- 1Shaanxi Key Laboratory of Earth Surface System and Environmental Carrying Capacity, College of Urban and Environmental Sciences, Northwest University, Xi’an, China
- 2Institute of Qinling Mountains, Northwest University, Xi’an, China
- 3State Key Laboratory of Water Resources and Hydropower Engineering Science, Wuhan University, Wuhan, China
- 4Key Laboratory of Water Cycle and Related Land Surface Processes, Institute of Geographic Sciences and Natural Resources Research, Chinese Academy of Sciences, Beijing, China
As significant players in material cycling and energy flow, bacteria and eukaryotes play a vital role in the ecosystem. Nevertheless, the community dynamics of bacteria and eukaryotes in rivers and their responses to changes in ecological hydrology have not been studied thoroughly. Based on eDNA technology, this study investigated the bacterial and eukaryotic communities in the upper, middle and lower reaches of the Weihe River in different seasons. The seasonal variation and geographical distribution of bacterial and eukaryotic community structures showed significant heterogeneity. The selective theory well explained the response of microbial community assembly to seasonal changes. Deterministic processes dominate microbial community assembly in the middle and lower reaches. The composition and metabolic potential of key functional genes of nitrogen and phosphorus cycling (nosZ, pqqB, pqqD, and pqqE) exhibited strong seasonal patterns and were significantly correlated with the physical and chemical properties of water. There were significant differences in molecular ecological networks in different periods (p < 0.05), with a gradually increasing trend in the complexity of the network from winter to summer. The keystone species (Hub) of the microbial food web in each season included microorganisms (Malikia), algae (Stephanodiscus), and invertebrates (Polyarthra). Structural equation modeling (SEM) results indicated that invertebrate was an important driving factor affecting the changes in community structures. In micro-food webs, both “bottom-up” (resources) and “top-down” (predation) forces strictly controlled the relationship between taxa. Nitrogen and phosphorus concentrations affected microbial networks, and there was a significant correlation between bacterial and eukaryotic groups and eco-hydrological variables (p < 0.05). Furthermore, we identified the taxon’s change point using threshold indicator taxa analysis (TITAN), quantitatively revealing the response thresholds of taxa to eco-hydrological changes.
Introduction
Climate change and human activities significantly reduce the river’s resilience to disturbance, and aquatic biodiversity controls the basic ecological processes and functions of the river (Haase et al., 2018). As major players in the material cycle and energy flow, bacteria and eukaryotic communities are crucial in driving ecosystem processes (Wang et al., 2017). Dynamic changes in microbial communities exert direct and profound impacts on the ecological integrity of freshwater ecosystems (Tang et al., 2020). Such changes are deemed essential to understanding the rapid changes in the structure and function of aquatic ecosystems (Niu et al., 2018). Researchers have revealed how human activity affects microbes’ functional changes and metabolic potential by describing the spatial distribution patterns of microbial and eukaryotic, including fluctuations in diversity, dispersal patterns, and levels of species interactions in different environments (Webster et al., 2018). Related studies showed a close correlation between bacteria and eukaryotic community composition (Logares et al., 2018). Their diversity was influenced by land-use practices, environmental factors, hydrological properties, and geographic distance between sampling sites (Xie et al., 2017). However, challenges remain in studying the interactions of a wide range of bacterial and eukaryotic taxa across different trophic levels in rivers with strong anthropogenic impacts (Hu et al., 2022).
Environmental DNA (eDNA) can extract the community composition information from the samples by expanding the target DNA fragments, suitable for environments with rich biodiversity, such as rivers (Suter et al., 2021). eDNA technology can simultaneously investigate organisms at multiple trophic levels, providing critical information about the complex biological interactions associated with ecosystem changes (Chen et al., 2021). The eDNA technology has allowed for accurate monitoring of organisms, effectively improving the timeliness of biological evaluation and validating the potential of the technology to assess the status of aquatic ecosystems (Bagley et al., 2019). The 16S rRNA and 18S rRNA genes have high species coverage and relatively complete sequence databases (Walters et al., 2016; Xu et al., 2020; Sun et al., 2021). These gene markers have been widely applied to monitor the species composition and community structure of marine and freshwater bacteria and eukaryotes (Harper et al., 2019). In addition, some researchers have studied the seasonal changes and spatial composition of eukaryotic phytoplankton diversity based on the 18SrRNA gene and further revealed the impact of environmental variables on phytoplankton functional groups and community composition (Yang et al., 2021a).
However, these studies may fail to accurately describe the response of aquatic communities to environmental changes. To explore the mostly non-linear relationships between stress factors and biological parameters, ecologists have proposed non-linear thresholds analysis methods, such as nonparametric change-point analysis (nCPA), threshold indicator taxa analysis (TITAN), and random forest (Sultana et al., 2019; Triadó-Margarit et al., 2019). These non-linear methods show higher accuracy in building models to resolve biological-environmental data relationships than the linear models. Especially, TITAN can detect the critical point where the distribution law of each community’s characteristic indicators changes along an environmental gradient (Martins et al., 2021). Further, it can assess the synchrony of the crucial issue of different community characteristic indicators and determine the critical point where the whole community responds to environmental factors. Recently, the application of TITAN to identify thresholds with habitat changes and habitat conditions improved the ability to balance resource conservation and development while identifying sensitive indicators (Xuan et al., 2019).
Revealing the dynamic evolution mechanism of communities at different trophic levels is of great scientific significance and practical urgency to guarantee water safety and ecological security in river basins. At present, the ecological environment of the Yellow River basin is fragile, the water security situation is complex, and the quality of development needs to be improved (Yang et al., 2021b). As the largest tributary of the Yellow River, the Weihe River also faces problems such as a sharp decline in biodiversity, an increase in hydrological rate, and severe water pollution (Zhao et al., 2020). However, existing studies in the Yellow River Basin have only focused either on revealing individual organismal groups or single functions, seriously ignoring the in-depth understanding of the structures and functions of the multi-trophic communities in the river under changing environments (Wang et al., 2019).
In addition, they have yet to quantify the response relationship between aquatic communities and eco-hydrological changes (Su et al., 2019). Therefore, based on eDNA metabarcoding technology, we explored the dynamic evolution mechanism of biodiversity from bacteria to invertebrates. This study aims to 1) characterize the spatiotemporal changes in the structure and function of bacterial and eukaryotic communities in Weihe River, 2) elucidate the coexistence phenomenon and potential interactions between coexisting taxa at different trophic levels, and 3) quantitatively analyze the threshold of biocommunity response to eco-hydrological variables.
Materials and Methods
Study Area
The Weihe River basin covers an area of 134,766 km2 with complex geomorphological features, spanning three different landform units: the Loess Plateau, the Guanzhong Basin, and the Qinling Mountains (Song et al., 2018). The annual rainfall of the Weihe River is between 350 and 700 mm, concentrated in summer, with an apparent seasonal difference (Song et al., 2021). In recent years, due to the rapid development of the Guanzhong urban agglomeration, the water pollution in the Weihe River Basin has intensified, the biodiversity declining sharply, and the ecological function of the river deteriorating (Liang et al., 2022).
Site Description and Sample Collection
Through field investigation, based on comprehensively considering the impact of flow direction, geomorphology, and human activities, 12 sampling sections were set up in consideration of the national hydrological and water quality monitoring stations (Figure 1). Samples were collected in winter (January), spring (April), summer (August), and autumn (November) between January 2018 and November 2019, with sampling sections covering the Weihe River’s upper, middle and lower reaches (104.74′E - 110.10′E, 35.44′N-34.38′N). The specific sampling sections were located in Weiyuan (WY), Wushan (WS), Tianshui (TS), Chencang (CC), Weibin (WB), Lijiacun (LJC), Meixian (MX), Xianyang (XY), Gaoling (GL), Lintong (LT), Huaxian (HX) and Huayin (HY).
When sampling bacteria and eukaryotes, the operation person wore masks and disposable medical gloves to collect 2,000 ml of water samples from each water layer of the monitoring sections and cryopreserved them until transported to the laboratory (Bahram et al., 2021). Under sterile conditions, a 0.45 μm micropore filter was applied to filter eukaryotes, and a membrane filter with 0.22 μm pore size to filter microorganisms from water samples (Chi et al., 2021). The filter membranes were placed in sterile centrifuge tubes and stored in a -80 °C cryogenic freezer for the biome sequencing analysis.
Water Quality and Hydrology
A portable multifunctional water quality analyzer (HACH HQ40days) was used to measure water temperature (WT), pH, and dissolved oxygen (DO) on site (Wu et al., 2019). Water quality variables, i.e., total phosphorus (TP), total nitrogen (TN), and ammonia nitrogen (NH4+-N), were measured by spectrophotometry (State Environmental Protection Administration, 2002). Flow velocity (V), river discharge (Q), and water level (D) data were obtained by using a portable flow meter (MGG/KL-DCB). The sections located in the national hydrological and water quality monitoring stations Beidao (BD), Xianyang (XY), and Huaxian (HX) hydrological stations) adopt the real-time monitoring data of the national monitoring station.
DNA Extraction and Sequencing
According to the instruction, DNA was extracted via the Fast DNA SPIN extraction kit (MP Biomedicals, Santa Ana, CA, United States), and the samples were stored at -80°C for further analysis. The quantity and quality of the extracted DNA were measured by using a NanoDrop ND-1000 spectrophotometer (Thermo Fisher Scientific, Waltham, MA, United States) and agarose gel electrophoresis, respectively. A detailed description of the amplification protocol has been published (Valentini et al., 2016). This present study performed samples for two-end sequencing on the Illumina MiSeq PE250 platform (Illumina, San Diego, CA).
Among them, the amplification target of the microbes was the V3-V4 region of 16S rRNA. The sequence of the primers for PCR amplification were (338F, ACTCCTACGGGAGGCAGCA) and (806R, GGACTACHVGGGTWTCTAAT) (Walters et al., 2016); the amplification of eukaryotes targets was the V4 region of 18S rRNA, the sequence of the primers for PCR amplification was (547F, CCAGCASCYGCGGTAATTCC) and (V4R, ACTTTCGTTCTTGATYRA) (Stoeck et al., 2018); The COI primer pairs were mICOIintF 5′-GGWACWGGWTGAACWGTWTAYCCYCC-3′ and jgHCO 5′-TAAACTTCAGGGTGACCAAARAAYCA-3’ (Leray et al., 2013). Species were annotated by comparing the 18S rRNA gene sequences to the NCBI Ge-nbank reference database; 16S rRNA gene sequences were aligned to the Silva reference database to annotate the species.
Bioinformatics and Statistical Analysis
After sequencing, the species composition, diversity index, and principal coordinates analysis (PCoA) of the microorganisms were performed using the R (version 3.5.2) (https://www.r-project.org/) “vegan” and the “ggplot2” package, QIIME (v1.8.0) software, and the mothur software. Network analysis of the bacterial and eukaryotic communities was performed using the “Hmisc” and “igraph” packages. In order to reduce false-positive prediction and increase the sensitivity of the network, we used samples with a proportion above 0.01% for network analysis (Liu et al., 2022). To better understand the potential functional and metabolic pathways of specific biological taxa in water bodies, we used PICRUSt version 2.0.0 to analyze the functional content of the 16S rRNA and 18S rRNA marker genes. Compared to its original version, the reference genome database of PICRUSt 2.0.0 was more than 10-fold expanded, and its prediction results are more rigorous and accurate (Douglas et al., 2020). To analyze the direct and indirect effects of nitrogen and phosphorus nutrient changes on multi-trophic communities, we used a Structural equation modeling (SEM) to determine the direct and indirect paths among environmental change and trophic transfer efficiencies (Li et al., 2020; Ma et al., 2020). The SEM model was constructed using Amos (version 18.0, IBM, United States).
Identification of Threshold Responses and Indicator Taxa
Finally, TITAN was applied to determine the ecological protection thresholds of eco-hydrological indicators for species composition changes in Weihe River (Simonin et al., 2019). Before data analysis, the top 50 species samples (genus level) were selected to identify the best change point by TITAN to obtain maximum indication merit (IndVal). All species were negative response taxa (z-) and positive response taxa (z+) based on the relative abundance and frequency on both sides of the change points (Berger et al., 2018). After defining the indicator species, TITAN provides a community-level threshold reflecting the size of community change as a point of coincident change indicator of the community structure [sum (z)], indicating the presence of community thresholds if there were significant synchronous changes in the distribution of taxa (Valente-Neto et al., 2021). In addition, bootstrap technology was employed to measure the uncertainty, purity, and reliability. The thresholds and the reliability of the indicated species in various group change points were validated by the measured uncertainty (p < 0.05), purity (≥0.95), and reliability (≥0.95).
Results
Eco-Hydrological Variables
There were significant seasonal changes in the water environment and hydrological monitoring indicators (p < 0.05) (Table 1). As one of the primary pollutants in the Weihe River, the average concentration of TN was higher than the Class V standard limit stated in the Environmental Quality Standards for Surface Water (GB3838-2002) in all seasons. The mean flow, velocity, and water depth values were significantly higher than those in the other seasons (p < 0.05). The mean autumn and winter temperatures were much lower than those in the other seasons, while the mean autumn and winter DO and NH4+-N were higher than those in other seasons.
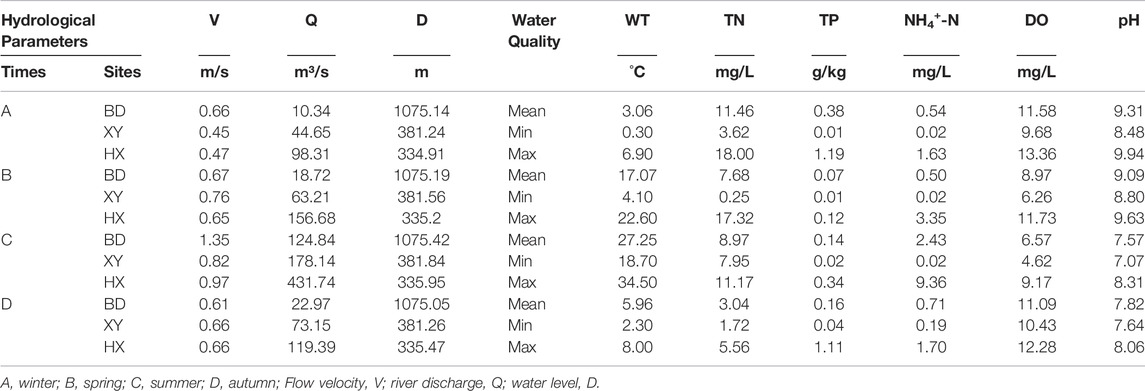
TABLE 1. Physical and chemical properties and characteristics of hydrological variables in different seasons.
Spatial Patterns and Seasonal Changes in Bacterial and Eukaryotic Communities
A total of 2,233,453 high-quality sequences and 1,616,821 high-quality sequences were retained from the 12 monitoring sections at four seasons. The average number of sequences of the 16S rRNA genes was 46,530, ranging from 32,526 to 73,569, generating a total of 74,365 ASVs. The average number of sequences of the 18S rRNA genes was 34,400, ranging from 23,192 to 49,166, generating a total of 6,680 OTUs. Major taxa in the bacterial communities were Proteobacteria, Bacteroides, Actinobacteria, Cyanobacteria, Firmicutes, Epsilonbacteraeota, Verrucomicrobia, Patescibacteria, Chloroflexi, and Acidobacteria. Meanwhile, the sequence amount of Proteobacteria was 49.94%, significantly higher than the other taxa, indicating that it was the dominant taxa. Eukaryotic communities mainly included phytoplankton algae, fungi, protozoa, invertebrate metazoans, anneldans, molluscs, etc. Algae had the most significant number of sequences and ASVs, followed by fungi and fewer protozoa and invertebrate metazoans. The relative abundance of Bacillariophyta, Ciliophora, Chlorophyta, Cryptophyta, Ascomycota, Pyrrophyta, and Rotifera was relatively high, of which Proteobacteria (29.58%) was the dominant group. Seasonal variation and geographic distribution of the bacterial and eukaryotic communities showed notable heterogeneity (p < 0.05; Figure 2). The relative abundance of Proteobacteria was higher in the middle stream and downstream than upstream during autumn and winter. The relative abundance of Proteobacteria did not vary significantly between upstream and downstream during spring and summer. The relative abundance of Bacillariophyta was low during the spring and autumn and showed a decreasing trend from upstream to downstream.
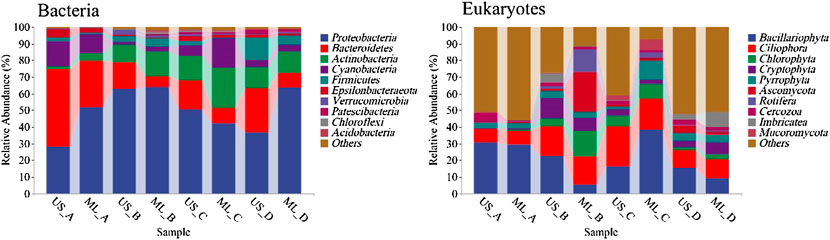
FIGURE 2. Relative abundance of bacterial and eukaryotic dominant taxa (phylum level; (A), winter; (B), spring; (C), summer; (D), autumn; US, upstream monitoring sections; ML, middle and downstream monitoring sections).
The diversity of bacterial and eukaryotic communities showed significant seasonal changes (p < 0.05; Figure 3). The PCoA analysis results showed notable differences between the bacterial and eukaryotic communities (p < 0.05; Figure 4). The dispersion degree between bacterial samples was highest in spring, while for eukaryotic community samples, it was higher in spring and summer than in autumn and winter.
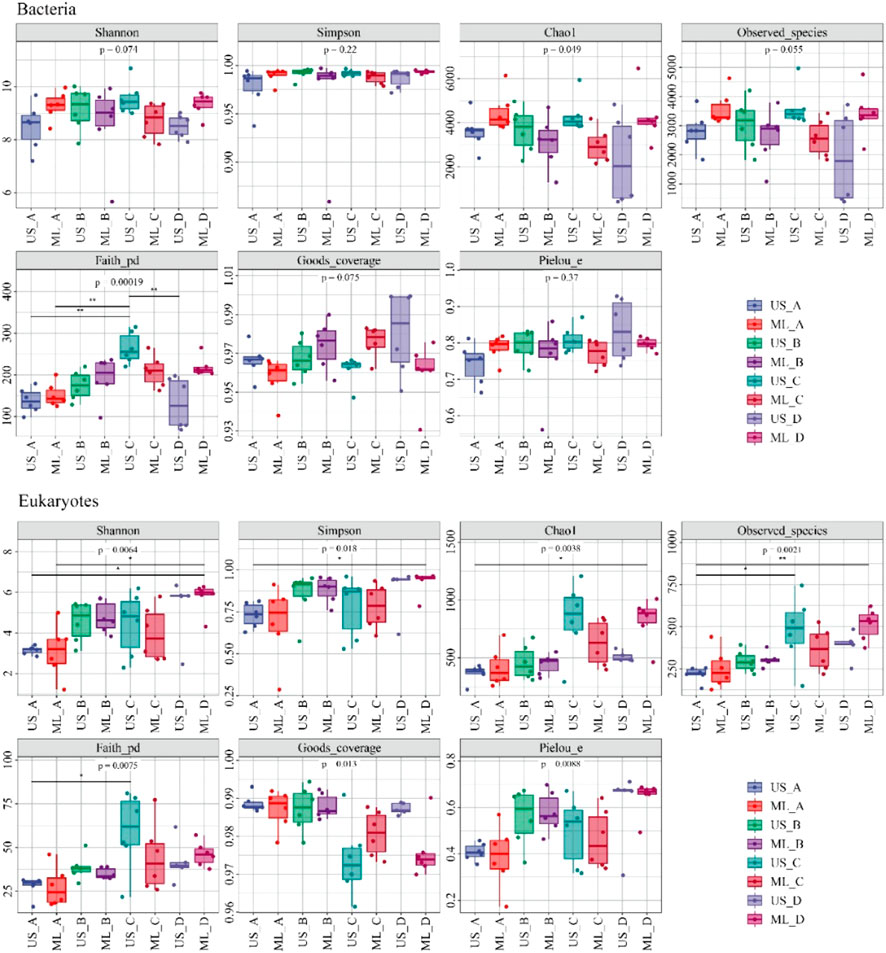
FIGURE 3. The diversity indices of the bacterial and eukaryotic communities (A), winter; (B), spring; (C), summer; (D), autumn; US, upstream monitoring sections; ML, middle and downstream monitoring sections).
Spatio-Temporal Changes of Bacterial and Eukaryotic Community Function
Microbial genes contain a large number of pollutant degradation functions and nitrogen and phosphorus metabolism functions, and the related nitrogen and phosphorus cycle function genes show strong seasonal patterns (Smith et al., 2019). Among the 36 functional genes related to the nitrogen cycle, 11 of them showed a relative abundance that was significantly affected by seasonal changes (p < 0.01; Figure 5). The relative abundance of nosZ and NORC genes used to characterize denitrification, hdh gene used to describe anammox, and napC gene used to represent dissimilatory nitrogen reduction were relatively high, showing the seasonal variation characteristics of the lowest in winter and higher in other seasons. Moreover, seasonal variation significantly affected the relative abundance of 15 among the 52 phosphorus cycle-related functional genes (p < 0.01). These 15 genes were mainly distributed in three functional groups, phosphonate mineralization, inorganic phosphate solubilization, and phosphonate transport, mainly including phnF、phnG、phnI、phnJ、phnL、phnM、phnN、phnK、gcd、glpT、glpP、pqqC、pqqB、pqqD and pqqE genes.
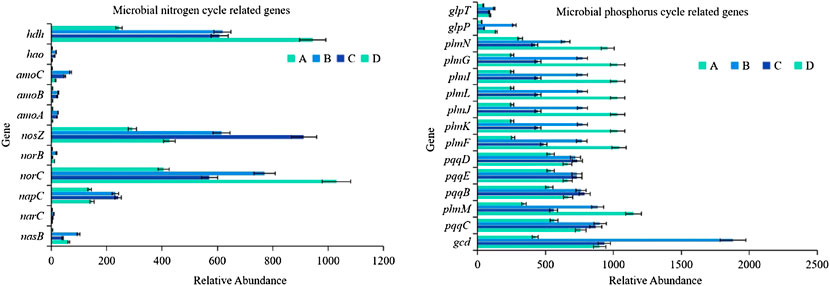
FIGURE 5. Variability of nitrogen and phosphorus cycle functional genes (A), winter; (B), spring; (C), summer; (D), autumn).
A significant correlation was between the physical and chemical properties of water and the genes of nitrogen and phosphorus cycle function (Figure 6). WT had a significant positive correlation with the denitrification gene nosZ and the dissimilatory nitrate reduction gene in nitrogen cycle function genes (p < 0.01), while it had a significant positive correlation with the inorganic phosphorus dissolution genes pqqB, pqqD, and pqqE in the phosphorus cycle genes (p < 0.01). TN and TP were significantly correlated with nitrogen and phosphorus cycle function genes (p < 0.01). The increase of TN concentration affects the expression of phosphorus cycle genes, while the rise of TP concentration affects the expression of nitrogen cycle function genes. The change of DO concentration notably affects the expression of pqqB, pqqD, and pqqE genes, indicating that dissolved oxygen would impact the biomass of some strains containing inorganic phosphorus dissolving genes. In addition, there is a significant positive correlation between nitrogen and phosphorus transformation functional genes (p < 0.001; Figure 7).
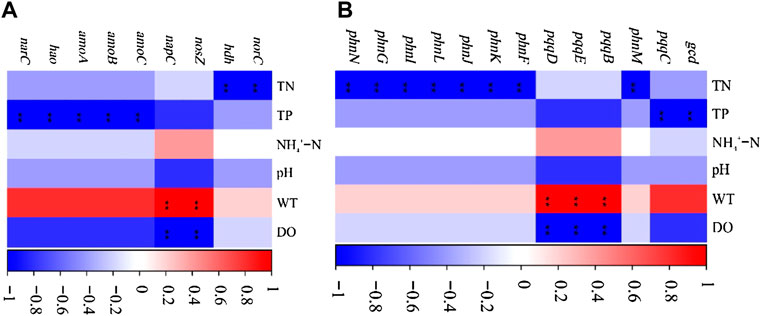
FIGURE 6. Relationships between microbial functional genes and environmental variables (A), nitrogen cycle function genes; (B), phosphorus cycle function genes; *p < 0.05).
OTU Co-occurrence Network of Multi-Trophic Groups
In order to clarify which species have significant correlations, we used different colors to represent different biological groups. The species screening and significance test results indicated that the biological network comprises 592 bacteria, 40 fungi, 126 algae, and 291 invertebrates (Figure 8). A gradual increasing trend from winter to summer existed in the complexity of the network. Compared with the separately generated random network, the heterotrophic food web was more significantly clustered and forms a “small world”. Further analysis of critical nodes in the bacterial and eukaryotic ecological networks revealed that nutrient distribution of taxa included decomizers, producers, and consumers.
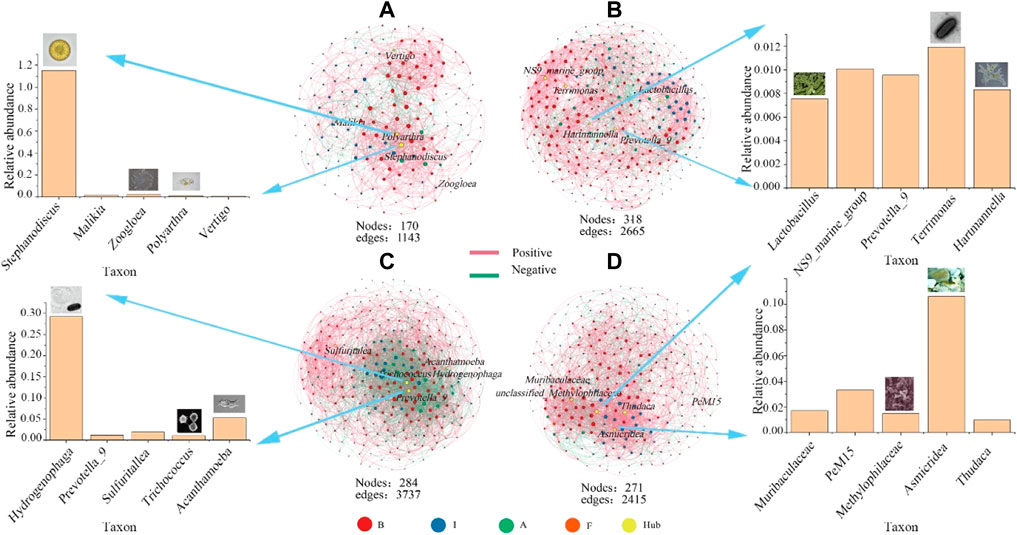
FIGURE 8. Co-occurrence network patterns of multitrophic-level groups (B), bacteria; I, invertebrate; (A), algae; F, fungus). Hub OTUs (keystone species) were labeled, and node size was displayed according to the degree of connections. Larger size indicates more connections (A), winter; (B), spring; (C), summer; (D), autumn).
The positive and negative correlation in the network can infer the symbiosis or competition/predation relationship between water biological communities. In this study, the positive and negative correlations of networks coexisted between different seasons, among which the more intense competition/predation phenomenon between different biological communities in the water network existed in summer. In contrast, the cooperative relationship and competitive relationship of networks coexisted in other seasons. The positive interactions in the network were mainly concentrated in modules with the same species classification, such as Cryptomonas and Chlamydomonas, showing synergy among species, while the other species were mainly negatively correlated. The species that are significantly related in multi-trophic group networks usually come from different trophic levels and a large number of edges target species with low trophic levels to those with high trophic levels or species with high to low trophic levels.
Moreover, we focused on the keystone species (Hub) of the microbial food web in each season (Figure 8), which were mainly composed of microorganisms (malikia), algae (stephanodiscus), and invertebrates (polyarthra). The abundance of bacteria was much higher than that of other groups, indicating its leading role in heterotrophic food webs. Amoeba, which could feed on attached bacteria, was the main protozoan predator of bacteria in the river ecosystem. Arthropods constituted a high nutritional level in the microbial food web, in which rotifers were the main predators of most algae and animals in the network. The low centrality of some hub groups indicated that the network structure was less dependent on individual species.
The running results of the SEM model showed that in the micro-food webs, both “top-down” (predation) and “bottom-up” (resources) forces strictly controlled the relationship between multi-trophic groups (Figure 9). Invertebrate was a critical driving factor in the change in community structure. Heterotrophic bacteria, fungi, and algae had significant effects on invertebrates (p < 0.05), with the path coefficients of 1.01, 0.89, and 0.63, respectively, indicating that invertebrates had the most significant direct impact, followed by algae. Invertebrates had a significant (p < 0.001) effect on heterotrophic bacteria, fungi and algae with path coefficients of 1.01, 0.89 and 0.63, respectively. Algae were remarkably associated with fungi (p < 0.01), with path coefficient of 0.17. In addition, a negative correlation existed between microorganisms (heterotrophic bacteria and fungi) and the nitrogen and phosphorus cycles. Algae positively correlated with nutrients (nitrogen and phosphorus), indicating that algae promoted nutrient circulation via absorption and utilization of nitrogen and phosphorus. Biodiversity-mediated pathways had a crucial impact on ecosystem function.
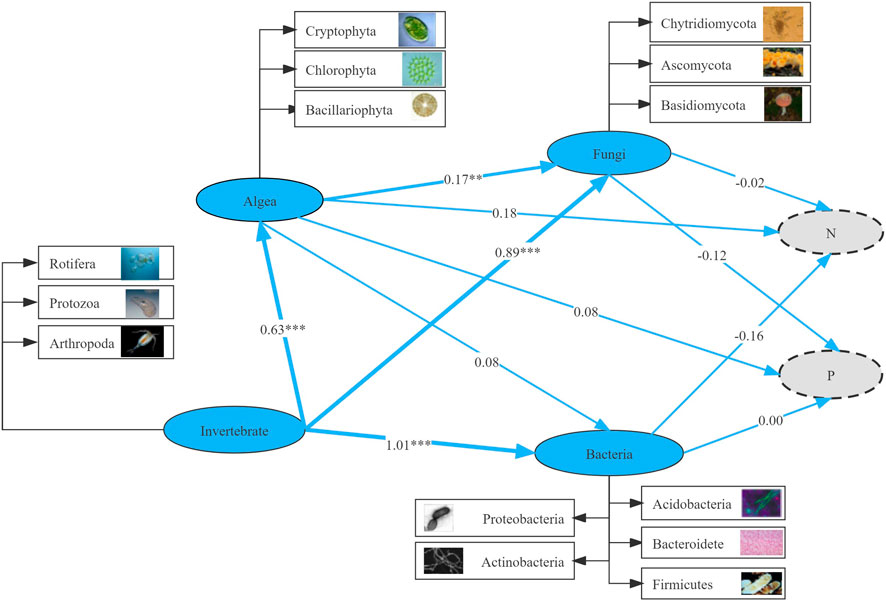
FIGURE 9. Structural equation modeling (SEM) analyzed interactions among multi-trophic levels and direct and indirect effects on nutrient migration and transformation. Numbers next to arrows indicate normalized path coefficients with different levels of significance (*p < 0.05; **p < 0.01; ***p < 0.001).
Effects of Eco-hydrological Variables on Bacterial and Eukaryotic Communities
Correlation analysis (Figure 10) showed a significant correlation between bacteria and environmental and hydrological variables (p < 0.05). Distinct positive correlation between Proteobacteria, Actinobacteria, Chloroflexi, and Acidobacteria and water temperature (p < 0.05). Hydrological variables strongly affected microorganisms, with Actinobacteria, Chloroflexi, and Acidobacteria having a notable positive correlation with the river discharge (p < 0.05). Moreover, a remarkable correlation existed between eukaryotes and environmental and hydrological variables (p < 0.05). Bacillariophyta was significantly positively associated with total phosphorus (p < 0.05), and the relative abundance of Ciliophora, Chlorophyta, Cryptophyta, Ascomycota, Rotifera, and Chordata were significantly positively associated with water temperature (p < 0.05). Bacillariophyta, Chlorophyta, Ascomycota, Pyrrophyta, and Rotifera were significantly positively associated with the river discharge (p < 0.05).
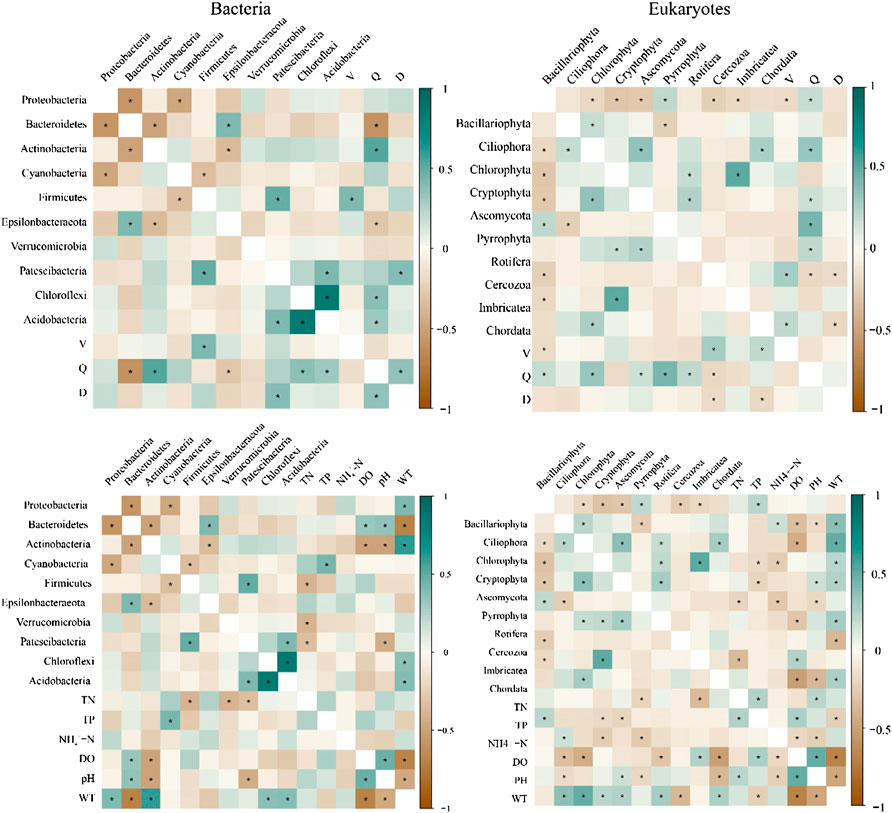
FIGURE 10. The correlation analysis between eco-hydrological variables and the taxa at the phylum level (*p < 0.05). Flow velocity (V), river discharge (Q), and water level (D).
Non-Linear Responses of Bacterial and Eukaryotic Communities to Key Eco-Hydrological Variables
Based on the correlation results, the most significant two factors, water temperature, and river discharge were selected for TITAN analysis with the top 50 abundance species, considering the change points in significant indicator taxa with purity and reliability greater than 0.95 (Figure 11, Figure 12). The TITAN results for bacterial communities indicated that the change point of WT was 24.70°C for the sum (z−) and 5.70 °C for the sum (z+). The change point of river discharge was 152.79 m3/s for the sum (z−), whereas the change point was 12.30 m3/s for sum (z+), and the filtered score was 106.81 for fsum (z+). The threshold range was 18.25–24.70°C for the negative indicator taxa (Arcobacter and Flavobacterium), and 1.75–17.65°C for the positive indicator taxa (CL500-29 marine group and Mycobacterium). The threshold range for the negative response taxa (Flavobacterium and Sediminibacterium) in the runoff indication taxa was 106.80–371.79 m3/s, while for the positive response taxa (Arenimonas and Mycobacterium) was 8.00–106.78 m3/s.
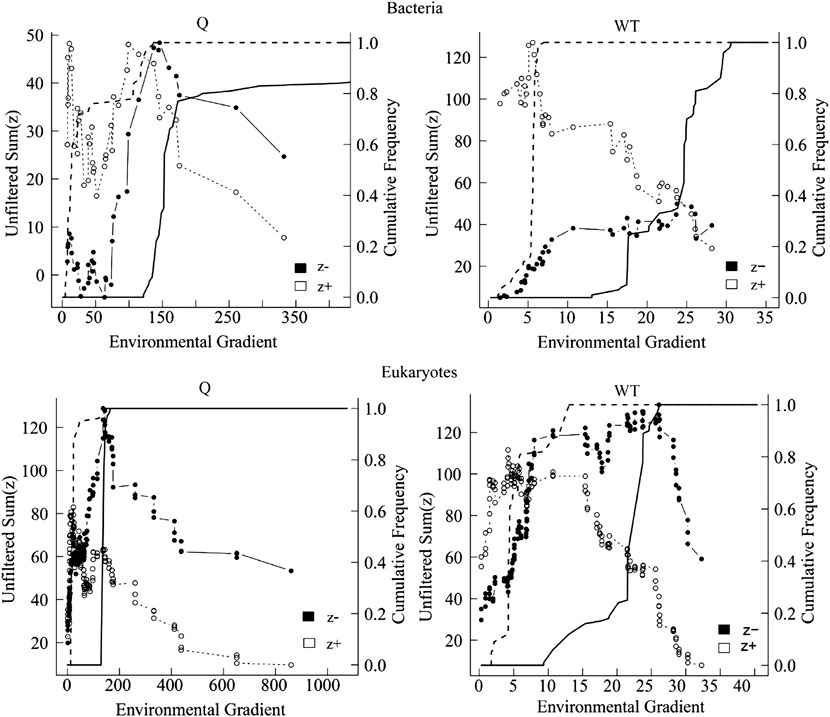
FIGURE 11. TITAN sum (z−) and sum (z+) values corresponding to all candidate change points along the analyzed environmental gradient. Dashed and solid lines represent the cumulative frequency distribution of change points for 500 bootstrap replicates for sum z+ and sum z−, respectively. Peaks in the sums of z− and z + are the sites along the gradient with synchronic decrease and increase of taxa, respectively.
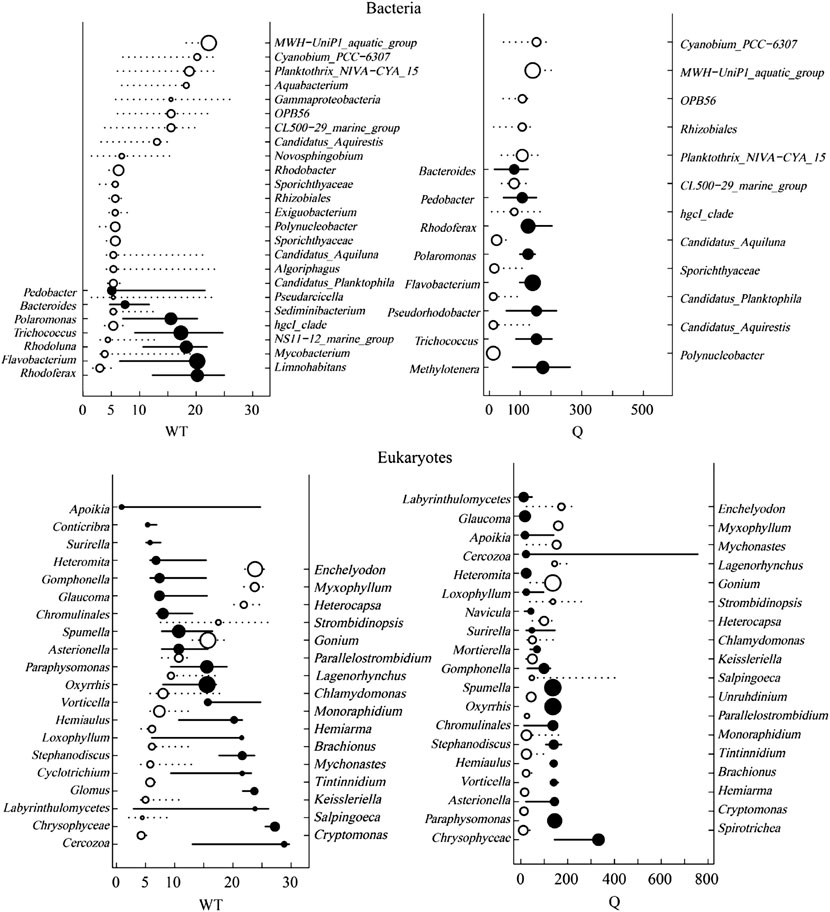
FIGURE 12. Taxon-specific TITAN responded to environmental change, showing significant indicator taxa (p ≤ 0.05). z + and z − represent taxa increased or decreased in the frequency of occurrence and abundance with an increased environment variable, respectively. Circles represent the change point of each taxon in proportion to the magnitude of the response.
The TITAN results of eukaryotic communities showed the change point of water temperature was 26.10 °C for the sum (z−), and 4.25 °C for the sum (z+). The change point of flow was 140.70 m3/s for the sum (z−), and 23.58 m3/s for the sum (z+). The threshold range was 8.00–29.65°Cfor the negative indicator taxa (Spumella and Stylonychia), and 0.90–8.00°C for the positive response taxa (Unruhdinium and Monoraphidium). The threshold range was 46.62–332.10 m3/s for the negative response taxa (Spumella and Glaucoma) in the runoff indication taxa, and 6.69–45.51 m3/s for the positive response taxa (Stylonychia and Monoraphidium).
Discussion
Evolution of Community Structure and Function of Bacteria and Eukaryotes
It is challenging to assess the temporal and spatial variability in complex biomes, as individual taxa respond differently to changing environmental conditions (Meng et al., 2020; Xiao et al., 2021). In spring and autumn, the water temperature varies considerably, and the types of organisms differ, resulting in different species composition of biological communities. In summer, high temperature is suitable for the growth and reproduction of thermophiles. In winter, owing to the low water temperature, biological species reduce, but suitable for the growth of some psychrophilic organisms (Salem, 2021). Due to the influence of habitat differences, nutrients, and water conservancy facilities, the biogeographic distribution of biodiversity and community structure in the upper, middle and lower reaches had significant heterogeneity (Cantonati et al., 2022). The microbial community in the middle and lower reaches is affected by more deterministic selection (Newbold et al., 2020). Previous studies have shown that microorganisms in water may be related to input from industrial sources within the watershed (Sagova-Mareckova et al., 2020). Acinetobacter is a core taxon of the sewage bacterial community and was used to indicate water contamination (Fan et al., 2019). Changes in hydrological factors have also led to differences in the dispersal capacity of aquatic organisms in water, enabling spatial similarity or heterogeneity in biome structure (Ćmiel et al., 2020). In addition, the restriction of biome diffusion by the Baojixia Dam might further contribute to the apparent differences in the species composition between upstream, middle, and downstream communities (Rasdi et al., 2021).
Increasing studies have highlighted the importance of bacterial and eukaryotic communities in nutrient cycling and biochemical degradation (Stewart et al., 2018). The intensity of human disturbance impacted biological communities’ composition and functional attributes and the relationship between them. Previous studies indicated that nitrogen, phosphorus, and excessive nutrients had profound effects on microbial communities’ functional attributes in water (Ouyang et al., 2020). We also observed that most bacteria were involved in metabolic pathways. This result is probably because the microorganisms with pollutant degradation function are selected by high-intensity environmental effects, leading to the synchronous changes of microbial community composition with the alteration of functional attributes (Dini-Andreote et al., 2015). With the increase in water temperature and flow, the microbial activity from spring to autumn was promoted, probably contributing to the degradation of pollutants by microorganisms. Consistent with previous studies, hydrochemical characteristics, temperatures, and nutrients, alone or together, affect microbial-mediated nutrient cycling (Chakraborty et al., 2021). Several studies have shown that a higher abundance of pollutant-degrading bacteria was observed in industrially polluted water, and changes in land use types promoted alterations in the metabolic functions and structures of bacterial communities (Zhang et al., 2016; Zhang et al., 2021).
In this study, Cyanobacteria and Gammaproteobacteria, as the primary nitrogen-fixing microorganisms, are the dominant groups in warm seasons and downstream reaches. Previous studies have shown that nifH could be used as a characterization gene of nitrogen fixation to detect the population structure and diversity of nitrogen-fixing microorganisms in the water environment (Byappanahalli et al., 2019). The amplified sequence of nosZ gene was similar to that of nitrogen-fixing microorganisms, indicating that nitrogen-fixing bacteria are closely associated with denitrifying bacteria (Bao et al., 2021). The decrease in temperature and the increase of DO content would limit the function of reducing microbial bacteria in biological nitrogen removal, reducing the nitrogen source required for microbial growth (Ying et al., 2020). Most phosphorus cycle function genes with significant differences in abundance were inorganic phosphate solubilization, indicating that temperature and water quality changes mainly affect the microbial utilization of inorganic phosphorus such as phosphonates and phosphates (Luo et al., 2017). The biochemical process of phosphorus was closely coupled with the nitrogen cycle, which supports the highly significant positive correlation between the functional groups involved in the transformation of nitrogen and phosphorus (p < 0.001) (Stoliker et al., 2016).
Network Interaction Mechanism Among Multi-Trophic Groups
Most studies on river time series and geographic distribution have focused on identifying changes in limited organisms but rarely explored biological interactions between them (Zhao et al., 2013). The eDNA metabarcoding combined with network analysis provided a unique perspective on the interpretation of river aquatic biodiversity, offering vital information about the complex biological interactions associated with ecosystem changes by simultaneously examining multiple biological domains and trophic levels (Vasselon et al., 2017). In the molecular ecological network analysis, the modular characteristics of biological interaction networks between bacteria and eukaryotes were relatively prominent, indicating that species within the module may be more closely related in survival or function (Chun et al., 2020). The close association and modular phenomenon of taxa in the community also indicate that these biological groups occupy a similar niche and have similar ecological functions (Shi et al., 2019). Vital modules maintain connectivity in the network, conducive to defining critical species in the system.
The complex interactions between multi-trophic groups may fundamentally impact species richness, community composition, ecosystem function, and stability (Zhang et al., 2020). Multi-trophic communities are characterized by high taxonomic and functional diversity. The increase of network nodes and connectivity may provide more functional redundancy, complementary effects, and diversification effects, which was conducive to the stability of the community, so as to improve the utilization efficiency of resources and enhance the resistance and adaptability to environmental changes (Saleem et al., 2019). Heterotrophic bacteria, algae, protozoa (ciliophora and heterotrophic flagellates), and metazoa (arthropods, nematodes, and rotifers) constitute the main components of the microbial food web (Houliez et al., 2021). The results of this study suggested that the interaction between environmental variables and microbial network controls the relationship between different biological groups and directly affects microbial food web structure. Heterotrophic bacteria and invertebrates play a vital regulatory role in the microbiota in the microbial food web. Algae decomposition in rivers is achieved mainly through the direct feeding of invertebrates and the decomposition of extracellular enzymes derived from fungi and bacteria (Marks, 2019). Previous studies have shown that invertebrate and protozoa communities may profoundly impact the abundance, structure, and composition of primary producers, bacteria, and fungi (Yang et al., 2022). Seasonal changes affect the interaction between organisms at different nutrient levels. Due to the low temperature and the decrease of flow and velocity in winter, the enrichment of nutrients in the water body could reduce the competition of organisms for resources and the degree of niche sharing among biological groups (Saleem et al., 2019).
The predator-prey relationships are essential interactions between organisms with different nutritional levels (Kruk et al., 2021). Consistent with most food web studies, we emphasized the crucial role of nutrient interactions in biota. The coexistence of bacteria, algae, and plankton in the Weihe River seasonal networks is speculated to be an interspecies predator-prey relationship. Sufficient food resources can maximize the growth rate of the microbial community and enable organisms to convert resources quickly (Brose et al., 2019). In the hubs of all ecological networks of this study, keystone species (hub) were located at the edge of the network module in different seasons. Not all OTUs in the ecological network play a dominant role in the food web and show a predator-prey relationship. Therefore, we further analyzed the relationships between microbial food web biota and its response to nutrient changes through a structural equation model. The results showed that the interaction of dominant and rare groups was vital for multi-trophic groups to maintain community relationships and enhance community stability under nutrient changes. Nutrient concentrations such as nitrogen and phosphorus are not the only variables affecting the microbial food web model. Nutrients transferred from microorganisms to animals is a vital mechanism of nutrient regeneration, especially for nitrogen and phosphorus (Yang et al., 2020).
Response Mechanism of Multi-Trophic Community to Eco-hydrological Changes
River ecology and hydrology changes may affect the food web model, thus altering the structure and relationship of different groups in the microbial food web (Mor et al., 2018). Previous studies have pointed out that hydrological factors play a critical role in controlling the interaction between predators and prey (Yang et al., 2015). This research reveals that the land use of farmland and urban construction reduce biological diversity and affect the community interdependence of multi-trophic groups, inhibiting typical ecosystem processes within biological communities, and reducing the ecosystem function. In addition to the direct impact of eco-hydrological variables, the indirect cascade effects may also be the reason for the notable enhancement of species interactions in microbial networks in the Weihe River. For instance, invertebrates directly affected the shaping of algae and microorganisms, thus indirectly modulating the function of ecosystems. Compared with the influence of eco-hydrological variables, top-down forces (predation/competition) also significantly impact the nutrient transfer from bacteria to invertebrates. Some studies have suggested that the process of species selection and diffusion result in the differences in the composition of heterotrophic bacteria, algae, fungi, and protozoa communities (Epsztein et al., 2020). In addition, ecological drift plays an essential role in shaping the structure of the water microbial community in the Weihe River (Huber et al., 2020).
Influenced by the hydrological regime and water environment, quantitative analysis of the response of aquatic organisms to changes in ecological and hydrological elements is crucial to river health and determining ecological flow (Xuan et al., 2019). Some studies have shown that changes in hydrology may alter plankton spatial distribution and species structures, and that temperature plays a direct role in plankton growth (Qu et al., 2018). The negative response taxa in microbial taxa, Flavobacterium, indicates that aerobic microorganisms are sensitive to environmental changes (Kim et al., 2021). In this study, there were certain but slight differences in eco-hydrological thresholds between bacteria and eukaryotes, indicating that multi-trophic groups can better determine the range of ecological thresholds (Moutinho et al., 2021). In addition, biological taxa gradually adapt and continue to survive under environmental stress (Bruno et al., 2019). The driving relationship between organisms and habitat may be responsible for the difference in indicator taxa and eco-hydrological thresholds in the Weihe River (Baker and King, 2010).
Conclusion
In this study, eDNA technology was used to analyze the characteristics of the changing structures and functions of bacterial and eukaryotic communities. The community structures of bacteria and eukaryotes showed notable seasonal changes (p < 0.05). The multi-trophic groups in the middle and lower reaches of the Weihe River were mainly affected by habitat differences. The abundance of functional genes varied slightly (p < 0.001), among which nitrogen and phosphorus cycle function genes showed the characteristics of abundance fluctuation in spring, summer, and autumn. The interaction between biological taxa was complex, and network modularity existed within the community. Multi-trophic groups showed an apparent predator-prey relationship. In the aquatic ecosystem, a microbial food web with multi-nutrient levels played a vital role in the circulation of nitrogen and phosphorus.
According to correlation analyses, a significant association existed between bacterial and eukaryotic groups and eco-hydrological variables (p < 0.05). TITAN analysis was used to identify eco-hydrological thresholds and potential indicator taxa for the multi-trophic community. Our approach demonstrated the value of multi-trophic taxa for setting goals for biodiversity conservation. However, due to the limitations of study subjects and spatiotemporal scales, we suggest that further work is required to determine suitability thresholds of taxa. The study demonstrated the application of eDNA technology in the field of river biodiversity conservation and ecological restoration. Meanwhile, it also provides a new perspective on how the food web respond to interferes with the environment and promote the nutrient cycle under the changing environment, reinforcing the concept of the assembly of bacteria and eukaryotes communities (Moutinho et al., 2021; Cantonati et al., 2022).
Data Availability Statement
The raw data supporting the conclusion of this article will be made available by the authors, without undue reservation.
Author Contributions
DL: Investigation, Data curation, Writing Original draft, Reviewing and Editing. JX and JS: Conceptualization, Methodology, Supervision, Project administration, Funding acquisition. HS and WX: Software, Validation, Visualization.
Funding
This study was jointly supported by the National Natural Science Foundation of China (Grant No. 51679200), the Key Research and Development Program of Shaanxi (Grant No. 2019ZDLSF05-02) and Shaanxi Province Water Conservancy Science and Technology Project (Grant No. 2020slkj-13).
Conflict of Interest
The authors declare that the research was conducted in the absence of any commercial or financial relationships that could be construed as a potential conflict of interest.
Publisher’s Note
All claims expressed in this article are solely those of the authors and do not necessarily represent those of their affiliated organizations, or those of the publisher, the editors and the reviewers. Any product that may be evaluated in this article, or claim that may be made by its manufacturer, is not guaranteed or endorsed by the publisher.
References
Bagley, M., Pilgrim, E., Knapp, M., Yoder, C., Santo Domingo, J., and Banerji, A. (2019). High-throughput Environmental DNA Analysis Informs a Biological Assessment of an Urban Stream. Ecol. Indic. 104, 378–389. doi:10.1016/j.ecolind.2019.04.088
Bahram, M., Netherway, T., Frioux, C., Ferretti, P., Coelho, L. P., Geisen, S., et al. (2021). Metagenomic Assessment of the Global Diversity and Distribution of Bacteria and Fungi. Environ. Microbiol. 23 (1), 316–326. doi:10.1111/1462-2920.15314
Baker, M. E., and King, R. S. (2010). A New Method for Detecting and Interpreting Biodiversity and Ecological Community Thresholds. Methods Ecol. Evol. 1 (1), 25–37. doi:10.1111/j.2041-210X.2009.00007.x
Bao, S., Xu, J., Tang, W., and Fang, T. (2021). Effect and Mechanism of Silver Nanoparticles on Nitrogen Transformation in Water-Sediment System of a Hypereutrophic Lake. Sci. Total Environ. 761, 144182. doi:10.1016/j.scitotenv.2020.144182
Berger, E., Haase, P., Schäfer, R. B., and Sundermann, A. (2018). Towards Stressor-specific Macroinvertebrate Indices: Which Traits and Taxonomic Groups Are Associated with Vulnerable and Tolerant Taxa? Sci. Total Environ. 619-620, 144–154. doi:10.1016/j.scitotenv.2017.11.022
Brose, U., Archambault, P., Barnes, A. D., Bersier, L.-F., Boy, T., Canning-Clode, J., et al. (2019). Predator Traits Determine Food-Web Architecture across Ecosystems. Nat. Ecol. Evol. 3 (6), 919–927. doi:10.1038/s41559-019-0899-x
Bruno, D., Belmar, O., Maire, A., Morel, A., Dumont, B., and Datry, T. (2019). Structural and Functional Responses of Invertebrate Communities to Climate Change and Flow Regulation in Alpine Catchments. Glob. Change Biol. 25 (5), 1612–1628. doi:10.1111/gcb.14581
Byappanahalli, M. N., Nevers, M. B., Przybyla‐Kelly, K., Ishii, S., King, T. L., and Aunins, A. W. (2019). Great Lakes Cladophora Harbors Phylogenetically Diverse Nitrogen‐fixing Microorganisms. Environ. DNA 1 (2), 186–195. doi:10.1002/edn3.20
Cantonati, M., Hofmann, G., Spitale, D., Werum, M., and Lange-Bertalot, H. (2022). Diatom Red Lists: Important Tools to Assess and Preserve Biodiversity and Habitats in the Face of Direct Impacts and Environmental Change. Biodivers. Conservation 31, 1–25. doi:10.1007/s10531-021-02339-9
Chakraborty, S., Andersen, K. H., Visser, A. W., Inomura, K., Follows, M. J., and Riemann, L. (2021). Quantifying Nitrogen Fixation by Heterotrophic Bacteria in Sinking Marine Particles. Nat. Commun. 12 (1), 1–14. doi:10.1038/s41467-021-23875-6
Chen, J., Chen, Z., Liu, S., Guo, W., Li, D., Minamoto, T., et al. (2021). Revealing an Invasion Risk of Fish Species in Qingdao Underwater World by Environmental DNA Metabarcoding. J. Ocean. Univ. China 20 (1), 124–136. doi:10.1007/s11802-021-4448-2
Chi, Z., Zhu, Y., Li, H., Wu, H., and Yan, B. (2021). Unraveling Bacterial Community Structure and Function and Their Links with Natural Salinity Gradient in the Yellow River Delta. Sci. Total Environ. 773, 145673. doi:10.1016/j.scitotenv.2021.145673
Chun, S.-J., Cui, Y., Lee, J. J., Choi, I.-C., Oh, H.-M., and Ahn, C.-Y. (2020). Network Analysis Reveals Succession of Microcystis Genotypes Accompanying Distinctive Microbial Modules with Recurrent Patterns. Water Res. 170, 115326. doi:10.1016/j.watres.2019.115326
Ćmiel, A. M., Strużyński, A., Wyrębek, M., Lipińska, A. M., Zając, K., and Zając, T. (2020). Response of Freshwater Mussel Recruitment to Hydrological Changes in a Eutrophic Floodplain Lake. Sci. Total Environ. 703, 135467. doi:10.1016/j.scitotenv.2019.135467
Dini-Andreote, F., Stegen, J. C., Van Elsas, J. D., and Salles, J. F. (2015). Disentangling Mechanisms that Mediate the Balance between Stochastic and Deterministic Processes in Microbial Succession. Proc. Natl. Acad. Sci. U. S. A. 112 (11), E1326–E1332. doi:10.1073/pnas.1414261112
Douglas, G. M., Maffei, V. J., Zaneveld, J., Yurgel, S. N., Brown, J. R., Taylor, C. M., et al. (2020). PICRUSt2: An Improved and Customizable Approach for Metagenome Inference. New York, NY: Cold Spring Harbor Laboratory, 672295.
Epsztein, R., DuChanois, R. M., Ritt, C. L., Noy, A., and Elimelech, M. (2020). Towards Single-Species Selectivity of Membranes with Subnanometre Pores. Nat. Nanotechnol. 15 (6), 426–436. doi:10.1038/s41565-020-0713-6
Fan, Y.-Y., Li, B.-B., Yang, Z.-C., Cheng, Y.-Y., Liu, D.-F., and Yu, H.-Q. (2019). Mediation of Functional Gene and Bacterial Community Profiles in the Sediments of Eutrophic Chaohu Lake by Total Nitrogen and Season. Environ. Pollut. 250, 233–240. doi:10.1016/j.envpol.2019.04.028
Haase, P., Tonkin, J. D., Stoll, S., Burkhard, B., Frenzel, M., Geijzendorffer, I. R., et al. (2018). The Next Generation of Site-Based Long-Term Ecological Monitoring: Linking Essential Biodiversity Variables and Ecosystem Integrity. Sci. Total Environ. 613-614, 1376–1384. doi:10.1016/j.scitotenv.2017.08.111
Harper, L. R., Buxton, A. S., Rees, H. C., Bruce, K., Brys, R., Halfmaerten, D., et al. (2019). Prospects and Challenges of Environmental DNA (eDNA) Monitoring in Freshwater Ponds. Hydrobiologia 826 (1), 25–41. doi:10.1007/s10750-018-3750-5
Houliez, E., Lefebvre, S., Dessier, A., Huret, M., Marquis, E., Bréret, M., et al. (2021). Spatio-temporal Drivers of Microphytoplankton Community in the Bay of Biscay: Do Species Ecological Niches Matter? Prog. Oceanogr. 194, 102558. doi:10.1016/j.pocean.2021.102558
Hu, N., Bourdeau, P. E., Harlos, C., Liu, Y., and Hollander, J. (2022). Meta-analysis Reveals Variance in Tolerance to Climate Change across Marine Trophic Levels. Sci. Total Environ. 827, 154244. doi:10.1016/j.scitotenv.2022.154244
Huber, P., Metz, S., Unrein, F., Mayora, G., Sarmento, H., and Devercelli, M. (2020). Environmental Heterogeneity Determines the Ecological Processes that Govern Bacterial Metacommunity Assembly in a Floodplain River System. Isme J. 14 (12), 2951–2966. doi:10.1038/s41396-020-0723-2
Kim, M., Lee, B.-H., Lee, K.-E., and Park, W. (2021). Flavobacterium Phycosphaerae Sp. Nov. Isolated from the Phycosphere of Microcystis Aeruginosa. Int. J. Syst. Evol. Microbiol. 71 (3), 004735. doi:10.1099/ijsem.0.005185
Kruk, M., Paturej, E., and Obolewski, K. (2021). Zooplankton Predator-Prey Network Relationships Indicates the Saline Gradient of Coastal Lakes. Machine Learning and Meta-Network Approach. Ecol. Indic. 125, 107550. doi:10.1016/j.ecolind.2021.107550
Leray, M., Yang, J. Y., Meyer, C. P., Mills, S. C., Agudelo, N., Ranwez, V., et al. (2013). A New Versatile Primer Set Targeting a Short Fragment of the Mitochondrial COI Region for Metabarcoding Metazoan Diversity: Application for Characterizing Coral Reef Fish Gut Contents. Front. Zool. 10, 34. doi:10.1186/1742-9994-10-34
Li, F., Altermatt, F., Yang, J., An, S., Li, A., and Zhang, X. (2020). Human Activities' Fingerprint on Multitrophic Biodiversity and Ecosystem Functions across a Major River Catchment in China. Glob. Change Biol. 26 (12), 6867–6879. doi:10.1111/gcb.15357
Liang, D., Song, J., Xia, J., Chang, J., Kong, F., Sun, H., et al. (2022). Effects of Heavy Metals and Hyporheic Exchange on Microbial Community Structure and Functions in Hyporheic Zone. J. Environ. Manag. 303, 114201. doi:10.1016/j.jenvman.2021.114201
Liu, Y., Li, D., Gao, H., Li, Y., Chen, W., Jiao, S., et al. (2022). Regulation of Soil Micro-foodwebs to Root Secondary Metabolites in Cultivated and Wild Licorice Plants. Sci. Total Environ. 828, 154302. doi:10.1016/j.scitotenv.2022.154302
Logares, R., Tesson, S. V. M., Canbäck, B., Pontarp, M., Hedlund, K., and Rengefors, K. (2018). Contrasting Prevalence of Selection and Drift in the Community Structuring of Bacteria and Microbial Eukaryotes. Environ. Microbiol. 20 (6), 2231–2240. doi:10.1111/1462-2920.14265
Luo, G., Ling, N., Nannipieri, P., Chen, H., Raza, W., Wang, M., et al. (2017). Long-term Fertilisation Regimes Affect the Composition of the Alkaline Phosphomonoesterase Encoding Microbial Community of a Vertisol and its Derivative Soil Fractions. Biol. Fertil. Soils 53 (4), 375–388. doi:10.1007/s00374-017-1183-3
Ma, Y., Wang, Y., Chen, Q., Li, Y., Guo, D., Nie, X., et al. (2020). Assessment of Heavy Metal Pollution and the Effect on Bacterial Community in Acidic and Neutral Soils. Ecol. Indic. 117, 106626. doi:10.1016/j.ecolind.2020.106626
Marks, J. C. (2019). Revisiting the Fates of Dead Leaves that Fall into Streams. Annu. Rev. Ecol. Evol. Syst. 50, 547–568. doi:10.1146/annurev-ecolsys-110218-024755
Martins, R. T., Brito, J., Dias-Silva, K., Leal, C. G., Leitão, R. P., Oliveira, V. C., et al. (2021). Low Forest-Loss Thresholds Threaten Amazonian Fish and Macroinvertebrate Assemblage Integrity. Ecol. Indic. 127, 107773. doi:10.1016/j.ecolind.2021.107773
Meng, C., Song, X., Tian, K., Ye, B., Si, T., and Yurdakal, S. (2020). Spatiotemporal Variation Characteristics of Water Pollution and the Cause of Pollution Formation in a Heavily Polluted River in the Upper Hai River. J. Chem. 2020, 1–15. doi:10.1155/2020/6617227
Mor, J.-R., Ruhí, A., Tornés, E., Valcárcel, H., Muñoz, I., and Sabater, S. (2018). Dam Regulation and Riverine Food-Web Structure in a Mediterranean River. Sci. Total Environ. 625, 301–310. doi:10.1016/j.scitotenv.2017.12.296
Moutinho, F. H. M., Marafão, G. A., Calijuri, M. d. C., Moreira, M. Z., Marcarelli, A. M., and Cunha, D. G. F. (2021). Environmental Factors and Thresholds for Nitrogen Fixation by Phytoplankton in Tropical Reservoirs. Int. Rev. Hydrobiol. 106 (1), 5–17. doi:10.1002/iroh.202002057
Newbold, T., Oppenheimer, P., Etard, A., and Williams, J. J. (2020). Tropical and Mediterranean Biodiversity Is Disproportionately Sensitive to Land-Use and Climate Change. Nat. Ecol. Evol. 4 (12), 1630–1638. doi:10.1038/s41559-020-01303-0
Niu, L., Li, Y., Wang, P., Zhang, W., Wang, C., Li, J., et al. (2018). Development of a Microbial Community-Based Index of Biotic Integrity (MC-IBI) for the Assessment of Ecological Status of Rivers in the Taihu Basin, China. Ecological Indicators 85, 204–213. doi:10.1016/j.ecolind.2017.10.051
Ouyang, L., Chen, H., Liu, X., Wong, M. H., Xu, F., Yang, X., et al. (2020). Characteristics of Spatial and Seasonal Bacterial Community Structures in a River under Anthropogenic Disturbances. Environ. Pollut. 264, 114818. doi:10.1016/j.envpol.2020.114818
Qu, Y., Wu, N., Guse, B., and Fohrer, N. (2018). Riverine Phytoplankton Shifting along a Lentic-Lotic Continuum under Hydrological, Physiochemical Conditions and Species Dispersal. Sci. Total Environ. 619-620, 1628–1636. doi:10.1016/j.scitotenv.2017.10.139
Rasdi, N. W., Qin, J. G., Naseer, N. M., Ikhwanuddin, M., Yik Sung, Y., and Hagiwara, A. (2021). The Impact of Feeding Algae and Canola Oil on the Growth, Survival and Reproduction of Moina Sp. Songklanakarin J. Sci. Technol. 43 (3), 864–870. doi:10.14456/sjst-psu.2021.114
Sagova-Mareckova, M., Boenigk, J., Bouchez, A., Cermakova, K., Chonova, T., Cordier, T., et al. (2020). Expanding Ecological Assessment by Integrating Microorganisms into Routine Freshwater Biomonitoring. Water Res. 191, 116767. doi:10.1016/j.watres.2020.116767
Saleem, M., Hu, J., and Jousset, A. (2019). More Than the Sum of its Parts: Microbiome Biodiversity as a Driver of Plant Growth and Soil Health. Annu. Rev. Ecol. Evol. Syst. 50, 145–168. doi:10.1146/annurev-ecolsys-110617-062605
Salem, T. A. (2021). Changes in the Physicochemical and Biological Characteristics in the Lentic and Lotic Waters of the Nile River. Egypt. J. Aquatic Res. 47 (1), 21–27. doi:10.1016/j.ejar.2020.12.003
Shi, Y., Fan, K., Li, Y., Yang, T., He, J. S., and Chu, H. (2019). Archaea Enhance the Robustness of Microbial Co‐occurrence Networks in Tibetan Plateau Soils. Soil Sci. Soc. Am. J. 83 (4), 1093–1099. doi:10.2136/sssaj2018.11.0426
Simonin, M., Voss, K. A., Hassett, B. A., Rocca, J. D., Wang, S. Y., Bier, R. L., et al. (2019). In Search of Microbial Indicator Taxa: Shifts in Stream Bacterial Communities along an Urbanization Gradient. Environ. Microbiol. 21 (10), 3653–3668. doi:10.1111/1462-2920.14694
Smith, S. R., Dupont, C. L., McCarthy, J. K., Broddrick, J. T., Oborník, M., Horák, A., et al. (2019). Evolution and Regulation of Nitrogen Flux through Compartmentalized Metabolic Networks in a Marine Diatom. Nat. Commun. 10 (1), 4552–4614. doi:10.1038/s41467-019-12407-y
Song, J., Tang, B., Zhang, J., Dou, X., Liu, Q., and Shen, W. (2018). System Dynamics Simulation for Optimal Stream Flow Regulations under Consideration of Coordinated Development of Ecology and Socio-Economy in the Weihe River Basin, China. Ecol. Eng. 124, 51–68. doi:10.1016/j.ecoleng.2018.09.024
Song, J., Yang, Z., Xia, J., and Cheng, D. (2021). The Impact of Mining-Related Human Activities on Runoff in Northern Shaanxi, China. J. Hydrology 598, 126235. doi:10.1016/j.jhydrol.2021.126235
State Environmental Protection Administration (2002). The National Standards of the People’s Republic of China: Environmental Quality Standards for Surface Water (GB 3838-2002). Beijing, China: Chinese Environmental Sciences Press
Stewart, S. D., Hamilton, D. P., Baisden, W. T., Verburg, P., and Duggan, I. C. (2018). The Role of Mobile Consumers in Lake Nutrient Cycles: a Brief Review. Hydrobiologia 818 (1), 11–29. doi:10.1007/s10750-018-3603-2
Stoeck, T., Frühe, L., Forster, D., Cordier, T., Martins, C. I. M., and Pawlowski, J. (2018). Environmental DNA Metabarcoding of Benthic Bacterial Communities Indicates the Benthic Footprint of Salmon Aquaculture. Mar. Pollut. Bull. 127, 139–149. doi:10.1016/j.marpolbul.2017.11.065
Stoliker, D. L., Repert, D. A., Smith, R. L., Song, B., LeBlanc, D. R., McCobb, T. D., et al. (2016). Hydrologic Controls on Nitrogen Cycling Processes and Functional Gene Abundance in Sediments of a Groundwater Flow-Through Lake. Environ. Sci. Technol. 50 (7), 3649–3657. doi:10.1021/acs.est.5b06155
Su, P., Wang, X., Lin, Q., Peng, J., Song, J., Fu, J., et al. (2019). Variability in Macroinvertebrate Community Structure and its Response to Ecological Factors of the Weihe River Basin, China. Ecol. Eng. 140, 105595. doi:10.1016/j.ecoleng.2019.105595
Sultana, J., Recknagel, F., Tibby, J., and Maxwell, S. (2019). Comparison of Water Quality Thresholds for Macroinvertebrates in Two Mediterranean Catchments Quantified by the Inferential Techniques TITAN and HEA. Ecol. Indic. 101, 867–877. doi:10.1016/j.ecolind.2019.02.003
Sun, J., Chen, X., Yu, J., Chen, Z., Liu, L., Yue, Y., et al. (2021). Deciphering Historical Water-Quality Changes Recorded in Sediments Using eDNA. Front. Environ. Sci. 9, 669582. doi:10.3389/fenvs.2021.669582
Suter, L., Polanowski, A. M., Clarke, L. J., Kitchener, J. A., and Deagle, B. E. (2021). Capturing Open Ocean Biodiversity: Comparing Environmental DNA Metabarcoding to the Continuous Plankton Recorder. Mol. Ecol. 30 (13), 3140–3157. doi:10.1111/mec.15587
Triadó-Margarit, X., Capitán, J. A., Menéndez-Serra, M., Ortiz-Álvarez, R., Ontiveros, V. J., Casamayor, E. O., et al. (2019). A Randomized Trait Community Clustering Approach to Unveil Consistent Environmental Thresholds in Community Assembly. ISME J. 13 (11), 2681–2689. doi:10.1038/s41396-019-0454-4
Valente-Neto, F., Martínez, B. T., Hughes, R. M., Ferreira, A., Severo-Neto, F., Souza, F. L. d., et al. (2021). Incorporating Costs, Thresholds and Spatial Extents for Selecting Stream Bioindicators in an Ecotone between Two Brazilian Biodiversity Hotspots. Ecol. Indic. 127, 107761. doi:10.1016/j.ecolind.2021.107761
Valentini, A., Taberlet, P., Miaud, C., Civade, R., Herder, J., Thomsen, P. F., et al. (2016). Next-generation Monitoring of Aquatic Biodiversity Using Environmental DNA Metabarcoding. Mol. Ecol. 25 (4), 929–942. doi:10.1111/mec.13428
Vasselon, V., Rimet, F., Tapolczai, K., and Bouchez, A. (2017). Assessing Ecological Status with Diatoms DNA Metabarcoding: Scaling-Up on a WFD Monitoring Network (Mayotte Island, France). Ecol. Indic. 82, 1–12. doi:10.1016/j.ecolind.2017.06.024
Tang, X., Xie, G., Shao, K., Hu, Y., Cai, J., Bai, C., et al. (2020). Contrast Diversity Patterns and Processes of Microbial Community Assembly in a River-Lake Continuum Across a Catchment Scale in Northwestern China. Environmental Microbiome 15 (1), 1–17. doi:10.1186/s40793-020-00356-9
Walters, W., Hyde, E. R., Berg-Lyons, D., Ackermann, G., Humphrey, G., Parada, A., et al. (2016). Improved Bacterial 16S rRNA Gene (V4 and V4-5) and Fungal Internal Transcribed Spacer Marker Gene Primers for Microbial Community Surveys. Msystems 1 (1), e00009–00015. doi:10.1128/mSystems.00009-15
Webster, T. M. U., Consuegra, S., Hitchings, M., and de Leaniz, C. G. (2018). Interpopulation Variation in the Atlantic Salmon Microbiome Reflects Environmental and Genetic Diversity. Applied and Environmental Microbiology 84 (16). doi:10.1128/aem.00691-18
Wang, J., Zhang, T., Li, L., Li, J., Feng, Y., and Lu, Q. (2017). The Patterns and Drivers of Bacterial and Fungal β-Diversity in a Typical Dryland Ecosystem of Northwest China. Frontiers in Microbiology 8. doi:10.3389/fmicb.2017.02126
Wang, X., Su, P., Lin, Q., Song, J., Sun, H., Cheng, D., et al. (2019). Distribution, Assessment and Coupling Relationship of Heavy Metals and Macroinvertebrates in Sediments of the Weihe River Basin. Sustain. Cities Soc. 50, 101665. doi:10.1016/j.scs.2019.101665
Wu, H., Li, Y., Zhang, W., Wang, C., Wang, P., Niu, L., et al. (2019). Bacterial Community Composition and Function Shift with the Aggravation of Water Quality in a Heavily Polluted River. J. Environ. Manag. 237, 433–441. doi:10.1016/j.jenvman.2019.02.101
Xiao, Z., Li, G., Zhao, Y., Xiao, K., Chen, Q., Bao, P., et al. (2021). Bacterioplankton Richness and Composition in a Seasonal Urban River. Front. Environ. Sci. 417, 731227. doi:10.3389/fenvs.2021.731227
Xie, Y., Wang, J., Yang, J., Giesy, J. P., Yu, H., and Zhang, X. (2017). Environmental DNA Metabarcoding Reveals Primary Chemical Contaminants in Freshwater Sediments from Different Land-Use Types. Chemosphere 172, 201–209. doi:10.1016/j.chemosphere.2016.12.117
Xu, H., Zhang, S., Ma, G., Zhang, Y., Li, Y., and Pei, H. (2020). 18S rRNA Gene Sequencing Reveals Significant Influence of Anthropogenic Effects on Microeukaryote Diversity and Composition along a River-To-Estuary Gradient Ecosystem. Sci. Total Environ. 705, 135910. doi:10.1016/j.scitotenv.2019.135910
Xuan, L., Sheng, Z., Lu, J., Qiu, Q., Chen, J., and Xiong, J. (2019). Bacterioplankton Community Responses and the Potential Ecological Thresholds along Disturbance Gradients. Sci. Total Environ. 696, 134015. doi:10.1016/j.scitotenv.2019.134015
Yang, Z., Liu, X., Zhou, M., Ai, D., Wang, G., Wang, Y., et al. (2015). The Effect of Environmental Heterogeneity on Species Richness Depends on Community Position along the Environmental Gradient. Sci. Rep. 5, 723. doi:10.1038/srep15723
Yang, N., Li, Y., Zhang, W., Lin, L., Qian, B., Wang, L., et al. (2020). Cascade Dam Impoundments Restrain the Trophic Transfer Efficiencies in Benthic Microbial Food Web. Water Res. 170, 115351. doi:10.1016/j.watres.2019.115351
Yang, J., Lv, J., Liu, Q., Nan, F., Li, B., Xie, S., et al. (2021a). Seasonal and Spatial Patterns of Eukaryotic Phytoplankton Communities in an Urban River Based on Marker Gene. Sci. Rep. 11 (1), 23147. doi:10.1038/s41598-021-02183-5
Yang, J., Xie, B., Zhang, D., and Tao, W. (2021b). Climate and Land Use Change Impacts on Water Yield Ecosystem Service in the Yellow River Basin, China. Environ. Earth Sci. 80 (3), 1–12. doi:10.1007/s12665-020-09277-9
Yang, N., Wang, L., Lin, L., Li, Y., Zhang, W., Niu, L., et al. (2022). Pelagic-benthic Coupling of the Microbial Food Web Modifies Nutrient Cycles along a Cascade-Dammed River. Front. Environ. Sci. Eng. 16 (4), 1–13. doi:10.1007/s11783-021-1484-5
Ying, C., Chang, M.-J., Chang, Y.-T., Chao, W.-L., Yeh, S.-L., and Hsu, J.-T. (2020). Photosynthetic Bacteria Enhanced Water Quality and Integrity of Microbial Community Composition of Integrated Multitrophic Aquaculture System of Milkfish Chanos Chanos Coastal Farming. Fish. Sci. 86 (2), 329–338. doi:10.1007/s12562-019-01387-z
Zhang, X., Gu, Q., Long, X.-E., Li, Z.-L., Liu, D.-X., Ye, D.-H., et al. (2016). Anthropogenic Activities Drive the Microbial Community and its Function in Urban River Sediment. J. Soils Sediments 16 (2), 716–725. doi:10.1007/s11368-015-1246-8
Zhang, Y., Pavlovska, M., Stoica, E., Prekrasna, I., Yang, J., Slobodnik, J., et al. (2020). Holistic Pelagic Biodiversity Monitoring of the Black Sea via eDNA Metabarcoding Approach: From Bacteria to Marine Mammals. Environ. Int. 135, 105307. doi:10.1016/j.envint.2019.105307
Zhang, L., Li, X., Fang, W., Cheng, Y., Cai, H., and Zhang, S. (2021). Impact of Different Types of Anthropogenic Pollution on Bacterial Community and Metabolic Genes in Urban River Sediments. Sci. Total Environ. 793, 148475. doi:10.1016/j.scitotenv.2021.148475
Zhao, C., Liu, C., Zhao, J., Xia, J., Yu, Q., and Eamus, D. (2013). Zooplankton in Highly Regulated Rivers: Changing with Water Environment. Ecol. Eng. 58, 323–334. doi:10.1016/j.ecoleng.2013.07.035
Zhao, G., Pan, B., Li, Y., Zheng, X., Zhu, P., Zhang, L., et al. (2020). Phytoplankton in the Heavy Sediment-Laden Weihe River and its Tributaries from the Northern Foot of the Qinling Mountains: Community Structure and Environmental Drivers. Environ. Sci. Pollut. Res. 27 (8), 8359–8370. doi:10.1007/s11356-019-07346-6
Keywords: multi-trophic biodiversity, predator-prey, eco-hydrological changes, eDNA, weihe river
Citation: Liang D, Xia J, Song J, Sun H and Xu W (2022) Using eDNA to Identify the Dynamic Evolution of Multi-Trophic Communities Under the Eco-Hydrological Changes in River. Front. Environ. Sci. 10:929541. doi: 10.3389/fenvs.2022.929541
Received: 27 April 2022; Accepted: 25 May 2022;
Published: 16 June 2022.
Edited by:
Yuanchun Zou, Northeast Institute of Geography and Agroecology (CAS), ChinaReviewed by:
Guangqiu Jin, Hohai University, ChinaLi Chen, Nanjing University of Information Science and Technology, China
Copyright © 2022 Liang, Xia, Song, Sun and Xu. This is an open-access article distributed under the terms of the Creative Commons Attribution License (CC BY). The use, distribution or reproduction in other forums is permitted, provided the original author(s) and the copyright owner(s) are credited and that the original publication in this journal is cited, in accordance with accepted academic practice. No use, distribution or reproduction is permitted which does not comply with these terms.
*Correspondence: Jun Xia, eGlhakBpZ3NucnIuYWMuY24=