- 1Department of Meteorology, University of Reading, Reading, United Kingdom
- 2Department of Atmospheric Physics, Wuppertal, Germany
- 3Institute of Energy and Climate Research, Stratosphere (IEK-7), Forschungszentrum Jülich, Jülich, Germany
- 4Department of Biogeochemical Integration, Max Planck Institute for Biogeochemistry, Jena, Germany
- 5Institute of Environmental Physics, University of Bremen, Bremen, Germany
- 6Department of Geography, College of Life and Environmental Sciences, University of Exeter, Exeter, United Kingdom
- 7National Centre for Earth Observation, Department of Physics and Astronomy, University of Leicester, Leicester, United Kingdom
- 8National Centre for Earth Observation, Plymouth Marine Laboratory, Plymouth, United Kingdom
- 9Jülich Supercomputing Centre, Forschungszentrum Jülich, Jülich, Germany
- 10School of Mathematics and Statistics, Faculty of Science, The University of Sheffield, Sheffield, United Kingdom
- 11Federal Office for the Environment, Bern, Switzerland
- 12Department of Chemistry, University of Cambridge, Cambridge, United Kingdom
- 13European Centre for Medium Range Weather Forecasts, Bonn, Germany
- 14LEGOS-CNES, Toulouse, France
- 15Universidad de Alcalá, Department of Geology, Geography and the Environment, Alcala de Henares, Spain
- 16Laboratoire des Sciences du Climat et de l'Environnement, Gif sur Yvette, France
- 17NASA Jet Propulsion Laboratory, California Institute of Technology (Retired), Pasadena, CA, United States
- 18European Centre for Medium-Range Weather Forecasts, Reading, United Kingdom
- 19Center for Climate Change Research, Indian Institute of Tropical Meteorology, Pune, India
- 20Helmholtz GFZ German Research Centre for Geosciences, Section 1.4 Remote Sensing and Geoinformatics, Potsdam, Germany
- 21Institute of Planetary Geodesy, Technische Universität Dresden, Dresden, Germany
- 22The Swedish Environmental Protection Agency, Stockholm, Sweden
- 23Sierra Leone Meteorological Agency, Freetown, Sierra Leone
- 24National Centre for Earth Observation, Department of Meteorology, University of Reading, Reading, United Kingdom
- 25German Federal Environment Agency, Dessau, Germany
- 26ENVEO Environmental Earth Observation IT GmbH, Innsbruck, Austria
- 27Department of Geography, University of Zurich, Zurich, Switzerland
- 28Deutsches Zentrum für Luft- und Raumfahrt e. V. (DLR), Wessling, Germany
- 29Met Office Hadley Centre (MOHC), Exeter, United Kingdom
- 30Centre Interprofessionnel Technique d'Etudes de la Pollution Atmosphérique, Paris, France
- 31German Weather Service, Offenbach, Germany
- 32Group on Earth Observations (GEO), Geneva, Switzerland
- 33Planet, Haarlem, Netherlands
- 34INRAE ISPA, Villenave d'Ornon, France
- 35School of Ocean Sciences, College of Environmental Sciences and Engineering, Bangor University, Bangor, United Kingdom
Space-based Earth observation (EO), in the form of long-term climate data records, has been crucial in the monitoring and quantification of slow changes in the climate system—from accumulating greenhouse gases (GHGs) in the atmosphere, increasing surface temperatures, and melting sea-ice, glaciers and ice sheets, to rising sea-level. In addition to documenting a changing climate, EO is needed for effective policy making, implementation and monitoring, and ultimately to measure progress and achievements towards the overarching goals of the United Nations Framework Convention on Climate Change (UNFCCC) Paris Agreement to combat climate change. The best approach for translating EO into actionable information for policymakers and other stakeholders is, however, far from clear. For example, climate change is now self-evident through increasingly intense and frequent extreme events—heatwaves, droughts, wildfires, and flooding—costing human lives and significant economic damage, even though single events do not constitute “climate”. EO can capture and visualize the impacts of such events in single images, and thus help quantify and ultimately manage them within the framework of the UNFCCC Paris Agreement, both at the national level (via the Enhanced Transparency Framework) and global level (via the Global Stocktake). We present a transdisciplinary perspective, across policy and science, and also theory and practice, that sheds light on the potential of EO to inform mitigation, including sinks and reservoirs of greenhouse gases, and adaptation, including loss and damage. Yet to be successful with this new mandate, EO science must undergo a radical overhaul: it must become more user-oriented, collaborative, and transdisciplinary; span the range from fiducial to contextual data; and embrace new technologies for data analysis (e.g., artificial intelligence). Only this will allow the creation of the knowledge base and actionable climate information needed to guide the UNFCCC Paris Agreement to a just and equitable success.
Introduction
The UNFCCC Paris Agreement aims to strengthen the global response to the threat of climate change by keeping the increase in global mean temperature this century to well below 2°C above pre-industrial levels, and to pursue efforts to limit the temperature increase even further to 1.5°C through multi-lateral directed mitigation, adaptation, and finance measures (UNFCCC, 2015a). The transition to a low-emission and climate-resilient world requires not only strengthened international cooperation and technological advances, but also scientific evidence and guidance. Importantly, the relevance of science in underpinning political action was enshrined in the leading paragraph of the Glasgow Climate Pact—the decision of the UN Climate Change Conference 2021 (COP26)—by stating “[The conference of the Parties] Recognizes the importance of the best available science for effective climate action and policymaking”.
Space-based Earth observation (hereafter EO), yielding information on the Earth’s atmosphere, biosphere, cryosphere, land and oceans, is an essential component of the wider observing system and a necessary tool for successful implementation of the Paris Agreement, recognised in its Article 7.7c where the Parties are specifically called upon to “…[strengthen] scientific knowledge on climate, including research, systematic observation of the climate system, and early warning systems, in a manner that informs climate services and supports decision-making” (UNFCCC, 2015b). However, the most effective approach for integrating and exploiting the information provided by EO to generate the knowledge base needed for implementation of the Paris Agreement is far from clear. The Global Climate Observing System (GCOS) defines about 50 essential climate variables (ECVs), two-thirds of which are derived from space-based observations, with the remainder obtained from in situ measurements (https://gcos.wmo.int/en/essential-climate-variables/table). A framework is needed to translate the information from these ECVs into knowledge that helps to answer specific policy questions. The EO community also needs to clearly identify the space-based products (e.g., imagery, estimates of greenhouse gases from spectroscopic or above ground biomass derived from microwave observations) that are most appropriate for answering specific questions. Early dialogue with policymakers and other stakeholders is therefore critical to identify end-user needs and to define requirements for building suitable indicators and tracing progress of adaptation and mitigation efforts, while involvement of the climate research community and the private sector is key to driving innovation in providing EO-based climate information and knowledge.
Significant efforts to address climate policy needs are ongoing. The international EO community and its organised entities including the World Meteorological Organisation (WMO), the Committee on Earth Observation Satellites (CEOS), and the Group on Earth Observations (GEO), among others, have established several working groups that help coordinate the research in the areas of observations for mitigation (e.g., GEO et al., 2021) and adaptation science (e.g., Michetti et al., 2021). For example, entities within WMO, including WMO Integrated Observing System (WIGOS), Global Climate Observing System (GCOS), Global Ocean Observing System (GOOS), Global Terrestrial Observing System (GTOS), and the Global Atmosphere Watch (GAW), produce products that address the needs of science users. Additional effort is needed to refine these products to respond to policy needs. Climate services, coordinated by a UN-wide initiative within the WMO, the Global Framework for Climate Services (GFCS), have made some progress towards bridging the gap between science and end-users by tailoring climate information derived from observations and modelling capacities to support decision-making (Hewitt et al., 2020). The Copernicus Climate Change Service (C3S), implemented by the European Centre for Medium-Range Weather Forecasts (ECMWF) on behalf of the European Union, and the National Oceanic and Atmospheric Administration (NOAA) Climate Services Portal (climate.gov) in the US, among other national climate services, are key repositories for climate data from which relevant adaptation and mitigation information can be drawn. These repositories also have proven to be greatly beneficial to the development of early warning systems and for disaster management. However, as highlighted by Findlater et al. (2021), climate services currently mainly focus on delivering better data and on improving products, rather than engaging in discourse with social sciences and the end-user to deliver truly demand-driven services that ultimately would lead to the transformational change needed to deal with climate change. There is, on the other hand, also a wealth of small-to-medium businesses that have started to deliver innovative, important, and useful climate information and solutions. The European Commission’s Destination Earth is a digital-twin project with the aim of further advancing the generation of and access to climate data and services in the future. The contributions of EO to these climate services are multi-faceted and often indirect, through their integration into data assimilation systems, including weather forecasting and carbon models. For example, research programmes such as the European Space Agency’s (ESA) Climate Change Initiative (Hollmann et al., 2013; Popp et al., 2020) perform the groundwork for the generation of the fundamental, high-quality data records from satellite observations accessible via the C3S. Also, the US National Aeronautics and Space Administration (NASA) provides a range of high-quality climate data records of satellite observations. The EUMETSAT Climate Monitoring Satellite Application Facility (CM SAF), amongst other SAFs, generates high quality climate data records from long records of operational weather forecasting satellite information. In situ measurements from coordinated and cross-calibrated observation networks (e.g., for land: GTN-G, GTN-H, GTN-P; for atmosphere: GRUAN, AGAGE, AERONET; for ocean: Argo, DBCP, GLOSS, see https://gcos.wmo.int/en/networks/ for abbreviations), when used for the validation of satellite information, are also key to ensure and assure the quality of EO data. Finally, large international research collaborations such as within RECCAP (Regional Carbon Cycle Assessment and Processes) (https://www.globalcarbonproject.org/reccap/index.htm; Canadell et al., 2012) contribute to quantifying global and regional GHG budgets. A vision for how these streams of activities could be further explored and developed to support the Paris Agreement has been provided by the UNFCCC’s Subsidiary Body for Scientific and Technological Advice (SBSTA), through its ad hoc coordination group on systematic observations (https://www4.unfccc.int/sites/SubmissionsStaging/Documents/202203012343---SO-in-GST-2022-final.pdf).
In the transdisciplinary analysis presented here, which brings together EO researchers, climate scientists, policymakers, and representatives from national agencies and the private sector, we take a holistic view of the landscape and suggest a potential way forward for exploiting EO in support of the Paris Agreement (Figure 1). The landscape obviously involves both policy and science, but also what we call “theory” and “practice” (recognizing that these terms should not be over-interpreted). We consider the theoretical aspect of policy as being the global, legal (UNFCCC) framework, including the Paris Agreement, and its practical aspect the implementation at national level. Within the science domain, we consider the theoretical aspect to be physical understanding of the climate system (including climate modelling), and the practical aspect the observation of the behaviour of the climate system. This framing is useful to clarify the role of EO in the present analysis, because there is often something of a disconnect between physical understanding and statistical analysis of observations in the practice of climate science (Shepherd 2021). By crossing the boundaries between policy and science, and between what we characterize as theory and practice, we aim to define an integrating methodological framework which provides policymakers with a knowledge base (or EO-derived evidence) that enables decision-making within the Paris Agreement context.
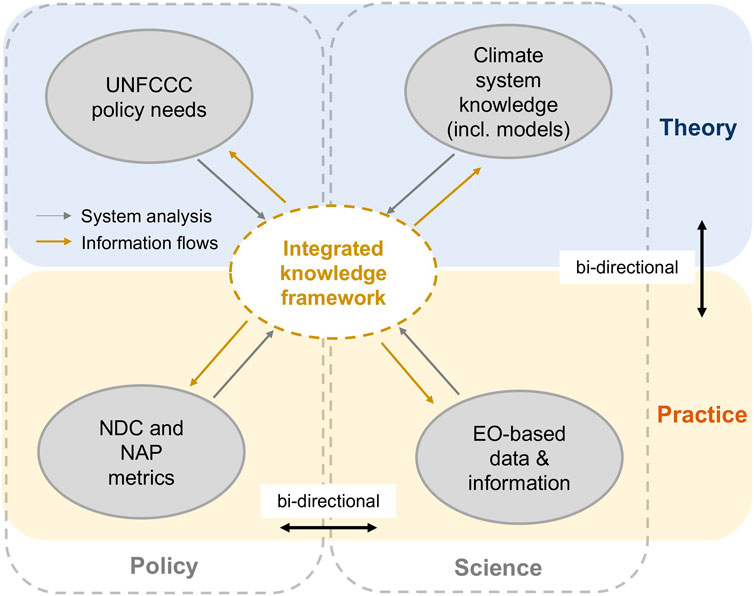
FIGURE 1. Multi-directional and trans-disciplinary methodological approach used in this study to derive an integrated knowledge framework that considers needs and opportunities from policy, science, theory, and practice on an equal footing. NDC and NAP are Nationally Determined Contributions and National Adaptation Plans. EO here refers to all available Earth observations, though the specific focus of this paper is on space-based observations.
This approach was chosen in order to derive a conceptual framework from reality, rather than deriving reality from a conceptual framework (paraphrasing Schumacher 1973, p240). Although climate assessment frameworks (such as the WMO/UNEP (United Nations Environment Programme) Scientific Assessment on Ozone Depletion or the Intergovernmental Panel on Climate Change (IPCC) Assessment Reports) are predicated on a clear separation between science and policy—sometimes called the “value-free ideal”—which can easily lead to a detachment from reality, it is increasingly acknowledged that societal values, rather than introducing biases, are in fact integral to guiding research and setting its aims (Pulkkinen et al., 2022). A failure to consider the realities involved in policy-making, including factors such as pragmatism, competing interests, values, and path dependencies (Rose 2015), can lead to a failure in using science (i.e., evidence) to address environmental problems through the transfer of knowledge to policy (Sarewitz 2004; Rose 2014; Thompson and Warburton 2019). Critically, the EO community needs to better understand the diverse needs of government operators who are attempting to implement mitigation or adaptation plans. For example, what kind of data are needed to support city planners trying to adapt to the increasing frequency of heat waves, droughts, fires, and flooding events? How can EO data support the needs of the agriculture and fishery sectors responding to threatened livelihoods? What kinds of EO data are needed to support members of the insurance and re-insurance communities who are providing financial protection against increasing climate risks? Crucially, this understanding will have to evolve as the climate continues to warm. In our study, societal values are brought in through the involvement of policy-makers and stakeholders.
This study focuses solely on space-based EO (which generally yields information with global coverage) although many of the same principles apply to other essential parts of the integrated global observing system, such as long-standing ground-based in situ measurement networks or observation systems using buoys, floats, balloons, or aircraft platforms. Although also not elaborated in further detail, the production of climate information from EO clearly depends on trans-disciplinary collaborations and methods, including multi-sensor (e.g., synthetic aperture radar and multi-spectral imaging for human settlement detection, Marconcini et al., 2020) and model-data fusion (such as envisaged in Digital Earth, Goodchild et al., 2012), data assimilation based on numerical weather prediction (for the production of dynamical reanalyses) and Earth system models (ESMs) (for the production of emissions estimates of greenhouse gases), and artificial intelligence (AI) approaches. This paper is directed primarily at the observation and climate research communities (including those in the private sector) to provide a fresh perspective on how EO research and its use in climate services could be made more valuable to policymakers. However, it is also directed at policymakers, national agencies, and other stakeholders to make them aware of the potential of EO in supporting their efforts to fulfil the requirements of the Paris Agreement. It should be noted that while the Paris Agreement is a legally-binding treaty (see https://www.un.org/en/climatechange/paris-agreement), most of its “objectives” are implemented on a best-effort basis. Thus, the aspect of how EO could support law enforcement is not treated in this study.
To meet the study’s objectives, we first summarise the legal text of the Paris Agreement to unpack the policy goals of the international treaty and thus indirectly its needs (illuminating the upper left process in Figure 1, and referred to below as step 1). The implication of these policy needs for implementation by national agencies and governments is then analysed (lower left in Figure 1, step 2). Next, these policy needs derived from the legal framework are put in the context of our scientific knowledge of the Earth (or climate) system (upper right in Figure 1, step 3). Finally, we use currently available examples of EO-derived metrics and indicators to help explore the potential of EO in the form of case studies (lower right in Figure 1, step 4). Gaps in the EO-derived knowledge base are identified, and some research and development needed to fill these gaps is formulated. The combined knowledge gained from this multi-directional analysis is then used to define a comprehensive, holistic framework as an aim for EO science to pursue. The suggested framework should particularly focus on how the uncertainties that are associated with climate information are represented. Particularly when the uncertainties are large, they may be misinterpreted by the user as indicating absence of knowledge rather than a range of possibilities (Corner et al., 2012; Løhre et al., 2019), thereby preventing and paralysing action (Kahneman and Tversky, 1982).
Step 1—Identifying UNFCCC Paris Agreement policy needs
An overview of the structure of the Paris Agreement’s legal text (UNFCCC, 2015a) is provided in Figure 2. The purpose of the Paris Agreement (cf., Klein et al., 2017) is set out in its Article 2, with the general aims of mitigation (the temperature goal mentioned above), adaptation (to enhance adaptive capacity and resilience to climate change), and financial flows (to make financing compatible with resilient and low GHG emissions development). From these aims follow the objectives of the Paris Agreement, with EO potentially providing valuable information on five out of seven of the most important objectives, namely mitigation, maintaining sinks and reservoirs, adaptation, protecting against loss and damage, and capacity building.
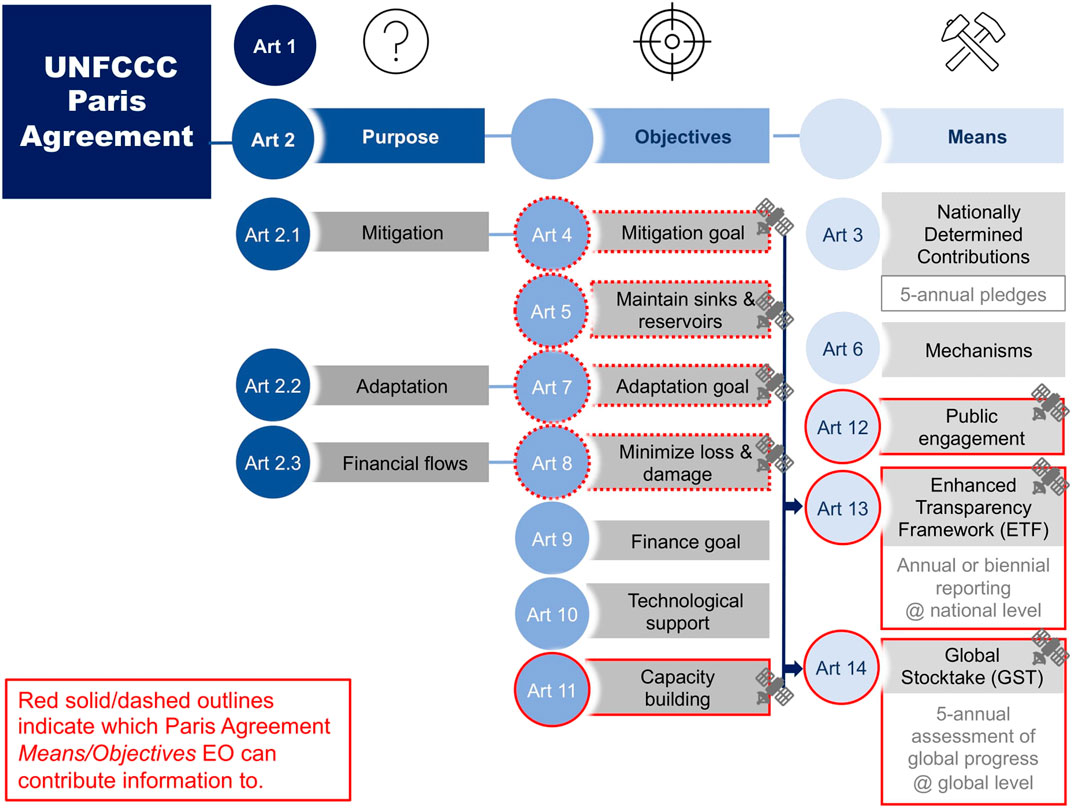
FIGURE 2. The UNFCCC Paris Agreement’s legal framework, focusing on the main Articles and the relationships between them. Article 1 defines the actors, Article 2 the purpose, Articles 4–5 and 7–11 the objectives, and Articles 3, 6, and 12–14 the available means of the Paris Agreement. Red circles (and grey satellite icons) denote where there is potential for EO to support the Paris Agreement’s objectives (dotted) and available means (solid). The blue vertical line indicates that all objectives are covered in the ETF and GST. Note, the reporting frequency within the ETF depends on the country.
While the first four of these objectives are discussed in case studies presented below, we first briefly discuss capacity building. Capacity building should be considered as a means to reach the Paris Agreement’s goals by getting maximum benefit from EO or other information, rather than a part of the Paris Agreement’s objectives. Understanding capacity building as a means to an end and not an end in itself (Eade, 1997) may ultimately lead to more directed and effective actions toward reaching the Paris Agreement’s climate goal. Indeed, enabling particularly the least-developed countries to take ownership of the Paris process and contribute in an active way to climate action was identified as a key priority at COP26. Exemplary work in this direction is already ongoing (e.g., within WIGOS).
Furthermore, EO could improve the efficiency of the means of implementation mandated by the Paris Agreement (see Figure 2). For example, it could provide quantitative information on key aspects of mitigation and adaptation for use within the Enhanced Transparency Framework (ETF) and document the overall progress toward the Paris Agreement’s goals within the Global Stocktake (GST; UNFCCC, 2015c). It could also provide meaningful pictures and stories used for public engagement (key to building the support base for policy and climate action).
Step 2—Identifying the UNFCCC Paris Agreement demands on national agencies
The long-term success of the Paris Agreement is acknowledged to be crucially dependent on the successful design of the operational tools for its implementation (World Resources Institute, 2021). To this end, the Paris Agreement has formalised a continual improvement cycle (also called ambition cycle or ratcheting-up mechanism, see Figure 3), which consists of planning, implementing, reporting, and reviewing the different national climate pledges every 5 years with the aim of raising ambition and strengthening climate action. The reporting duties within the ambition cycle are performed by national government agencies as part of the ETF, and are put in place to build mutual trust and confidence in the process as well as to promote its effective implementation.
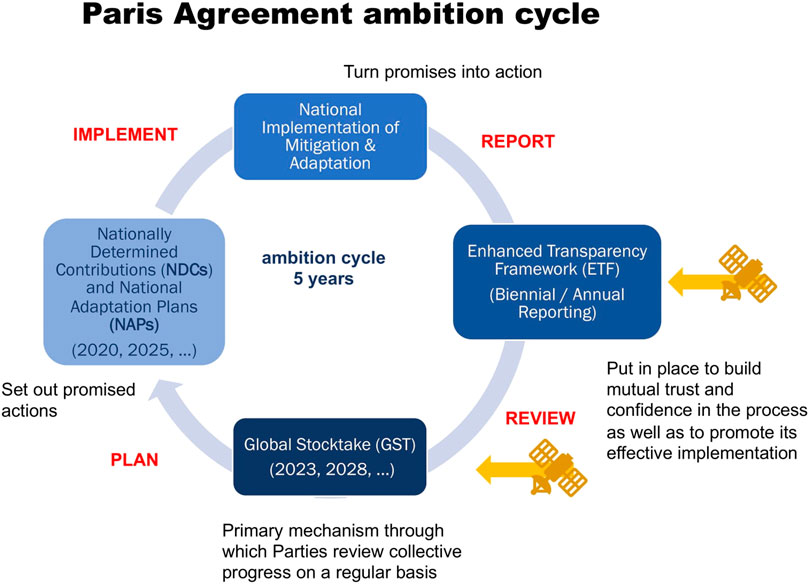
FIGURE 3. The UNFCCC Paris Agreement’s ambition cycle, which aims at strengthening the commitment of the world’s nations to combat climate change with defined milestones that repeat every 5 years. The ETF provides guidelines to countries on reporting of GHG emissions and thus progress toward NDCs, on adaptation to climate change impacts and thus progress on NAPs, and on both provided and received financial support (not treated further here) in documents known as Biennial Transparency Reports (BTRs). The ETF also aims at providing streamlined information for use in the GST. EO can help support the ETF in both the national reporting and global reviewing tasks (yellow arrows and satellite symbols). Adapted from Figure 1 in UNFCCC Climate Change Secretariat (2022).
EO represents an essential source of information that can support the ambition cycle and thus the successful implementation of the Paris Agreement by contributing to both the reporting activities at national levels and the reviewing stage for a global perspective on progress towards the Paris Agreement’s overall goals, i.e., the GST. Within the ETF, EO can, for example, help with reporting of anthropogenic GHG emissions (DeFries et al., 2007; Janssens-Maenhout et al., 2020; Palmer et al., 2021; Perugini et al., 2021; Deng et al., 2022) and emissions or removals in the LULUCF (land use, land-use change, and forestry) and agriculture sectors (Brandt et al., 2018; Fan et al., 2019) at the national level, especially in least developed countries, where in situ measurement networks (including data on land use and land use change) may provide insufficient information. EO will also be fundamental in helping countries assess loss and damage due to extreme climate events as well as their success in developing and implementing adaptation measures (Ahamed and Bolten, 2017; Chuvieco et al., 2019). In support of the GST, EO can provide aggregated information on the state of the climate (e.g., Blunden and Boyer, 2021; WMO, 2022) and thus the overall effectiveness of policy measures for mitigation and adaptation and their implementation. Such information informs governmental stakeholders and allows for evidence-based decision-making through expert assessments, the IPCC Assessment Reports, and, more generally, climate research as published in scientific articles.
Step 3—Adopting an Earth system perspective to frame the UNFCCC Paris Agreement
Integrated theoretical knowledge of the climate system is necessary to overcome challenges met when trying to assess progress towards the goals and to satisfy the requirements laid out by the Paris Agreement, which is written from a legal rather than a scientific perspective. Several challenges have already been recognised, not least by climate activists, who are sceptical of the effectiveness of current political action to combat climate change (e.g., Greta Thunberg, Youth4Climate Summit 2021). Moreover, if not addressed, these challenges risk destroying trust in the process between nations. While EO and climate scientists are often well aware of the challenges involved in assessing progress towards the Paris commitments (Victor et al., 2017), they need to provide more focussed, tailored, and compelling policy-relevant evidence to persuade policy-makers that these challenges are not being addressed and need further attention.
One challenge is to relate the emissions reported by countries in their BTRs on national GHG inventories to the levels of GHG concentrations observed in the atmosphere. BTRs only provide information on a country’s anthropogenic emissions and removals (with the notable exception of international military, aviation and ship emissions which are included only as memo-items rather than in the national totals), derived from either bottom-up estimates based on default IPCC or country-specific emission factors (sometimes derived from measurements), statistical approaches together with emission factors (e.g., for livestock emissions), specific modelling (e.g., for road traffic, cropland soil carbon), or detailed statistics on energy use. However, to relate these bottom-up to top-down estimates from global observations, and to ultimately measure progress in the reduction of GHG emissions within the GST, estimation of variations in natural GHG sources and sinks is also necessary in order to balance observed global GHG budgets (see also section EO to quantify sinks and reservoirs).
Over the time period 2011–2020, 26% of emitted carbon dioxide (CO2) was taken up by the ocean and another 29% by the land biosphere (including soils), sink capacities that are expected to change (Friedlingstein et al., 2021). Rising ocean temperatures, increasing ocean acidity, and climate-change-induced degradation of the biosphere, including soils (e.g., through wildfires or desertification), are likely to diminish sink capacity, although the biosphere may show increased net CO2 sequestration in regions where climate change increases primary productivity (Song et al., 2018). Not only long-term changes, but also pronounced interannual variability such as that induced by El Niño Southern Oscillation events (Betts et al., 2016; Zhao et al., 2020) could confound attribution of natural and anthropogenic sources and sinks of GHGs if not considered. Ultimately, reconciling bottom-up and top-down natural and anthropogenic sources and sinks within the GST has to be supported by estimates from inverse modelling systems in which different information streams (EO, in situ observations, and bottom-up inventories) are combined (e.g., BEIS (Department for Business, Energy & Industrial Strategy), 2021). Currently, there are major discrepancies between the two approaches: the reported LULUCF sink by all countries is of order 0.3 GtC yr−1, whereas the top-down estimates yield 2.5 GtC yr−1 (Grassi et al., 2021; Deng et al., 2022).
A particular aspect of the above challenge, which is of great relevance to the Paris Agreement, is the quantification of uptake of CO2 by forest activity. Reforestation and afforestation projects for carbon offsetting only provide a carbon sink if the planted trees establish themselves and grow effectively. For example, increased frequency of fires (deliberate, i.e., associated with land-use methods, or accidental) can counteract carbon savings from reducing emissions from deforestation and degradation (REDD, for example within the REDD+ process) (Aragão and Shimabukuro, 2010). While reporting should rely on actual forestry data, including tree counts and land cover extent, the growing number of such projects means there is a strong need to monitor the effectiveness of the carbon sequestration in these forests at an appropriate scale using EO. It is important to note that in the GHG inventories, emissions and removals for the national territory are reported. If a country wants to offset its own emissions by mitigation in a different country, the transfer is expected to be reported in the internationally transferred mitigation outcomes (ITMOs) of both countries’ BTRs in order to avoid double-counting of reforestation projects.
However, perhaps the greatest challenge in view of the Paris Agreement’s needs is that decision-relevant metrics used in the NDCs and NAPs normally do not map directly onto physically measurable quantities from EO. In other words, what we need to know is not always directly measurable by EO, and indirect indicators of progress are prone to confounding factors. To help address all these challenges, unambiguous attribution of the observed changes is needed. A cause-effect network could support such attribution, since it reflects system dependencies and can account for uncertainties in different drivers, which can then be propagated through the system (Figure 4). This cause-effect network highlights that the effectiveness of mitigation efforts can only be assessed if the full process from emissions to temperature change (the Paris Agreement’s main target) is known. On the other hand, climate adaptation and loss and damage can only be assessed if the full climate system response to GHG forcing is known. Climate sensitivity (a highly uncertain and changing characteristic of the Earth system; Sherwood et al., 2020) and land and ocean sinks need to be included in both considerations. EO (ideally anchored by in situ observations to ground truth) can deliver crucial information on many geophysical variables needed to inform and feed into such cause-effect networks, as exemplified in step 4.
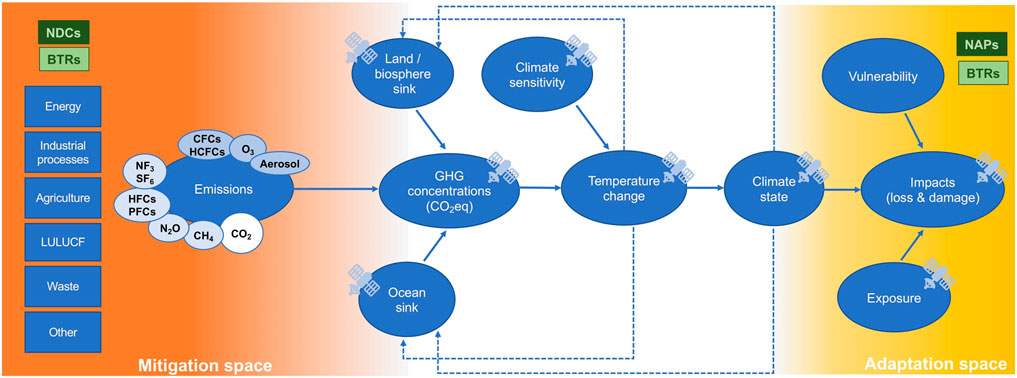
FIGURE 4. Cause-effect network depicting different pathways and Earth system feedbacks that need to be considered when trying to quantify how GHG emissions from different sectors (mitigation space) determine the climate state and thus climate impacts (adaptation space). Ozone and aerosol are generated from primary emissions of a range of shorter-lived trace gases that, along with CFCs and HCFCs (which are controlled under the Montreal Protocol), are not included under the Paris Agreement. However, they affect the Earth’s radiative forcing and thus need to be known to correctly attribute temperature changes. Both mitigation and adaptation spaces have blurred boundaries in the context of the Earth system because of options to alter the natural environment to deal with climate change (e.g., reforestation, ocean fertilisation, urban greening). The satellite icons indicate where EO offers useful information and constraints on the Earth system and its processes.
Step 4—Uncovering the potential of EO to support the UNFCCC Paris Agreement
In the following, the potential of EO to support the objectives of the Paris Agreement in the context of the climate system is discussed and illustrated by a set of four case studies. These case studies, illustrated in Figures 5–8, just provide examples; Table 1 offers an extended (but not exhaustive) list of potential applications of different sensors and their observables, including key characteristics. It is important to emphasize that deriving information on progress towards the Paris Agreement’s objectives from EO can only follow from sound knowledge of the climate state and its long-term changes that is built on consistent and systematic long-term observations from all elements of the observing system, including space-based EO (see Popp et al., 2020). This prerequisite is discussed, along with the list of the Paris Agreement’s objectives that EO can support (mitigation, sources and sinks, adaptation, loss and damage). The objectives are rephrased as questions, which help to elucidate their practical relevance.
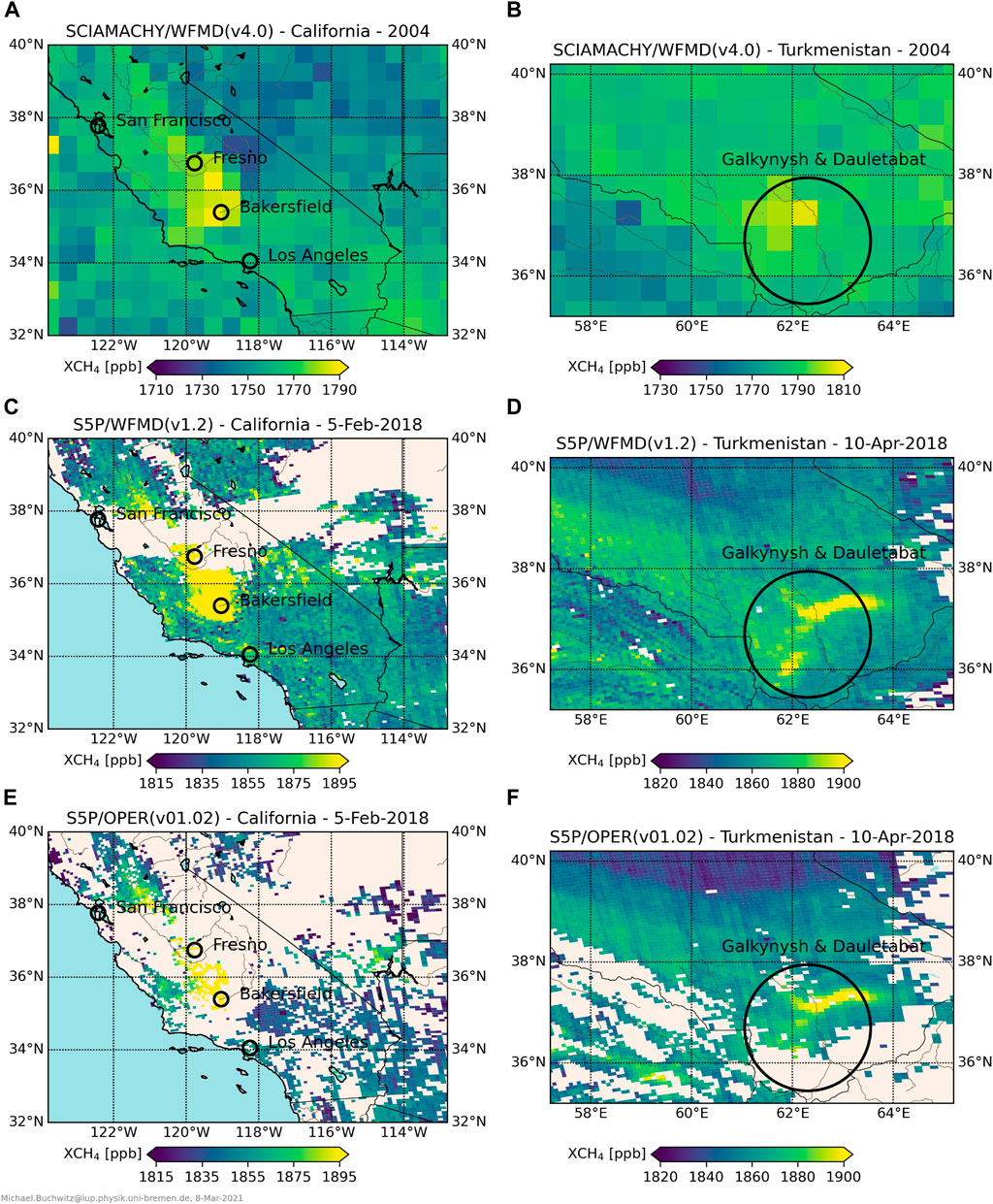
FIGURE 5. Satellite retrievals of column-averaged atmospheric methane (XCH4) over major methane source regions. (A) SCIAMACHY XCH4 retrievals at 0.5° × 0.5° resolution during 2004 over California (Buchwitz et al., 2017). (B) As (A) but for the Galkynysh and Dauletabad gas and oil fields located in south-east Turkmenistan. (C) Single overpass (5-Feb-2018) Sentinel-5P (S5P) XCH4 retrieved with a research algorithm over California (Schneising et al., 2019; Schneising et al., 2020). (E) As (C) but for the operational S5P XCH4 data product (Hu et al., 2018) (D,F) Same as in (C,E) but for a single overpass (10-Apr-2018) over Turkmenistan. The satellite observations yield information on hotspots of methane emissions, which can be used by local policy-makers and stakeholders to identify targeted mitigation strategies.
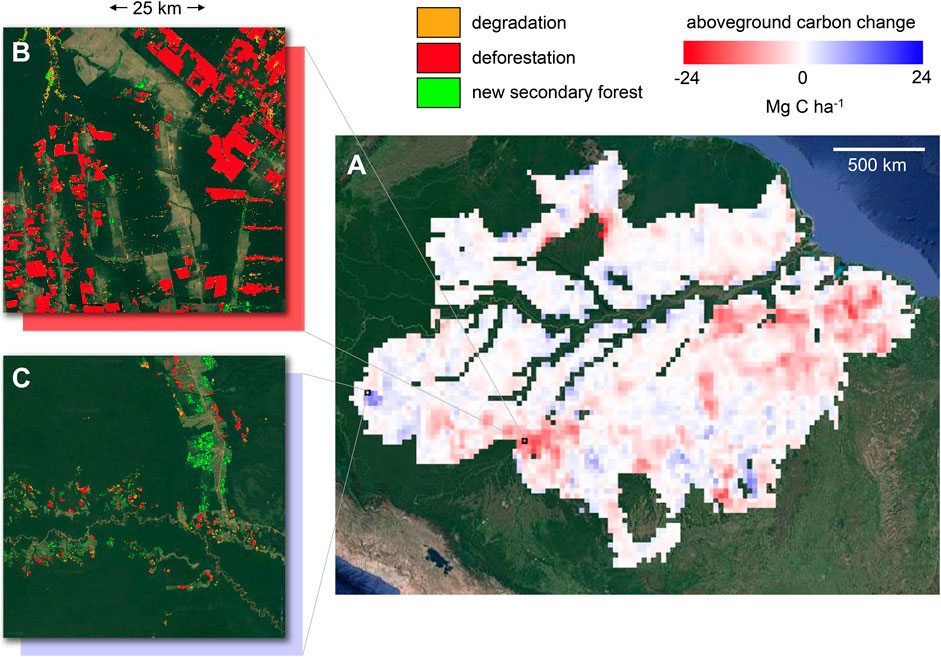
FIGURE 6. Remotely sensed carbon dynamics in the Brazilian Amazon and illustration of associated land-cover changes. (A) Net above-ground biomass carbon (AGC) difference map of the Brazilian Amazon between years 2014 and 2017. The AGC is derived using Vegetation Optical Depth in the L-band (L-VOD) from SMOS passive microwave observations (Wigneron et al., 2021), calibrated with the ESA Climate Change Initiative 2017 biomass map (Santoro and Cartus, 2021). High-resolution (30 m) mapping (B) of carbon loss pixel with large, deforested area, and (C) of carbon gain pixel with large intact forest fraction and forest regrowth. The observations help monitor and attribute gross changes in carbon stored in the above-ground land biosphere to human and natural drivers, thus contributing to the assessment of the integrity of carbon sinks and reservoirs. Importantly, systematic use of this approach could support verification of the efficacy of REDD and carbon offsetting schemes.
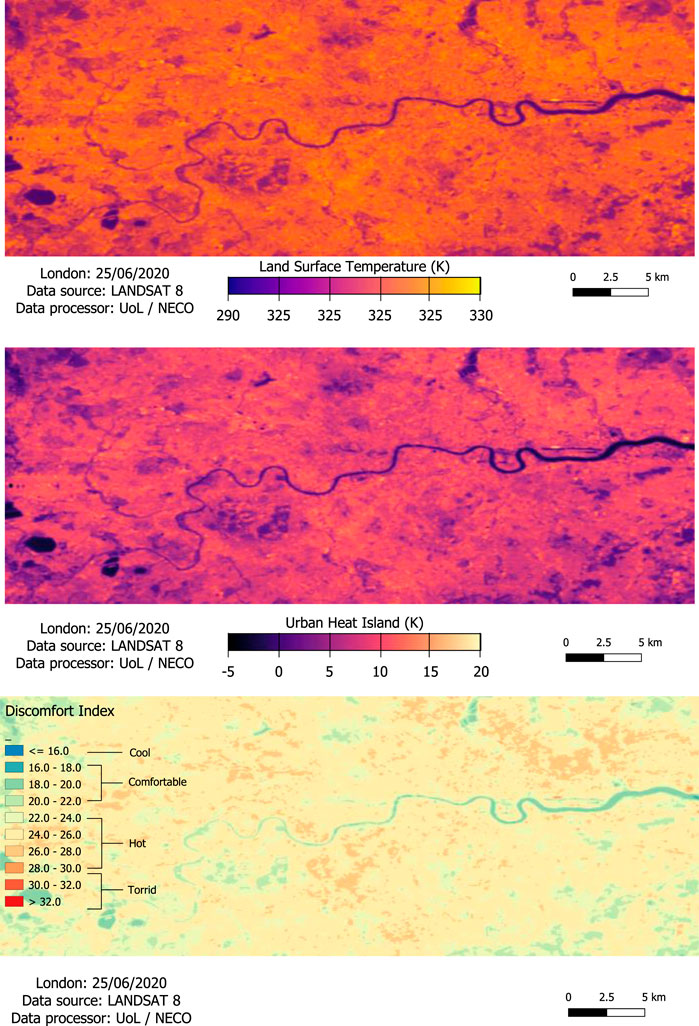
FIGURE 7. High-resolution land surface temperature from Landsat 8 (top) and resulting urban heat island (UHI) effect (middle). The surface temperature is combined with relative humidity from a meteorological reanalysis to derive the thermal discomfort index (DI) (bottom) which provides a measure of the temperature perceived by humans. The geophysical variable (temperature from infrared observations) is thereby translated into an indicator of its impact on the human body. Progress with adaptation in urban spaces could, for example, be measured by the degree of urban greening and the resulting across-city changes in the DI during a future heat wave.
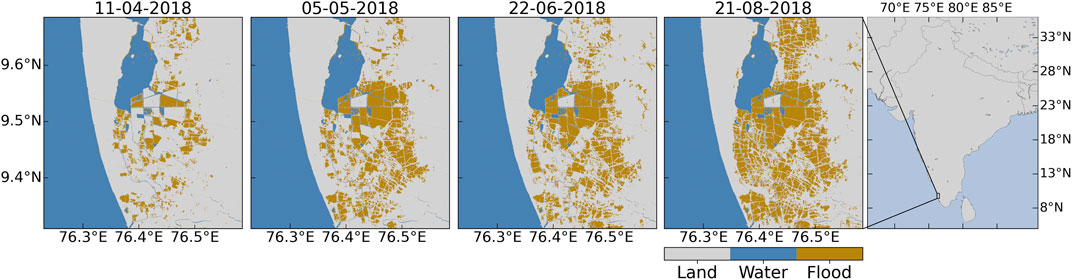
FIGURE 8. The surface images of Vembanad lake, Kerala, India, before and after the incidence of floods were retrieved from Sentinel-1A and -1B synthetic-aperture radar (SAR) images, available from the Copernicus Open Access Hub (https://scihub.copernicus.eu; 2018–2020, data accessed on 22 April 2021). Level-1 Ground Range Detected (GRD) SAR products were processed at 10 m spatial resolution using the Sentinel Application Platform (SNAP) from the European Space Agency (ESA). Blue indicates water bodies (ocean/lake), brown areas indicate flooding. The information on the extent of the flooding could be used in combination with socio-economic data to derive estimates of economic loss across the flooded area.
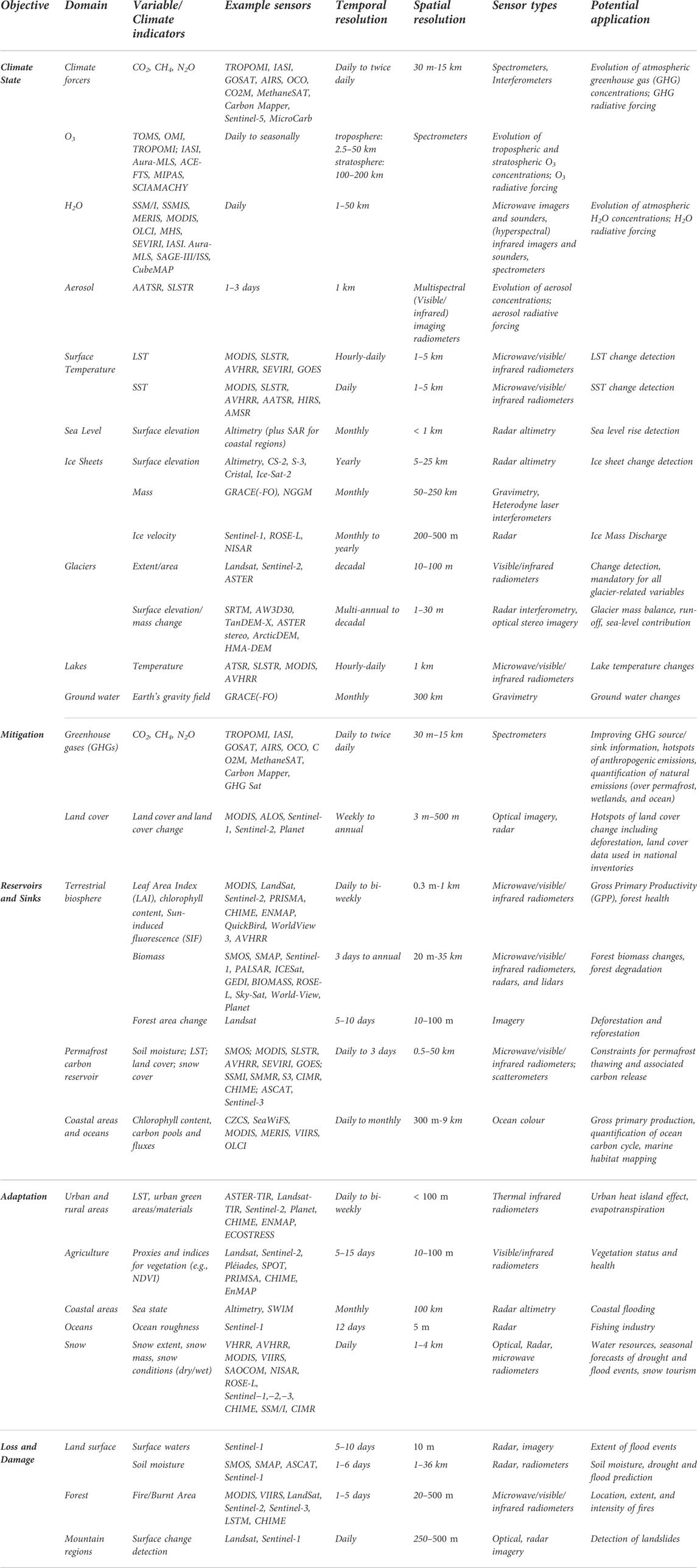
TABLE 1. Example list of Essential Climate Variables from various types of current and future sensors and their key characteristics, along with suggested applications for each of the Paris Agreement’s objectives.
EO to monitor the climate state—Is the Earth system responding to climate change as predicted?
The temperature goal of staying well below 2°C (or 1.5°C) above pre-industrial levels, as enshrined in Article 2.1 of the Paris Agreement, was based on the scientific judgment that beyond this threshold the risk of catastrophic consequences (e.g., massive thawing of permafrost, irreversible melting of ice sheets, or the collapse of tropical rainforests) increases substantially (IPCC, 2018). However, three major uncertainties are associated with this threshold. First, how can we determine when we have reached the threshold? Natural variability in the climate system may confound the attribution of climate change to rising GHG concentrations (e.g., Bonan et al., 2021), so observations will have to be interpreted with the help of models, a research endeavour that still needs further development. Second, what is the total amount of GHGs that can be emitted before this threshold is reached? As already outlined above, the answer to this question depends both on climate sensitivity (including cloud feedbacks) as well as on Earth system feedbacks such as the response of the biosphere/ocean carbon-uptake to climate change (see Figure 4), all of which remain poorly known and are not well constrained by current climate models. Third, what are the regional manifestations of such a 2°C global mean temperature increase? Climate change expresses itself in different ways in different regions of the world, with high altitude and high latitude climates predicted to experience much higher temperature change than the global mean (IPCC, 2021). These changes may induce local tipping points (e.g., in monsoon rainfall or alpine ecosystems) whose underlying mechanisms are poorly known or unresolved in current climate models (Lenton et al., 2008). Answering these questions is crucial for deciding whether the Paris Agreement’s temperature target needs to be revised, and what kind of adaptation measures that help build climate resilience to minimize loss and damage need to be put in place.
Essential climate variables and their derived climate data records (many of which are derived from EO) are widely used in the form of global climate indicators to provide information on the climate state and its changes. They contribute to the “big seven” indicators: global mean surface temperature, ocean heat content, atmospheric CO2, sea level, ocean acidification, glacier mass, and sea ice extent (e.g., GCOS, 2020; Trewin et al., 2021; WMO, 2022). However, these global indicators do not capture the full complexity of the Earth system needed for attribution of observed changes, including climate feedbacks (e.g., involving clouds, aerosols, and the biosphere). Promising applications of how EO (along with other observations) can be used to provide constraints on the sensitivity of Earth system processes to increases in GHGs (and thus contribute to constraining climate sensitivity) have been presented in Sherwood et al. (2020) and references therein. However, many of the required variables for attribution ultimately can only be inferred from combining modelling and EO in data assimilation systems, and the signal-to-noise ratio of attributed changes generally becomes smaller at finer spatial or temporal scales. Thus, to become more useful to climate assessments (e.g., Blunden and Boyer, 2021; IPCC, 2021) and the UNFCCC process, EO research needs a new focus on delivering climate data records from global down to local scales, with higher spatial and temporal resolution, and improved timeliness of data provision. A clear attribution of the observed changes in any case remains a great challenge, as expressed in the new lighthouse activity Explaining and Predicting Earth System Change of the World Climate Research Programme (WCRP).
EO to assess mitigation—Are our mitigation efforts effective?
To achieve the Paris Agreement’s long-term temperature goal, the Parties have accepted the obligation to define and communicate ambitious efforts to curb GHG emissions in the form of NDCs (Article 3). The collective goal (Article 4.1) thereby is to reach global peaking of GHG emissions as soon as possible, and to rapidly reduce emissions thereafter to achieve a balance between GHG sources (emissions) and sinks (removal, e.g., through land-based mitigation, see next subsection) in the second half of this century. Measuring atmospheric GHG concentrations, and the quantification and attribution to anthropogenic sources and sinks (which, in principle, also requires knowledge of natural sources and sinks), will be a key task for national governments, the private sector, and the global research community over the coming decades to effectively contribute to the ETF and GST at national and global levels (Janssens-Maenhout et al., 2020; Palmer et al., 2021). The private sector will play a key role in providing EO and other services needed by governments to help them fulfil legal requirements around mitigation reporting. These tasks follow the latest refined guidelines by the IPCC (IPCC, 2019) by reporting of emissions by sector (bottom-up estimates from inventories) with the option to provide estimates also from inverse modelling of emissions (top-down estimates), which currently are based primarily on in situ observation networks. However, bottom-up and top-down estimates can exhibit significant differences in emission estimates (e.g., Saunois et al., 2020) and require further efforts to be reconciled.
The potential for supporting the Paris Agreement in its mitigation objective is arguably the best-developed EO capacity. Evolving partnerships include government, university, and industry partners exemplifying the triple-helix model (Etzkowitz, 2003) which is needed to solve complex problems, but which may also have to engage with stakeholders beyond these sectors. A key focus hitherto has been the use of satellite observations of GHG concentrations (primarily CO2 and CH4) for detecting emission hotspots to identify targeted mitigation opportunities (Kort et al., 2014; Lauvaux et al., 2022; see also Figure 5), or for assimilation into inverse modelling systems to quantify surface fluxes from natural and anthropogenic sources and sinks on country to continental scales (Alexe et al., 2015; Chevallier et al., 2022; Kaminski et al., 2022). Post-processing of such inversions that remove inferred natural sinks and sources is needed to make them comparable to the UNFCCC emission inventories (Deng et al., 2022). This will help to identify whether NDC pledges are kept and mitigation mechanisms (e.g., carbon trading) work on a globally aggregated level. However, while these measurements in principle provide a global view, they still have limited temporal and spatial coverage, and (although improving) also lack the accuracy and precision needed to quantify emission sources satisfactorily. Indeed, the current OCO2 and OCO3 satellite capabilities for observing fossil CO2 emissions using atmospheric concentration signals is limited to some cities and large point sources (Zheng et al., 2020), although the future Copernicus CO2 Monitoring Mission (CO2M) will allow a better constraint on these emissions (Wang et al., 2020). Similar improvements will be obtained by new satellite missions measuring CH4 (MethaneSAT, Sentinel 5, and Carbon Mapper).
A few countries thus rely mostly on ground-based in situ measurement networks (which importantly also yield information on many gases not observed from space or in persistently cloudy regions) to verify their GHG emissions and deduce emission factors to verify and/or improve bottom-up estimates. However, for national agencies in other countries where in situ observations are often sparse or lacking, and/or bottom-up estimates have large uncertainties, satellites offer a major opportunity to constrain and/or verify their national emissions. Ground-based measurement networks can be used to bias-correct the satellite observations (addressing potential geographical dependencies in satellite biases). In principle, such initiatives for ground-based measurement networks in support of EO could be considered as a contribution to the Paris Agreement’s objective on technological support and transfer (Article 10, see Figure 2), which is however not discussed in this contribution in more detail. Also, the global extent of EO will help to constrain natural emission sources in remote or hard-to-access regions (e.g., permafrost, wetlands, oceans).
Another aspect of mitigation not explicitly mentioned in the Paris Agreement’s main Articles (see Figure 2), but which is of crucial importance to achieving net-zero and can be supported by the use of EO (e.g., ocean state, winds, clouds, aerosol, radiation, glaciers, snow, and biomass), is the planning, development, and optimisation of renewable energy systems based on solar, biofuel, hydro, wind, wave, or tidal energy (Solaun and Cerdá, 2019). Detailed knowledge on climate change and its impacts is therefore needed on a regional to local scale (with particular focus perhaps on transitional zones such as near-shore regions), and also on timescales from short-term, sub-seasonal and seasonal, to multi-decadal, since these will greatly affect the reliability and performance of renewable energy systems (Arent et al., 2015; Cronin et al., 2018). In principle, such EO-based information could also be considered as a contribution to the Paris Agreement’s objective on technological support (Article 10, see Figure 2), which is however not discussed here.
EO to quantify sinks and reservoirs—Are we conserving and enhancing GHG sinks and reservoirs?
Conserving and enhancing sinks and reservoirs of carbon is the second objective discussed in Article 5 of the Paris Agreement to achieve its mitigation goals (see previous section), with major research so far focussing on agriculture, forestry, and other land use change (AFOLU) (Bustamante et al., 2014). Significant potential for mitigation exists from REDD (Popp et al., 2014). In particular, sustainable practices in forestry and land use offer opportunities to reduce GHG emissions. Key tasks in monitoring these sinks and reservoirs include obtaining a global assessment of the temporal change in sink and reservoir sizes and attributing this change to both natural (e.g., wildfires, drought, diseases) and anthropogenic drivers (e.g., logging, agriculture, and urban expansion). Another important aspect is the quality and functionality of these sinks (e.g., in terms of their gross primary productivity). EO data already provide useful information for both global and national monitoring of forest/land use carbon sources and sinks (Harris et al., 2016, 2021; Nesha et al., 2021).
A case study highlighting the potential of EO to help quantify AFOLU changes is shown in Figure 6. Here, remotely sensed land-cover changes in the Brazilian Amazon based on imagery from the Landsat satellites and associated above-ground carbon change (AGC) based on microwave observations from the Soil Moisture and Ocean Salinity (SMOS) satellite, reveal the carbon dynamics of the region. Across the Amazon basin, major areas show a loss of AGC storage due to deforestation and forest degradation.
While not specifically pointed out in the Paris Agreement, but as highlighted above, ocean and land sinks and reservoirs will also be affected by climate feedbacks. In particular, climate-induced thawing of permafrost has the potential to release a large amount of methane (Schuur et al., 2008), while the duration of snow cover and snow melt, both affected by climate change, is important for the annual CO2 balance in the Arctic and Sub-arctic region (Aurela et al., 2004). Climate feedbacks also manifest themselves through changes in forest disturbance regimes, and satellite biophysical indices show that just a few extreme events were responsible for most of the year-to-year variability of photosynthesis (Zscheischler et al., 2014). Thus, any measurements to constrain emissions from changes in these reservoirs either directly (e.g., using GHG observations) or indirectly (e.g., through the measurement of permafrost or wetland area-related ecological variables such as air and surface temperatures, inundated area, snow mass, and soil moisture), will be of great value in assessing the overall progress of the Paris Agreement.
The value-added products based on EO that yield quantitative information on the temporal changes in sinks and reservoirs of GHGs will help to validate national pledges and policy implementation (including carbon offsetting). A suite of satellite-derived biophysical products (including photosynthetically active radiation, leaf area index, GPP, various empirical vegetation indices, and land cover) can provide qualitative and quantitative information on changes in the characteristics of sinks and reservoirs (including ocean, coastal and tidal ecosystems, forest disturbances, wetlands and permafrost) that may affect their capacity to absorb GHGs in the future (Running et al., 1994; Running, 2012). These EO data in conjunction with ground-based in situ and airborne remote observations will need to be interpreted with the help of modelling to attribute observed year-to-year variations in natural sinks and sources to their drivers (e.g., El Niño events) both over land (Woodward et al., 2008; Bastos et al., 2018) and ocean (Ilyina et al., 2021).
EO to inform adaptation—Are our adaptation efforts adequate?
It is unavoidable that the world will have to adapt to climate change (UNEP, 2021), with the Paris Agreement Article 7 prescribing its Parties to enhance adaptive capacity, strengthen resilience, and reduce vulnerability to climate change in the context of the temperature goal. However, unlike mitigation, with its 2°C temperature goal (which can use carbon, ocean heat, and energy budgets as simple measures), adaptation has no clearly defined global target or reporting guidelines, and common indicators are lacking due to adaptation being dependent on location-specific economic, social, and environmental conditions (Christiansen et al., 2020). Moreover, it is arguably impossible to measure progress, adequacy, and effectiveness of enhancing resilience and reducing vulnerability of societies and individuals as foreseen in the Paris Agreement since these aspects are by definition counter-factual, i.e., they are a difference between what did happen and what would have happened without the adaptation measures. Only the former is measurable, while the latter would have to be inferred (e.g., from comparison with observations of nearby locations where adaptation measures were absent; see Figure 7 for an example of spatial structure in EO observations that could be exploited for this purpose). Modelling systems with and without adaptation measures could be developed to quantify differences between the real world and the world that would have been without a given adaptation measure, with the construction of such counter-factuals offering the opportunity to explore inherent uncertainties. This approach is widely used in the context of Earth system modelling, e.g., to quantify the impact of ozone-depleting substances (ODSs) on stratospheric ozone loss by using model simulations with and without ODSs (e.g., Shepherd et al., 2014). However, a prerequisite of such studies is that the system in question can be modelled with a high degree of realism (i.e., it must be validated against observations).
While there is ample information from EO on adaptation-relevant geophysical variables, there is a need to co-develop EO-based adaptation indicators relevant for key sectors such as energy, water, coastal resources, human settlements and urban planning, real-estate, transport, communication, tourism, agriculture, forestry, and ecosystem management, in collaboration with national agencies (responsible for producing the NAPs) and other stakeholders (see e.g., Ffoulkes et al., 2021). These indicators should consider different aspects of climate-related hazards, vulnerability, and exposure, and be meaningful under local conditions and resulting needs. Outstanding issues might include missing knowledge on local climate extremes and their resulting impacts, which require spatially disaggregated information and can lead to a trade-off between reliability (usually achieved through temporal and/or spatial aggregation of data) and informativeness (particularly for events happening on small spatial scales or over short time periods); missing knowledge on the rate of the changes observed in the climate system (as opposed to state variables), provided in a timely manner and also providing a historical baseline; spatial and temporal gaps in available EO (e.g., not covering the Arctic, coastal regions, seasonality, or diurnal cycles).
Examples of geophysical variables from EO that can be used to derive observation-based indicators include: high-resolution land-surface temperature and canopy cover to track impact and adaptation to the urban heat island effect (e.g., Goldblatt et al., 2021; also Figure 7); groundwater (e.g., Tapley et al., 2019) and lake levels (e.g., Woolway et al., 2020), as well as glacier mass (e.g., Hugonnet et al., 2021) to track anthropogenic water use (Massari et al., 2021) and thus vulnerability and adaptation to changing water resources (Lawford et al., 2013); biomass, soil moisture, and land use to track exposure to flooding, droughts, and fires (e.g., Kumar et al., 2022); high-resolution land cover and land use to track vulnerability to soil erosion and adaptation by changes in agricultural practices (e.g., Tadesse et al., 2017); or sea-level rise and sea state, including flood defences, to track exposure and adaptation to coastal erosion and flooding (Nicholls and Cazenave, 2010).
EO to assess loss and damage—Are we sufficiently protected against economic and non-economic loss and damage?
The final objective discussed here, to minimise loss and damage, relates to Article 8 of the Paris Agreement and is directly connected to adaptation in that it is experienced when adaptation measures fail or are not implemented. Loss and damage can thereby occur due to both sudden-onset (e.g., cyclones, flooding, heat waves, fires) or slow-onset (e.g., sea-level rise, glacier melting, drought) events, and it can be both economic (e.g., assets, resources, goods or services that are traded in markets) and non-economic (including e.g., livelihoods, human health, territory, culture, biodiversity, which cannot be traded) (IPCC, 2013).
EO has the potential to offer evidence-based information for risk mapping, building early warning systems, and conducting impact assessments (e.g., Nakalembe et al., 2021). EO thereby provides a view on loss and damage where such events are not directly experienced and assessed by humans (e.g., ecosystem changes due to storm surges, Pisaric et al., 2011) or helps to assess the scale and extent of events that affect humans more immediately (e.g., storms, flooding, fires, droughts). As an example, Sentinel-1 imagery of the large-scale Kerala flooding event in 2018 (see Figure 8; also Vishnu et al., 2019) can be used in combination with socio-economic information to derive each event’s extent and impact. EO imagery can also be used to derive the extent of thawing permafrost soils which, through destabilisation and subsidence, can lead to damage to road and building infrastructures, land use, water resources, and even loss of livelihoods (Hjort et al., 2022). EO information on climate-induced changes in snow cover (e.g., Matiu et al., 2021) can help to assess economic losses, e.g., to the winter tourism sector (Scott et al., 2008) or agriculture in areas affected by changing water resources from melting glaciers (Biemans et al., 2019).
A key issue here, as for adaptation, is the co-development of indicators that help with a meaningful assessment of the progress in reducing the vulnerability and strengthening resilience of societies to loss and damage, involving national agencies and stakeholders. A way forward to realise the potential of EO to inform the NAPs and BTRs on climate-change related loss and damage, is to leverage the progress made in the use of EO for disaster risk reduction within the sustainable development framework, which aims at producing risk indicators and actionable information for decision-makers in that context (Lorenzo-Alonso et al., 2019). A promising way forward could involve a key user of such information (the insurance and reinsurance sector), which relies on evidence-based (often EO) information for the development of parametric insurance products, that is for decisions on how to spread economic risk and increase financial stability for their customers (e.g., in agriculture or real estate). These products could offer a market-driven tool and mechanism to assess progress in minimising loss and damage within the Paris Agreement framework (Erhardt et al., 2019).
Synthesis
Realising the full potential of EO in support of the Paris Agreement is a task of paramount importance. The global EO research community needs to tackle immediately the challenge of improving the policy-relevance of their research to contribute to the worldwide fight against climate change. If done correctly—and this requires imaginative bridging of the theory and practice divide we have discussed here—EO could become a central tool in driving the Paris Agreement’s ambition cycle. Lessons can be learned from similarly complex applications that are underway, for example to use EO in support of the sustainable development goals (Anderson et al., 2017), disaster risk reduction activities (Lorenzo-Alonso et al., 2019), or other operations (such as fisheries and agriculture, e.g., Whitcraft et al., 2019) that benefit from geospatial information.
Climate change is a complex systems phenomenon, which involves multi-scale processes and feedbacks (Holling 2004). Importantly, understanding the full manifestation of climate change involves considering both global and local scales (Berkes 2007). While these have traditionally been analysed largely separately, EO can provide information that bridges these perspectives, with many high-resolution data products of geophysical variables with global coverage becoming available. In seeking how this might be done, it is instructive to reflect on the key principles articulated by Cash et al. (2003) for knowledge systems for sustainable development: credibility, salience, and legitimacy. The EO community has tended to focus on credibility, including the ambition of developing ‘fiducial’ data and well-characterised uncertainties (Merchant et al., 2017). But salience at the local scale requires disaggregation in space and time, as well as timeliness, which can be challenging in this respect (since averaging observations reduces measurement noise and sampling errors, e.g., Hegglin et al., 2021). And legitimacy involves trustworthiness, which is highly contextual as it depends on the decision-maker, and thus inevitably brings in values. In this respect, transparency and interoperability may be more relevant concepts than the traditional scientific goal of objectivity. By transparency (or auditability) we mean that it is clear how results were obtained, and that one has the freedom to change some of the subjective methodological choices to see how the results might be different with different expert judgement. This is very much consistent with the move towards trans-disciplinarity (Hadorn et al., 2008) and “open science” (Munafò et al., 2017). In practice, these three principles will often be in tension with each other and the appropriate balance between them will need to be struck.
Thus, in order to bridge between global and local, and between different forms of observational knowledge (e.g., traditional EO and indigenous knowledge, Berkes, 2002, 2007), a paradigm shift is needed from the traditional inductive approach to a wider perspective where theory is used deductively. In the inductive approach (Figure 9A), which places a premium on credibility, one moves from the specific to the general to create scientific knowledge that supports policy. In the deductive approach, one moves from the general to the specific to interpret observations in order to achieve salience (Figure 9B; see also Shepherd 2021). Although this approach introduces an element of subjectivity in the form of expert judgement, the subjectivity is transparent, and the approach can help reconcile the Paris Agreement’s ETF (which is to be provided at a local/national level) and the GST (which is to be provided at the integrated, global level).
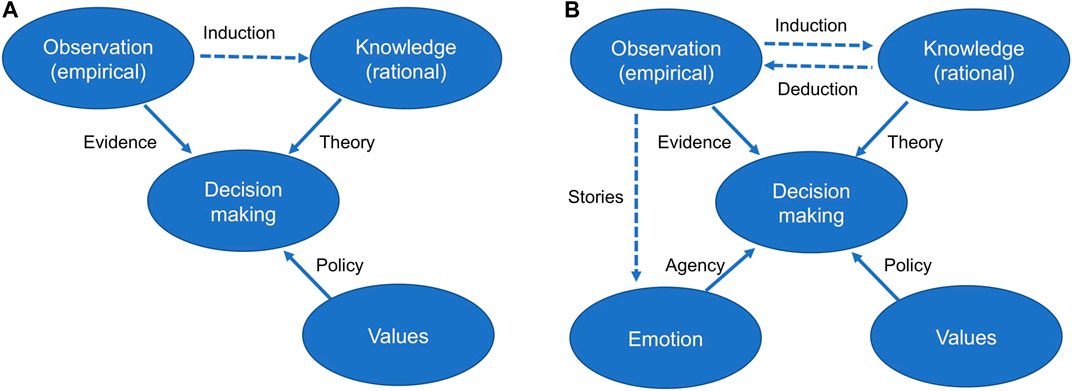
FIGURE 9. (A): The traditional paradigm of science for decision making. (B): The new paradigm proposed here.
Seen from this perspective, EO has the potential not only to measure and help manage our impact on the Earth, as the case studies above have illustrated, but also to make climate change salient and to stimulate emotions, which are needed for decision-making and ultimately for action (Damasio, 2006; Figure 9B). In short, EO allows us (to quote Schumacher again, p201) to “stop, look, and listen” rather than “look it up in the forecast”. Refocussing our attention on the past and present based on EO may be one way forward to help inform the pressing mitigation and adaptation decisions which have to deal with short-term risks and climate variability, and which current approaches focussing on end-of-century projections are unable to tackle in a satisfactory way (Nissan et al., 2019). The challenge is the creation of poignant visualisations and meaningful measures (metrics and indicators)—effectively, stories (Lloyd and Shepherd, 2021)—which are salient to policy makers, economic stakeholders, and society in general, yet which are anchored in knowledge and have representativity beyond the anecdotal. This is ultimately needed for stories to achieve legitimacy, traceability, and transparency. Outreach and education, essential means identified in the Paris Agreement’s Article 13, can very much use such stories and be greatly supported by EO.
Figure 10 provides a well-known example of such a story, namely the decreasing extent of Arctic sea-ice in September (the seasonal minimum), to illustrate the impact of climate change on polar regions. Such figures can be used analogously to the highly evocative images of the Antarctic ozone hole created by NASA soon after its discovery, which were a driving force in the global recognition of the destructive potential of chlorofluorocarbons (CFCs) that led to the 1987 Montreal Protocol and its Amendments, a treaty phasing out the production of ozone-depleting substances (Salawitch et al., 2018). Visualisation of EO promises the same potential when it comes to explaining the consequences of climate change in the form of the increased land and sea surface temperatures, melting sea ice, ice sheets and glaciers, rising sea-levels, and increased fire and flooding due to rising GHG concentrations.
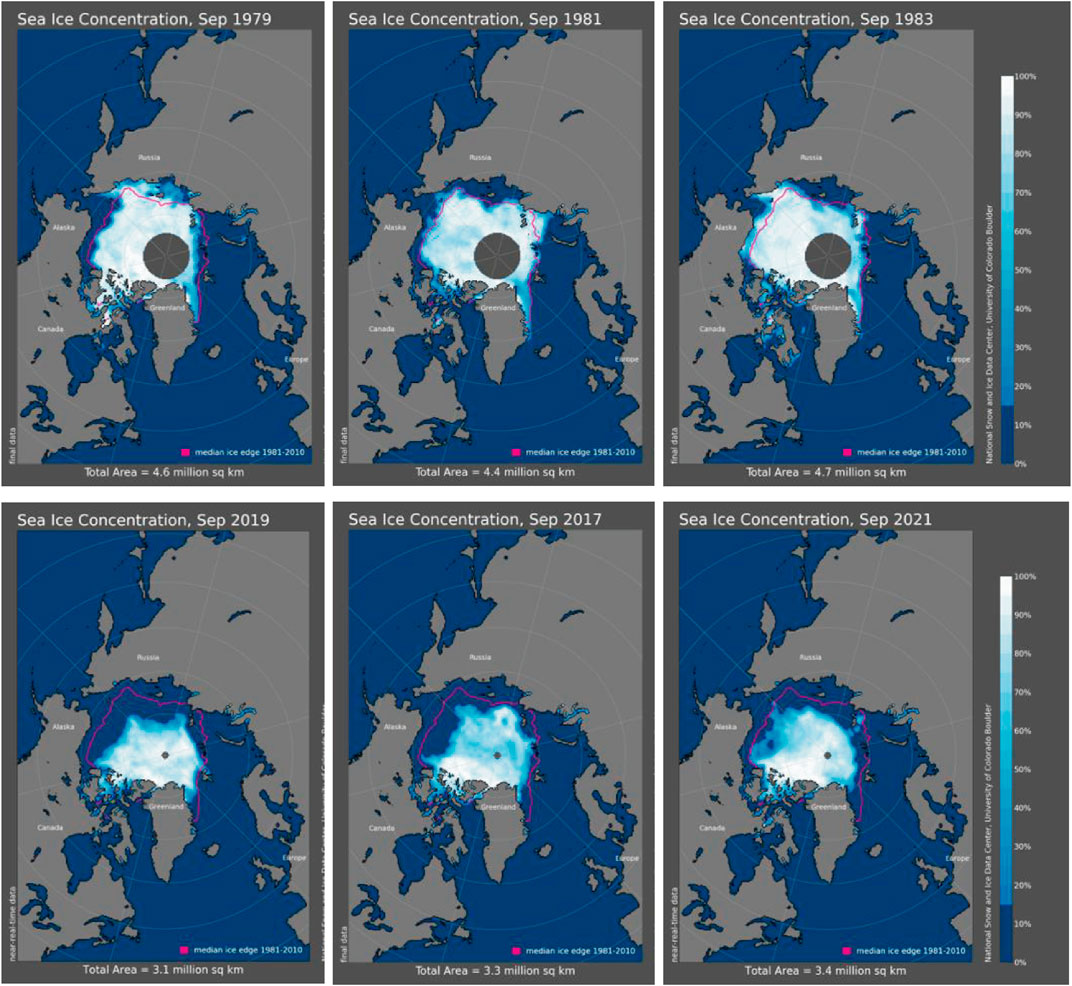
FIGURE 10. Arctic sea ice concentrations in September for 1979, 1981, and 1983 (upper row) compared to the years 2017, 2019, and 2021 (lower row). The pink line demarks the median sea ice edge over the time period 1981–2010. The total sea ice area has decreased by around 25% between these two time periods. Images are obtained from the National Snow and Ice Data Center (Fetterer et al., 2017).
Most importantly, EO can help us appreciate the full consequences of climate change and its current manifestations and ultimately keep us grounded in reality. Building cause-effect networks (Figure 4), informed by observational evidence and socio-economic data, could be a powerful approach to create a GST framework that allows us to account for the full range of known uncertainties and test overall consistency, and thus measure true progress from our actions. It would also help us to identify early warning signs where climate mitigation and adaptation measures have failed or need strengthening to achieve a desired goal, or where model predictions may have gone wrong due to the lack of realism in model components and parameterisations of subgrid-scale Earth system processes.
Importantly, the delivery and translation of EO into information that is actionable for local policymakers and practitioners has to be an iterative process (Vincent et al., 2018). Early dialogue with policymakers and other stakeholders in the lifecycle of indicator development is therefore critical in identifying end-user needs and defining requirements for how to build indicators that are just and equitable (as required by the Paris Agreement) and trace progress for adaptation and mitigation efforts, while involvement of the climate research community and the private sector is key in driving innovation in providing EO-based climate information and knowledge (e.g., including through AI). Introducing a feedback loop that allows co-development of climate information, as opposed to the traditional linear process, in which EO products were passed from the producer down the line to the end-user, will be crucial to realising the full potential of EO (Figure 11). This may have to be supported by a more formalised requirements management process, which collects and validates policy needs regularly, and also includes the evaluation of the actual benefits to operational organisations. Such research work can then feed into climate services, with the ultimate aim of providing salient products to policymakers in an operational and timely manner. Combining forces in this way will enhance the transdisciplinary knowledge needed to put the Paris Agreement into action.
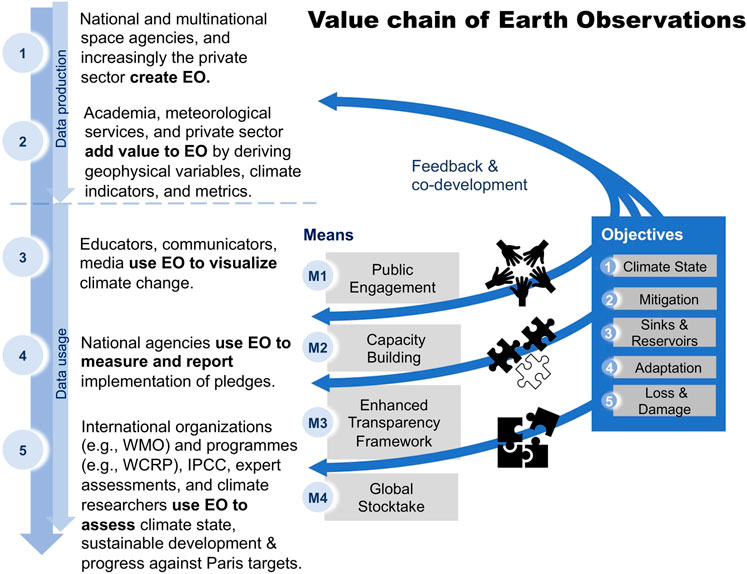
FIGURE 11. To increase the impact and societal benefit of EO, a feedback loop that helps co-development of salient EO products useful to policy-makers needs to be introduced in the value chain of EO, which starts with the creation of EO and ends with their use.
Author contributions
MIH conceived, carried out, designed schematics, and led the writing of the study. AB, HB, MB, DF, DG, GK, and SS provided case studies and associated figures. TGS helped with the conception and the writing, and provided Figure 9. All other authors provided input to extended discussions during a dedicated series of workshops to underpin the study and contributed to the editing.
Funding
This study “Earth Observation in support of the Paris Agreement (EO4Paris)” was funded by ESA (Contract No. 4000123554) via the Water_Vapour_cci project of ESA’s Climate Change Initiative (CCI).
Conflict of interest
The authors declare that the research was conducted in the absence of any commercial or financial relationships that could be construed as a potential conflict of interest.
Publisher’s note
All claims expressed in this article are solely those of the authors and do not necessarily represent those of their affiliated organizations, or those of the publisher, the editors and the reviewers. Any product that may be evaluated in this article, or claim that may be made by its manufacturer, is not guaranteed or endorsed by the publisher.
References
Ahamed, A., and Bolten, J. D. (2017). A MODIS-based automated flood monitoring system for southeast Asia. Int. J. Appl. Earth Observation Geoinformation 61, 104–117. doi:10.1016/j.jag.2017.05.006
Alexe, M., Bergamaschi, P., Segers, A., Detmers, R., Butz, A., Hasekamp, O., et al. (2015). Inverse modelling of CH<sub>4</sub> emissions for 2010–2011 using different satellite retrieval products from GOSAT and SCIAMACHY. Atmos. Chem. Phys. 15, 113–133. doi:10.5194/acp-15-113-2015
Anderson, K., Ryan, B., Sonntag, W., Kavvada, A., and Friedl, L. (2017). Earth observation in service of the 2030 agenda for sustainable development. Geo-spatial Inf. Sci. 20 (2), 77–96. doi:10.1080/10095020.2017.1333230
Aragão, L. E., and Shimabukuro, Y. E. (2010). The incidence of fire in Amazonian forests with implications for REDD. Science 328 (5983), 1275–1278. doi:10.1126/science.1186925
Arent, D. J., Tol, R. S., Faust, E., Hella, J. P., Kumar, S., Strzepek, K. M., et al. (2015). Key economic sectors and services. Climate change 2014 impacts, adaptation and vulnerability: Part a: Global and sectoral aspects, 659–708.
Aurela, M., Laurila, T., and Tuovinen, J.-P. (2004). The timing of snow melt controls the annual CO2 balance in a subarctic fen. Geophys. Res. Lett. 311 (16), L16119. doi:10.1029/2004GL020315
Bastos, A., Friedlingstein, P., Sitch, S., Chen, C., Mialon, A., Wigneron, J. P., et al. (2018). Impact of the 2015/2016 El Niño on the terrestrial carbon cycle constrained by bottom-up and top-down approaches. Phil. Trans. R. Soc. B 373 (1760), 20170304. doi:10.1098/rstb.2017.0304
BEIS (Department for Business, Energy & Industrial Strategy) (2021). Annual Report 2021: Verification of UK greenhouse gas emissions using atmospheric observations. available at https://www.gov.uk/government/publications/uk-greenhouse-gas-emissions-monitoring-and-verification (Accessed August 16, 2022).
Berkes, F. (2002). “Epilogue: Making sense of arctic environmental change?,” in The Earth is faster now: Indigenous observations of arctic environmental change. Arctic research consortium of the U.S. (ARCUS). Editors I. Krupnik, and D. Jolly (Fairbanks, AK, 335–349.
Berkes, F. (2007). Understanding uncertainty and reducing vulnerability: Lessons from resilience thinking. Nat. Hazards (Dordr). 41 (2), 283–295. doi:10.1007/s11069-006-9036-7
Betts, R. A., Jones, C. D., Knight, J. R., Keeling, R. F., and Kennedy, J. J. (2016). El Niño and a record CO2 rise. Nat. Clim. Chang. 6 (9), 806–810. doi:10.1038/nclimate3063
Biemans, H., Siderius, C., Lutz, A. F., Nepal, S., Ahmad, B., Hassan, T., et al. (2019). Importance of snow and glacier meltwater for agriculture on the Indo-Gangetic Plain. Nat. Sustain. 2 (7), 594–601. doi:10.1038/s41893-019-0305-3
Blunden, J., and Boyer, T. (Editors) (2021). “State of the climate in 2021,” 102. Si–S475. doi:10.1175/2021BAMSStateoftheClimate.1Bull. Amer. Meteor. Soc.8
Bonan, G. B., Lombardozzi, D. L., and Wieder, W. R. (2021). The signature of internal variability in the terrestrial carbon cycle. Environ. Res. Lett. 16 (3), 034022. doi:10.1088/1748-9326/abd6a9
Brandt, M., Wigneron, J. P., Chave, J., Tagesson, T., Penuelas, J., Ciais, P., et al. (2018). Satellite passive microwaves reveal recent climate-induced carbon losses in African drylands. Nat. Ecol. Evol. 2, 827–835. doi:10.1038/s41559-018-0530-6
Buchwitz, M., Schneising, O., Reuter, M., Heymann, J., Krautwurst, S., and Bovensmann, H. (2017). Satellite-derived methane hotspot emission estimates using a fast data-driven method. Amos. Chem. Phys. 17, 5751–5774. doi:10.5194/acp-17-5751-2017
Bustamante, M., Robledo‐Abad, C., Harper, R., Mbow, C., Ravindranat, N. H., Sperling, F., et al. (2014). Co‐benefits, trade‐offs, barriers and policies for greenhouse gas mitigation in the agriculture, forestry and other land use (AFOLU) sector. Glob. Chang. Biol. 20 (10), 3270–3290. doi:10.1111/gcb.12591
Canadell, J., Ciais, P., Sabine, C., and Joos, F. (Editors) (2012). REgional carbon cycle assessment and processes (RECCAP). Biogeosciences 9-11. http://www.biogeosciences.net/special_issue107.html.
Cash, D. W., Clark, W. C., Alcock, F., Dickson, N. M., Eckley, N., Guston, D. H., et al. (2003). Knowledge systems for sustainable development. Proc. Natl. Acad. Sci. U. S. A. 100 (14), 8086–8091. doi:10.1073/pnas.1231332100
Chevallier, F., Broquet, G., Zheng, B., Ciais, P., and Eldering, A. (2022). Large CO2 emitters as seen from satellite: Comparison to a gridded global emission inventory. Geophys. Res. Lett. 49, e2021GL097540. doi:10.1029/2021GL097540
Christiansen, L., Olhoff, A., and Dale, T. (2020). Understanding adaptation in the global Stocktake. UNEP DTU partnership. Part of the iGST designing a robust Stocktake discussion series.
Chuvieco, E., Mouillot, F., van der Werf, G. R., San Miguel, J., Tanasse, M., Koutsias, N., et al. (2019). Historical background and current developments for mapping burned area from satellite Earth observation. Remote Sens. Environ. 225, 45–64. doi:10.1016/j.rse.2019.02.013
Corner, A., Whitmarsh, L., and Xenias, D. (2012). Uncertainty, scepticism and attitudes towards climate change: Biased assimilation and attitude polarisation. Clim. change 114, 463–478. doi:10.1007/s10584-012-0424-6
Cronin, J., Anandarajah, G., and Dessens, O. (2018). Climate change impacts on the energy system: A review of trends and gaps. Clim. change 151 (2), 79–93. doi:10.1007/s10584-018-2265-4
DeFries, R., Achard, F., Brown, S., Herold, M., Murdiyarso, D., Schlamadinger, B., et al. (2007). Earth observations for estimating greenhouse gas emissions from deforestation in developing countries. Environ. Sci. policy 10 (4), 385–394. doi:10.1016/j.envsci.2007.01.010
Deng, Z., Ciais, P., Tzompa-Sosa, Z. A., Saunois, M., Qiu, C., Tan, C., et al. (2022). Comparing national greenhouse gas budgets reported in UNFCCC inventories against atmospheric inversions. Earth Syst. Sci. Data 14, 1639–1675. doi:10.5194/essd-14-1639-2022
Erhardt, R., Bell, J., Blanton, B., Nutter, F., Robinson, M., and Smith, R. (2019). Stronger climate resilience with insurance. Bull. Am. Meteorol. Soc. 100 (8), 1549–1552. doi:10.1175/BAMS-D-19-0073.1
Etzkowitz, H. (2003). Innovation in innovation: The triple helix of university-industry-government relations. Soc. Sci. Inf. 42 (3), 293–337. doi:10.1177/05390184030423002
Fan, L., Wigneron, J. P., Ciais, P., Chave, J., Brandt, M., Fensholt, R., et al. (2019). Satellite-observed pantropical carbon dynamics. Nat. Plants 5, 944–951. doi:10.1038/s41477-019-0478-9
Fetterer, F., Knowles, K., Meier, W. N., Savoie, M., and Windnagel, A. K. (2017). Updated daily. Sea ice index, version 3. Boulder, Colorado USA: NSIDC: National Snow and Ice Data Center, (date accessed July 18, 2022). doi:10.7265/N5K072F8
Ffoulkes, C., Hockridge, B., Illman, H., Holmes, G., Manning, F., and Wilson, L. (2021). Research to review and update indicators of climate-related risks and actions in England. ADAS report to the Committee on Climate Change.
Findlater, K., Webber, S., Kandlikar, M., and Donner, S. (2021). Climate services promise better decisions but mainly focus on better data. Nat. Clim. Chang. 11, 731–737. doi:10.1038/s41558-021-01125-3
Friedlingstein, P., Jones, M. W., O'Sullivan, M., Andrew, R. M., Bakker, D. C. E., Hauck, J., et al. (2021). Global carbon budget 2021, in review. Earth Syst. Sci. Data Discuss. [preprint]. doi:10.5194/essd-2021-386
GCOS (2020). Global climate indicators. Available at: https://gcos.wmo.int/en/global-climate-indicators (last access July 22, 2022).
GEO, ClimateTRACE, , WGIC, 2021. GHG monitoring from space: A mapping of capabilities across public, private, and hybrid satellite missions.
Goldblatt, R., Addas, A., Crull, D., Maghrabi, A., Levin, G. G., and Rubinyi, S. (2021). Remotely sensed derived land surface temperature (LST) as a proxy for air temperature and thermal comfort at a small geographical scale. Land 10 (4), 410. doi:10.3390/land10040410
Goodchild, M. F., Guo, H., Annoni, A., Bian, L., De Bie, K., Campbell, F., et al. (2012). Next-generation digital Earth. Proc. Natl. Acad. Sci. U. S. A. 109 (28), 11088–11094. doi:10.1073/pnas.1202383109
Grassi, G., Stehfest, E., Rogelj, J., van Vuuren, D., Cescatti, A., House, J., et al. (2021). Critical adjustment of land mitigation pathways for assessing countries’ climate progress. Nat. Clim. Chang. 11, 425–434. doi:10.1038/s41558-021-01033-6
Hadorn, G. H., Biber-Klemm, S., Grossenbacher-Mansuy, W., Hoffmann-Riem, H., Joye, D., Pohl, C., et al. (2008). “The emergence of transdisciplinarity as a form of research,” in Handbook of transdisciplinary research (Dordrecht: Springer), 19–39.
Harris, N. L., Gibbs, D. A., Baccini, A., Birdsey, R. A., de Bruin, S., Farina, M., et al. (2021). Global maps of twenty-first century forest carbon fluxes. Nat. Clim. Chang. 11, 234–240. doi:10.1038/s41558-020-00976-6
Harris, N. L., Hagen, S. C., Saatchi, S. S., Pearson, T. R. H., Woodall, C. W., Domke, G. M., et al. (2016). Attribution of net carbon change by disturbance type across forest lands of the conterminous United States. Carbon Balance Manag. 11 (1), 24–21. doi:10.1186/s13021-016-0066-5
Hegglin, M. I., Tegtmeier, S., Anderson, J., Bourassa, A. E., Brohede, S., Degenstein, D., et al. (2021). Overview and update of the SPARC data initiative: Comparison of stratospheric composition measurements from satellite limb sounders. Earth Syst. Sci. Data 13, 1855–1903. doi:10.5194/essd-13-1855-2021
Hewitt, C. D., Allis, E., Mason, S. J., Muth, M., Pulwarty, R., Shumake-Guillemot, J., et al. (2020). Making society climate resilient: International progress under the global framework for climate services. Bull. Am. Meteorological Soc. 101 (2), E237–E252. doi:10.1175/bams-d-18-0211.1
Hjort, J., Streletskiy, D., Doré, G., Wu, Q., Bjella, K., and Luoto, M. (2022). Impacts of permafrost degradation on infrastructure. Nat. Rev. Earth Environ. 3, 24–38. doi:10.1038/s43017-021-00247-8
Holling, C. S. (2004). From complex regions to complex worlds. Ecol. Soc. 9 (1), 11. doi:10.5751/es-00612-090111
Hollmann, R., Merchant, C. J., Saunders, R., Downy, C., Buchwitz, M., Cazenave, A., et al. (2013). The ESA climate change initiative: Satellite data records for essential climate variables. Bull. Am. Meteorological Soc. 94 (10), 1541–1552. doi:10.1175/bams-d-11-00254.1
Hu, H., Landgraf, J., Detmers, R., Borsdorff, T., Aan de Brugh, J., and Aben, I. (2018). Toward global mapping of methane with TROPOMI: First results and intersatellite comparison to GOSAT. Geophys. Res. Lett. 45, 3682–3689. doi:10.1002/2018GL077259
Hugonnet, R., McNabb, R., Berthier, E., Menounos, B., Nuth, C., Girod, L., et al. (2021). Accelerated global glacier mass loss in the early twenty-first century. Nature 592, 726–731. doi:10.1038/s41586-021-03436-z
Ilyina, T., Li, H., Spring, A., Müller, W. A., Bopp, L., Chikamoto, M. O., et al. (2021). Predictable variations of the carbon sinks and atmospheric CO2 growth in a multi-model framework. Geophys. Res. Lett. 48, e2020GL090695. doi:10.1029/2020GL090695
IPCC (2013). “Climate change 2013: The physical science basis,” in Contribution of working group I to the fifth assessment report of the intergovernmental panel on climate change. Editors T. F. Stocker, D. Qin, G.-K. Plattner, M. Tignor, S. K. Allen, J. Boschunget al. (Cambridge, United Kingdom and New York, NY: Cambridge University Press), 1535.
IPCC (2018). “Global Warming of 1.5°C. An IPCC Special Report on the impacts of global warming of 1.5°C above pre-industrial levels and related global greenhouse gas emission pathways,” in The context of strengthening the global response to the threat of climate change, sustainable development, and efforts to eradicate poverty [Masson-Delmotte. Editors P. Zhai, H.-O. Pörtner, D. Roberts, J. Skea, P. R. Shuklaet al. (Cambridge, UK and New York, NY, USA: Cambridge University Press), 616. doi:10.1017/9781009157940
IPCC (2019). in Refinement to the 2006 IPCC guidelines for national greenhouse gas inventories. Editors E. Calvo Buendia, K. Tanabe, A. Kranjc, J. Baasansuren, M. Fukuda, S. Ngarizeet al. (Switzerland: IPCC).
IPCC (2021). “Climate change 2021: The physical science basis,” in Contribution of working group I to the sixth assessment report of the intergovernmental panel on climate change. Editors V. Masson-Delmotte, P. Zhai, A. Pirani, S. L. Connors, C. Péan, S. Bergeret al. (Cambridge, United Kingdom and New York, NY: Cambridge University Press). doi:10.1017/9781009157896
Janssens-Maenhout, G., Pinty, B., Dowell, M., Zunker, H., Andersson, E., Balsamo, G., et al. (2020). Toward an operational anthropogenic CO2 emissions monitoring and verification support capacity. Bull. Am. Meteorological Soc. 101 (8), E1439–E1451. doi:10.1175/bams-d-19-0017.1
Kahneman, D., and Tversky, A. (1982). Variants of uncertainty. Cognition 11, 143–157. doi:10.1016/0010-0277(82)90023-3
Kaminski, T., Scholze, M., Rayner, P., Voßbeck, M., Buchwitz, M., Reuter, M., et al. (2022). Assimilation of atmospheric CO2 observations from space can support national CO2 emission inventories. Environ. Res. Lett. 17 (1), 014015. doi:10.1088/1748-9326/ac3cea
Klein, D., Carazo, M. P., Doelle, M., Bulmer, J., and Higham, A. (Editors) (2017). The Paris Agreement on climate change: Analysis and commentary (Oxford University Press).
Kort, E. A., Frankenberg, C., Costigan, K. R., Lindenmaier, R., Dubey, M. K., and Wunch, D. (2014). Four corners: The largest US methane anomaly viewed from space. Geophys. Res. Lett. 41 (19), 6898–6903. doi:10.1002/2014GL061503
Kumar, S., Getirana, A., Libonati, R., Hain, C., Mahanama, S., and Andela, N. (2022). Changes in land use enhance the sensitivity of tropical ecosystems to fire-climate extremes. Sci. Rep. 12, 964. doi:10.1038/s41598-022-05130-0
Lauvaux, T., Giron, C., Mazzolini, M., d’Aspremont, A., Duren, R., Cusworth, D., et al. (2022). Global assessment of oil and gas methane ultra-emitters. Science 375 (6580), 557–561. doi:10.1126/science.abj4351
Lawford, R., Strauch, A., Toll, D., Fekete, B., and Cripe, D. (2013). Earth observations for global water security. Curr. Opin. Environ. Sustain. 5 (6), 633–643. doi:10.1016/j.cosust.2013.11.009
Lenton, T. M., Held, H., Kriegler, E., Hall, J. W., Lucht, W., Rahmstorf, S., et al. (2008). Tipping elements in the Earth's climate system. Proc. Natl. Acad. Sci. U. S. A. 105 (6), 1786–1793. doi:10.1073/pnas.0705414105
Lloyd, E. A., and Shepherd, T. G. (2021). Meaningful climate science. Clim. Change 167, 28. doi:10.1007/s10584-021-03177-y
Løhre, E., Juanchich, M., Sirota, M., Teigen, K. H., and Shepherd, T. G. (2019). Climate scientists’ wide prediction intervals may be more likely but are perceived to be less certain. Weather Clim. Soc. 11 (3), 565–575. doi:10.1175/wcas-d-18-0136.1
Lorenzo-Alonso, A., Utanda, Á., Aulló-Maestro, M. E., and Palacios, M. (2019). Earth observation actionable information supporting disaster risk reduction efforts in a sustainable development framework. Remote Sens. (Basel). 11, 49. doi:10.3390/rs11010049
Marconcini, M., Metz-Marconcini, A., Üreyen, S., Palacios-Lopez, D., Hanke, W., Bachofer, F., et al. (2020). Outlining where humans live, the world settlement footprint 2015. Sci. Data 7, 242. doi:10.1038/s41597-020-00580-5
Massari, C., Modanesi, S., Dari, J., Gruber, A., De Lannoy, G. J., Girotto, M., et al. (2021). A review of irrigation information retrievals from space and their utility for users. Remote Sens. 13 (20), 4112. doi:10.3390/rs13204112
Matiu, M., Crespi, A., Bertoldi, G., Carmagnola, C. M., Marty, C., Morin, S., et al. (2021). Observed snow depth trends in the European alps: 1971 to 2019. Cryosphere 15 (3), 1343–1382. doi:10.5194/tc-15-1343-2021
Merchant, C. J., Paul, F., Popp, T., Ablain, M., Bontemps, S., Defourny, P., et al. (2017). Uncertainty information in climate data records from Earth observation. Earth Syst. Sci. Data 9 (2), 511–527. ISSN 1866-3516. doi:10.5194/essd-9-511-2017
Michetti, M., Cogo, E., and Venturini, S. (2021). “Monitoring adaptation using Earth observations in view of the “global Stocktake”,” in Handbook of climate change management. Editors W. Leal Filho, J. Luetz, and D. Ayal (Cham: Springer). doi:10.1007/978-3-030-22759-3_153-1
Munafò, M. R., Nosek, B. A., Bishop, D. V., Button, K. S., Chambers, C. D., Percie du Sert, N., et al. (2017). A manifesto for reproducible science. Nat. Hum. Behav. 1 (1), 0021–0029. doi:10.1038/s41562-016-0021
Nakalembe, C., Becker-Reshef, I., Bonifacio, R., Hu, G., Humber, M. L., Justice, C. J., et al. (2021). A review of satellite-based global agricultural monitoring systems available for Africa. Glob. Food Secur. 29, 100543. doi:10.1016/j.gfs.2021.100543
Nesha, M. K., Herold, M., De Sy, V., Duchelle, A. E., Martius, C., Branthomme, A., et al. (2021). An assessment of data sources, data quality and changes in national forest monitoring capacities in the Global Forest Resources Assessment 2005–2020. Environ. Res. Lett. 16 (5), 054029. doi:10.1088/1748-9326/abd81b
Nicholls, R. J., and Cazenave, A. (2010). Sea-level rise and its impact on coastal zones. science 328 (5985), 1517–1520. doi:10.1126/science.1185782
Nissan, H., Goddard, L., de Perez, E. C., Furlow, J., Baethgen, W., Thomson, M. C., et al. (2019). On the use and misuse of climate change projections in international development. WIREs Clim. Change 10 (3), e579. doi:10.1002/wcc.579
Palmer, P. I., Feng, L., Lunt, M. F., Parker, R. J., Bösch, H., Lan, X., et al. (2021). The added value of satellite observations of methane for understanding the contemporary methane budget. Philos. Trans. A Math. Phys. Eng. Sci., 20210106. doi:10.1098/rsta.2021.0106
Perugini, L., Pellis, G., Grassi, G., Ciais, P., Dolman, H., House, J. I., et al. (2021). Emerging reporting and verification needs under the Paris Agreement: How can the research community effectively contribute? Environ. Sci. policy 122, 116–126. doi:10.1016/j.envsci.2021.04.012
Pisaric, M. F., Thienpont, J. R., Kokelj, S. V., Nesbitt, H., Lantz, T. C., Solomon, S., et al. (2011). Impacts of a recent storm surge on an Arctic delta ecosystem examined in the context of the last millennium. Proc. Natl. Acad. Sci. U. S. A. 108 (22), 8960–8965. doi:10.1073/pnas.1018527108
Popp, A., Humpenöder, F., Weindl, I., Bodirsky, B. L., Bonsch, M., Lotze-Campen, H., et al. (2014). Land-use protection for climate change mitigation. Nat. Clim. Chang. 4, 1095–1098. doi:10.1038/nclimate2444
Popp, T., Hegglin, M. I., Hollmann, R., Ardhuin, F., Bartsch, A., Bastos, A., et al. (2020). Consistency of satellite climate data records for Earth system monitoring. Bull. Am. Meteorological Soc. 101 (11), E1948–E1971. doi:10.1175/bams-d-19-0127.1
Pulkkinen, K., Undorf, S., Bender, F., Wikman-Svahn, P., Doblas-Reyes, F., Flynn, C., et al. (2022). The value of values in climate science. Nat. Clim. Chang. 12, 4–6. doi:10.1038/s41558-021-01238-9
Rose, D. C. (2015). The case for policy‐relevant conservation science. Conserv. Biol. 29 (3), 748–754. doi:10.1111/cobi.12444
Rose, D. (2014). Five ways to enhance the impact of climate science. Nat. Clim. Change 4, 522–524. doi:10.1038/nclimate2270
Running, S. W. (2012). A measurable planetary boundary for the biosphere. Science 337 (6101), 1458–1459. doi:10.1126/science.1227620
Running, S. W., Justice, C. O., Salomonson, V., Hall, D., Barker, J., Kaufmann, Y. J., et al. (1994). Terrestrial remote sensing science and algorithms planned for EOS/MODIS. Int. J. remote Sens. 15 (17), 3587–3620. doi:10.1080/01431169408954346
Salawitch, R., (Lead Author), , Fahey, D. W., Hegglin, M. I., McBride, L. A., Tribett, W. R., et al. (2018). Twenty questions and answers about the ozone layer: 2018 update, scientific assessment of ozone depletion, 2019. Geneva, Switzerland: World Meteorological Organization, 84. (available at: https://csl.noaa.gov/assessments/ozone/2018/twentyquestions/.
Santoro, M., and Cartus, O. (2021). ESA biomass climate change initiative (Biomass_cci): Global datasets of forest above-ground biomass for the years 2010, 2017 and 2018, v2. Cent. Environ. Data Anal.
Sarewitz, D. (2004). How science makes environmental controversies worse. Environ. Sci. policy 7 (5), 385–403. doi:10.1016/j.envsci.2004.06.001
Saunois, M., Stavert, A. R., Poulter, B., Bousquet, P., Canadell, J. G., Jackson, R. B., et al. (2020). The global methane budget 2000–2017. Earth Syst. Sci. Data 12 (3), 1561–1623. doi:10.5194/essd-12-1561-2020
Schneising, O., Buchwitz, M., Reuter, M., Bovensmann, H., Burrows, J. P., Borsdorff, T., et al. (2019). A scientific algorithm to simultaneously retrieve carbon monoxide and methane from TROPOMI onboard Sentinel-5 Precursor. Atmos. Meas. Tech. 12, 6771–6802. doi:10.5194/amt-12-6771-2019
Schneising, O., Buchwitz, M., Reuter, M., Vanselow, S., Bovensmann, H., and Burrows, J. P. (2020). Remote sensing of methane leakage from natural gas and petroleum systems revisited. Atmos. Chem. Phys. 20, 9169–9182. doi:10.5194/acp-20-9169-2020
Schumacher, E. F. (1973). Small is beautiful: Economics as if people mattered, 259. London: Blond & Briggs., 259, 1973.
Schuur, E. A., Bockheim, J., Canadell, J. G., Euskirchen, E., Field, C. B., Goryachkin, S. V., et al. (2008). Vulnerability of permafrost carbon to climate change: Implications for the global carbon cycle. BioScience 58 (8), 701–714. doi:10.1641/B580807
Scott, D., Dawson, J., and Jones, B. (2008). Climate change vulnerability of the US Northeast winter recreation–tourism sector. Mitig. Adapt. Strateg. Glob. Chang. 13 (5), 577–596. doi:10.1007/s11027-007-9136-z
Shepherd, T. G. (2021). Bringing physical reasoning into statistical practice in climate-change science. Clim. Change 169, 2. ISSN 0165. doi:10.1007/s10584-021-03226-6
Shepherd, T. G., Plummer, D. A., Scinocca, J. F., Hegglin, M. I., Fioletov, V. E., Reader, M. C., et al. (2014). Reconciliation of halogen-induced ozone loss with the total-column ozone record. Nature Geosci. 7, 443–449. doi:10.1038/NGEO2155
Sherwood, S. C., Webb, M. J., Annan, J. D., Armour, K. C., Forster, P. M., Hargreaves, J. C., et al. (2020). An assessment of Earth's climate sensitivity using multiple lines of evidence. Rev. Geophys. 58 (4), e2019RG000678. doi:10.1029/2019rg000678
Solaun, K., and Cerdá, E. (2019). Climate change impacts on renewable energy generation. A review of quantitative projections. Renew. Sustain. energy Rev. 116, 109415. doi:10.1016/j.rser.2019.109415
Song, X. P., Hansen, M. C., Stehman, S. V., Potapov, P. V., Tyukavina, A., Vermote, E. F., et al. (2018). Global land change from 1982 to 2016. Nature 560, 639–643. doi:10.1038/s41586-018-0411-9
Tadesse, L., Suryabhagavan, K. V., Sridhar, G., and Legesse, G. (2017). Land use and land cover changes and soil erosion in yezat watershed, north western Ethiopia. Int. soil water conservation Res. 5 (2), 85–94. doi:10.1016/j.iswcr.2017.05.004
Tapley, B. D., Watkins, M. M., Flechtner, F., Reigber, C., Bettadpur, S., Rodell, M., et al. (2019). Contributions of GRACE to understanding climate change. Nat. Clim. Chang. 9, 358–369. doi:10.1038/s41558-019-0456-2
Thompson, M., and Warburton, M. (2019). “Decision making under contradictory certainties: How to save the himalayas when you can't find out what's wrong with them,” in Risk management (Abingdon, United Kingdom: Routledge), 447–478.
Trewin, B., Cazenave, A., Howell, S., Huss, M., Isensee, K., Palmer, M. D., et al. (2021). Headline indicators for global climate monitoring. Bull. Am. Meteorological Soc. 102 (1), E20–E37. doi:10.1175/bams-d-19-0196.1
UNFCCC (2015a). Decision 1/CP.21. Adoption of the Paris Agreement. FCCC/CP/2015/10/Add.1. Available at: https://unfccc.int/resource/docs/2015/cop21/eng/10a01.pdf#page=2 (last access May 1, 2021).
UNFCCC (2015b). The enhanced transparency framework. Available at: https://unfccc.int/enhanced-transparency-framework (last access November 25, 2021).
UNFCCC (2015c). The Global Stocktake. Available at: https://unfccc.int/topics/science/workstreams/global-stocktake-referred-to-in-article-14-of-the-paris-agreement (last access October 4, 2021).
UNFCCC, Climate Change Secretariat (2022). Reference manual for the enhanced transparency framework under the Paris Agreement. Available at: https://unfccc.int/fr/node/268136 (last access April 3, 2022).
United Nations Environment Programme, (2021). Adaptation Gap Report 2021: The gathering storm – adapting to climate change in a post-pandemic world – executive Summary. Nairobi. Retrieved from https://wedocs.unep.org/xmlui/bitstream/handle/20.500.11822/37312/AGR21_ESEN.pdf (last access July 13, 2022).
Victor, D. G., Akimoto, K., Kaya, Y., Yamaguchi, M., Cullenward, D., and Hepburn, C. (2017). Prove Paris was more than paper promises. Nature 548, 25–27. doi:10.1038/548025a
Vincent, K., Daly, M., Scannell, C., and Leathes, B. (2018). What can climate services learn from theory and practice of co-production? Clim. Serv. 12, 48–58. doi:10.1016/j.cliser.2018.11.001
Vishnu, C. L., Sajinkumar, K. S., Oommen, T., Coffman, R. A., Thrivikramji, K. P., Rani, V. R., et al. (2019). Satellite-based assessment of the August 2018 flood in parts of Kerala, India. Geomat. Nat. Hazards Risk 101, 758–767. doi:10.1080/19475705.2018.1543212
Wang, Y., Broquet, G., Bréon, F.-M., Lespinas, F., Buchwitz, M., Reuter, M., et al. (2020). PMIF v1.0: Assessing the potential of satellite observations to constrain CO2 emissions from large cities and point sources over the globe using synthetic data. Geosci. Model. Dev. 13, 5813–5831. doi:10.5194/gmd-13-5813-2020
Whitcraft, A. K., Becker-Reshef, I., Justice, C. O., Gifford, L., Kavvada, A., and Jarvis, I. (2019). No pixel left behind: Toward integrating Earth observations for agriculture into the united nations sustainable development goals framework. Remote Sens. Environ. 235, 111470. doi:10.1016/j.rse.2019.111470
Wigneron, J.-P., Li, X., Frappart, F., Fan, L., Al-Yaari, A., De Lannoy, G., et al. (2021). SMOS-IC data record of soil moisture and L-VOD: Historical development, applications and perspectives. Remote Sens. Environ. 254, 112238. doi:10.1016/j.rse.2020.112238
WMO (2022). State of the global climate 2021, WMO- No. 1290. Available at: https://library.wmo.int/index.php?lvl=notice_display&id=22080.
Woodward, F. I., Lomas, M. R., and Quaife, T. (2008). Global responses of terrestrial productivity to contemporary climatic oscillations. Phil. Trans. R. Soc. B 363 (1504), 2779–2785. ISSN 0962-8436. doi:10.1098/rstb.2008.0017
Woolway, R. I., Kraemer, B. M., Lenters, J. D., Merchant, C. J., O’Reilly, C. M., and Sharma, S. (2020). Global lake responses to climate change. Nat. Rev. Earth Environ. 1, 388–403. doi:10.1038/s43017-020-0067-5
World Resources Institute (2021). Navigating the Paris Agreement rulebook: Enhanced transparency framework. Available at: https://www.wri.org/paris-rulebook/enhanced-transparency-framework (last access August 1, 2021).
Zhao, Y., Saunois, M., Bousquet, P., Lin, X., Berchet, A., Hegglin, M. I., et al. (2020). On the role of trend and variability in the hydroxyl radical (OH) in the global methane budget. Atmos. Chem. Phys. 20, 13011–13022. doi:10.5194/acp-20-13011-2020
Zheng, B., Chevallier, F., Ciais, P., Broquet, G., Wang, Y., Lian, J., et al. (2020). Observing carbon dioxide emissions over China's cities and industrial areas with the Orbiting Carbon Observatory-2. Atmos. Chem. Phys. 20, 8501–8510. doi:10.5194/acp-20-8501-2020
Keywords: climate change, Earth observation, Paris Agreement, enhanced transparency framework, mitigation, adaptation, loss and damage, global stocktake
Citation: Hegglin MI, Bastos A, Bovensmann H, Buchwitz M, Fawcett D, Ghent D, Kulk G, Sathyendranath S, Shepherd TG, Quegan S, Röthlisberger R, Briggs S, Buontempo C, Cazenave A, Chuvieco E, Ciais P, Crisp D, Engelen R, Fadnavis S, Herold M, Horwath M, Jonsson O, Kpaka G, Merchant CJ, Mielke C, Nagler T, Paul F, Popp T, Quaife T, Rayner NA, Robert C, Schröder M, Sitch S, Venturini S, van der Schalie R, van der Vliet M, Wigneron J-P and Woolway RI (2022) Space-based Earth observation in support of the UNFCCC Paris Agreement. Front. Environ. Sci. 10:941490. doi: 10.3389/fenvs.2022.941490
Received: 11 May 2022; Accepted: 25 July 2022;
Published: 05 October 2022.
Edited by:
Lynn Donelson Wright, William & Mary's Virginia Institute of Marine Science, United StatesReviewed by:
Antonio T. Monteiro, University of Lisbon, PortugalCharles Reid Nichols, Marine Information Resources Corporation (MIRC), United States
Copyright © 2022 Hegglin, Bastos, Bovensmann, Buchwitz, Fawcett, Ghent, Kulk, Sathyendranath, Shepherd, Quegan, Röthlisberger, Briggs, Buontempo, Cazenave, Chuvieco, Ciais, Crisp, Engelen, Fadnavis, Herold, Horwath, Jonsson, Kpaka, Merchant, Mielke, Nagler, Paul, Popp, Quaife, Rayner, Robert, Schröder, Sitch, Venturini, van der Schalie, van der Vliet, Wigneron and Woolway. This is an open-access article distributed under the terms of the Creative Commons Attribution License (CC BY). The use, distribution or reproduction in other forums is permitted, provided the original author(s) and the copyright owner(s) are credited and that the original publication in this journal is cited, in accordance with accepted academic practice. No use, distribution or reproduction is permitted which does not comply with these terms.
*Correspondence: Michaela I. Hegglin, bS5pLmhlZ2dsaW5AcmVhZGluZy5hYy51aw==