- 1College of Economics and Management, Southwest University, Chongqing, China
- 2School of Economics and Business Administration, Chongqing University, Chongqing, China
- 3School of Public Policy and Administration, Chongqing University, Chongqing, China
- 4College of Business, Feng Chia University, Taichung, Taiwan
Under contract farming, companies usually develop compulsory standardized technical systems for farmers in developing economies. However, in actual production, farmers often have differences in adoption intensity. Using data from 384 households and multinomial endogenous treatment effect regression, this study analyzes factors affecting the adoption intensity of farmers regarding standardized technical systems and estimates the impacts of adoption intensity of the standardized technical systems on household incomes in Chongqing, China. We adapt a multinomial endogenous treatment effect regression framework to correct for selection bias and endogeneity originating from both observed and unobserved heterogeneity. The results show that some factors could significantly affect the adoption intensity, such as farmers, satisfaction with technicians, perception of technology, attitude toward risks of adopting technologies, number of technical trainings, the proportion of leased land, and distances to townships and technology extension stations. Different from current perspectives, the results also indicate that the highest adoption intensity of farmers regarding standardized technical systems does not bring the greatest benefits. Based on the characteristics of a plot, medium adoption intensity has a significant effect on quality improvements and income growth. The conclusion of this study provides an empirical basis for the formulation and evaluation of policies for farmers that apply standardized technology systems in developing countries.
1 Introduction
Advanced agricultural technologies are critical for combating climate change, increasing agricultural productivity and farmers’ welfare, and ensuring food security (Tesfaye et al., 2020; Hörne and Wollni, 2021). Academicians have conducted studies on the effects of adopting a single technology (Manda et al., 2020), a combination of technologies (Hörner and Wollni, 2022), and complex technological systems (Nakano et al., 2018) on farmers’ input, yield, and income.
The binary analysis framework is commonly used in existing studies on technology adoption, but in reality, most agricultural technology adoption is partial, dynamic, and progressive (Arslan et al., 2014). Some scholars have pointed out that the use of dichotomy was oversimplified, potentially resulting in the misleading conclusion (Foster and Rosenzweig, 2010; Brown et al., 2017). For a deeper study on technology adoption, some scholars have analyzed it from a perspective of adoption intensity, such as the impact of adoption intensity on differences in yield and income.
Most studies on adoption intensity use the proportion of the cultivated land area with technology applied (Ainembabazi et al., 2018; Mwaura et al., 2021; Thompson et al., 2021), the cumulative number of different technologies adopted (Isgin et al., 2008; Sharma et al., 2011; Gao et al., 2019; Kolady et al., 2021), or adoption intensity indicators (Kabir and Rainis, 2015; Rodthong et al., 2020). Gao et al. (2017) used the reduction rate of chemical pesticides per acre before and after the adoption of green control techniques (GCT), and Yahaya et al. (2019) used the number of acres from the total cultivable land used for the System of Rice Intensive (SRI) practices. Noltze et al. (2012) used cultivated land area, and Ainembabazi et al. (2018) used the proportions of improved varieties in the total yield and among agricultural products on the market to represent the adoption intensity of different technologies. An examination of the aforementioned studies on adoption intensity shows that existing studies generally ignore the behavior of technology implementers. The pursuit of leisure by household labor, difficulty in supervising hired labor, and timing requirements for input-based technologies, such as pesticides and fertilizers, could have an impact on adoption intensity, which in turn affects yield and income.
In terms of the factors that affect adoption intensity, several researchers (e.g., Sharma et al., 2011; Li et al., 2021; Thompson et al., 2021) conducted empirical studies on the factors of adoption intensity of different technologies and found that due to the heterogeneity of technologies, the impact direction and effect degree of different factors varied. Rodthong et al. (2020) and Kolady et al. (2021) observed that farmers’ technology adoption decisions and adoption intensity were two different choices and that some factors affecting initial technology adoption decisions were similar and some different.
There are few empirical studies on the impact of adoption intensity on income. Ainembabazi et al. (2018) found that the adoption intensity of agricultural research-for-development (AR4D) technologies in Central Africa had different effects on the welfare of poor farmers at different levels. Gao et al. (2019) found that compared with family farms that did not adopt GCTs, the welfare of family farms with high and low intensity of GCTs in China increased by 22.63% and 16.42%, respectively. In the study by Gao et al. (2019), the adoption of two or more sub-technologies was considered high intensity, and the adoption of one sub-technology was considered low intensity, which was a simple classification, ignoring differences among sub-technologies. Ma and Wang (2020) found that the use of the internet by Chinese farmers increased the adoption intensity of sustainable agricultural practices (SAPs). The 25th and 75th quantiles of adoption of SAPs were negatively correlated with farmers’ income and the highest negative effect occurred at a high quantile. Mensah-Bonsu et al. (2017) found that the number of land and water management practices (LWMPs) used by Ghana maize farmers was negatively correlated with land productivity (yield) and that the net effect of LWMPs was negative, i.e., adopting more LWMPs may not result in high land productivity.
The aforementioned literature review shows that farmers face increasingly complex agricultural technologies, there are often complex interactions between technologies, and there are significant differences in land endowment; therefore, it is necessary to extensively study the impact of differences in adoption intensity on income. However, studies in this area are rare. Tobacco is a critical crop its production is relatively labor- and technology-intensive, yet it is an important cash crop in many developing countries (Appau et al., 2020; Talukder et al., 2020; Wouter, 2020; Shonhe and Scoones, 2022). Dimara and Skuras (1998) investigated actors of the adoption of new varieties of flue-cured tobacco by farmers in Greece, and Omara et al. (2021) analyzed views and factors of the adoption of rocket barn technology by farmers in Uganda. Other than the aforementioned studies, there are very few published studies on the influence of tobacco technology adoption on income. Contract production of tobacco is practiced globally (Wang et al., 2014; Scoones et al., 2018) and requires farmers to implement tobacco production following established technical standards. This contract system has greatly improved the technical level and productivity of small farmers (Kuijpers and Swinnen, 2016; Mao et al., 2019), which also benefits the environment.
China implements strict quota management on tobacco production; tobacco farmers must sign a contract with tobacco companies to plant tobacco, and the production process must be conducted in strict accordance with the standardized technical system developed by the companies. It is generally believed that the high intensity of standardized technical systems by tobacco farmers is conducive to the income growth. However, there are often differences in the adoption intensity of tobacco farmers because of subjective and objective reasons. The purpose of this study is to examine factors affecting the adoption intensity of standardized technical systems by tobacco farmers and the effects of adoption intensity differences on the income of tobacco farmers. This study not only obtains empirical results in terms of the factors of adoption intensity and the impact on income but also finds that different from empirical judgment, the highest adoption intensity of standardized technical systems by tobacco farmers does not generate the greatest benefits.
This study guides tobacco farmers to reduce unnecessary technical input and improve input-output efficiency, and also has significance for protecting the environment and realizing sustainable development. The conclusion of our research study also can be used as a reference for other cash and grain crops and can help farmers in other regions of China and developing countries adopt appropriate technology intensity, which can maximize the output of limited resources taking into account social and environmental benefits.
The remainder of the study proceeds as follows: materials and methods are presented in Section 2. Section 3 describes the empirical results of the study. Section 4 reports the discussion and Section 5 highlight our major findings and conclude on policy implications.
2 Materials and methods
2.1 Study area
The planting area and yield of tobacco in China rank first in the world. Chongqing is located in the southwest of China, as one of the major areas for tobacco planting. Its planting area is distributed in 12 districts and counties in the Three Gorges Reservoir and Wuling Mountain area (see Figure 1). In 2018, the area was 4,05,700 mu (1 mu = 1/15 hectares), and 46.7 million kg of flue-cured tobacco was purchased. The average altitude of tobacco planting areas (typical mountainous areas) in Chongqing is 800–1,400 m, and tobacco is one of the few cash crops in these areas similar to that in Indonesia and the Philippines (Appau et al., 2019). Because of the long-term planting of tobacco in Chongqing, it is generally difficult to realize fallow tillage and crop rotation, resulting in continuous land degradation, serious ecological environmental pollution, frequent occurrence of pests and diseases, increasing production risks, and increasing planting costs, which restrict high and stable yields as well as quality improvements in tobacco production (Chen et al., 2018; Reichert et al., 2019). This seriously impacts the income of tobacco farmers.
2.2 Standardized technical systems for tobacco production
Chongqing Tobacco Company (CTC) established the ISO9000 quality management system for tobacco production in 2008, implemented the Good Agricultural Practices (GAPs) for tobacco in 2012, incorporated standardized production and protective agricultural practices into sustainable development goals, and established written requirements, such as prohibitions on the use of high-residue fertilizers and pesticides, in planting contracts. Based on natural conditions of tobacco planting areas, CTC has explored and developed a standardized technical system for the entire production process, including raising seedlings, tillage preparation, transplanting, field management, plant protection, fertilization, harvesting, and flue-curing, and provides standardized requirements for each key production link.
At the beginning of each year, CTC publishes 42 tobacco purchasing prices and signs planting contracts with tobacco farmers, formally agreeing on planting areas and purchase amounts. Tobacco technology extension stations are set up in various production areas, and farmers establish cooperatives (tobacco farmers are members of cooperatives) to help solve technical difficulties during the production process. However, in reality, many tobacco farmers have different adoption intensities of standardized technical systems due to subjective and objective reasons.
2.3 Data collection
In this study, a semi-structured questionnaire survey was conducted in 12 tobacco-producing districts/counties in Chongqing from March to June of 2019; 500 questionnaires were distributed. The sample size of one district/county was proportional to its tobacco production quota. Tobacco-growing villages were randomly selected for household surveys by professionals. Before the survey officially started, in February of 2019, a pre-survey was conducted in Qianjiang District, one of the main producing areas, to improve the design of the questionnaire and the survey process.
The questionnaire collected basic information about tobacco farmers, information about their families, perceptions of tobacco production technology, and adoption of the standardized technical system in 2018. The actual tobacco purchasing data in 2018 were obtained from the Chongqing Tobacco Science Research Institute affiliated with CTC; the data included sales volume, average sales price, and sales revenue of each farmer.
Referring to the work of Suri (2011), the income function is approximated by the total income function of technology adoption. Therefore, household labor was not included in the cost calculation, and the questionnaire did not collect relevant data on the production costs of tobacco farmers.
There were 384 valid questionnaires, an effective rate of 76.8%. A possible reason for this low rate is that the questionnaire data were matched with the tobacco farmer information provided by the Chongqing Tobacco Science Research Institute, and tobacco farmers with incomplete data or who did not plant tobacco in 2018 were excluded to ensure most accurate results.
2.4 Variable selection
Explanatory variables were selected based on a the theoretical analysis of existing studies of adoption intensity (Feder et al., 1985; Sharma et al., 2011; Omara et al., 2021). In addition to the head of household, household, and plot characteristics, following variables were also considered based on the actual conditions of the tobacco farmers in Chongqing:
The distance to the nearest township: this variable reflects the transaction costs of purchasing production inputs and hiring workers and affects the availability of new technologies, information, and credit institutions;
The distance to the nearest tobacco technology extension station: this variable reflects how convenient it is for tobacco farmers to obtain the technical information and technical guidance from tobacco technicians;
Loan: tobacco farmers can easily obtain bank credit support through planting contracts, and the collected information only reflects the conditions of tobacco farmers who need loans.
Satisfaction with tobacco technicians: tobacco technicians are responsible for promoting the standardized technical system and training and guiding tobacco farmers. Additionally, tobacco technicians are also responsible for supervising tobacco farmers and helping them solve difficulties in the adoption of technologies taking into account their needs. The technical service level of tobacco technicians directly affects farmers’ understanding and adoption of new technologies.
Number of technical training: considering that providing technical training to tobacco farmers by CTC and providing technical guidance to tobacco farmers by tobacco technicians are routine practices, the number of technical training in which farmers participated is used to reflect their understanding and mastery of the standardized technical system.
The proportion of leased land: tobacco farmers in Chongqing generally achieve large-scale cultivation through leased lands, and the proportion of the leased land to the total area of the tobacco farmland is used to reflect the impact of land ownership.
Perception of technology: this study determined tobacco farmers’ perception of technology based on their self-assessment of the role of technology. A five-point Likert scale was used to measure tobacco farmers’ perception of technology, i.e., 1 = it is completely useless, 2 = it is not very useful, 3 = it is useful partly, 4 = it is more useful, and 5 = it is very useful.
2.5 Research methods
In terms of factors that affect adoption intensity, existing studies generally use count data models (Isgin et al., 2008; Sharma et al., 2011), Tobit models (Arslan et al., 2014), and double-Hurdle models (Thompson et al., 2021). In terms of the impact of technology adoption on income, existing studies generally use propensity score matching (PSM) (Nakano et al., 2018) to measure the average treatment effect on the treated (ATT) and average treatment effect on the untreated (ATU). However, PSM can only correct the problem of sample selection bias caused by observable factors but it fails to explain the impact of unobservable factors (Fischer and Qaim, 2012), potentially leading to bias in estimates (Abdulai, 2016). In addition, multinomial endogenous switching regression (MESR) is applied to multi-valued processing and to explain the observable and unobservable factors that affect the allocation and outcome of the treatment (Hörne and Wollni, 2022). Yet, it cannot estimate the average effect of the treatment from one treatment level to another. Based on the method used by Gao et al. (2019) to evaluate the impact of GCT adoption intensity on the welfare of Chinese farmers, the multinomial endogenous treatment effects (METE) model proposed by Deb and Trivedi (2006b) was used to assess the impact of adoption intensity on income.
Technology adoption decisions are the result of farmers’ optimization of expected utility or revenue, where revenue is a function of factors, such as land allocation, production technology, input costs, and output prices (Feder et al., 1985). Using Tesfaye et al. (2020) and Kolady et al. (2021) as references, the random utility framework was used to analyze adoption behavior related to standardized technical systems in tobacco production. Tobacco farmers may choose to maximize their utility
The METE model has two stages: the decision equation and the outcome equation. In the first stage of the model, farmers choose one of the aforementioned three intensities. Based on Deb and Trivedi (2006a), Deb and Trivedi (2006b), let
where
where
The MMNL model in Eq. 3 is estimated by using the mlogit command of STATA 14.
The second stage evaluates the impact of different adoption intensities on outcome variables, including the natural logarithms of YPM (yield per mu), ASPT (average sales price, ASPT = total tobacco income/total tobacco yield, which indirectly reflects the tobacco quality), and IPHL (income per household labor engaged in tobacco planting). The expected outcome formula is as follows:
In this equation,
Instrumental variables are used to address the endogeneity of technology adoption decisions (Suri, 2011). Instrumental variables should affect technology adoption decisions but only through the effect of adoption on output. Based on Abdulai (2016), this study selects tobacco farmers’ attitudes toward the risks of adopting technologies as the instrumental variable. Tobacco farmers’ acceptance of new technologies is used to reflect their attitudes toward the risks of new technologies. Four types of adoption methods are described, i.e., 1 = did not adopt new technologies, 2 = adopted after others action, 3 = decided based on the result of the small area trial, and 4 = direct mass adoption. A simple falsification test was used to indicate that the selected instrumental variable is valid and relevant since it affects adoption intensity decisions (the significance of the instrumental variable in Table 1) but does not affect outcome variables (Table 2).
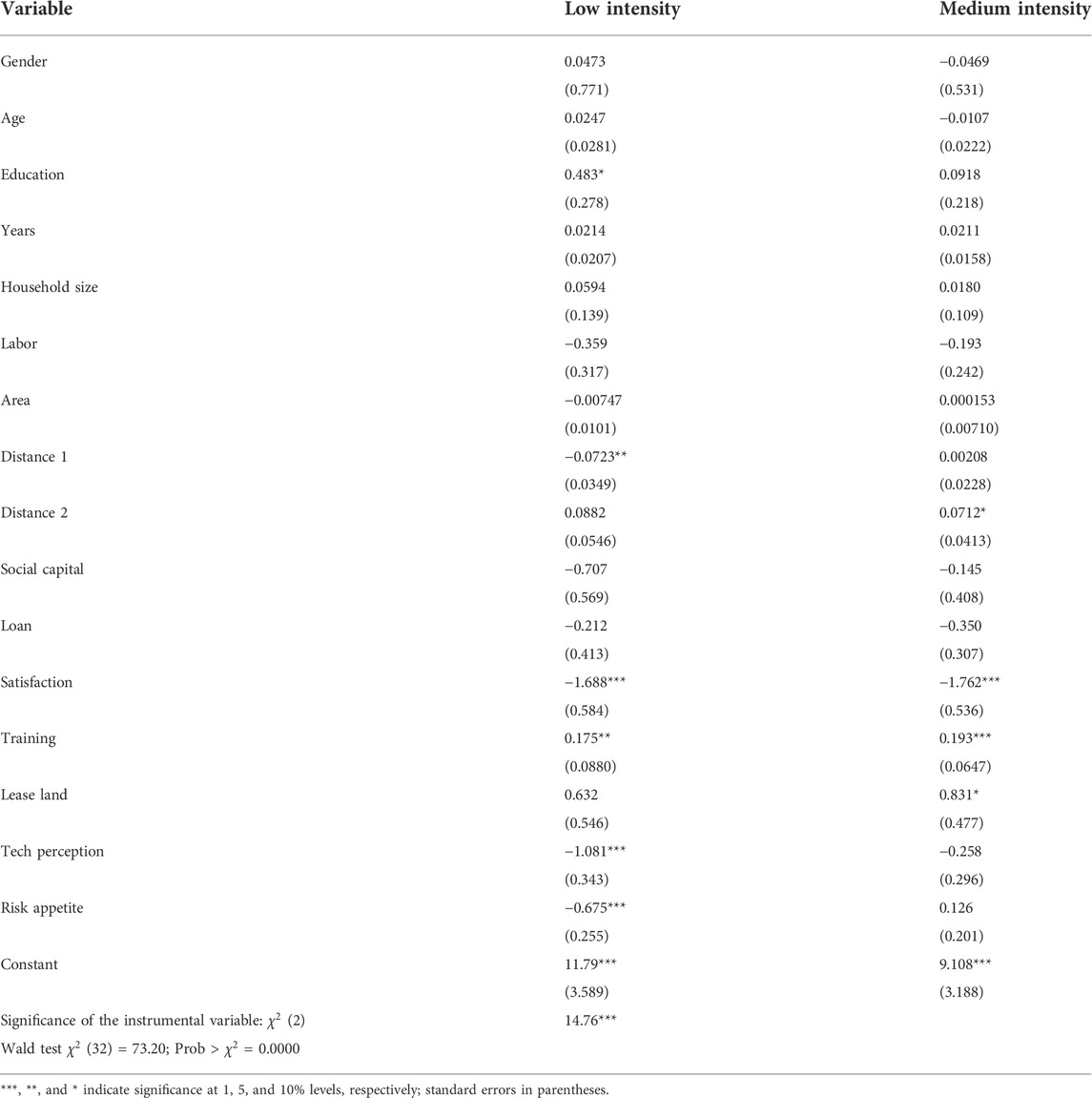
TABLE 1. MMNL model estimation of the adoption intensity of tobacco farmers regarding the standardized technical system in Chongqing, China (the baseline is high intensity).
3 Results
3.1 Characteristics of tobacco farmers’ adoption intensity of the standardized technical system
Table 3 reports the descriptive statistics of relevant variables. The adoption intensity is measured by the self-assessment of tobacco farmers on the adoption degree of the standardized technology system according to the interval of [0, 10]. Based on the distribution of survey samples, we divided the adoption intensity level into three categories: high, medium, and low. The distributions are shown in Table 4.
3.2 Determinants of different adoption intensities
Considering the characteristics of the logit model, the high intensity was used as the baseline, which is different from the study by Gao et al. (2019) because they used samples that did not adopt the technologies as the baseline. Table 1 shows the estimated parameters of the MMNL model, which is the first stage of the METE model. Wald test results indicate that the MMNL model fits well (
Empirical results show that among these factors, first, satisfaction with tobacco technicians and several technical training are statistically significant for different intensities. Second, four indicators, i.e., the farmers’ education level, perception of technology, attitude toward risk, and the distance to the nearest township, have significant effects on high and low intensity but nonsignificant effects on medium intensity. Third, the distance to the nearest tobacco technology extension station and proportion of leased land have significant positive impacts on medium intensity.
Empirical results also show that tobacco farmers’ gender and age, family social capital, the household size, the number of tobacco farming labor, the farming area, and loans are not significant.
3.3 Average treatment effect of each adoption intensity
Table 5 shows estimated impacts of different adoption intensities on YPM, ASPT, and IPHL. The results of two normal regressions (the second stage) are shown in Table 6 (due to word count restraints, the results of the mixed multinomial treatment effect regression are not shown). High intensity is still used as the baseline, and average treatment results are estimated under the assumptions of exogenous and endogenous adoption decisions.
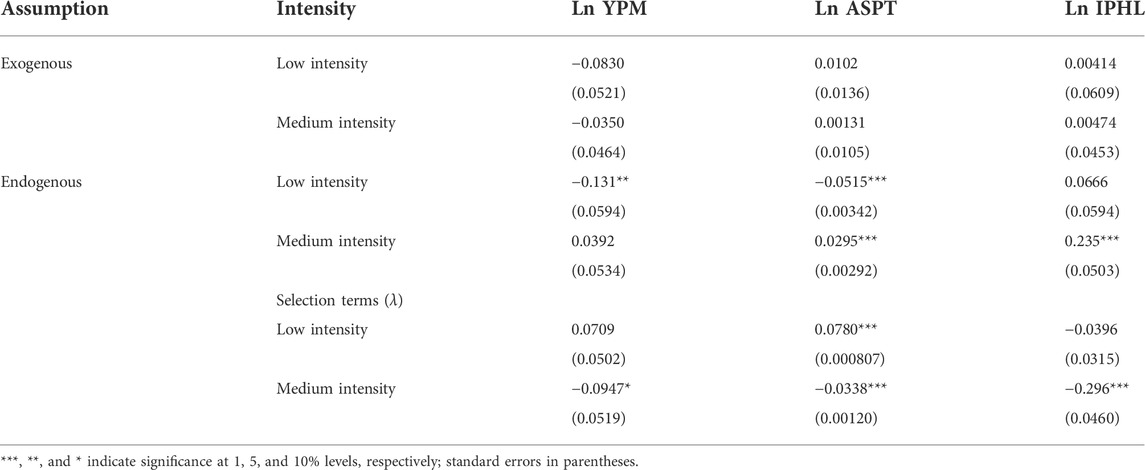
TABLE 5. METE model estimation of the impact of different adoption intensities on the tobacco yield and household income in Chongqing, China (the baseline is high intensity).
Estimation results show that under the exogenous assumption, on average, effects of low and medium intensity on YPM, ASPT, and IPHL are not significant. After considering unobserved factors and treating the endogeneity, obtained average adoption results significantly improve in terms of effectiveness and significance. Low intensity has a significant negative impact on YPM and ASPT, whereas medium intensity has a significant positive impact on ASPT and IPHL. Compared with high intensity, for low intensity, on average, YPM of tobacco farmers significantly decreased by 13.10%, ASPT significantly decreased by 5.15%, and the change in IPHL was not significant. Although the results for YPM under medium intensity were not significant, ASPT increased by 2.95% and IPHL significantly increased by 23.50%.
4 Discussion
4.1 Analysis of factors that affect adoption intensity
The empirical results show that in Chongqing, China, the factors that affect the adoption intensity of tobacco farmers include satisfaction with tobacco technicians, the number of technical training, the distance to the nearest tobacco technology extension station, the perception of technology, attitude toward risk, the distance to the nearest township, the proportion of leased land, and the education level.
The effect of tobacco technician satisfaction on high intensity is the most significant, followed by low and medium intensity, which indicates that the higher farmers’ satisfaction with technicians, the higher the adoption intensity of farmers. When farmers are highly satisfied with the service provided by tobacco technicians, they are more likely to accept and adopt the promoted standardized technical system.
The number of technical training had the most significant positive impact on medium-intensity, followed by low-intensity, and high-intensity adoption is the lowest, indicating that participation in more technical training leads to medium intensity. The results are quite different from that of Rodthong et al. (2020) and Mwaura et al. (2021). They proposed that participation in more technical training leads to high intensity. A reasonable explanation for results in this study is that with the increase in the amount of technical training, the farmers have a comprehensive and in-depth understanding of the standardized technical system and understand that the standardized technical system is constructed based on the commonality of the whole planting area. Because the standardized technical system does not fully consider the individuality of each plot, those farmers often adopt an appropriate intensity based on the characteristics of their plots rather than strictly adopting the standardized technical system based on the requirements of CTC.
Regarding distance to the nearest tobacco technology extension station, as the distance increases, farmers are more inclined to choose medium-intensity adoption of the standardized technical system. This finding is similar to that reported by Aryal et al. (2018), who found that increase in the distance to extension stations had a sustained negative impact on adoption intensity. Correspondingly, closer a technology extension station, the higher the adoption intensity of farmers because it is easier for farmers to obtain guidance from technicians. Additionally, it is easy for tobacco technicians to supervise the adoption of standardized technical systems by farmers.
Risk is an important aspect of farmers’ decision-making on the adoption of new technologies. Farmers’ opinions on risks and benefits of new technologies could affect their adoption decisions. It is generally believed that higher the risk appetite, higher the adoption intensity. The results indicate that tobacco farmers’ attitude to risk has a significant negative impact on low intensity, indicating that more open a tobacco farmer is toward risk, higher the adoption intensity.
Farmers’ perception of technology has a significant negative impact on low intensity, indicating that more farmers understand the role of technology, the higher their adoption intensity. In other words, if farmers believe that the standardized technical system is useful, adoption intensity increases. This finding is consistent with the results reported by Kabir and Rainis (2015) and Gao et al. (2017).
Regarding distance to the nearest township, there is a significant negative impact on low intensity, indicating that adoption intensity decreases with the increase in distance to the nearest township, i.e., farmers’ exhibit high intensity. This finding is contrary to results reported by Kunzekweguta et al. (2017), who found that the distance to the township had a significant negative impact on adoption intensity. The field survey in this study found that the farther tobacco farmers live from townships, fewer information sources they have; therefore, they are more inclined to strictly adopt standard technical systems. This result may be because if tobacco farmers live far from a township, they often worry about adverse effects caused by poor adoption.
The proportion of leased land reflects the situation of land ownership, and results show that the proportion of leased land has a significant positive impact on medium intensity, indicating that the higher the proportion of leased land, the more moderate of adoption intensity. This finding is different from results of previous studies. Kabir and Rainis (2015) and Mwaura et al. (2021) found that land ownership had a positive impact on adoption intensity, and Aryal et al. (2018) found that the adoption intensity of climate-smart agriculture (CSA) in plots cultivated by owners was significantly higher than that in leased plots. Because positive effects of some agricultural technologies usually have time lags (Giller et al., 2009), land tenants need to balance short-term profits and long-term investment returns. In this study, tobacco farmers with a large proportion of leased land may implement specialized production and large-scale operations, have a relatively professional understanding of standard technical systems and plots, and often do not completely adopt standardized technical systems.
In terms of education, the results of medium and low intensity are positive, but the result of medium intensity is not significant. The possible reason is that tobacco farmers in Chongqing generally have a low level of education. Also, they trust in technologies provided by CTC, so tend to adopt whole standardized technical systems.
Variables such as gender, age, social capital, household size, number of tobacco farming labor, farming area, and loans are not significant, which may be due to the following factors: 1) the implementation of contract production of tobacco and the adoption of the standardized technical system promoted by CTC is mandatory; 2) the service of tobacco technicians is universal, and there are no differences in gender, age, social capital, etc.; 3) tobacco farmers generally hire temporary workers during busy farming seasons, enabling fewer laborers to plant larger areas. The results for loans are not significant, a finding that is contrary to the prevailing view that access to credit has a significant positive effect on adoption intensity (Mensah-Bonsu et al., 2017). An explanation is that funds for tobacco farmers in Chongqing are guaranteed and that it is easy for farmers to obtain special bank loans through planting contracts.
4.2 Analysis of the impact of adoption intensity on income
Under the exogenous assumption, results for the impact of adoption intensity on income are not significant, which is a statistical inference based on observed characteristics, without considering unobserved factors; therefore, endogeneity occurs, and results are biased. Through the METE model, some unobserved factors are considered, and the validity and significance of results significantly improve.
As seen in Table 5, when high intensity is set to the baseline, the adoption loading factor (
Under the assumption of endogenous adoption decisions, compared with those under high intensity, YPM and ASPT under low intensity show significant negative effects. ASPT and IPHL under medium intensity have significant effects, indicating that low intensity leads to a decrease in the yield and quality of tobacco, whereas medium intensity facilitates significant tobacco quality improvements and income growth.
Results for effects of medium intensity on YPM are not significant. The possible reason is that the yield sold by tobacco farmers to CTC is subject to contract constraints and tobacco farmers are usually responsible to dispose of excess yield; therefore, the collected YPM is calculated based on the sales volume, which may be lower than the actual YPM. After considering this possible reason, in the case of total yield exceeding the contractual purchase volume, the effect of medium intensity on income cannot be reflected by the increase in YPM but is mainly reflected by improvements in the tobacco quality, which is indirectly reflected by the significant increase in ASPT.
Standardized technical systems designed by CTC are of great significance in improving the quality of tobacco production, ensuring the homogenization of tobacco raw materials and achieving sustainable tobacco farming. However, results of this study show that the high intensity of tobacco farmers regarding standardized technical systems does not guarantee greatest benefits. Arouna et al. (2021) obtained similar results in a study of fertilizer adoption by farmers in Nigeria.
5 Conclusion
In many developing countries, the level of farmers’ technology adoption is relatively low due to various constraints, which affect the growth of yield and income. It is generally believed that the higher adoption intensity of standardized technical systems could generate higher output under the condition of contract farming. Thus, most existing research pays attention to how to improve production efficiency by improving the intensity of technology adoption. Standardization technology only takes into account commonalities in production, but its adoption intensity should be carried out based on the heterogeneity of the plot and other factors. Too high of an intensity may lead to low input-output. Based on the micro-survey data of 384 households of tobacco farmers in Chongqing, China, this study uses a METE model to investigate factors that affect the adoption intensity of tobacco farmers regarding standardized technical systems and analyzes the impact of intensity differences on households’ income.
These results show that various factors affect adoption intensity. Specifically, there is higher adoption intensity with farther distances to the nearest township. The closer the distance to the tobacco technology extension stations, the higher the farmers’ satisfaction with tobacco technicians, and the higher the perception of technology, the more open farmers are toward risks of adopted technologies. There is more medium adoption intensity with farther distances from a farmer’s residence to a tobacco technology extension station as well as with a higher proportion of leased land. It is found that greater the number of technical training, the more medium intensity is adopted.
The results also show that the adoption intensity has a significant heterogeneous effect on output. Different from current empirical conclusion, this study finds that a high adoption intensity does not guarantee the greatest benefits. The empirical results of the METE model show that estimation bias is generated without considering unobservable factors, which could affect the effectiveness of the estimation. After treating the endogeneity, YPM and ASPT under low adoption intensity have significant negative effects, whereas ASPT and IPHL under medium adoption intensity have significant positive effects. This indicates that compared with those under high adoption intensity, the tobacco yield and quality under low adoption intensity decrease, whereas medium adoption intensity results in significant tobacco quality improvements and income growth.
Contract farming is an effective way to improve farmers’ technology adoption levels. Our findings provide important insights for policy recommendations. First, it is by the generality of production that the company makes the standardized technical system, which is difficult when taking into account the differences in resource endowments spatially. As such, farmers need to select the appropriate adoption intensity according to characteristics of the focal plot to improve the input–output efficiency. Second, it is challenging for farmers to determine the adoption intensity in isolation —— the company needs to help farmers make appropriate decisions. The results of this study also revealed that the input of pesticides, chemical fertilizers, herbicides, and other chemical substances should be appropriately reduced in Chongqing, which not only reduces unnecessary input of factors of production but is also beneficial to the protection of the environment and the health of farmers. Third, although most tobacco farmers take medium or high adoption intensity, there are about 16% of tobacco farmers that utilize a lower adoption intensity. CTC needs to take positive steps to promote these tobacco farmers to increase the level of adoption intensity by enhancing their perception of technology.
In future studies, combined with the evaluation of tobacco farmers’ self-adoption, a more effective technology adoption intensity measurement system should be established. Farmers’ input–output can be further empirically analyzed to help tobacco farmers choose the appropriate adoption intensity.
Data availability statement
The raw data supporting the conclusion of this article will be made available by the authors, without undue reservation.
Ethics statement
Ethics review and approval/written informed consent were not required as per local legislation and institutional requirements.
Author contributions
Conceptualization, YL and BL; methodology, XY and BL; validation, YL and YH; investigation, YL; data curation, ZZ; statistical analysis, YL and ZZ; writing—original draft preparation, YL and YH; writing—review and editing, XY and BL; supervision, XY and BL; and project administration, XY and BL.
Funding
The research was supported by the Fundamental Research Funds for the Central Universities (Grant No. 2019CDJSK02XK06) and the Humanities and Social Science Fund of the Ministry of Education of China (Grant No. 21YJA790024).
Conflict of interest
The authors declare that the research was conducted in the absence of any commercial or financial relationships that could be construed as a potential conflict of interest.
Publisher’s note
All claims expressed in this article are solely those of the authors and do not necessarily represent those of their affiliated organizations, or those of the publisher, the editors, and the reviewers. Any product that may be evaluated in this article, or claim that may be made by its manufacturer, is not guaranteed or endorsed by the publisher.
References
Abdulai, A. N. (2016). Impact of conservation agriculture technology on household welfare in Zambia. Agric. Econ. 47 (6), 729–741. doi:10.1111/agec.12269
Ainembabazi, J. H., Abdoulaye, T., Feleke, S., Alene, A., Dontsop-Nguezet, P. M., Ndayisaba, P. C., et al. (2018). Who benefits from which agricultural research-for-development technologies? Evidence from farm household poverty analysis in Central Africa. World Dev. 108, 28–46. doi:10.1016/j.worlddev.2018.03.013
Appau, A., Drope, J., Goma, F., Magati, P., Labonte, R., Makoka, D., et al. (2020). Explaining why farmers grow tobacco: Evidence from Malawi, Kenya, and Zambia. Nicotine Tob. Res. 22 (12), 2238–2245. doi:10.1093/ntr/ntz173
Appau, A., Drope, J., Witoelar, F., Chavez, J. J., and Lencucha, R. (2019). Why do farmers grow tobacco? A qualitative exploration of farmers perspectives in Indonesia and Philippines. Int. J. Environ. Res. Public Health 16 (13), 2330. doi:10.3390/ijerph16132330
Arouna, A., Michler, J. D., Yergo, W., and Saito, K. (2021). One size fits all? Experimental evidence on the digital delivery of personalized extension advice in Nigeria. Am. J. Agric. Econ. 103 (2), 596–619. doi:10.1111/ajae.12151
Arslan, A., McCarthy, N., Lipper, L., Asfaw, S., and Cattaneo, A. (2014). Adoption and intensity of adoption of conservation farming practices in Zambia. Agric. Ecosyst. Environ. 187, 72–86. doi:10.1016/j.agee.2013.08.017
Aryal, J. P., Rahut, D. B., Maharjan, S., and Erenstein, O. (2018). Factors affecting the adoption of multiple climate-smart agricultural practices in the Indo-Gangetic Plains of India. Nat. Resour. Forum 42 (3), 141–158. doi:10.1111/1477-8947.12152
Brown, B., Nuberg, I., and Llewellyn, R. (2017). Stepwise frameworks for understanding the utilisation of conservation agriculture in Africa. Agric. Syst. 153, 11–22. doi:10.1016/j.agsy.2017.01.012
Chen, S., Qi, G. F., Luo, T., Zhang, H. C., Jiang, Q. K., Wang, R., et al. (2018). Continuous-cropping tobacco caused variance of chemical properties and structure of bacterial network in soils. Land Degrad. Dev. 29 (11), 4106–4120. doi:10.1002/ldr.3167
Deb, P., and Trivedi, P. K. (2006a). Maximum simulated likelihood estimation of a negative binomial regression model with multinomial endogenous treatment. Stata J. 6 (2), 246–255. doi:10.1177/1536867X0600600206
Deb, P., and Trivedi, P. K. (2006b). Specification and simulated likelihood estimation of a non-normal treatment-outcome model with selection: Application to health care utilization. Econom. J. 9 (2), 307–331. doi:10.1111/j.1368-423X.2006.00187.x
Dimara, E., and Skuras, D. (1998). Adoption of new tobacco varieties in Greece: Impacts of empirical findings on policy design. Agric. Econ. 19 (3), 297–307. doi:10.1111/j.1574-0862.1998.tb00533.x
Feder, G., Just, R. E., and Zilberman, D. (1985). Adoption of agricultural innovations in developing countries: A survey. Econ. Dev. Cult. Change 33 (2), 255–298. doi:10.1086/451461
Fischer, E., and Qaim, M. (2012). Linking smallholders to markets: Determinants and impacts of farmer collective action in Kenya. World Dev. 40 (6), 1255–1268. doi:10.1016/j.worlddev.2011.11.018
Foster, A. D., and Rosenzweig, M. R. (2010). Microeconomics of technology adoption. Annu. Rev. Econ. 2 (1), 395–424. doi:10.1146/annurev.economics.102308.124433
Gao, Y., Niu, Z. H., Yang, H. R., and Yu, L. L. (2019). Impact of green control techniques on family farms’ welfare. Ecol. Econ. 161, 91–99. doi:10.1016/j.ecolecon.2019.03.015
Gao, Y., Zhang, X., Wu, L., Yin, S., and Lu, J. (2017). Resource basis, ecosystem and growth of grain family farm in China: Based on rough set theory and hierarchical linear model. Agric. Syst. 154, 157–167. doi:10.1016/j.agsy.2017.03.013
Giller, K. E., Witter, E., Corbeels, M., and Tittonell, P. (2009). Conservation agriculture and smallholder farming in Africa: The heretics' view. Field Crops Res. 114 (1), 23–34. doi:10.1016/j.fcr.2009.06.017
Hörne, D., and Wollni, M. (2021). Integrated soil fertility management and household welfare in Ethiopia. Food Policy 100, 102022. doi:10.1016/j.foodpol.2020.102022
Hörner, D., and Wollni, M. (2022). Does Integrated Soil Fertility Management increase returns to land and labor?. Agric. Econ. 53 (3), 337–355. doi:10.1111/agec.12699
Isgin, T., Bilgic, A., Forster, D. L., and Batte, M. T. (2008). Using count data models to determine the factors affecting farmers’ quantity decisions of precision farming technology adoption. Comput. Electron. Agric. 62 (2), 231–242. doi:10.1016/j.compag.2008.01.004
Kabir, M. H., and Rainis, R. (2015). Adoption and intensity of integrated pest management (IPM) vegetable farming in Bangladesh: An approach to sustainable agricultural development. Environ. Dev. Sustain. 17 (6), 1413–1429. doi:10.1007/s10668-014-9613-y
Kolady, D. E., Van der Sluis, E., Uddin, M. M., and Deutz, A. P. (2021). Determinants of adoption and adoption intensity of precision agriculture technologies: Evidence from South Dakota. Precis. Agric. 22, 689–710. doi:10.1007/s11119-020-09750-2
Kuijpers, R., and Swinnen, J. (2016). Value chains and technology transfer to agriculture in developing and emerging economies. Am. J. Agric. Econ. 98 (5), 1403–1418. doi:10.1093/ajae/aaw069
Kunzekweguta, M., Rich, K. M., and Lyne, M. C. (2017). Factors affecting adoption and intensity of conservation agriculture techniques applied by smallholders in Masvingo district, Zimbabwe. Agrekon 56 (4), 330–346. doi:10.1080/03031853.2017.1371616
Li, W., Ruiz-Menjivar, J., Zhang, L., and Zhang, J. (2021). Climate change perceptions and the adoption of low-carbon agricultural technologies: Evidence from rice production systems in the Yangtze River Basin. Sci. Total Environ. 759, 143554. doi:10.1016/j.scitotenv.2020.143554
Ma, W., and Wang, X. (2020). Internet use, sustainable agricultural practices and rural incomes: Evidence from China. Aust. J. Agric. Resour. Econ. 64 (4), 1087–1112. doi:10.1111/1467-8489.12390
Manda, J., Alene, A. D., Tufa, A. H., Abdoulaye, T., Kamara, A. Y., Olufajo, O., et al. (2020). Adoption and ex-post impacts of improved cowpea varieties on productivity and net returns in Nigeria. J. Agric. Econ. 71 (1), 165–183. doi:10.1111/1477-9552.12331
Mao, H., Zhou, L., Ifft, J., and Ying, R. Y. (2019). Risk preferences, production contracts and technology adoption by broiler farmers in China. China Econ. Rev. 54 (4), 147–159. doi:10.1016/j.chieco.2018.10.014
Mensah-Bonsu, A., Sarpong, D. B., Al-Hassan, R., Asuming-Brempong, S., Egyir, I. S., Kuwornu, J. K. M., et al. (2017). Intensity of and factors affecting land and water management practices among smallholder maize farmers in Ghana. Afr. J. Agric. Resour. E. 12 (2), 142–157.
Mwaura, G. G., Kiboi, M. N., Bett, E. K., Mugwe, J. N., Ngetich, F. K., Muriuki, A., et al. (2021). Adoption intensity of selected organic-based soil fertility management technologies in the central highlands of Kenya. Front. Sustain. Food Syst. 4, 570190. doi:10.3389/fsufs.2020.570190
Nakano, Y., Tanaka, Y., and Otsuka, K. (2018). Impact of training on the intensification of rice farming: Evidence from rainfed areas in Tanzania. Agric. Econ. 49 (2), 193–202. doi:10.1111/agec.12408
Noltze, M., Schwarze, S., and Qaim, M. (2012). Understanding the adoption of system technologies in smallholder agriculture: The system of rice intensification (SRI) in Timor Leste. Agric. Syst. 108, 64–73. doi:10.1016/j.agsy.2012.01.003
Omara, H., Odongo, W., and Kule, E. K. (2021). Adoption of environmentally friendly agricultural technologies among smallholder farmers: The case of rocket barn technology in flue-cured tobacco curing in Uganda. Land Degrad. Dev. 32, 965–974. doi:10.1002/ldr.3765
Pannell, D. J., Llewellyn, R. S., and Corbeels, M. (2014). The farm-level economics of conservation agriculture for resource-poor farmers. Agric. Ecosyst. Environ. 187, 52–64. doi:10.1016/j.agee.2013.10.014
Reichert, J. M., Pellegrini, A., Rodrigues, M. F., Tiecher, T., and dos Santos, D. R. (2019). Impact of tobacco management practices on soil, water and nutrients losses in steeplands with shallow soil. Catena 183, 104215. doi:10.1016/j.catena.2019.104215
Rodthong, W., Kuwornu, J. K. M., Datta, A., Anal, A. K., and Tsusaka, T. W. (2020). Factors influencing the intensity of adoption of the roundtable on sustainable palm oil practices by smallholder farmers in Thailand. Environ. Manage. 66 (3), 377–394. doi:10.1007/s00267-020-01323-3
Scoones, I., Mavedzenge, B., Murimbarimba, F., and Sukume, C. (2018). Tobacco, contract farming, and agrarian change in Zimbabwe. J. Agrar. Change 18 (1), 22–42. doi:10.1111/joac.12210
Sharma, A., Bailey, A., and Fraser, I. (2011). Technology adoption and pest control strategies among UK cereal farmers: Evidence from parametric and non-parametric count data models. J. Agric. Econ. 62 (1), 73–92. doi:10.1111/j.1477-9552.2010.00272.x
Shonhe, T., and Scoones, I. (2022). Private and state-led contract farming in Zimbabwe: Accumulation, social differentiation and rural politics. J. Agrar. Change 22 (1), 118–138. doi:10.1111/joac.12473
Suri, T. (2011). Selection and comparative advantage in technology adoption. Econometrica 79 (1), 159–209. doi:10.3982/ECTA7749
Talukder, A., Haq, I., Ali, M., and Drope, J. (2020). Factors associated with cultivation of tobacco in Bangladesh: A multilevel modelling approach. Int. J. Environ. Res. Public Health 17, 4277. doi:10.3390/ijerph17124277
Tesfaye, W., Blalock, G., and Tirivayi, N. (2020). Climate‐smart innovations and rural poverty in Ethiopia: Exploring impacts and pathways. Am. J. Agric. Econ. 103 (3), 878–899. doi:10.1111/ajae.12161
Thompson, N. M., Reeling, C. J., Fleckenstein, M. R., Prokopy, L. S., and Armstrong, S. D. (2021). Examining intensity of conservation practice adoption: Evidence from cover crop use on U.S. Midwest farms. Food Policy 101, 102054. doi:10.1016/j.foodpol.2021.102054
Wang, H. H., Wang, Y., and Delgado, M. S. (2014). The transition to modern agriculture: Contract farming in developing economies. Am. J. Agric. Econ. 96 (5), 1257–1271. doi:10.1093/ajae/aau036
Wouter, Z. (2020). If smallholder farmers have access to the world market: The case of tobacco marketing in Malawi. Eur. Rev. Agric. Econ. 47 (4), 1402–1437. doi:10.1093/erae/jbz039
Keywords: the standardized technical system, adoption intensity, income, multinomial endogenous treatment effects, contract farming
Citation: Li Y, Hua Y, Liao B, Yuan X and Zhou Z (2022) Adoption intensity of a standardized technical system: The higher the better?—Evidence from China. Front. Environ. Sci. 10:937287. doi: 10.3389/fenvs.2022.937287
Received: 06 May 2022; Accepted: 21 July 2022;
Published: 29 August 2022.
Edited by:
Cem Işık, Anadolu University, TurkeyCopyright © 2022 Li, Hua, Liao, Yuan and Zhou. This is an open-access article distributed under the terms of the Creative Commons Attribution License (CC BY). The use, distribution or reproduction in other forums is permitted, provided the original author(s) and the copyright owner(s) are credited and that the original publication in this journal is cited, in accordance with accepted academic practice. No use, distribution or reproduction is permitted which does not comply with these terms.
*Correspondence: Bing Liao, bGlhb2JpbmdAY3F1LmVkdS5jbg==; Xiaohao Yuan, eXVhbnhpYW9oYW9AY3F1LmVkdS5jbg==