- 1Suzhou Center for Disease Control and Prevention, Suzhou, China
- 2National Institute of Parasitic Diseases, Chinese Center for Disease Control and Prevention, Chinese Center for Tropical Diseases Research, National Health Commission Key Laboratory of Parasite and Vector Biology, WHO Collaborating Center for Tropical Diseases, National Center for International Research on Tropical Diseases, Shanghai, China
- 3College of Geography and Remote sensing Sciences, Xinjiang University, Urumqi, China
- 4State Key Laboratory of Desert and Oasis Ecology, Xinjiang Institute of Ecology and Geography, Chinese Academy of Sciences, Urumqi, China
Objective: This study aimed to predict the spatial and temporal distribution pattern of Oncomelania hupensis (O. hupensis) on a fine scale based on ecological niche models, so as to provide insights into O. hupensis surveillance.
Methods: Geographic distribution and environmental variables of O. hupensis in Suzhou City were collected from 2016 to 2020. Five machine learning algorithms were used, including eXtreme gradient boosting (XGB), support vector machine (SVM), random forest (RF), generalized boosted (GBM), and C5.0 algorithms, to predict the distribution of O. hupensis and investigate the relative contribution of each environmental variable. The accuracy of the five ecological niche models was evaluated using the area under the receiver operating characteristic (ROC) curve (AUC) with ten-fold cross-validation.
Results: Five models predicted that the potential distribution of O. hupensis was in southwestern areas of Wuzhong, Wujiang, Taichang, and Xiangcheng counties. The AUC of RF, XGB, GBM, SVM, and C5.0 algorithms were 0.8233, 0.8051, 0.7938, 0.7897, and 0.7282, respectively. Comparing the predictive results and the truth of O. hupensis distribution in 2021, XGB and GBM models were shown to be more effective. The six greatest contributors to predicting potential O. hupensis distribution included silt content (13.13%), clay content (10.21%), population density (8.16%), annual accumulated temperatures of ≥0°C (8.12%), night-time lights (7.67%), and average annual precipitation (7.23%).
Conclusions: Environmental factors play a key role in the spatial and temporal distribution pattern of O. hupensis. The XGB and GBM machine learning algorithms are effective and highly accurate for fine-scale prediction of potential O. hupensis distribution, which provides insights into the surveillance of O. hupensis.
Introduction
Schistosomiasis japonica, a zoonotic parasitic disease caused by infection of the Schistosoma species, seriously endangers human health and socioeconomic development, which is one of the major global public health concerns (Song et al., 2016). China once bore the world’s highest burden of Schistosomiasis japonica (Zhou et al., 2021). Following concerted efforts for more than 70 years, remarkable achievements have been made in the national schistosomiasis control program of China (Cao et al., 2020; Yang et al., 2020). There were 74.89% of the total 450 schistosomiasis-endemic counties which achieved schistosomiasis elimination, 21.78% achieved transmission interruption, and 3.33% achieved transmission control by 2020 (Qian et al., 2019; Zhang et al., 2021). The shift moving toward schistosomiasis elimination suggests that the schistosomiasis control emphasis shifting from controlling the source of S. japonicum infections to risk monitoring, and the surveillance of the intermediate host O. hupensis distribution is the most important part for the monitoring of the schistosomiasis control risk (Gong et al., 2017).
Suzhou City was once highly prevalent for schistosomiasis in China with accumulative O. hupensis habitats of 414.33 km2. Following the long-term implementation of integrated interventions targeting schistosomiasis, including O. hupensis survey, O. hupensis control with chemical treatment and environmental improvements, the transmission of schistosomiasis has been effectively interrupted in Suzhou City, with more than 95% reduction in the area of O. hupensis habitats (Zhang, 2018; Li et al., 2019). Ecological environments play a key role in the distribution of O. hupensis snails, so there are still O. hupensis habitats found in local areas of the city, since the ecological environments have not completely changed. During the period from 2011 to 2020, a total of 0.683 km2 of O. hupensis habitats have been identified in Suzhou City, suggesting the long-term potential schistosomiasis transmission risk. O. hupensis survey is an important part of schistosomiasis control. The currently used massive sampling survey or census is time-consuming and high in cost, which is difficult for timely and accurate identification of the schistosomiasis transmission risk. Therefore, a rapid, accurate, and low-cost approach is urgently needed for the O. hupensis survey during the early stage of O. hupensis population spread, which may provide a valuable basis for O. hupensis control.
The ecological niche model, which combines environmental variables with known biological distribution, is effective in quantitatively describing the environmental factors associated with biological distribution and recognizing the environmental similarity with known distribution areas in the study regions through modeling based on machine learning algorithms, thus speculating the potential species distribution (Samy et al., 2018; Hu et al., 2020b). As an important tool in ecology and biogeography (Wang and Qiao, 2020), ecological niche models show a high ability for prediction of the geographical distribution of species, and have been widely used to map the temporospatial distribution of species (Mulieri and Patitucci, 2019; Wang et al., 2020; Gong Y. et al., 2021; Liu C. Y. et al., 2021; Ta et al., 2021; Yang et al., 2021), forecast the invasion of alien species (Wang et al., 2018), evaluate the effect of climate changes on species distribution (Liu et al., 2020), and identify the disease transmission risk (Alkishe et al., 2021). Liao’s study based on 16 ecological niche models found that climate changes were predicted to pose a great impact on the distribution of O. hupensis snails, resulting in north expansion and south shrinkage of the ecologically suitable O. hupensis habitats (Liao, 2011). Hu’s study based on 10 ecological niche models suggested that the areas at a high risk of schistosomiasis transmission were predicted to be mainly distributed in northern Heqing County, eastern Eryuan County, central Dali City, northeastern Weishan County, and northern Midu County (Hu et al., 2020a). This study aimed to predict the spatial and temporal distribution pattern of O. hupensis in Suzhou City using ecological niche models based on multiple environmental factors, so as to provide a basis for O. hupensis survey and control and assessment of the potential schistosomiasis transmission risk.
Materials and Methods
Study Area
Suzhou City is located in the lower reaches of the Yangtze River, in which there are plenty of rivers and lakes, and the area of rivers, lakes, and marshlands consists of 36.6% of total land areas in the city. In addition, Suzhou City has a moderate climate, abundant rainfall, fertile soil, and widespread vegetation, which is very suitable for O. hupensis breeding (Wang and Qiao, 2020).
Data Collection
O. hupensis distribution data, which were retrieved from O. hupensis habitat report cards in Suzhou City from 2016 to 2020, were provided by the Suzhou Center for Disease Control and Prevention (SZCDC), including the longitude, latitude, and area of O. hupensis habitats. There were 32 O. hupensis habitats found from 2016 to 2020, and all data were managed using the software Microsoft Excel 2013.
The datasets of factors affecting O. hupensis distribution in Suzhou City were collected, including five categories of geographical and environmental factors, climatic factors, socioeconomic factors, soil index, and vegetation index (Table 1), and 19 variables: altitude (ALT), distance from watercourse (DST), gradient, annual accumulated temperature of ≥0°C (AAT0), annual accumulated temperature ≥10°C (AAT10), aridity (AR), moisture index (MI), average annual precipitation (Pa), average annual temperature (Ta), gross domestic product (GDP), night-time lights (NTL), population density (PD), clay content, sand content, silt content, normalized difference vegetation index for the 1st quarter (NDVI01), normalized difference vegetation index for the 2nd quarter (NDVI02), normalized difference vegetation index for the 3rd quarter (NDVI03), and normalized difference vegetation index for the 4th quarter (NDVI04). Climatic data, socioeconomic status, soil index, and vegetation index were captured from the Resource and Environment Science and Data Center, Chinese Academy of Sciences (CAS) (https://www.resdc.cn/), and the geographical and environmental data were retrieved from the WorldPop Data Portal (https://www.worldpop.org). The administrative division map of Suzhou City was downloaded from the National Geomatics Center of China (http://www.ngcc.cn/ngcc/). All raster data were re-sampled to the resolution of 500 m × 500 m using the software ArcGIS version 10.2 and cut to match the map of Suzhou City for the subsequent analysis.
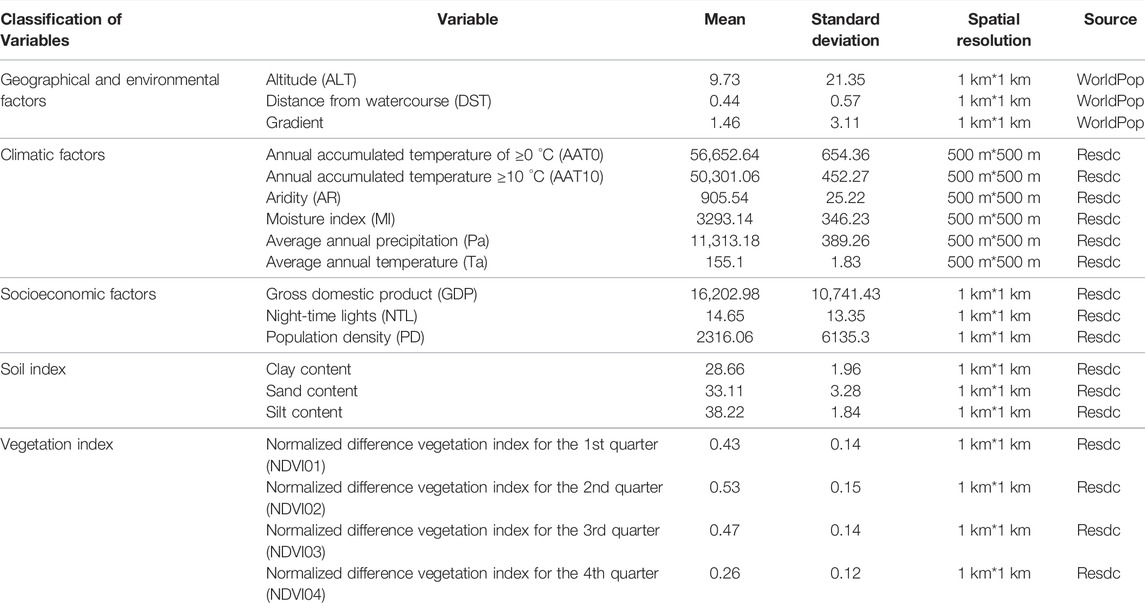
TABLE 1. Variables included for using the five ecological niche models for prediction of potential Oncomelania hupensis distribution in Suzhou City from 2016 to 2020.
Ecological Niche Modeling
Ecological niche models were used based on five machine learning algorithms using the Classification and Regression Training (CARET) package in the R version 3.6.1, including eXtreme gradient boosting (XGB), support vector machine (SVM), random forest (RF), generalized boosted (GBM), and C5.0 algorithms. O. hupensis habitats detected in Suzhou City from 2016 to 2020 and all background data were included in ecological niche models, and 80% were randomly selected as a training dataset, with 20% as a test dataset. The probability of O. hupensis distribution in each grid was estimated. The settings with a 0–30% probability of O. hupensis distribution were defined as non-suitable habitats, 30.1%–50% as low-probability suitable habitats, 50.1%–70% as moderate-probability suitable habitats, and 70.1%–100% as high-probability suitable habitats. The relative contribution of each variable to the prediction of potential O. hupensis distribution was estimated using the CARET package.
Assessment of the Predictive Accuracy of Ecological Niche Models
The accuracy of the five ecological niche models for the prediction of potential O. hupensis distribution was evaluated using the area under the receiver operating characteristic (ROC) curve (AUC) with ten-fold cross-validation. The mean values of AUC were calculated with a 95% confidence interval. The AUC mean value, ranging from 0 to 1, indicates the predictive accuracy of the ecological niche models, and an AUC value approaching 1 indicates higher accuracy (Hu, 2020).
Field Validation
A cross-sectional survey was conducted by means of systematic sampling and environmental sampling according to the Technical Guidelines for O. hupensis in China in Suzhou City in 2021, to investigate the longitude and latitude of O. hupensis habitats and O. hupensis density. The O. hupensis survey results were recorded in O. hupensis habitat report cards, and managed using the software Microsoft Excel 2013. The degree of concordance between the prediction results by ecological niche models and actual O. hupensis distribution was examined.
Results
Current Distribution of O. hupensis Habitats
A total of 0.659 km2 of O. hupensis habitats were found in Suzhou City during the period from 2016 to 2020, which peaked in 2018 (0.499 km2). During the 5-year study period, the highest mean density of O. hupensis was seen in 2017 (0.068 O. hupensis snails/0.1 m2), followed by 2016 (0.044 O. hupensis snails/0.1 m2), and O. hupensis habitats were predominantly identified in three townships of Huqiu, four townships of Wuzhong, two townships of Xiangcheng, and one township of Taicang (Figure 1).
During the 5-year period from 2016 to 2020, the largest O. hupensis habitats were identified in Wuzhong (0.252 km2), followed by in Xiangcheng (0.218 km2), Huqiu (0.183 km2), and Taichang (0.007 km2) (Table 2). O. hupensis habitats were found in Guangfu and Jinting townships of Wuzhong in each of the 5 years, and O. hupensis habitats were found in the Zhenhu Township of Huqiu and Dongshan Township of Wuzhong in 4 years, while O. hupensis habitats were detected in the Dongzhu Township of Huqiu during the past 3 years.
Ecological Niche Modeling and Prediction
Fine-scale ecological niche models were used based on five machine learning algorithms to predict the probability of O. hupensis distribution in Suzhou City. Suitable habitats of O. hupensis were predicted in Wuzhong, Wujiang, Taicang, and southwestern Xiangcheng by all five ecological niche models, and high-probability suitable habitats were found in central Wuzhong (Dongshan and Jinting townships) (Figure 2). The SVM and C5.0 algorithms predicted high-probability suitable habitats of O. hupensis in northern Wuzhong (Guangfu Township) and southwestern Xiangcheng (Wangting Township), and GBM and XGB models predicted high-probability suitable habitats in northern Wuzhong (Guangfu, Xukou, Xiangshan, and Hengjing townships) and central Huqiu (Jinghu and Dongzhu townships). Overall, the suitable habitats of O. hupensis predicted by GBM and C5.0 algorithms were predominantly located in the southern half of Suzhou City, and across the city by XGB, SVM, and RF models.
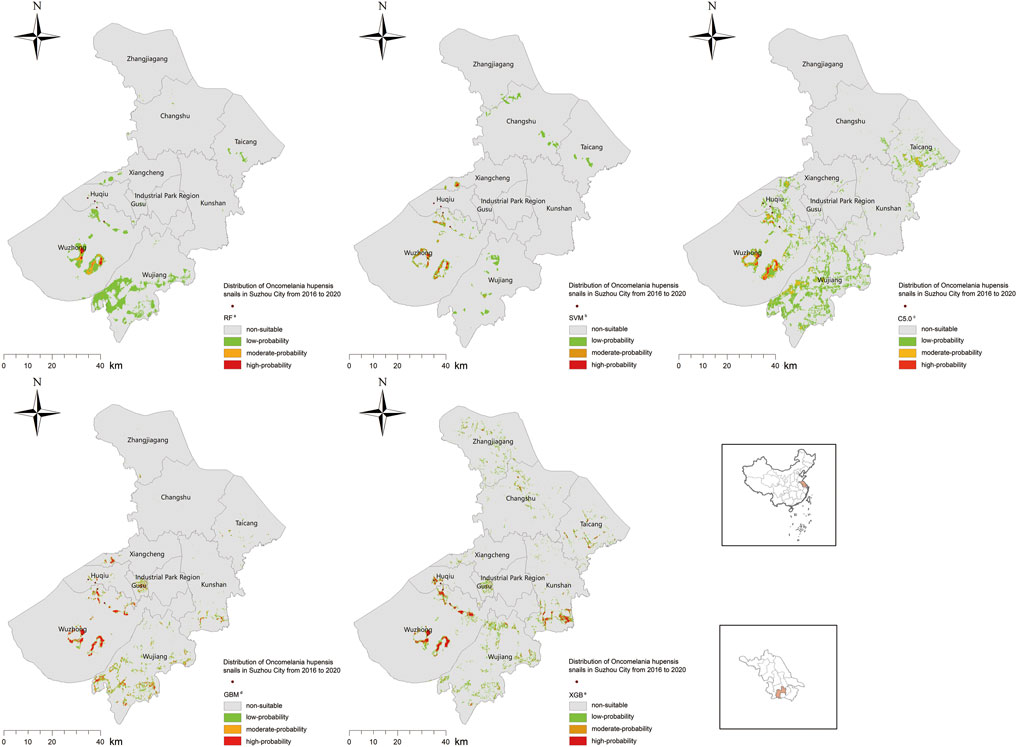
FIGURE 2. Prediction of suitable habitats of Oncomelania hupensis using five ecological niche models.
The C5.0 algorithm predicted the largest suitable habitats of O. hupensis (8.71% of total areas in Suzhou City), followed by RF (5.09%), GBM (5.06%), XGB (3.31%), and SVM models (2.2%), and the XGB model predicted the largest high-probability suitable habitats (0.7% of total areas in Suzhou City), followed by GBM (0.69%), C5.0 (0.26%), SVM (0.12%), and RF models (0.11%) (Table 3).
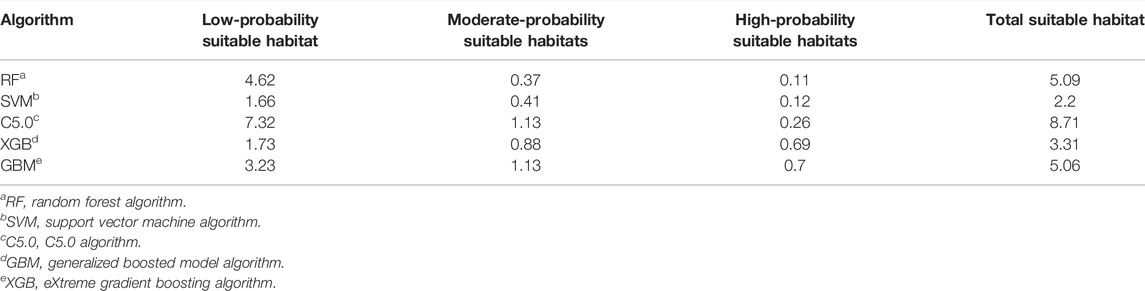
TABLE 3. Proportion of the predicted by low-, moderate-, and high-probability suitable habitats by five algorithms in total suitable habitats in Suzhou City (%).
Accuracy of Ecological Niche Models for Prediction of Potential O. hupensis Distribution
According to the ROC curve (Figure 3), the performance of prediction accuracy of these five models all showed a high accuracy (the AUC mean values > 0.7). The AUC mean values of RF, XGB, GBM, SVM, and C5.0 algorithms were 0.8233, 0.8051, 0.7938, 0.7897, and 0.7282, respectively. For the prediction performance of O. hupensis potential distribution in Suzhou City, the RF, XGB, and GBM indicated a higher accuracy among these five ecological niche models for the prediction of potential O. hupensis distribution.
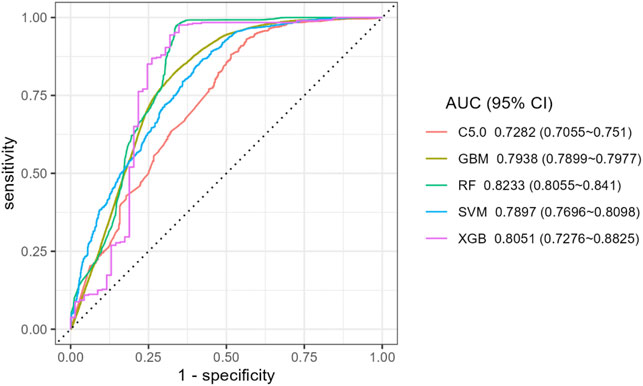
FIGURE 3. Area under the receiver operating characteristic curve (ROC) of five ecological niche models. C5.0, C5.0 algorithm; GBM, generalized boosted model algorithm; RF, random forest algorithm; SVM, support vector machine algorithm; XGB, eXtreme gradient boosting algorithm.
Among the 19 variables, the six greatest contributors to the prediction of O. hupensis distribution included the silt content in soil, clay content, population density, annual accumulated temperatures of ≥0°C, night-time lights, and average annual precipitation (Figure 4).
The results from the response-curve analysis showed that the silt content of more than 40%, clay content of less than 28%, population density between 2000 and 3000 persons/km2, annual accumulated temperatures of ≥0°C between 55,500 and 56,500°C days, night-time lights of more than 10 nW/cm2/sr, and average annual precipitation between 11,250 and 12,000 mm3 were the most suitable habitats for O. hupensis breeding (Figure 5).
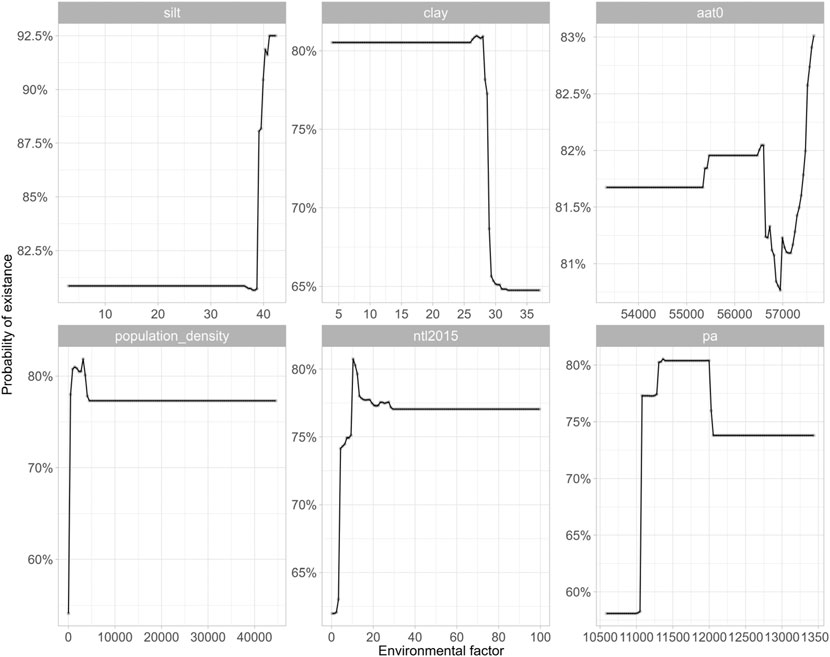
FIGURE 5. Response curve of the six greatest contributors to potential Oncomelania hupensis distribution.
O. hupensis survey was performed in current O. hupensis habitats and potential O. hupensis habitats predicted by ecological niche models in Suzhou City in 2021, and covered 83 townships (streets) and 1021 villages (communities) (Table 4). The O. hupensis survey covered an area of 14.825 km2, including 1.973, 1.856, 1.734, 0.171, 1.402, 0.582, 3.210, 0.050, 2.043, and 1.804 km2 in Huqiu, Wuzhong, Xiangcheng, Gusu, Wujiang, Industrial Park Region, Changshu, Zhangjiagang, Kunshan, and Taicang, respectively. A total of five O. hupensis habitats were found, which were located in Guangfu, Jinting, and Xiangshan townships of Wuzhong and Dongzhu and Zhenhu townships of Huqiu (Figure 2).
The forecast result of the C5.0 algorithm covered all these five habitats; however, only one habitat was located in the predicted high-probability suitable habitats, and the other four habitats were all located in predicted low- and moderate-probability suitable habitats. The predicted result of the XGB model covered four habitats, which were all located in the predicted high-probability suitable habitats. Three habitats were located in the predicted high-probability suitable habitats of the GBM model, with another approaching the predicted high-probability suitable habitats. In addition, RF and SVM models predicted only two habitats.
Discussion
O. hupensis is the only intermediate host of S. japonicum (Burton et al., 2019), and the O. hupensis survey is the most important part of schistosomiasis transmission risk monitoring (Gong et al., 2017; Huang et al., 2021). O. hupensis population expansion presents a specific pattern, widely influenced by climate factors such as ambient temperature, precipitation, and distribution of the river system. It may peak 2–3 years after colonization of O. hupensis populations after invading a new environment with a comfortable climate. It is very difficult to accurately identify the distribution of O. hupensis populations using conventional O. hupensis survey methods, which requires a large number of manpower and material resources. Therefore, precise prediction of suitable O. hupensis habitats is of great significance for O. hupensis surveys.
In this study, using climatic and environmental variables, five machine learning algorithm models GBM, C5.0, XGB, RF, and SVM algorithms, predicted the potential distribution of O. hupensis in Suzhou City accurately, which may be used to guide and optimize O. hupensis surveys. The climatic and ecological variables, including temperature and precipitation, were usually considered to be the most important impact factors for the snail distribution and they play a decisive role in many big-scale studies (Gong et al., 2022). However, considering the difference in the microenvironment and it may affect the survival of the snail in a fine-scale study (Liu M.-M. et al., 2021), more environmental variables were picked up into machine learning algorithm models, including the silt content in soil, clay content in soil, population density, and night-time lights (Zheng et al., 2014; Gao et al., 2015), and they show a significant contribution role for the O. hupensis distribution prediction in this study. These variables may have a direct or indirect effect on snail survival in a small-scale environment (Lackey and Horrall, 2021), and therefore, the prediction of five ecological niche models all showed AUC values of >0.7 for the prediction of potential O. hupensis distribution in Suzhou City, indicating a high predictive accuracy.
In the present study, based on the prediction of potential O. hupensis distribution by ecological niche models, conducting more surveys in high-probability suitable habitats of O. hupensis and fewer surveys in low-probability suitable habitats may allow the greatest likelihood for identification of O. hupensis habitats with the least workload. The predicted moderate- and high-probability suitable habitats were predominantly located in central and northern Wuzhong (Dongshan, Jinting, Guangfu, Xukou, Xiangshan, and Hengjing townships), southwestern Xiangcheng (Wangting Township) ,and central Huqiu (Zhenhu and Dongzhu townships). According to the 2021 O. hupensis survey in Suzhou City, C5.0, XGB, and GBM models were found to have the greatest accuracy for the prediction of potential O. hupensis distribution. However, the C5.0 algorithm predicted the largest suitable habitats of O. hupensis (8.71% of total areas in Suzhou City), and only one of the five habitats with O. hupensis was located in the predicted high-probability suitable habitats. XGB and GBM models, which were also accurate in predicting the potential distribution of O. hupensis snails, seem more effective to improve the detection of O. hupensis and save manpower, material, and financial resources than the C5.0 algorithm. To compare the efficiency of six ecological niche models for the prediction of potential O. hupensis distribution Zheng (2021) calculated the AUC, accuracy, Kappa value, sensitivity, and specificity of the models, and the XGB model was found to show high accuracy, sensitivity, and specificity. In a recent study to estimate the AUC and true skill statistic (TSS) values of 10 ecological niche models, GBM, multivariate adaptive regression splines (MARS), and RF models were found to have better performance than other models (Hu, 2020). InGong’s study, to predict the transmission risk of visceral leishmaniasis in the extension region of Loess Plateau, China, nine ecological niche models were used and RF and GBM models were reported to have higher predictive values (Gong Y. F. et al., 2021). In addition, GBM and RF models were found to present the greatest accuracy for fine-scale mapping of O. hupensis diffusion in Shanghai, and the prediction results by GBM and RF models were almost in agreement with field O. hupensis surveys during the recent years, which is consistent with our findings. Besides the AUC, Kappa value, a new statistical metric named DISO (Distance between Indices of Simulation and Observation) was developed to evaluate the overall performance of different models (Hu et al., 2019; Zhou et al., 2021). DISO will be employed in our future study to measure the different models' performance.
The high-probability suitable habitats of O. hupensis predicted by the five ecological niche models all covered Jinting and Dongshan townships of Wuzhong County, and these two high-probability suitable habitats are located in Xishan Island in Taihu Lake and along the margin of Taihu Lake in Dongshan Township. Previous studies have shown that ecological restoration projects may cause the re-breeding of O. hupensis (Mulieri and Patitucci, 2019; Wang et al., 2020; Gong Y. et al., 2021). Therefore, these two high-probability suitable habitats of O. hupensis should be given much attention. Even though no O. hupensis were detected along the margin of Taihu Lake in Dongshan Township in 2021, much attention should be paid during the 2022 O. hupensis survey. In addition, the five ecological niche models all predicted large suitable habitats of O. hupensis in Wujiang. There are plenty of lakes and rivers in Wujiang, and the water regions cover 267 km2, accounting for 22.69% of total areas in Wujiang. On October 12, 2021, Wujiang was designated as a demonstration region of national ecological cultivation construction by the Ministry of Ecology and Environment of the People’s Republic of China. Although low-probability suitable habitats of O. hupensis were predicted in Wujiang, high attention should be given to O. hupensis breeding. In terms of other infection diseases, with the global warming, the environment has been changed significantly, which plays a key role for the occurrence, transmission and outbreak of the infection disease (Wang et al., 2021). One health theory was proposed to develop a new system including human health, environmental health and animal health (Yang 2021; Lu 2021; Yang 2022). It provides a new approach to investigate the infection diseases according to the one health concept in future.
This study has three innovations. First, there have been few reports pertaining to fine-scale prediction of potential O. hupensis distribution based on ecological niche modeling. Second, field O. hupensis surveys were performed to validate the predictive accuracy of ecological niche models in this study. Therefore, our study offers more real and objective assessment. Third, our data may provide insights into the optimization of O. hupensis surveys. However, the current study has some limitations. The impact of geographical barriers on O. hupensis diffusion was not included in ecological niche models. Currently, the definition of ecological niche is mainly based on the BAM diagram, where B indicates biotic niche, A indicates abiotic niche, and M indicates movement (Liao, 2011; Alkishe et al., 2021). The biotic niche and abiotic niche jointly determine the suitable habitats of species; however, geographical barriers may restrict species diffusion (Hu, 2020). For example, GBM and XGB models predicted the high-probability suitable habitats of O. hupensis in Pingjiang, Guanqian, and Taohuawu of Gusu. Although there are lots of rivers in these blocks, concrete hardening is given along the river banks and vegetation is scattered along the streets, which forms barriers to directly affect O. hupensis diffusion. The inclusion of river modification and urbanization construction into ecological niche models may improve the accuracy of prediction of potential O. hupensis habitats, which deserves further investigation. Further studies to include geographical barriers data into ecological niche models seem justified.
Conclusion
In this study, ecological niche models were used based on five machine learning algorithms, including eXtreme gradient boosting (XGB), support vector machine (SVM), random forest (RF), generalized boosted (GBM), and C5.0 algorithms, to predict the 2021 potential distribution of O. hupensis with data from 2016 to 2020 in Suzhou, China. Comparing the predictive results and the truth, XGB and GBM models showed more effectiveness in the fine-scale prediction of potential O. hupensis distribution, which provides insights into the surveillance of O. hupensis snails. Based on the results, conducting more surveys in high-probability suitable habitats of O. hupensis and fewer surveys in low-probability suitable habitats may allow the greatest likelihood for identification of O. hupensis habitats with the least workload.
Data Availability Statement
The datasets used and analyzed during the current study are available from the corresponding author on reasonable request.
Author Contributions
Study designing: QS, JZ, ZH, SL.Data curation: QS, JZ, JW, ZH. Data analyses: QS, YG and JZ. Writing— original draft: QS, ZH and SL. Writing—review & editing: ZQ, JZ, ZH, JW and SL.
Funding
This research work was supported by the National Tutor System (Qngg2021031); National Natural Science Foundation of China (No.32161143036, No. 12001305); the National Key Research and Development Program of China (No. 2021YFC2300804); the Fifth Round of Three-Year Public Health Action Plan of Shanghai(No. GWV-10.1-XK13); the National Youth Talent Program (Grant No. E1190301), the Alliance of International Science Organizations (Grant No. ANSO-CR-KP-2021-02); the Shenzhen Science and Technology Program (JCYJ20210324101406019); Schistosomiasis Japonica, Endemic Disease and Parasitic Disease Foundation of Jiangsu Province (x202106). The funders had no role in study design, data collection and analysis, decision to publish, or preparation of the manuscript. The author would like to express gratitude to all the staff from provincial schistosomiasis control institutes, schistosomiasis control stations at the county level for their efforts on O. hupensis surveys.
Conflict of Interest
The authors declare that the research was conducted in the absence of any commercial or financial relationships that could be construed as a potential conflict of interest.
Publisher’s Note
All claims expressed in this article are solely those of the authors and do not necessarily represent those of their affiliated organizations, or those of the publisher, the editors, and the reviewers. Any product that may be evaluated in this article, or claim that may be made by its manufacturer, is not guaranteed or endorsed by the publisher.
References
Alkishe, A., Raghavan, R. K., and Peterson, A. T. (2021). Likely Geographic Distributional Shifts Among Medically Important Tick Species and Tick-Associated Diseases under Climate Change in North America: A Review. Insects 12 (3), 225. doi:10.3390/insects12030225
Cao, C.-L., Zhang, L.-J., Deng, W.-P., Li, Y.-L., Lv, C., Dai, S.-M., et al. (2020). Contributions and Achievements on Schistosomiasis Control and Elimination in China by NIPD-CTDR. Adv. Parasitol. 110, 1–62. doi:10.1016/bs.apar.2020.04.002
Gao, J. C., Zhou, Y. B., Li, L. H., Wu, J. Y., Zheng, S. B., Song, X. X., et al. (2015). Analysis of Relationship between Natural Death of Oncomelania Hupensis Snails and Water Level in Eastern Dongting Lake District. Chin. J. Schisto Control 27 (03), 302–305. doi:10.16250/j.32.1374.2015023
Gong, W., Hong, Q. B., Lv, S., Xu, J., and Li, S. Z. (2017). Research Progress of Control Techniques on Oncomelania Hupensis. Chin. J. Schisto Control 29 (02), 6. doi:10.16250/j.32.1374.2016177
Gong, Y., Li, Y., Li, Y., Zhang, L., Lv, S., Xu, J., et al. (2021a). The Potential Distribution Prediction of Oncomelania Hupensis Based on Newly Emerging and Reemergent Habitats - China, 2015−2019. China CDC Wkly. 3 (5), 90–93. doi:10.46234/ccdcw2021.023
Gong, Y. F., Hu, X. K., Zhou, Z. B., Zhu, H. H., Hao, Y. W., Wang, Q., et al. (2021b). Ecological Niche Modeling-Based Prediction on Transmission Risk of Visceral Leishmaniasis in the Extension Region of Loess Plateau, China. Chin. J. Parasitol. Parasit. Dis. 39 (02), 218–225. doi:10.12140/j.issn.1000-7423.2021.02.015
Gong, Y.-F., Hu, X.-K., Hao, Y.-W., Luo, Z.-W., Feng, J.-X., Xue, J.-B., et al. (2022). Projecting the Proliferation Risk of Oncomelania Hupensis in China Driven by SSPs: A Multi-Scenario Comparison and Integrated Modeling Study. Adv. Clim. Change Res. 13 (2), 258–265. doi:10.1016/j.accre.2022.02.004
Hu, Z., Chen, X., Zhou, Q., Chen, D., and Li, J. (2019). DISO: A rethink of Taylor diagram. International Journal of Climatology 39 (5), 2825–2832. doi:10.1002/joc.5972
Hu, X. K., Hao, Y. W., Xia, S., Guo, Y. H., Xue, J. B., Zhang, Y., et al. (2020a). Detection of Schistosomiasis Transmission Risks in Yunnan Province Based on Ecological Niche Modeling. Chin. J. Parasitol. Parasit. Dis. 38 (01), 80–86+94. doi:10.12140/j.issn.1000-7423.2020.01.012
Hu, X. K., Xia, S., Guo, Y. H., Hao, Y. W., Xue, J. B., Lv, S., et al. (2020b). Ecological Niche Modeling and its Applications in Research on Transmission Risks of Parasitic Diseases. Chin. J. Parasitol. Parasit. Dis. 38 (02), 238–244.
Hu, X. K. (2020). Study on the Evaluation of Schistosomiasis Transmission Based on Ecological Niche Modeling. Master.
Huang, S., Mao, Q., Mao, Q., Zhong, Q., Fan, X., Li, W., et al. (2021). Reappearance of Risk of Schistosomiasis Transmission and the Response after 27 Years of Interrupted Transmission - Guangdong Province, China, 2019. China CDC Wkly. 3 (51), 1093–1097. doi:10.46234/ccdcw2021.264
Lackey, E. K., and Horrall, S. (2021). “Schistosomiasis,” in StatPearls (Treasure Island, FL: StatPearls Publishing).
Li, W., Zhang, J. F., Wu, F., Shi, L., Xiong, C. R., Yao, Y. Y., et al. (2019). Progress of Interruption of Schistosomiasis Transmission in Jiangsu Province. Chin. J. Schisto Control 31 (06), 583–590. doi:10.16250/j.32.1374.2019184
Liao, J. S. (2011). Study on the Potential Transmission Risks of Schistosomiasis Japonica in China Based on Ecological Niche Models. Master.
Liu, C., Wolter, C., Xian, W., and Jeschke, J. M. (2020). Species Distribution Models Have Limited Spatial Transferability for Invasive Species. Ecol. Lett. 23 (11), 1682–1692. doi:10.1111/ele.13577
Lu, J. H. (2020). One Health: An effective strategy to tackle new challenges in human health. One Health Bull 1 (1), 2. doi:10.4103/2773-0344.329023
Liu, C. Y., Li, D. W., Yang, S. J., Pan, Z. L., Jin, R. H., Chen, F., et al. (2021a). Potential Suitable Area and Niche Shift of Different Ploidy Kiwifruit. Chin. J. Appl. Ecol. 32 (09), 3167–3176. doi:10.13287/j.1001-9332.202109.014
Liu, M.-M., Feng, Y., and Yang, K. (2021b). Impact of Micro-environmental Factors on Survival, Reproduction and Distribution of Oncomelania Hupensis Snails. Infect. Dis. Poverty 10 (1), 47. doi:10.1186/s40249-021-00826-3
Mulieri, P. R., and Patitucci, L. D. (2019). Using Ecological Niche Models to Describe the Geographical Distribution of the Myiasis-Causing Cochliomyia Hominivorax (Diptera: Calliphoridae) in Southern South America. Parasitol. Res. 118 (4), 1077–1086. doi:10.1007/s00436-019-06267-0
Qian, M.-B., Chen, J., Bergquist, R., Li, Z.-J., Li, S.-Z., Xiao, N., et al. (2019). Neglected Tropical Diseases in the People's Republic of China: Progress towards Elimination. Infect. Dis. Poverty 8 (1), 86. doi:10.1186/s40249-019-0599-4
Samy, A. M., Alkishe, A. A., Thomas, S. M., Wang, L., and Zhang, W. (2018). Mapping the Potential Distributions of Etiological Agent, Vectors, and Reservoirs of Japanese Encephalitis in Asia and Australia. Acta Trop. 188, 108–117. doi:10.1016/j.actatropica.2018.08.014
Song, L.-G., Wu, X.-Y., Sacko, M., and Wu, Z.-D. (2016). History of Schistosomiasis Epidemiology, Current Status, and Challenges in China: On the Road to Schistosomiasis Elimination. Parasitol. Res. 115 (11), 4071–4081. doi:10.1007/s00436-016-5253-5
Ta, Q., Li, Y. K., Fan, W. Q., Shan, J. H., Tu, X. B., Ying, Q., et al. (2021). Predicting the Potential Distribution of Chinese Pangolin Using the MaxEnt Model. Acta Ecol. Sin. 41 (24), 9941–9952. doi:10.5846/stxb202009152403
Wang, R., and Qiao, H. J. (2020). Matters Needing Attention about Invoking Ecological Niche Model in Epidemiology. Biodivers. Sci. 28 (05), 579–586. doi:10.17520/biods.2020155
Wang, Y. C., Zheng, P., and Pan, W. B. (2018). Predicting the Potential Suitable Distribution Area of Pomacea Canliculate in China Based on the GARP Ecological Niche Modeling. J. Fujian Agric. Forest. Univ. Nat. Sci. Ed. 47 (01), 21–25. doi:10.13323/j.cnki.j.fafu(nat.sci.).2018.01.004
Wang, X., Xue, J., Xue, J., Xia, S., Han, S., Hu, X., et al. (2020). Distribution of Suitable Environments for Phlebotomus Chinensis as the Vector for Mountain-type Zoonotic Visceral Leishmaniasis - Six Provinces, China. China CDC Wkly. 2 (42), 815–819. doi:10.46234/ccdcw2020.223
Wang, X., Yin, G., and Hu, Z. (2021). Dynamical variations of the Global COVID-19 Pandemic based on a SEICR disease model: a new approach of Yi Hua Jie Mu. GeoHealth 5, 2021GH000455. doi:10.1029/2021GH000455
Yang, F., Zhou, Z., Zhou, Z., Fang, Y., Feng, X., Chen, Q., et al. (2020). Surveillance Progress for Crucial Vector-Borne Parasitic Diseases in China. China CDC Wkly. 2 (33), 638–642. doi:10.46234/ccdcw2020.177
Yang, K., Zhao, X. P., Zhang, X., and Zhu, D. (2021). Prediction of Potential Distribution of Mongolian Medicine Panzerina Lanata Var. Alaschanica Based on Maxent Niche Model. J. Chin. Med. Mater. (08), 1–5. doi:10.13863/j.issn1001-4454.2021.08.007
Yang, J. (2021). One Health: Transboundary challenges and prospect for cooperation. One Health Bull 1 (1), 1. doi:10.4103/2773-0344.329022
Yang, J. (2022). "One Health" perspective and management strategy of public health. One Health Bull 2, 1. doi:10.4103/2773-0344.342344
Zhang, L. J., Xu, Z. M., Yang, F., Dang, H., Li, Y. L., Lv, S., et al. (2021). Endemic Status of Schistosomiasis in People's Republic of China in 2020. Chin. J. Schisto Control 33 (03), 225–233. doi:10.16250/j.32.1374.2021109
Zhang, L. M. (2018). Schistosomiasis Control in Suzhou Area (1950-1990). Master, Suzhou University of Science and Technology.
Zheng, S. B., Li, L. H., Zhou, Y. B., Wu, J. Y., Song, X. X., He, Z., et al. (2014). Contrastive Analysis of Environmental Factors between Oncomelania Hupensis Snail Marshland and Snail Natural Death Marshland in Eastern Dongting Lake Schistosomiasis Endemic Areas. Chin. J. Schisto Control 26 (02), 121–126. doi:10.16250/j.32.1374.2014.02.011
Zheng, J. X. (2021). Prediction on Transmission Risk of Schistosomiasis and Liver Flukes Diseases in China and Mekong River Basin. Doctor.
Zhou, Q., Chen, D., and Hu, Z. (2021). Decompositions of Taylor diagram and DISO performance criteria. International Journal of Climatology 41 (12), 5726–5732. doi:10.1002/joc.7149
Keywords: spatial and temporal distribution, ecological niche model, Oncomelania hupensis, Suzhou City, environmental factors
Citation: Shi Q, Gong Y, Zhao J, Qin Z, Zhang J, Wu J, Hu Z and Li S (2022) Spatial and Temporal Distribution Pattern of Oncomelania hupensis Caused by Multiple Environmental Factors Using Ecological Niche Models. Front. Environ. Sci. 10:942183. doi: 10.3389/fenvs.2022.942183
Received: 12 May 2022; Accepted: 31 May 2022;
Published: 12 July 2022.
Edited by:
Yanfang Sang, Institute of Geographic Sciences and Natural Resources (CAS), ChinaReviewed by:
Mingwang Shen, Xi’an Jiaotong University, ChinaYuanjian Yang, Nanjing University of Information Science and Technology, China
Copyright © 2022 Shi, Gong, Zhao, Qin, Zhang, Wu, Hu and Li. This is an open-access article distributed under the terms of the Creative Commons Attribution License (CC BY). The use, distribution or reproduction in other forums is permitted, provided the original author(s) and the copyright owner(s) are credited and that the original publication in this journal is cited, in accordance with accepted academic practice. No use, distribution or reproduction is permitted which does not comply with these terms.
*Correspondence: Zengyun Hu, aHV6ZW5neXVuQG1zLnhqYi5hYy5jbg==; Shizhu Li, bGlzekBjaGluYWNkYy5jbg==