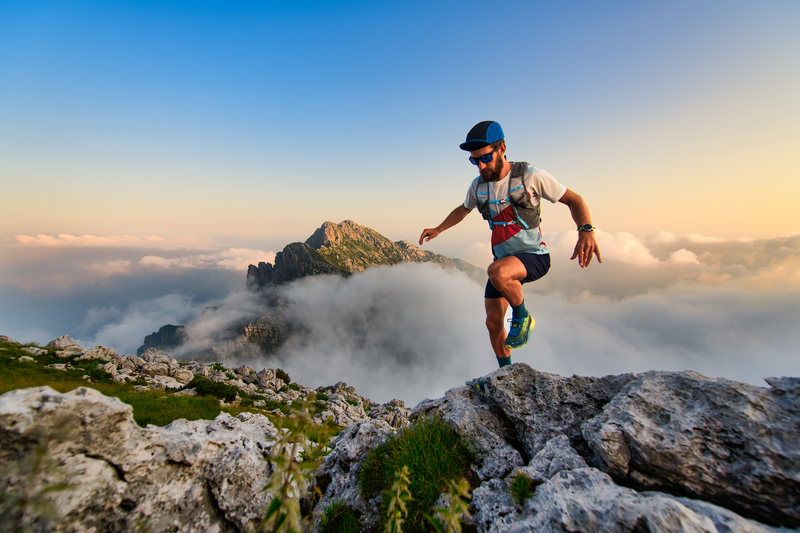
95% of researchers rate our articles as excellent or good
Learn more about the work of our research integrity team to safeguard the quality of each article we publish.
Find out more
ORIGINAL RESEARCH article
Front. Environ. Sci. , 10 August 2022
Sec. Environmental Economics and Management
Volume 10 - 2022 | https://doi.org/10.3389/fenvs.2022.939243
This article is part of the Research Topic Managing Climate Risk in the Global Economic System in the Post-COVID-19 Era View all 11 articles
The usage of data production factor (DPF) has been extensively studied in academic research and industry. The purpose of this study is to examine the causal effects of DPF adoption on company performance. We firstly provide a measurement of DPF adoption by text mining, which is superior to previous studies that use only single metric. Then, based on PSM-DID method, we use the data of China’s listed companies from 2011 to 2019 to identify the causal relationship between data elements adoption and company’s performance. We find that the adoption of DPF can significantly increase companies’ performance. Further heterogeneity tests show that companies from the service industry and state-owned companies achieve a significant improvement in the performance after adopting DPFs in production. Altogether, this study provides the micro evidence on the relationship between the adoption of DPFs and company performance, providing significant implications for the development of digitalization and intelligence production.
The widespread application and innovation of new-generation information technology have greatly promoted the digital transformation of companies and the reconstruction of productivity and production relations. For the first time, the Fourth Plenary Session of the 19th Central Committee of the Communist Party of China recognized data production factor (DPF) as the seventh production factor, reflecting the important role of data in improving productivity in the context of high-quality development. In April 2020, the State Council of the CPC Central Committee issued a document specifically for production factor market, clearly emphasizing the need to “accelerate the cultivation of DPF market, enhance the value of social data resources, and cultivate new industries, new business models and new modes of the digital economy.”
DPF provides the main source of potential for companies to achieve exponential and significant growth. Consequently, companies are gradually increasing their dynamic investments in DPF. The two-way promotion of DPF and companies has enhanced the rate of marketization of DPF and laid the technical foundation for companies to enter the new era of the digital economy. Considering that production factor is a new and powerful resource, there are two major strategic issues that need to be urgently addressed. Is DPF able to create higher value in the process of interaction with traditional production factors, such as labor, capital, land, technology, knowledge, and management? Is DPF conducive to improve the dynamic capability and innovation capacity of companies?
There is already a good number of literatures on the topic of DPF’s role in productivity (Evangelista et al., 2012; Enrique et al., 2018). It has been believed that DPF do not act in a single form on economic development, but mainly realize the duty of data empowerment through interaction with traditional production factors. Specifically, DPF do not automatically provide the required information and values without going through appropriate steps, such as data filtering and processing. This means that companies need to use various analytical tools to filter out the useful information contained in data as a scientific basis for decision-making (Baesens et al., 2016). For example, by adopting big data technology in the “precision marketing” strategy, companies can comprehensively grasp consumer demand and market trends in a timelier manner through market analysis, pinpoint the target group of products, and increase marketing interaction (Xue, 2021). The combination of DPF with traditional production factors has shown innovative effects on the development of companies. For instance, when DPF is combined with capital, the increasing investments in R&D lead to a significant increase in the technological innovation of companies. Similarly, a combination with DPF and labors can effectively improve production efficiency. Furthermore, the most significant results are achieved when combining DPF with technology, which can help advance robust technological progress and establish an efficient digital system for companies by taking advantage of the multidimensionality and large capacity of big data (Lin and Meng, 2021). While the accumulation of data capital will further improve data processing efficiency, the combination also increases the overall productivity of companies and boosts economic growth. In summary, DPF provide new resources and strong guarantees for companies to transform and accelerate their adaptation to the era of the digital economy, activate industrial digitization, and promote productivity, innovation, and development.
Recent years have witnessed an increase of literatures concerning the effect of DPF adoption on company performance (Ferreira et al., 2019; Nasiri et al., 2020). The ease of access to DPF is an important reason for the widening gap in size and performance between large- and medium-sized companies (Begenau et al., 2018). The combination of data collected and published by the government and various statistical agencies, as well as companies’ own data, enhances the efficiency of companies’ decision-making (Hughes-Cromwick and Coronado, 2019). To further evaluate the impact of DPF adoption, some scholars have proposed to open the “black box” of the value realization process and multidimensional value creation mechanism of DPF by establishing the benchmark model of “factor-mechanism-performance,” combining its social attributes and dynamic integration theory (Yin et al., 2022). This can provide effective theoretical and practical insights into the sustainable development of companies.
However, the existing literatures on the impact of DPF on company performance is still inadequate. A primary drawback is the lack of comprehensive measurement of DPF adoption. Common measurements include property (Liu et al., 2022), the quality of the corporate website (Bernal et al., 2018), AI related technologies or Big Data analytics. Besides, executives’ subjective perception of the technology application is also frequently used, which is measured by questionnaire (Tsou and Chen, 2021; Nasiri et al., 2020). Another shortcoming is that previous literatures have largely failed to focus on causal effects of DPF on company performance. The endogenous issues between company performance and its decision on DPF adoption should not be ignored. In light of this, causal inference methods, such as Difference-In-Difference model (DID), should be used in the empirical studies.
In this paper, we aim to answer the question: does DPF adoption has a positive influence on company performance? Empirical results with 3,233 Chinese listed companies are provided. Compared with previous works, this paper contributes to two points. First, a method for accurately determining whether a company adopts DPF is proposed based on text mining. Second, the adoption of DPF is treated as a quasi-natural experiment, and causal inference is performed through the PSM-DID model to accurately estimate the average gain in performance due to the adoption of DPF. Based on this, the channels of causal effects are further analyzed through heterogeneity analysis of industry and ownership.
The followings are organized as below: Section 2 presents the data sources and indicator settings, Section 3 describes the model setting, Section 4 shows the empirical results, and Section 5 concludes the paper.
The initial sample of this paper is all A-share listed companies in the Shanghai and Shenzhen stock markets. The research interval is from 2011 to 2019. Before 2011, there were more missing values in the data of companies. Due to the outbreak of the COVID-19 pandemic in 2020, which greatly impacted companies, there was a certain incompatibility compared to previous years. After removing sample companies with missing data and stocks with ST label, in short of Special Treatment, we finally obtain a sample of 3,366 companies.
Two sources of data are used in this paper. On the one hand, financial data disclosed by listed companies’ annual statements are from the Wind Economic and Financial Database. On the other hand, the Guotaian database provides textual data of companies’ basic information, such as company history, main business, technical innovation, and shareholding, which are reported annually.
We provide a measurement method of DPF adoption in a two-step process.
The measurement of DPF adoption in the aspect of company has been little studied. Therefore, the primary aim of this paper is to clarify the definitions of DPF. China’s 14th Five-Year Plan highlighted “giving full play to the advantages of massive data and rich application scenarios, promoting the deep integration of digital technology and the real economy and growing new engines of economic development” and discussed the specific path to activate the potential of DPF from three aspects: strengthening the application of key digital technology innovation, accelerating digital industrialization, and promoting industrial digital transformation. Accordingly, this paper defines companies that applying DPF from three perspectives: the supporting hardware facilities, the supporting digital technology, and the application in industrial development. Therefore, a list of keywords was selected according to the above definition.
1) Hardware-facility-supporting DPFs
To improve the complete system and industry chain composed of information collection, mining, analysis, and application, as well as the sharing of DPF, companies should establish a mature new digital infrastructure. Therefore, this paper selected the key terms as the Internet of Things, cloud computing, edge computing, artificial intelligence, blockchain, data center, big data, data technology, information technology, information system, information software, platform support, and database.
2) Technology-supporting DPFs
The existing forms of data elements mostly depend on the development of “big data” and have the characteristics of large flow, diversity, and multiple levels, which are distinctive from traditional data, especially with the role of new media, such as the internet, which expands the channels and scale of data collection, requiring companies to have high data processing and analysis capabilities and to be able to fully exploit the value of data. Based on this, we identified the following keywords: data processing, machine learning, cloud technology, data analysis, data transmission, information and intelligent manufacturing, data drive, information and system integration, internet information application, software definition, and intelligent leadership.
3) Application of DPFs in industrial development
The adoption of DPF has accelerated the upgrading of industrial structures and the transformation of companies. The rise of the digital economy has greatly contributed to the rapid development of online platforms. It is worth mentioning that the labor results of its formation are all digital results. In other words, after being applied to basic industries, such as industry, agriculture, and services, the digital economy has made outstanding contributions to the value of the products created. As such, this paper selected digital economy, electronic commerce, digital industrialization, digitalization, and company informatization as keywords.
The list of keywords in the above three cases is shown in Table 1, with a total of 29 keywords.
We perform a text mining process to determine whether a company adopt DPFs. First, we perform word splitting on the text data of the basic company profile, which is a required disclosure for China’s listed companies including the information of company history, main business, technological innovation, shareholding, etc. Then, the keywords contained in Table 1 are automatically checked by computer to see whether it appears in the word splitting results for each company. Further, we manually examine whether the appeared keywords conform to the semantic meaning. For example, the business scope of Company No. 2177 is related to the provision of “digital processing services” and other businesses involved in “data and information processing services.” The semantic meaning of the keywords “digital,” “data” and “information” here is consistent to our study. Therefore, the company is classified as a data element company. Another example is Company No. 2074, who has a business scope that includes “digital electrical equipment.” Since this product is a traditional production equipment, the keyword digitalization here is not in line with the semantic meaning. Therefore, the company is considered as not to adopt DPFs. The year in which the eligible keywords first appear is used as the initial year for adopting DPFs.
Table 2 presents how many companies use data elements as production inputs in each year from 2011 to 2019. In 2011, McKinsey reported that data have swept into every industry and business function and are now an important factor of production, alongside labor and capital (Manyika et al., 2011). Therefore, we consider 2011 as the initial year of our sample. Obviously, the number of companies using DPFs increase more than double in the sample period.
To reflect the economic efficiency of companies, this paper selected Earnings Per Share (EPS) as the dependent variable. EPS reflects the after-tax profit created per share and is one of the most important financial indicators of the profitability of listed companies. Generally speaking, the higher the EPS, the better the economic efficiency of the company.
To control for the factors that may trigger changes in the economic efficiency of companies other than the adoption of DPFs, this paper referred to Sheng et al. (2020) and Yang and Yang. (2019) on the factors influencing earnings per share. Details of the control variables are shown in Table 3. Additionally, the effects of province, company ownership, and industry on earnings per share are controlled as the fixed effects.
To investigate the effects of adopting DPFs on the growth of economic efficiency, a Difference-In- Difference (DID) method was used. The baseline model is set as follows:
The dependent variable
There may be a reverse causal relationship between DPF adoption and a company’s economic efficiency; that is, the behavior of a company using data elements in production may have a self-selection effect. The company will decide whether to use DPFs in production according to its own production situation. If we want to identify the causal effect of DPF adoption on the company’s economic efficiency, we need to solve the endogenous problem of reverse causality. Therefore, we draw on the practices of Heckman et al. (1998) and Loecker (2007) and use the PSM-DID method to identify the causal effects of DPF adoption on the company’s economic efficiency. The PSM-DID method is based on the PSM method. It further differentiates the outcome variable, effectively eliminating the common trend between the treated and control groups. Thus, using the PSM-DID method in analysis can help solve the problems of sample selection bias and reverse causality.
Specifically, we establish a logit model, as shown in Eq. 2, whose dependent variable is
A total of 3,323 company samples that adopt DPFs were selected as the treatment group through the company screening method described in the previous section, and 3,184 company samples were selected as the control group through Model (2). Table 5 presents descriptive statistics of the main variables.
The results of the baseline regression model are shown in Table 6, where the coefficient of the dummy variable cross term DATA × YEAR reflects the net effect of using DPFs on the economic efficiency. The results showed that the coefficient of the cross term was significantly positive, indicating that the companies that adopted DPFs have obtained significant improvements in their earnings per share.
From the regression coefficients of the control variables, the coefficient of total asset size
In the DID model, “parallel trends” is a very important assumption. If the parallel trend assumption holds, then there should be no significant difference between the treatment and control groups before the point at which the company adopts DPFs. The multi-period DID model used in this paper examined the treatment effects before and after the treatment period to test whether the model satisfies the parallel trend assumption. The model was set up as follows.
where
This paper examined
Since there are some differences between technical conditions and economic benefits among different industries, to explore the heterogeneous effect of adopting DPFs on the economic benefits of companies between industries. We divided the sample into two groups, i.e., industrial and service companies, according to the Classification of Industries of National Economy (GB/T 4754-2017), and perform regressions separately. Additionally, the fixed effects of the subsectors are controlled. The regression results of the industry heterogeneity model are shown in Table 8.
TABLE 8. Impact of DPF adoption on earnings per share (results of industry heterogeneity model regression).
In the regression results for the sample of service-sector companies, the coefficients of the dummy variable cross term
In the regression results for industrial companies, the coefficient of the dummy variable cross term
In the following, we examine the heterogeneity of ownership, mainly caused by varying degrees of influence by macro policies, different channels and management mechanisms for the introduction of new technologies and new elements. We divide the whole sample into two sub-samples, including state-owned companies (SOEs) and non-state-owned companies (nSOEs).
Table 9 shows the regression results of SOEs and nSOEs. Regarding to SOEs, the coefficient of the dummy variable cross term
TABLE 9. Impact of DPF adoption on earnings per share (ownership heterogeneity model with regression results for SOEs and non-SOE classification).
In the regression results for nSOEs, the coefficient of the dummy variable cross term
This paper focused on the role of data production factor in the context of the digital economy. We conducted an empirical study to test whether the adoption of DPFs has a significant impact on company performance, which addresses a current concern in economic development.
The study showed that 1) the adoption of DPFs has a positive effect on the earnings per share of companies, which will lead to a significant improvement in company performance; 2) in the study of the heterogeneity of company industries, it was found that the economic efficiency of the service industry companies that adopting DPFs showed a significant improvement, but this could not indicate a significant improvement in the performance of industrial companies; and 3) to analyze the heterogeneity effect of company ownership, this paper divided the sample into SOEs and non-SOEs. From the perspective of the lag in R&D investment on company performance, SOEs have a certain time advantage over non-SOEs in implementing the national policy in DPF, so improvements in SOEs’ performance are significant.
Based on the research findings, this paper put forward the following three policy recommendations: 1) Standardize the market for DPF, including the decision mechanism of data value, contribution, and remuneration as well as trading rules, and focus on the integration of data and knowledge management, while establishing and developing a knowledge value-oriented remuneration policy. 2) Vigorously promote the construction of infrastructure technical facilities, such as cloud computing, 5G networks, and distributed data centers, and improve the big data application environment. A large amount of data resources alone is not enough to support the improvement of company performance, and only by strengthening companies’ own data analysis capabilities and dynamic innovation capabilities can we achieve scientific decision-making and win in the market competition. 3) Implement various national development policies on DPF, especially for non-SOEs, as the uncertainty of the policy business environment will have a greater impact on the business vitality of companies. Furthermore, because DPF is an emerging concept, their developmental immaturity leads to instability, and adaptability to the market environment still needs to be improved. Therefore, various supporting policies should be improved as soon as possible.
There are three limitations of this study. First, collecting data only from Chinese listed companies may bias the findings and lack generalization. In future studies, more countries and industries should be investigated to enrich the existing theory and practice of data production factor. Second, measuring company performance through only a single metric is not comprehensive enough. Beyond Earnings Per Share (EPS), other performance measurements should be included, such as Tobin’s Q and Return on Equity (ROE). Finally, there are some factors that may affect company’s digital innovation, such as company’s status (Liu et al., 2021) and the attitudes of managers and staff concerning DPF adoption. Future study should consider the mediate effects of these factors.
The raw data supporting the conclusion of this article will be made available by the authors, without undue reservation.
RG and HW contributed to conception and design of the study. RF organized the database. RF and FL performed the statistical analysis. RF and YR wrote sections of the manuscript. All authors contributed to manuscript revision, read, and approved the submitted version.
RG is financially supported by the disciplinary funding of Central University of Finance and Economics (CUFE). HW is partially supported by the disciplinary funding of CUFE, Program for Innovation Research in CUFE, and the Emerging Interdisciplinary Project of CUFE.
The authors declare that the research was conducted in the absence of any commercial or financial relationships that could be construed as a potential conflict of interest.
All claims expressed in this article are solely those of the authors and do not necessarily represent those of their affiliated organizations, or those of the publisher, the editors and the reviewers. Any product that may be evaluated in this article, or claim that may be made by its manufacturer, is not guaranteed or endorsed by the publisher.
Baesens, B., Bapna, R., Marsden, J. R., Vanthienen, J., and Zhao, J. L. (2016). Transformational issues of big data and analytics in networked business. Mis Q. 40 (4), 807–818. doi:10.25300/misq/2016/40:4.03
Begenau, J., Farboodi, M., and Veldkamp, L. (2018). Big data in finance and the growth of large firms. J. Monetary Econ. 97, 71–87. doi:10.1016/j.jmoneco.2018.05.013
Bernal, E., Moral, A., Viruel, M., and Fernández Uclés, D. (2018). Evaluation of corporate websites and their influence on the performance of olive oil companies. Sustainability 10 (4), 1274. doi:10.3390/su10041274
Dai, X.-Y., and Cheng, L. (2013). Study on the threshold effect of R&D investment intensity on enterprise performance. Sci. Res. 31 (11), 1708–1716. (in Chinese). doi:10.15918/j.jbitss1009-3370.2018.1185
Enrique, B. J., Adoración, M. M., Miguel, M. V., and Domingo, F. U. (2018). Evaluation of corporate websites and their influence on the performance of olive oil companies. Sustainability 10 (4), 1274. doi:10.3390/su10041274
Evangelista, P., Mogre, R., Raspagliesi, P. A., and Sweeney, E. (2012). A survey based analysis of it adoption and 3PLs' performance. Supply Chain Manag. Int. J. 17 (2), 172–186. doi:10.1108/13598541211212906
Ferreira, J., Fernandes, C. I., and Ferreira, F. (2019). To be or not to be digital, that is the question: Firm innovation and performance. J. Bus. Res. 101, 583–590. doi:10.1016/j.jbusres.2018.11.013
Heckman, J. J., Ichimura, H., and Todd, P. (1998). Matching as an econometric evaluation estimator. Rev. Econ. Stud. 65 (2), 261–294. doi:10.1111/1467-937x.00044
Hughes-Cromwick, E., and Coronado, J. (2019). The value of US government data to US business decisions. J. Econ. Perspect. 33 (1), 131–146. doi:10.1257/jep.33.1.131
Lin, Z.-J., and Meng, Z.-X. (2021). The combination mechanism of data production factors-the perspective of complementary assets. J. Beijing Jiaot. Univ. Soc. Sci. Ed. 20 (02), 28–38. (in Chinese). doi:10.3969/j.issn.1672-8106.2021.02.003
Liu, Y., Dong, J., Mei, L., and Shen, R. (2022). Digital innovation and performance of manufacturing firms: An affordance perspective. Technovation, 102458. doi:10.1016/j.technovation.2022.102458
Liu, Y., Dong, J., Ying, Y., and Jiao, H. (2021). Status and digital innovation: A middle-status conformity perspective. Technol. Forecast. Soc. Change 168, 120781. doi:10.1016/j.techfore.2021.120781
Loecker, J. D. (2007). Do exports generate higher productivity? Evidence from Slovenia. J. Int. Econ. 73 (1), 69–98. doi:10.1016/j.jinteco.2007.03.003
Manyika, J., Chui, M., Brown, B., Bughin, J., Dobbs, R., Roxburgh, C., et al. (2011). Big data: The next frontier for innovation, competition, and productivity. McKinsey Global Institute. Available at: https://www.mckinsey.com/business-functions/mckinsey-digital/our-insights/big-data-the-next-frontier-for-innovation.
Meng, T.-Y., Fang, M.-F., and Zhu, J.-M. (2018). Analysis of influencing factors of earnings per share based on multiple linear regression - taking Anhui Province as an example. J. Pingxiang Univ. 2018 (03), 37–41. (in Chinese).
Nasiri, M., Ukko, J., Saunila, M., and Rantala, T. (2020). Managing the digital supply chain: The role of smart technologies. Technovation 96-97, 102121. doi:10.1016/j.technovation.2020.102121
Sheng, C.-C., Wu, W.-X., and Cai, R. (2020). Analysis on the influencing factors of earnings per share—Taking 115 information technology listed companies as examples. China’s Collect. Econ. 2020 (03), 70–71. (in Chinese).
Song, J.-L. (2019). Research on factors influencing stock prices of listed companies in China. China: Jilin University. (in Chinese).
Sun, Y. (2018). Research on the innovation of cross-border e-commerce business model in the context of big data. China: Northeast Normal University. (in Chinese).
Tsou, H.-T., and Chen, J.-S. (2021). How does digital technology usage benefit firm performance? Digital transformation strategy and organisational innovation as mediators. Technol. Analysis Strategic Manag. doi:10.1080/09537325.2021.1991575
Xue, H.-M. (2021). China’s enterprise marketing strategy innovation under the background of big data. China Mark. 2014 (14), 129–130. (in Chinese). doi:10.13939/j.cnki.zgsc.2021.14.129
Yang, H.-Y., and Yang, Y.-M. (2019). An empirical study on the influencing factors of earnings per share of agricultural listed companies. Contemp. Econ. 2019 (05), 3639. (in Chinese). doi:10.3969/j.issn.1007-9378.2019.05.011
Yin, X.-M., Lin, Z.-Y., Chen, J., and Lin, Y.-J. (2022). Research on dynamic process mechanism of data element value. Sci. Res. 40 (02), 220–229. (in Chinese). doi:10.16192/j.cnki.1003-2053.20210524.001
Keywords: data production factor, performance, text mining, PSM-DID, causal effect
Citation: Guan R, Fan R, Ren Y, Lu F and Wang H (2022) The casual effect of data production factor adoption on company performance: Empirical evidence from Chinese listed companies with PSM-DID. Front. Environ. Sci. 10:939243. doi: 10.3389/fenvs.2022.939243
Received: 08 May 2022; Accepted: 13 July 2022;
Published: 10 August 2022.
Edited by:
Yan Xia, Chinese Academy of Sciences (CAS), ChinaReviewed by:
Jiangbo Geng, Zhongnan University of Economics and Law, ChinaCopyright © 2022 Guan, Fan, Ren, Lu and Wang. This is an open-access article distributed under the terms of the Creative Commons Attribution License (CC BY). The use, distribution or reproduction in other forums is permitted, provided the original author(s) and the copyright owner(s) are credited and that the original publication in this journal is cited, in accordance with accepted academic practice. No use, distribution or reproduction is permitted which does not comply with these terms.
*Correspondence: Huijuan Wang, aHVpanVhbi13YW5nQDE2My5jb20=
Disclaimer: All claims expressed in this article are solely those of the authors and do not necessarily represent those of their affiliated organizations, or those of the publisher, the editors and the reviewers. Any product that may be evaluated in this article or claim that may be made by its manufacturer is not guaranteed or endorsed by the publisher.
Research integrity at Frontiers
Learn more about the work of our research integrity team to safeguard the quality of each article we publish.