of lightning disaster risk and establish the lightning disaster risk assessment model. The other is to calculate the weights of influencing factors. In the early years, people mainly considered the risk of lightning disaster from the risk of hazard factors and the frangibility of a hazard-bearing body. Most of them selected thunderstorm days, CG lightning density, lightning current intensity, lightning disaster frequency, economic loss, casualty, population density, GDP per capita (or per land area) as the influencing factors (Yin et al., 2009; Guo and Xiong, 2008; Yuan et al., 2017). With the deepening of lightning research, people began to realize that the spatial difference of lightning disaster risk is also closely related to climate, terrain, landform, air composition, and local thermal and dynamic conditions (Carey and Buffalo, 2007; Kar et al., 2009; Kar and Liou, 2014; Xia et al., 2015; Rathindra et al., 2020; Nicora et al., 2021; Yu et al., 2021). Therefore, in the recent research on lightning disaster risk assessment and zoning, more lightning disaster influencing factors are selected. Some sensitivity factors of hazard-pregnant environment are included, such as altitude, drainage density, topographic relief, soil utilization type, defense capacity, and soil electric conductivity (Cheng and Wang, 2017; Chen et al., 2019; Li et al., 2021; Wu and Xu, 2021).
Moreover, a large number of studies have adopted analytic hierarchy process to calculate the weights of influencing factors (Wang et al., 2007; Tian et al., 2012; Li et al., 2017; Liu et al., 2021). Combining qualitative and quantitative methods, AHP can deal with many practical problems that cannot be solved by traditional optimization technology. However, the construction of judgment matrix is subjective. In general, the AHP is a subjective weighting method, and the results may be unacceptable for some decision makers. Some studies tried other methods to assess the weights of lightning disaster risk indexes. Chen et al. (2021) used entropy weight comprehensive evaluation model to evaluate the regional lightning disasters in 68 counties and cities in Fujian Province. The entropy weight method (EWM) is an objective method to determine the weight, which is more accurate than subjective methods such as AHP. By using the support vector machine, Sheng et al. (2021) explored a way to combine artificial intelligence algorithms with lightning disaster risk assessment. Zhang and Han (2021) solved the problem of imbalanced lightning disaster data in geographic grid samples based on the K-means clustering algorithm, which calculates the feature of lightning disaster in each small field with the help of neural network structure.
Jiangsu Province is in the middle coastal area of East China (30°45′N–35°08′N, 116°21′E–121°56′E). It is of humid or semi-humid monsoon climate. With the advance and retreat of the western Pacific subtropical high, cold, and warm air frequently converge in Jiangsu Province from spring to autumn, making it the sub–high-value area of lightning density in China (Wang and Chen, 2015). Some studies on the risk and vulnerability of lightning disasters in Jiangsu have been carried out (Zhang et al., 2007; Chen et al., 2017), but some of them have not considered the natural environment and socio-economic development. Moreover, the assignment methods for factor weights in their studies are somewhat subjective, and the research results take city as the basic unit, resulting in a low spatial accuracy in zoning results. Therefore, based on the previous research and the actual situation of Jiangsu, this study selects the evaluation indexes from the risk of hazard factors, the sensitivity of hazard-pregnant environment and the frangibility of a hazard-bearing body. Then, the subjective and objective weight-assignment methods are combined, and the AHP and EWM are used to quantitatively calculate the weight of each index. Afterward, each index is weighted-stacked in the geographic information system (GIS) to draw the lightning disaster risk zoning map (1 × 1 km grid) of Jiangsu. We hope this study could provide a scientific basis for lightning disaster prevention and reduction in Jiangsu.
The remainder of this article is organized as follows. Section 2 shows the data and methods used in this study. Section 3 introduces the establishment of lightning disaster risk zoning model. The lightning disaster risk zoning is given in Section 4. Finally, the summary and conclusions are presented in section 5.
2 Data and Methods
2.1 Data Introduction
The research data in this study include three categories, that is, the lightning location data, the geographic information data and the socio-economic data. The lightning location data are obtained from the CG lightning data detected by the Jiangsu ADTD-II lightning positioning system from 2016 to 2020. The system is composed of 16 stations (Figure 1), and can accurately detect the arriving time of the electromagnetic pulse generated in the discharging of thunderstorms. Therefore, it can obtain the occurrence time, position, height, polarity, intensity, steepness, and other parameters of lightning in real time (Feng et al., 2015), and achieve the 3-dimensional lightning positioning. Meng et al. (2022) found that the horizontal and vertical location errors of the Jiangsu ADTD-II lightning positioning system are less than 300 and 500 m, respectively.
If there is lightning record in 1 day, then this day is counted as a thunderstorm day. The lightning density denotes the average frequency of lightning per area. The lightning intensity denotes the average current intensity per area. The thunderstorm days, lightning density, and lightning intensity are counted in the 1 × 1 km grids. According to the quality control method specified in the industrial group document of the Institute of Electrical and Electronics Engineers, the lightning data with the absolute value of lightning current being 0–2 kA and greater than 200 kA are eliminated (IEEES Std., 1997). The elevation data come from the Shuttle Radar Topography Mission, with an accuracy of 90 m, and it can be obtained from the geospatial data cloud (http://srtm.csi.cgiar.org/). The drainage density data are from the National Basic Geographic Information Center (https://www.ngcc.cn). The soil electric conductivity is from the Harmonized World Soil Database (http://www.ncdc.ac.cn). The soil utilization type is from the Resource and Environment Science and Data Center (https://www.resdc.cn). The population and GDP data are from the Resource and Environment Science and Data Center (https://www.resdc.cn). The geographic information and social economy data are from 2016 to 2020 with a resolution of 1 × 1 km.
2.2 Methods
2.2.1 Data Normalization
To eliminate the differences in physical dimension among the indexes, each index that is involved in grid calculation is normalized. The calculation formula is as follows.
where
2.2.2 Analytic Hierarchy Process
The AHP is a multicriteria decision-making method that combines qualitative and quantitative analysis. It was first proposed by the well-known American operational research scientist Saaty (1980). The operation steps of AHP include judgment matrix construction, consistency test, and weight ranking. Each index is compared in pairs by using the 1–9 scale method in judgment matrix construction (Table 1). By solving the maximum eigenvalue
According to the order of the judgment matrix, the average random consistency index value
If C.R.≤ 0.1, the judgment matrix meets the consistency requirement, otherwise the judgment matrix should be rebuilt.
2.2.3 Entropy-Weight Method
The EWM is an objective assignment method. According to the characteristics of entropy, the randomness and disorder degree of an event can be judged by calculating the entropy, and the dispersion degree of an index can also be judged by the entropy. The greater the dispersion degree of the index is, the greater the weight of the index to the comprehensive evaluation is. Entropy weight can be calculated by Eqs. 4–6.
wi is the entropy weight of the ith evaluation index, hi is the entropy of the ith evaluation index, n is the number of evaluation indexes, m is the number of samples, and pij is the proportion of the jth sample value to ith index.
3 Establishment of Lightning Disaster Risk Zoning Model
3.1 Construction of Lightning Disaster Risk Evaluation Index System
Lightning disaster risk refers to the possibility of lightning disaster and its possible losses. Based on the theory of natural disaster risk assessment and zoning, this study constructs the lightning disaster risk assessment index system of “target layer-criterion layer-index layer” (Figure 2). It can be seen that the risk of lightning disaster is the target layer; the risk of hazard factors, the sensitivity of hazard-pregnant environment, and the frangibility of hazard-bearing body form the criterion layer; the thunderstorm days, CG lightning density, CG lightning current intensity, altitude, topographic relief, drainage density, soil electric conductivity, population density, average GDP per land area, and soil utilization type form the index layer.
3.2 Calculation of Lightning Disaster Risk Index
The lightning disaster risk index is composed of the risk of hazard factors, the sensitivity of hazard-pregnant environment, and the frangibility of hazard-bearing body. The corresponding relationships among each index and disaster risk are shown in Eqs. 7–10:
In Equation 8, the greater the lightning disaster risk evaluation index is LDRI, the greater the risk degree is, Rh, Re, and Rf are evaluation indexes of the risk of hazard factors, the sensitivity of hazard-pregnant environment, and the frangibility of hazard-bearing body, respectively; wr, we, and wf are the weights corresponding to each influencing factor of the criterion layer. In Eqs. 9, 10, Wi is the weight of each index in the index layer; Qh1, Qh2, and Qh3 are the normalized values of thunderstorm days, CG lightning density, and CG lightning current intensity, respectively; Qe1, Qe2, Qe3, and Qe4 are the normalized values of altitude, topographic relief, soil electric conductivity, and drainage density, respectively; and Qf1, Qf2, and Qf3 are the normalized values of population density, average GDP per land area, and soil utilization type, respectively. The normalized values of each index in the index layer are multiplied by the corresponding weights to obtain the evaluation index of the factors in the criterion layer of the lightning disaster risk evaluation model. Then, the weighted stack analysis is carried out level by level to obtain the comprehensive evaluation index value of lightning disaster risk in the target layer.
3.3 Determining Weights of the Index
The AHP-EWM model, using a combinative weighting approach, considers both subjective and objective weighted procedures and is adopted to yield more accurate criteria weight results as recommended by some researchers (Jahan et al., 2012; Alemi et al., 2016; Ding et al., 2018; Dehdasht et al., 2020). According to the Technical Guidelines for Lightning Disaster Risk Zoning (QX/T 405-2017), the weights of the indexes in the criterion level are determined by the AHP, and the weights of the indexes in the index level are determined by the EWM. According to past studies (Li et al., 2018; Long et al., 2019; Shi et al., 2019; Ren et al., 2020; Cui et al., 2021), it is clear that the hazard-bearing body is the object of hazard factors, and the interaction between the two contributes greatly to the formation of lightning disasters. The order of the indexes in the criterion level is determined as the risk of hazard factors, the frangibility of the hazard-bearing body, and the sensitivity of the hazard-pregnant environment. By constructing the priority judgment matrix and giving the relative scores of various indexes, the AHP is carried out based on the scoring results. The weight coefficients of the risk of hazard factors, the sensitivity of the hazard-pregnant environment, and the frangibility of the hazard-bearing body are 0.41, 0.24, and 0.35, respectively. The consistency test coefficient C.R.= 0.002 < 0.10, showing that the judgment matrix is acceptable. The weights of indexes in the index layer are determined by the EWM. We use GIS to generate 5,000 random points within the study area, extract the normalized factor values of 10 indexes in the index layer to the random points, derive the attribute table, and calculate the weights of each index according to Eqs. 4–6. Finally, the weights of the index layer and the criterion layer are weighted to obtain the comprehensive weight of each index, as shown in Table 3.
4 Lightning Disaster Risk Zoning
4.1 Risk of Hazard Factors
Figure 3A shows the spatial distribution of thunderstorm days in Jiangsu. As can be seen, the number of thunderstorm days in Jiangsu is generally large in the south and small in the north, which is consistent with the spatial distribution of convective activities in Jiangsu (Shu et al., 2022). The areas with thunderstorm days greater than 60 days per year (d/a) are mainly in Suzhou, Wuxi, Changzhou, Nanjing, and northwestern Xuzhou. The average thunderstorm days in most parts of northern-middle Jiangsu are 30–50 d/a, and the areas with thunderstorm days less than 30 d/a are mainly in Yancheng of eastern Jiangsu. Figure 3B shows the spatial distribution of CG lightning density in Jiangsu. It can be seen that the annual average CG lightning density in Jiangsu is 2.35 times/(km2·a). Few areas are with CG lightning density greater than 6 times/(km2·a), such as eastern Suzhou, northern Changzhou, northern Nanjing, and southern Huai’an. The areas with lightning density less than 3 times/(km2·a) are the eastern coastal cities and Taizhou. Figure 3C shows the spatial distribution of CG lightning current intensity in Jiangsu. It can be seen that the CG lightning current intensity is generally low in Jiangsu, with an average value of 9.9 kA, indicating that the lightning in Jiangsu is mainly negative CG lightning with low energy. The high-value areas of CG lightning current intensity are mainly in central Nanjing, eastern Changzhou, southeastern Nantong City, Yangzhou City, western Zhenjiang City, and southern Yangzhou, where the CG lightning current intensity is 50–70 kA, indicating that the proportion of positive CG lightning in these areas is higher than that in other areas. The CG lightning current intensity in northern Jiangsu is relatively low. Specifically, it is within 20–40 kA in Xuzhou, Suqian, Huai’an, and central-northern Yancheng; while it is below 20 kA in Lianyungang, southern Yancheng, Taizhou, and southern Nanjing. It is indicated that the lightning activities are weak in the above areas.
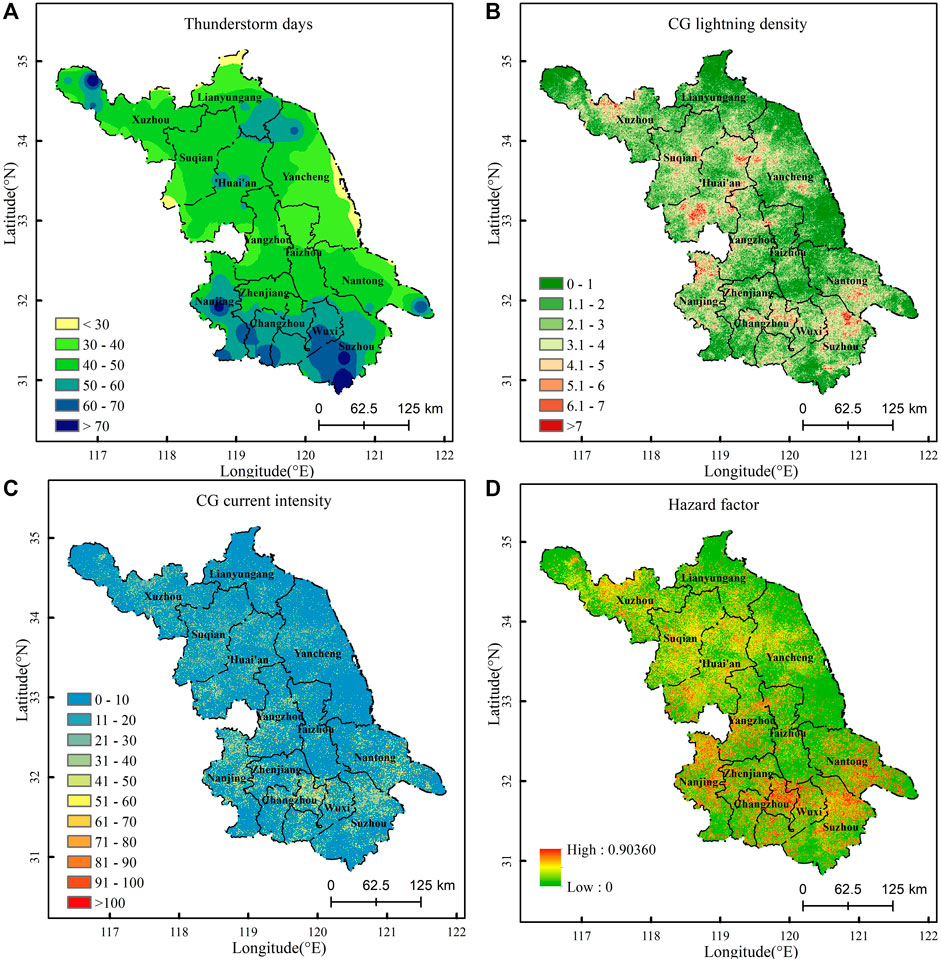
FIGURE 3. Distribution of the risk of lightning hazard factors in Jiangsu. (A) Thunderstorm days, unit: days/year; (B) CG lightning density, unit: km2/year; (C) CG current intensity, unit: KA; (D) hazard factor.
Lightning disaster hazard factors are natural and uncontrollable. The risk of hazard factors reflects the activity degree of lightning in the study area. The higher the risk of hazard factors, the greater the risk of lightning disaster. The risk of lightning hazard factors in Jiangsu is calculated with Eq. 8, as shown in Figure 3D. The risk of lightning hazard factors in Jiangsu is generally high in the south and low in the north, which is consistent with the distribution of thunderstorm days. The high-risk areas of hazard factors are mainly in northern Nanjing, the junction area between Nanjing and Zhenjiang, eastern Changzhou, and eastern Suzhou. There are also some scattered distributions in central Nantong, northwestern Yangzhou, and eastern Wuxi. The CG lightning density and current intensity in these areas are high, resulting in the high risk of hazard factors. The sub–high-risk areas of hazard factors are mainly distributed in Xuzhou, Suqian, Huai’an, and parts of Funing County of Yancheng City. The low-risk areas are concentrated in Lianyungang, southern Yancheng, Taizhou, and central Zhenjiang, southern Nanjing, western Suzhou. The low-risk area is dominant in Jiangsu, accounting for about 70.56%.
4.2 Analysis of the Sensitivity of the Hazard-Pregnant Environment
Figure 4A shows the altitude distribution of Jiangsu Province. It can be seen that the main landform of Jiangsu is plain with generally low altitude, and the average altitude is only about 13 m. 80% of the areas are less than 15 m above sea level. Most relatively, high-altitude areas are in the southwest. There are few areas with an altitude over 100 m, mainly distributed in the Xuyi hills in southwestern Huai’an, western Zhenjiang, the hills extending from Zhenjiang to Nanjing, Laoshan Mountain in Pukou District of northern Nanjing, Maoshan Mountain in the junction area of Changzhou and Zhenjiang, hilly areas in Wuxi and southern Changzhou, and the Yuntai Mountain range in eastern Lianyungang. Figure 4B shows the topographic relief of Jiangsu. Topographic relief can reflect the local relief of landform. In ArcGIS, the neighborhood analysis tool is used to count the standard deviation of maximum elevation and minimum elevation at 1 km resolution, which is taken as the topographic relief of the study area. The overall terrain of Jiangsu is flat, with 90% of the topographic relief less than 10 m. The areas with topographic relief of 15–45 m are mainly in the middle of Xuzhou, western Suqian, Nanjing, and parts of Zhenjiang. The areas with topographic relief over 45 m are mainly in western Wuxi. Figure 4C shows the distribution of drainage density in Jiangsu. It can be seen that there are many water systems in Jiangsu, showing the characteristics of high in the middle and low in the surrounding areas. The areas with a density over 0.5 km/km2 are mainly in eastern Huai’an, the middle of Suqian, western Yancheng, northern Yangzhou, and northern Taizhou. The soil electric conductivity indicates the strength of soil conductivity. The greater the soil electric conductivity is, the stronger the ability to discharge lightning current is, and the larger the possibility to form lightning disasters is. Figure 4D shows the distribution of soil electric conductivity in Jiangsu. As can be seen, the overall distribution of soil electric conductivity in Jiangsu is uniform, which is high in the north and east, and low in the south and west. The high-value areas are mainly in a narrow strip along the coastline of Lianyungang and Yancheng. These areas have high soil humidity, high salt content, and low resistance, which are conducive to conducting electricity. Most sub–high-value areas are in Xuzhou, Suqian, northern Huai’an, and eastern Nantong. Low-value areas are mainly in central and southern Jiangsu.
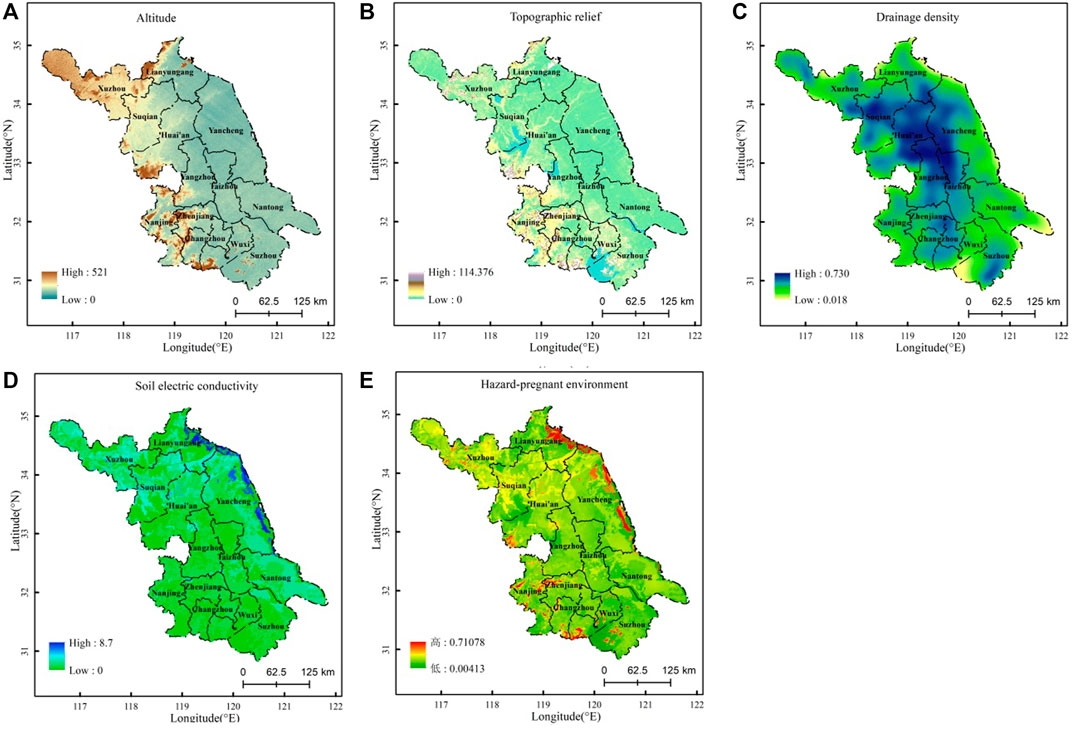
FIGURE 4. Distribution of the sensitivity of hazard-pregnant environment in Jiangsu. (A) Altitude, unit: m; (B) Topographic relief, unit: m; (C) Drainage density, unit: km/km2. (D) Soil electric conductivity, unit: ds/m; (E) Hazard-pregnant environment.
The sensitivity of the hazard-pregnant environment is calculated by using Eq. 9, as shown in Figure 4E. It can be seen that the sensitivity of lightning hazard-pregnant environment in Jiangsu is generally low. Areas with high sensitivity are scattered, such as northern-central Nanjing, northern Zhenjiang, southwestern Wuxi, southwestern Huai’an, and western Suzhou. These areas are in the low mountains or hills in southwestern Jiangsu, and the altitude is relatively high. The comparison of altitude and topographic relief with the distribution of thunderstorm days shows that most high-value areas of thunderstorm days in Jiangsu are in the hilly and low mountain areas with topographic relief greater than 15 m and altitude more than 100 m, indicating that the hilly terrain may be one of the important conditions for frequent lightning in Jiangsu. The low-value areas of thunderstorm days are mainly in the plain areas in northern Jiangsu, which may be related to its flat terrain. Meanwhile, the salt ions dissolved in the water vapor in the sea breeze of coastal cities can prevent the ionization in the lightning channel from bringing clean atmosphere, which is not conducive to the generation of lightning. The specific reasons need to be further analyzed. Another area with high sensitivity of the hazard-pregnant environment is in Guanyun County of Lianyungang City, Xiangshui County, and Sheyang County of Yancheng City, and a narrow strip along the coastline of Dafeng District of Yancheng City. These areas have high soil electric conductivity. Large areas in central-eastern Xuzhou, Suqian, and Huai’an have high drainage density and high soil electric conductivity, so the sensitivity of hazard-pregnant environment is also high. The comparison of the distribution of drainage density and CG lightning density shows that there is a circle of high-value area of CG lightning density around the high-value area of drainage density, indicating that the difference of the underlying surface between land and water body in Jiangsu may also be one of the important conditions for lightning. The areas with low sensitivity of the hazard-pregnant environment are widely distributed in Lianyungang, Yancheng, Taizhou, Nantong, and Yangzhou, and the low sensitivity of hazard-pregnant environment is dominant in Jiangsu.
4.3 Frangibility of Hazard-Bearing Body
Population density and GDP per land area directly affect the magnitude of disaster losses. Figure 5A and Figure 5B show the spatial distribution of population density and GDP per land area in Jiangsu, respectively. Population density and GDP per land area both belong to socio-economic data, and as seen from Figure 5, their distributions are basically the same, showing the characteristics of high in the south and low in the north. High-value areas are mainly in Nanjing, Wuxi, Changzhou, Suzhou, Tongshan District of Xuzhou, and Chongchuan District of Nantong. Other regions are of small population and low GDP, and the distribution is relatively uniform. Soil utilization type can reflect the protection ability of a hazard-bearing body against lightning disaster to a certain extent. According to the Land Use Status Classification (GB/T 21010-2017), there are three soil utilization types: construction land, agricultural land, and unused land. Agricultural land includes cultivated land, forest land, garden land, etc. Studies have shown that strong lightning activities with high average lightning intensity mainly occur in forest land, followed by cultivated land (Yu and Liu, 2020). The agricultural land is usually in an open area without an effective lightning protection device, so strong lightning activity can cause serious casualties there. Construction land includes the land for urban and rural housing and public facilities. Heat island effect is likely to occur in construction land which is densely populated, and aerosol may promote CG lightning activities on construction land by changing the cloud microphysical process (Steiger and Orville, 2003). Fortunately, construction land is generally equipped with lightning protection devices, which can effectively resist the risk of lightning disasters. Unused land refers to wasteland, sandy land, swamp, etc. Although lightning protection devices are not installed, the cumulative losses of life and property are small due to the low population density there.
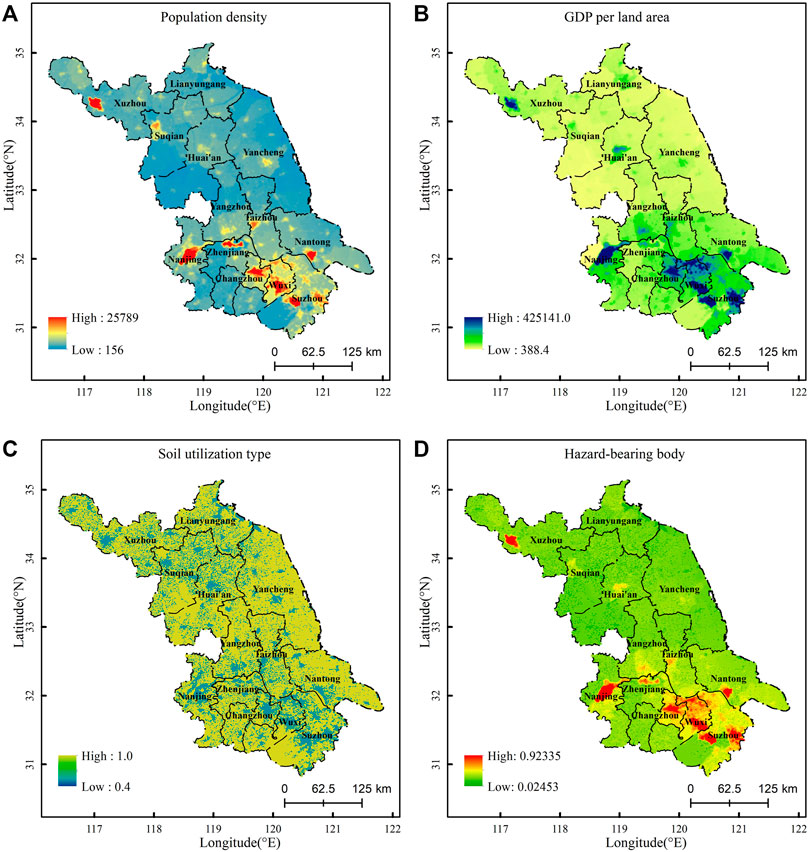
FIGURE 5. Frangibility of lightning hazard-bearing body in Jiangsu. (A) Population density, unit: person/km2; (B) GDP value of the region, unit: ten thousand Yuan/km2. (C) Soil utilization type. (D) Hazard-bearing body.
The risk assignment shows that the risk of agricultural land is 1.0, followed by construction land (0.6) and unused land (0.4), and the land use risk distribution map of Jiangsu is shown in Figure 5C. Due to the wide distribution and the high risk of cultivated land, high-risk values are widely distributed. The frangibility of lightning hazard-bearing body in Jiangsu is calculated with Eq. 10, as shown in Figure 5D. The frangibility of a hazard-bearing body in Jiangsu is highly consistent with the distribution of population density and GDP, which is high in the south and low in the north. High-vulnerability areas account for a relatively small proportion, mainly in Nanjing, Changzhou, Wuxi, Suzhou, Xuzhou, the center of Nantong, and economically developed county-level city Kunshan governed by Suzhou. The aforementioned areas have high population density and high GDP per land area. Around the high-vulnerability areas, there are some sub–high-vulnerability areas, mainly in the suburbs or county-level cities of economically developed cities in southern Jiangsu.
4.4 Analysis of Lightning Disaster Risk Zoning in Jiangsu
The comprehensive evaluation index of lightning disaster risk in the target layer is obtained by weighting the indexes of hazard factors, hazard-pregnant environment, and hazard-bearing body. The risk levels are divided by using the natural breakpoint method in GIS, and the lightning disaster risk zoning in Jiangsu is shown in Figure 6. The risk of lightning disaster in Jiangsu is high in the south and low in the north. The area of high risk is the smallest (1,338.22 km2, accounting for 1.25%), and it increases with the decrease of risk level. The high-risk areas are mainly distributed in central Nanjing, eastern Changzhou, eastern Wuxi, and central Suzhou. These areas are all downtown, with a large population density and developed economy. The risk of human life loss and economic loss caused by lightning is higher. At the same time, there are plenty of construction land and electric equipment in these areas, and the electric equipment should be protected from being damaged by induced lightning. The medium-risk area covers an area of 14,272.88 km2, accounting for 13.31% of the total area. It is mainly in northern Nanjing, eastern Changzhou, eastern Suzhou, Tongshan District of Xuzhou, Chongchuan District of Nantong, Lianyun District of Lianyungang, and Xiangshui County, Sheyang County, and Dafeng District of Yancheng. These areas have high CG lightning density, high-risk hazard factors, high soil electric conductivity, and high sensitivity of a hazard-pregnant environment. The sub–low-risk area covers an area of 22,648.44 km2, accounting for 21.13% of the total area, mainly in Xuzhou, Suqian, Huai’an, and the middle of Yangzhou. The drainage density in these areas is high, and there is a high risk for the people near the water area to suffer direct lightning attack. Therefore, the protection of people from direct lightning strike in these areas should be strengthened. The low-risk area covers an area of 68,940.47 km2, accounting for 64.31% of the total area, and it is the main type of lightning disaster risk in Jiangsu. It is distributed in Lianyungang, southern Yancheng, northern Nantong, Taizhou, central Zhenjiang, southern Nanjing, western Changzhou, western Wuxi, and western Suzhou. These areas are mainly plain areas, and have a low value of CG lightning density and current intensity, low population density, and low GDP. However, the aforementioned areas are mostly agricultural land which lacks effective lightning protection measures. A lightning disaster can easily cause casualties. It is necessary to strengthen the publicity of lightning protection knowledge.
5 Conclusion and Discussions
Focused on the lightning disaster and based on the theory of natural disaster risk assessment, this study investigates the risk of hazard factors, the sensitivity of hazard-pregnant environment, and the frangibility of a hazard-bearing body in Jiangsu Province. Then, we select thunderstorm days, CG lightning density, CG lightning current intensity, altitude, topographic relief, soil electric conductivity, drainage density, population density, GDP per area, and soil utilization type as the evaluation indexes, and establish the quantitative relationship and calculation program between the impact indexes and lightning disaster risk.
The correlations among hazard factors, hazard-pregnant environment, and hazard-bearing body have been analyzed. It is found that the high-value areas of thunderstorm days in Jiangsu are mainly in the hilly and low mountain areas with topographic relief greater than 15 m and altitude over 100 m. The low-value areas of thunderstorm days are mainly in the plain areas of northern Jiangsu. The hilly terrain in Jiangsu may be one of the important conditions for frequent lightning. A circle of high-value area of CG lightning density is wrapped around the high-value area of drainage density, indicating that the difference of underlying surface between land and water body in Jiangsu may also be one of the important conditions for lightning.
We use the AHP and EWM to determine the comprehensive weight of indexes, calculate the risk value of lightning disaster, divide the risk level, and draw the lightning disaster risk zoning map. The results show that the risk of lightning disaster in Jiangsu is high in the south and low in the north, and the risk area is inversely proportional to the risk level. The areas in Jiangsu are mainly with low risk of lightning disaster. High-risk areas are mainly in the middle of Nanjing, eastern Changzhou, eastern Wuxi, and the middle of Suzhou. Medium-risk areas are mainly in northern Nanjing, eastern Changzhou, eastern Suzhou, Tongshan District of Xuzhou, Chongchuan District of Nantong, Lianyun District of Lianyungang, and Xiangshui County, Sheyang County, and Dafeng District of Yancheng. The sub–low-risk areas are mainly in Xuzhou, Suqian, Huai’an, and the middle of Yangzhou. The low-risk areas are in Lianyungang, southern Yancheng, northern Nantong, Taizhou, central Zhenjiang, southern Nanjing, western Changzhou, western Wuxi, and western Suzhou.
Although this study only focuses on the Jiangsu Province of China, the results obtained in this study are robust and of great significance. We hope this study can provide some references for lightning disaster prevention and mitigation in Jiangsu Province and East China.
Data Availability Statement
The original contributions presented in the study are included in the article/Supplementary Material; further inquiries can be directed to the corresponding author.
Author Contributions
Conceptualization, YS; methodology, YS; software, ZH; validation, CJ; formal analysis, YS; investigation, JH; resources, CJ; data curation, QC; writing original draft preparation, CJ; writing review and editing, YS; visualization, SW; supervision, YS; project administration, YS; and funding acquisition, CJ.
Funding
This research was funded by the Jiangsu Province “333” project (BRA2020429), and the National Natural Science Foundation of China (41305051).
Conflict of Interest
The authors declare that the research was conducted in the absence of any commercial or financial relationships that could be construed as a potential conflict of interest.
Publisher’s Note
All claims expressed in this article are solely those of the authors and do not necessarily represent those of their affiliated organizations, or those of the publisher, the editors, and the reviewers. Any product that may be evaluated in this article, or claim that may be made by its manufacturer, is not guaranteed or endorsed by the publisher.
Acknowledgments
We thank Nanjing Hurricane Translation for reviewing the English language quality of this article.
References
Alemi-Ardakani, M., Milani, A. S., Yannacopoulos, S., and Shokouhi, G. (2016). On the Effect of Subjective, Objective and Combinative Weighting in Multiple Criteria Decision Making: A Case Study on Impact Optimization of Composites. Expert Syst. Appl. 46, 426–438. doi:10.1016/j.eswa.2015.11.003
Black, A. W., and Mote, T. L. (2015). Effects of Winter Precipitation on Automobile Collisions, Injuries, and Fatalities in the United States. J. Transp. Geogr. 48, 165–175. doi:10.1016/j.jtrangeo.2015.09.007
Carey, L. D., and Buffalo, K. M. (2007). Environmental Control of Cloud-To-Ground Lightning Polarity in Severe Storms. J.Monthly Weather Rev. 135 (4), 1327–1353. doi:10.1175/mwr3361.1
Chen, G. C., Cui, X., and Tian, X. R. (2017). Quantitative Assessment and Zoning of Lightning Hazard in Jiangsu Province. J. Catastrophology 32 (1), 32–35. doi:10.3969/j.issn.1000-811X2017.01.007
Chen, L., Chen, C. Z., Hu, Y. J., Yang, X. M., and Zhu, Y. F. (2021). Thunder Disaster Risk Division in Fujian Province Based on Entropy Weight Comprehensive Evaluation Mode. J. Meteorological Res. Appl. 42 (1), 46–52. doi:10.19849/j.cnki.CN45-1356/P.2021.2.09
Chen, L. T., Long, S., Yu, H. Y., Li, H. Y., and Wang, Y. (2019). Research on Risk Zoning of Lightning Disaste in Hebei Province. J.Journal Catastrophology 34 (3), 189–195. doi:10.3969/j.issn.1000-811X.2019.03.035
Cheng, M., and Wang, X. L. (2017). Risk Vulnerability Zoning of Lightning Disaster in Southwest Shandong Based on the Data of Lightning Orientation. J.Meteoro-logical Environ. Sci. 40 (4), 126–131. doi:10.16765/j.cnki.1673-7148.2017.04.018
Christian, H. J., Blakeslee, R. J., Boccippio, D. J., Boeck, W. L., Buechler, D. E., Driscoll, K. T., et al. (2003). Global Frequency and Distribution of Lightning as Observed from Space by the Optical Transient Detector. J.Geophysical Res. Atmos. 108 (D1), 4–15. doi:10.1029/2002jd002347
Cui, X. Q., Fu, J., Dai, J., Liu, Y., Hu, Z. H., and Tang, J. (2021). Lightning Disaster Risk Zoning on the High-Speed Railway (City Railway) Lines in Hubei Province Based on Arcgis and AHP. J. Catastrophology. 36 (02), 79–83. doi:10.3969/j.issn.1000-811X.2021.02.014
Dehdasht, G., Ferwati, M. S., Zin, R. M., and Abidin, N. Z. (2020). A Hybrid Approach Using Entropy and TOPSIS to Select Key Drivers for a Successful and Sustainable Lean Construction Implementation. PLoS One 15 (2), e0228746. doi:10.1371/journal.pone.0228746
Ding, Z., Zhu, M., Wu, Z., Fu, Y., and Liu, X. (2018). Combining AHP-Entropy Approach with GIS for Construction Waste Landfill Selection-A Case Study of Shenzhen. Ijerph 15 (10), 2254. doi:10.3390/ijerph15102254
Feng, M. X., Zhou, Y., Yu, M., and Zhang, Y. H. (2015). Evaluation of Jiangsu ADTD Lightning Location System Data in 2013. J. Sci. Technol. Eng. 15 (7), 79–84.
Guo, H., and Xion, Y. J. (2008). Vulnerability Analysis, Evaluation and Vulnerability Zoning of Lightning Disaster in Beijing. J. Appl. Meteorological Sci. 19 (1), 35–40.
IEEES Std (1997). IEEE Guide for Improving the Lightning Performance of Transmission Lines. NewYork: IEEE, 1243–1997.
Jahan, A., Mustapha, F., Sapuan, S. M., Ismail, M. Y., and Bahraminasab, M. (2012). A Framework for Weighting of Criteria in Ranking Stage of Material Selection Process. Int. J. Adv. Manuf. Technol. 58, 411–420. doi:10.1007/s00170-011-3366-7
Kar, S. K., Liou, Y.-A., and Ha, K.-J. (2009). Aerosol Effects on the Enhancement of Cloud-To-Ground Lightning over Major Urban Areas of South Korea. Atmos. Res. 92 (1), 80–87. doi:10.1016/j.atmosres.2008.09.004
Kar, S. K., and Liou, Y. A. (2014). Enhancement of Cloud-To-Ground Lightning Activity over Taipei, Taiwan in Relation to Urbanization. Atmos. Res. 147-148, 111–120. doi:10.1016/j.atmosres.2014.05.017
Li, W. H., Liu, X., Wu, R., Zhong, Y., Mai, Y., Wang, J., et al. (2021). Comprehensive Risk Regionalization of Lightning Disaster over Qinghai Province from 2010 to 2019. J. Arid. Meteorol. 39 (6), 1017–1024. doi:10.11755/j.issn.1006-7639(2021)-06-1017
Li, X. L., Chen, J. H., Gu, S. Q., and Tong, X. (2009). Statistics and Analysis of Lightning Flashovers of Transmission Lines during 2000–2007. J. High. Volt. Eng. 35 (3), 705–710. doi:10.1109/ICHVE.2008.4773871
Li, Y. C., Wu, L. F., Yu, C. H., Lin, W. H., and Wang, T. T. (2017). Lightning Characteristics and Lightning Disaster Vulnerability Zoning in Sanming of Fujian Province. J. Nanjing Univ. Inf. Science&Technology Nat. Sci. Ed. 9 (1), 220–226. doi:10.13878/j.cnki.jnuist.2017.02.016
Li, Y. C., Shen, Y. S., Chen, A. Z., Zhang, Z. F., Cai, H. Z., and Sun, C. L. (2018). Vulnerability Zoning Model of Fine Lightning Disasters Based on GIS Overlay Method. Meteorological Sci. Technol. 46 (1), 182–188. doi:10.19517/j.1671-6345.20170136
Liu, P. Y., Zhang, T. F., Yin, L. Y., Zhou, Q. Q., and Yang, X. P. (2021). Comprehensive Evaluation and Zoning of Lightning Disaster Risks in Yunnan Province Based on Multi-Source Data Fusion. J. Meteorological Sci. Technol. 49 (2), 269–277. doi:10.19517/j.1671-6345.20200034
Long, S., Yu, H. Y., Li, T., Li, Q., Zou, Z. X., and Wang, Y. (2019). Research on Lightning Disaster Risk Zoning in the Rural Areas of Hebei Province. J. Chin. J. Agric. Resour. Regional Plan. 40(11),174–179.
Mahdariza, F. (2018). The Determination of Lightning Disaster Hazard Index Using Analytical Hierarchy Process. J. Islamic Sci. Technol. 3 (2), 233–238. doi:10.22373/ekw.v3i2.1630
Meng, X. Y., Wang, J. Q., Ma, Q. M., Yuan, S. B., Song, Y. J., and Zhou, X. (2022). A Dataset of Lightning in China Based on VLF/LF Lightning Location Monitoring System. J. China Sci. Data 7 (1). doi:10.11922/11-6035.csd.2021.0059
Miller, P. W., and Mote, T. L. (2018). Characterizing Severe Weather Potential in Synoptically Weakly Forced Thunderstorm Environments. J. Nat. Hazards &Earth Syst. Sci. 18 (4), 1–39. doi:10.5194/nhess-18-1261-2018
Nicora, M., Mestriner, D., Brignone, M., Bernardi, M., Procopio, R., and Fiori, E. (2021). A 10-year Study on the Lightning Activity in Italy Using Data from the SIRF Network. Atmos. Res. 256 (2021), 105552. doi:10.1016/j.atmosres.2021.105552
Qie, X. S., Zhang, Q. L., Yuan, T., and Zhang, T. L. (2013). Lightning Physics. Beijing: Science Press.
Rathindra, N. B., Islam, Md. N., Mia, Md. J., and Islam, M. N. (2020). Modeling on the Spatial Vulnerability of Lightning Disaster in Bangladesh Using GIS and IDW Techniques. J. Spatial Inf. Res. 28 (5), 507–521. doi:10.1007/s41324-019-00311-y
Ren, Z. H., Xu, W., Yu, S. Y., and Luo, F. (2020). The Lightning Disaster Regionalization in Chongqing. J.Chinese J. Agric. Resour. Regional Plan. 41 (04), 317–324. doi:10.7621/cjarrp.1005-9121.20200437
Sheng, J., Xu, M., Han, J., and Deng, X. (2021). A Lightning Disaster Risk Assessment Model Based on SVM. J. Big Data. 3 (4), 183–190. doi:10.32604/jbd.2021.024892
Shi, J. M., Xu, W. J., Xu, L., Jin, X., and Zhu, Y. J. (2019). Risk Assessment of Lightning Disaster Events in the Agricultural Areas of Eastern Qinghai Province. J. Glaciol. Geocryol. 41 (06), 1359–1366. doi:10.7522/j.issn.1000-0240.2019.0079
Shu, Y., Sun, J., and Chenlu, J. (2022). A 10-Year Climatology of Midlevel Mesoscale Vortices in China. J. Appl. Meteorology Climatol. 61, 309–328. doi:10.1175/JAMC-D-21-0095.1
Steiger, S. M., and Orville, R. E. (2003). Cloud-to-ground Lightning Enhancement over Southern Louisiana. Geophys. Res. Lett. 30 (19), 1975. doi:10.1029/2003GL017923
Tian, Y. T., Wu, M. H., Shi, F. Q., and Wei, X. M. (2012). Evaluation and Regionalization of Lightning Disaster Vulnerability over Hebei. J. Meteorological Sci. Technol. 40 (3), 507–512. doi:10.19517/j.1671-6345.2012.03.032
Wang, H., Deng, Y., Yin, L. Y., Xu, Y. J., and Jing, Y. S. (2007). Vulnerability Analysis and Zoning of Lightning Disaster in Yunnan Province. J. Meteorol. 12, 83–87. doi:10.7519/j.issn.1000-0526.2007.12.012
Wang, J., and Chen, Y. (2015). Analysis of the 2009-2012 Lightning Distribution Characteristics inChina. J. Meteorol. Mon. 41 (2), 160–170. doi:10.7519/j.issn.1000-0526.2015.02.004
Wu, B., and Xu, X. F. (2021). Risk Assessment and Zoning of Lightning Disasters of Neijiang City. J.Plateau Mt. Meteorology Res. 41 (3), 115–120. doi:10.3969/j.issn.1674-184.2021.03.016
Xia, R. D., Zhang, D. L., and Wang, B. L. (2015). A 6-yr Cloud-To-Ground Lightning Climatologyand its Relationship to Rainfall over Central and Eastern China. J. Appl. Meteorology Climatol. 540 (12), 2443–2460. doi:10.1175/JAMC-D-15-0029.1
Xu, X. F. (2004). Lightning Induced Disasters and its Detecting and Forecasting. J. Meteorol. 30 (12), 17–21. doi:10.7519/j.issn.1000-0526.2004.12.004
Yin, X., Xiao, W. A., Feng, M. X., Jiao, X., Wang, Z. H., and Li, X. (2009). Regional Distribution and Vulnerability Regionalization of Lightning Disaster. J. Meteorological Sci. Technol. 37 (2), 216–222. doi:10.19517/j.1671-6345.2009.02.021
Yu, J. H., and Liu, H. B. (2020). Extreme Lightning Activity and its Correlation to Topography and Land Use Type. J. Meteorological Sci. Technol. 48 (6), 898–902. doi:10.19517/j.1671-6345.20190443
Yu, R., Zhang, X. L., Du, M. Y., Ma, H. Z., Yuan, H. F., and Zhu, C. L. (2021). Analysis of Characteristics of Cloud-To-Groundlightning: Activity of Thunderstorms over Different Topography in Central China. J. Trop. Meteorol. 37 (3), 329–340. doi:10.16032/j.issn.1004-4965.2021.031
Yuan, X. L., Zhou, Q., and Wang, Z. H. (2017). A Study on the Lightning Disaster Risk Gradimethods. J. Catastrophology. 32 (1), 26–31. doi:10.3969/j.issn.1000-811X.2017.01.006
Zhang, G. C. (2014). Theory and Methods in Natural Disaster Risk Assessment and Zoning. Beijing: China Meteorological Press.
Zhang, K., Yu, H. R., and Pu, J. W. (2021a). Study on Risk Assessment of Lightning Disaster in Sichuan Province. J. Agric. Catastrophology 11 (4), 130–131.
Zhang, W., Meng, Q., Ma, M., and Zhang, Y. (2011). Lightning Casualties and Damages in China from 1997 to 2009. Nat. Hazards 57 (2), 465–476. doi:10.1007/s11069-010-9628-0
Zhang, X. H., Wu, H. Y., Xu, X., Li, Y. C., and Jiao, S. M. (2007). An Analysis on Thunder Storm Vulnerability in Jiangsu Province. J. Sci. Meteorol. Sin. 27 (5), 536–542.
Zhang, Y., Han, J., Yuan, C. S., Yang, S., Li, C. L., and Sun, X. M. (2021b). A Rasterized Lightning Disaster Risk Method for Imbalanced Sets Using Neural Network. J. Comput. Mater. Continua 66 (1), 563–574. doi:10.32604/cmc.2020.012502
Keywords: lightning disaster, risk zoning, analytic hierarchy process, entropy weight method, cloud-to-ground lightning
Citation: Jin C, Shu Y, Han Z, Chen Q, He J and Wang S (2022) Lightning Disaster Risk Zoning in Jiangsu Province of China Based on the Analytic Hierarchy Process and Entropy Weight Method. Front. Environ. Sci. 10:943000. doi: 10.3389/fenvs.2022.943000
Received: 13 May 2022; Accepted: 23 May 2022;
Published: 13 July 2022.
Edited by:
Honglei Wang, Nanjing University of Information Science and Technology, ChinaReviewed by:
Xiufeng Guo, Wuxi University, ChinaZheng Shi, Nanjing University of Information Science and Technology, China
Copyright © 2022 Jin, Shu, Han, Chen, He and Wang. This is an open-access article distributed under the terms of the Creative Commons Attribution License (CC BY). The use, distribution or reproduction in other forums is permitted, provided the original author(s) and the copyright owner(s) are credited and that the original publication in this journal is cited, in accordance with accepted academic practice. No use, distribution or reproduction is permitted which does not comply with these terms.
*Correspondence: Yu Shu, njushuyu@sina.com