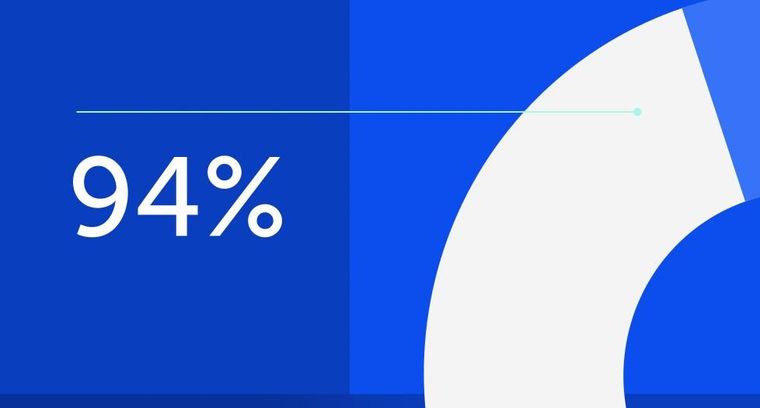
94% of researchers rate our articles as excellent or good
Learn more about the work of our research integrity team to safeguard the quality of each article we publish.
Find out more
HYPOTHESIS AND THEORY article
Front. Environ. Sci., 30 June 2022
Sec. Environmental Economics and Management
Volume 10 - 2022 | https://doi.org/10.3389/fenvs.2022.927668
This article is part of the Research TopicGreen Innovation and Industrial Ecosystem Reconstruction in Achieving Environmental SustainabilityView all 34 articles
With the development of the economy and society, environmental pollution and resource waste problems are emerging, especially in agricultural production, and the adoption of ecological agricultural technologies is a prerequisite to alleviate ecological pressure. Based on the Technology Acceptance Model—Theory of Planned Behavior (TAM-TPB) and using research data from Hubei, Hunan, and Anhui provinces, this paper empirically analyzes the factors influencing farmers’ intention to adopt rice and shrimp crop technologies using the PLS-SEM method. The configuration path of high technology intention was further investigated by the fsQCA method. The results showed that: 1) farmers’ intention to adopt rice-shrimp crop technology was mainly positively influenced by behavioral attitude, subjective norm, perceived behavioral control, behavioral attitude; 2) Perceived usefulness and perceived ease of use had a direct effect on farmers’ intention to adopt and an indirect effect with behavioral attitude as a mediating variable, while perceived ease of use had a positive effect and perceived usefulness did not. In doing so, four configuration paths of high technology acceptance intention were obtained. Given this, this paper makes relevant suggestions, suggesting that the relevant departments focus on the comprehensive benefits of rice-shrimp crop technology; agricultural technology departments provide technical assistance to farmers, and village committees organize regular inter-farmer exchanges.
The rapidly developing ecological farming industry in recent years has caused serious threats to the ecological environment and public health and safety (Xue et al., 2021), while ensuring a stable supply of waterfowl products in domestic and international markets and promoting farmers’ income growth (Li et al., 2021; Zhang et al., 2022). The modern ecological agriculture technology (EAT) of resourceful recycling of waterfowl waste is an effective measure to achieve economic, ecological and social benefits (Huang, 2021; Spanaki et al., 2022). Innovations in new technologies or innovative use of technologies can better mitigate or address global energy, environmental and other carbon emissions (Sun et al., 2021b), especially in rural areas that lack adequate infrastructure and manpower (Sun et al., 2021a). For this reason, governments have been actively promoting ecological agriculture to improve environmental quality (de Lima Vieira et al., 2021). For example, Iran is actively promoting integrated pest management technology to protect agricultural production (Rezaei et al., 2019), the United States is carrying out the construction of eco-farms to reduce environmental pollution (Floress et al., 2017), and China is also vigorously promoting eco-agriculture technology, but has not yet achieved the expected results. The results of available studies show that farmers’ awareness of eco-agriculture technology is low and fewer farmers adopt eco-agriculture technology. What is the motivation of farmers, as the main adopters of ecological agricultural technologies? How does intention arise? What are the factors that enhance (or hinder) the formation of willingness? The answers to these questions will help the government to formulate reasonable policies and incentives to increase farmers’ motivation to adopt agroecological technologies.
Existing studies on the factors influencing the adoption of EAT by farmers have found that farmers’ intention to adopt is influenced by a combination of subjective and objective factors. Objective factors include social learning and social networks, socioeconomic factors, price factors, immediate conditions and social climate, green technology adoption risks and government regulation, the share of farmers’ income in total household income and economic benefits of ecological production technologies, technological environment, plot elements and access to raw materials (Connor et al., 2021; Li et al., 2021; Ruzzante et al., 2021; Sapbamrer and Thammachai, 2021; Shang et al., 2021; Wang et al., 2021). The subjective factors are ecological compensation perception and ecological environment perception, ecological compensation intention, environmental crisis awareness, ecological environment improvement effect, and ecological environment policy perception (Savari et al., 2021; Tama et al., 2021). The above literature combines the subjective and objective factors that affect farmers’ intention to adopt, but less often combines the two theories to study the relationship between the subjective and objective factors and the impact on farmers’ intention.
Regarding the study’s theoretical basis, some scholars have studied farmers’ intention to adopt from the theory of planned behavior (TPB), technology acceptance model (TAM), or a combination of both (Adnan et al., 2019; Ataei et al., 2021; Bakker et al., 2021; Damalas, 2021; Nguyen et al., 2021). Some studies suggest that the behavioral attitudes (BA), subjective norms (SN) and perceptual behavioral control (PBC) in the TPB directly and significantly affect the intention to produce ecologically, while others suggest that the above factors indirectly affect farmers’ intention through influencing their perceptions and moderated by environmental regulations; perceived usefulness (PU), perceived ease of use (PEOU) and SN are the key factors affecting farmers’ intention to adopt, and the effect of PU on farmers with different endowments is There is no difference in PEOU, but some scholars believe that PU significantly affects farmers’ intention, and the effect of PEOU is not significant. Thus, it can be seen that there are different conclusions based on the TAM and TPB for the analysis of the influence of farmers’ intention, i.e., whether there is a direct or indirect influence of the same factor on farmers’ intention and whether its influence is significant are controversial.
In summary, the research results on the factors influencing farmers’ intention to adopt EAT are extensive and have important implications for improving the adoption mechanism of farmers’ rice and shrimp crop technologies, but there is still some room for progress: first, from the theoretical basis of the study, TPB studies the formation of a behavioral intention, while TAM measures the influencing factors of farmers’ acceptance of new technologies, which is a very flexible system. For the accuracy of the analysis, this paper combines the two and extends the variables horizontally to analyze the detailed link between farmers’ behavioral intention and the influencing factors of accepting new technologies, and then distinguishes the direct and indirect influencing effects of intention to adopt. Second, in terms of the object of study, few studies have applied TAM-TPB to the study and analysis of farmers’ rice-shrimp crop technology, which is an element of agroecological technology that is receiving increasing attention. Therefore, based on the research data from Anhui, Hubei, and Hunan provinces in 2020–2021, this paper combines TAM and TPB from a social psychological perspective and uses partial least squares structural equation modeling (PLS-SEM) to analyze the research data, focusing on the influence of subjective factors on farmers’ intention to adopt rice-shrimp coppicing technology. In doing so, the configuration path of high technology intention was further investigated by the fsQCA method.
TPB is a theory by Ajzen (1991) based on rational behavior theory and multi-attribute attitude theory to add human perceptual control over behavior results, which studies how people change their behavior patterns and believes that human behavior is the result of thoughtful planning and intention indirectly affects behavior, mainly including SN, BA, perceptual behavior control, behavioral intention, and behavior results. TPB has a good openness, and the introduction of other variables that have a significant impact on intention can further enhance the explanatory strength of the model based on the traditional model. For example, Bamberg’s study demonstrated that past behaviors can influence individuals’ intention to perform similar behaviors (Bamberg et al., 2003), and Conner further pointed out that the inclusion of past behaviors in TPB increased the explanatory strength of intention by 7% (Conner and Armitage, 1998). Drawing on these findings, this study extends the traditional TPB to explore how the inclusion of past habits will affect individual attitudes, perceived behavioral control, and intention formation.
Subjective norms (SN) are the pressures felt by individuals to perform or not perform a behavior, and this pressure comes primarily from individuals or groups that have influence over their behavioral decisions. In other words, individuals usually prefer to align themselves with the expectations of the reference group. In general, the more positive the individual or group with whom the individual is close to perform a behavior, the more willing the individual is to perform the behavior; conversely, the less willing the individual is to perform the behavior (Lynne et al., 1995). Cook et al. (2021) showed that social expectations from family, friends, or important colleagues, for example, had a significant positive effect on farmers’ behavioral intentions to reduce fertilizer application. This was also confirmed by Arli et al. (2018) in their study on green product purchase intentions. In the practice of agroecological technologies, the adoption of agroecological technologies by village officials and relatives and friends may have a demonstration effect due to farmers’ own knowledge and judgment, which in turn may increase farmers’ willingness to adopt agroecological technologies (Bayes and Druckman, 2021). Therefore, it is hypothesized:
HYPOTHESIS H1. SN has a significant positive effect on farmers’ intention to adopt EAT, and the stronger the influence of SN, the more likely farmers will be an intention to adopt.Attitude refers to an individual’s perception and evaluation of whether it is favorable or unfavorable to perform a particular behavior. Generally, if farmers have higher awareness and more positive evaluation of ecological farming technologies, their willingness to adopt ecological farming models will be higher; conversely, their subjective willingness to adopt ecological farming models will be lower (Vu et al., 2022). A study by Rezaei et al. (2019) showed that farmers’ attitudes and perceptions of integrated pest management technologies are the most important factors influencing technology adoption. Floress et al. (2017) found that farmers’ perceptions significantly increased the willingness to adopt abatement technologies, and that each unit increase in perceived ability contributed 25.5% to low-carbon technology adoption. Theoretically, the application of eco-agricultural technologies can not only achieve comprehensive and effective use of resources, reduce environmental pollution, and generate ecological benefits, but also produce pollution-free and high-quality green products, which bring economic benefits to farmers. Therefore, when farmers perceive that EAT are useful and can bring positive results, they will have a higher willingness to adopt them. Therefore, it is hypothesized:
HYPOTHESIS H2. BA has a significant positive effect on farmers’ intention to adopt EAT, i.e., the more positive BA, the more likely farmers are to adopt the technology.PBC refers to the ease with which individuals can master resources and is often influenced by condition assessment and perceived ability (Adnan et al., 2018). Farmers’ intention to adopt rice-shrimp crop technology is limited by their conditions and the abundance of resources. From the perspective of condition assessment, farmers often need certain human resources to adopt rice-shrimp crop technology. Before forming the intention to adopt, farmers will self-assess the adequacy of household labor, to measure whether they can divest part of their surplus labor to engage in rice-shrimp crop technology based on existing agricultural production, which ensures that they can engage in new products based on gaining previous agricultural production to reduce the risk of uncertainty and loss. Therefore, the more abundant the household labor force is, the more likely it is to divest a certain amount of labor to try out the new technology, and the stronger its intention to adopt it. From the perspective of ability perception, before making behavioral decisions, individuals perceive their ability and compare it with the ability required to learn the new technology, and only when the ability is higher than the ability required to learn the technology will they be an intention to adopt it. The riskiness of new technology directly affects the ability and resourcefulness required to master the technology. Therefore, it is hypothesized:
HYPOTHESIS H3. PBC has a significant positive effect on farmers’ intention to adopt EAT, and the stronger the PBC, the more likely farmers are to adopt it.
TAM emphasizes that intention influences behavior and intention is influenced by attitude, but Davis proposes the concepts of PU and PEOU and argues that PU and PEOU not only directly influence intention but also influence attitude and indirectly influence intention through attitude (Lee et al., 2003).
PU refers to the number of dividends that an individual believes can be brought to him or her by adopting a certain behavior. Before farmers are intending to adopt rice-shrimp crop technology, they will measure the expected benefits and value of the technology to measure whether the technology is useful for them. The expectation theory shows that when the benefits of adopting the technology are greater than the costs, meaning that the farmer can get economic satisfaction from the technology, then the technology is useful for the farmer, otherwise, it is useless (Suvedi et al., 2017). If farmers perceive that the benefits of the technology are expected to be high enough, they will subjectively form the idea that the technology is worth trying and can bring them the incentives they need to grow and develop, and they will be an intention to adopt it, so PU will have a direct impact on farmers’ intention to adopt it.
When individuals perceive that implementing a behavior is useful and brings resources to their development, they will investigate and learn more to determine whether they should implement the behavior (Atkins et al., 2017). When farmers learn more about the benefits of rice-shrimp crop technology, they find the technology promising and develop a positive attitude toward it. Based on the previous analysis, this positive behavioral attitude will lead to the intention to adopt, so the PU will have a direct impact on farmers’ behavioral attitude, and indirectly affect the intention to adopt through the influence of attitude. Therefore, it is hypothesized:
HYPOTHESIS H4. PU positively and significantly affects farmers’ intention to adopt, and the greater the PU, the stronger farmers’ intention to adopt.
HYPOTHESIS H5. PU indirectly affects farmers’ intention to adopt by using BA as a mediating variable, and the greater the PU, the more positive behavioral attitude, the stronger farmers’ intention to adopt.PEOU refers to how easy an individual perceives it is to perform a behavior; the easier an individual perceives a technique to be to master, the more likely they are to adopt that behavior. Based on traditional smallholder theory, most people tend to try simpler technologies than more complex ones, which is close to the conservative mentality. Therefore, the simpler and easier farmers perceive the technology of rice and shrimp farming, the more confident they will be in mastering the technology and the more likely they are to adopt it, so the PEOU has a direct impact on farmers’ intention to adopt it.In addition, from the input side, the high difficulty of mastering the technology means that farmers have to take risks and pay more costs to learn the technology, while on the contrary, the costs are relatively low, and farmers are likely to have an initial positive BA, thus inducing them to learn more about the technology and make adoption decisions. In terms of benefits, farmers try new technology to gain more benefits. The simplicity of the technology means that farmers are more sure of mastering the technology and gaining the dividends of the technology, forming positive BA, and then giving more knowledge and examination to the technology, which finally influences farmers’ adoption decisions. Based on the previous analysis, PEOU affects farmers’ BA, and BA affects farmers’ intention to adopt. Therefore, PEOU will indirectly influence farmers’ adoption intention through BA. Therefore, it is hypothesized:
HYPOTHESIS H6. PEOU positively and significantly affects farmers’ adoption intention, and the stronger the PEOU, the stronger farmers’ adoption intention.
HYPOTHESIS H7. PEOU indirectly affects farmers’ adoption intention by BA as a mediating variable, and the greater the PU, the more positive the behavioral attitude, the stronger farmers’ adoption intention.
Related studies have shown that behavioral decisions are the result of a combination of individual consideration of their cognition, environmental factors, and expected effects (Ji et al., 2019). Both TPB and TAM are derived from the Theory of Rational Behavior, which creates a basis for the integration of the two analyses. PU and PEOU in TAM are only some of the components of farmers’ awareness, but farmers’ awareness is the main force that influences their BA, so PU and PEOU have an impact on farmers’ attitudes, which in turn, work together to influence farmers’ intention to adopt. It has been shown that the combination of TPB and TAM is more reasonable and scientific than analyzing TPB or TAM alone (Chih Chung, 2013; Hossain et al., 2019), so this paper combines TAM and TPB and proposes the following theoretical framework (Figure 1).
The data for this paper come from a questionnaire survey conducted by the research team in Anhui, Hubei, and Hunan provinces in 2020–2021, and the research program uses a combination of stratified and random sampling to select the sample farmers. The first stage targeted the central grain-producing regions, i.e., sample counties were selected in the three provinces in the middle and lower reaches of the Yangtze River according to factors such as paddy grain and geographical location; the second stage aimed to find townships with large grain production with the help of relevant agricultural departments in the sample counties; the third stage was to find typical farmers in the selected townships. Finally, Changfeng, Huoqiu, and Quanjiao counties in Anhui province, Chibi, Xianning, Qianjiang, and Huanggang cities in Hubei province, and Anxiang, Linxiang, and Yiyang cities in Hunan province were selected as the sample areas. The group selected these study areas because, first, Hubei, Hunan, and Anhui provinces are located in the middle and lower reaches of the Yangtze River, which is rich in water and fertile soil and is an important food producer in China, providing space for the development of rice-shrimp crop technology; second, according to previous research findings, rice-shrimp crop technology is more mature in the region and the sample data are more representative. The content of this survey focused on the technical sources and communication, agricultural inputs and outputs, and ecological production behavior of rice-shrimp crop households. A total of 425 questionnaires were obtained, and after eliminating invalid samples, 295 valid samples were obtained, with an effective rate of 69%.
A questionnaire was designed, including 1) demographic characteristics; 2) evaluation of EAT adoption predictors; and 3) evaluation of EAT adoption implications. Each construct was measured using a validated research instrument developed by previous studies (modified to fit the research context) and based on the literature review findings and the theoretical foundation. A seven-point Likert scale was used to overcome measurement errors. The Likert scale ranges from “strongly disagree” (i.e., 1) to “strongly agree” (i.e., 7). Supplementary Table S1 in the Appendix Survey items describes the used survey items.
1. Potential biases were considered in the survey, protocol design, and data analysis. Several approaches (e.g. direct contact by phone and assurance to share the results) were adopted to ensure the highest response rate and avoid a non-response bias (Frohlich and Westbrook, 2002). To test the research model and answer our research questions, we used both PLS-SEM and fsQCA. SmartPLS3.0 and fsQCA3.0 were used for the analyzes. The partial least squares structural equation (PLS-SEM) was chosen for the study method, and the specific reasons for using this method are as follows (Hair et al., 2019): 1) when the analysis is concerned with testing a theoretical framework from a prediction perspective; 2) when the structural model is complex and includes many constructs, indicators and/or model relationships; 3) when the research objective is to better understand increasing complexity by exploring theoretical extensions of established theories (exploratory research for theory development); 4) when the research is based on secondary/archival data, which may lack a comprehensive substantiation on the grounds of measurement theory; 5) when a small population restricts the sample size (e.g., business-to-business research); but PLS-SEM also works very well with large sample sizes. This technique has been adopted because this process gives better results in the analysis of this type of exploratory study. This process can also analyze those data that are not normally distributed (Hair et al., 2012). This technique does not impose any sample restriction to conduct the survey. This process involves the quantification of responses on a specific scale. The fsQCA analysis follows the configuration theory paradigm, which enables the examination of holistic interplays between elements of a messy and non-linear nature.
The results of the measurement (based on confirmatory factor analysis) and structural (based on bootstrapping) models and fsQCA are reported in the following sections.
The reflective constructs were validated by testing internal consistency, composite reliability, convergent, and discriminant validity (Table 1). To verify the internal consistency and composite reliability of the constructs, we verified that the value of Cronbach s alpha and composite reliability indices exceeded 0.7 (Hair et al., 2011). This condition was valid for all the constructs. To test convergent validity, we verified that the average variance extracted (AVE) index was greater than 0.5. The lowest observed value (0.597) was substantially higher than this threshold. The discriminant validity of the reflective constructs was tested in three ways (Fornell and Larcker, 1981). The correlation matrix proved that the AVE was greater than the square correlation between each pair of latent constructs. These results suggest the validity of the reflective constructs used in our analysis and the adequacy of the items used as construct indicators.
TABLE 1. Construct consistency, reliability, convergent and discriminant validity squared value of the AVE reported on the main diagonal of the correlation matrix.
Table 2 shows the results of the structural model from the PLS analysis, including standardized path coefficients with two-tailed t-tests for the hypotheses and the posthoc tests for testing the mediation effect of BA.
The results partially confirm the hypotheses proposed by the research model (Figure 1). The path analysis confirms that SN (H1: β = 0.251, p < 0.001), BA (H2: β = 0.106, p < 0.05), PBC (H3: β = 0.172, p < 0.05), PU (H4: β = 0.154, p < 0.001) and PEOU (H5: β = 0.297, p < 0.001) all positively impact IAE. Although both PU (β = 0.115, p < 0.05) and PEOU (β = 0.497, p < 0.001) positively impact BA, the mediation effect is present only for the case of PEOU, as the post-hoc test for the indirect effect is statistically significant for the path PEOU not for the path PU→BA→IAE (β = 0.012, p > 0.05), but PEOU→BA→IAE (β = 0.053, p < 0.05).
The structural model explained a variance rate of 0.31 for BA and 0.595 for SCA. These values can be considered as the predictive accuracy of the models between moderate and strong (Hair et al., 2019). The analysis of the composite-based standardized root means square residual (SRMR) yielded a value of 0.042, below the 0.10 threshold, which confirms the robustness of the model (Henseler et al., 2015).
First, we need to calibrate the data before we can start fsQCA analysis. Membership ranges from 0 to 1 (Fiss, 2011), where one represents a Full membership, 0 represents a complete non-membership, and 0.5 represents the vaguest crossover point. This study follows the studies of Greckhamer (2016), Delmas and Pekovic (2018), using membership values of 0.95, 0.5, and 0.05 of the results and conditions to determine the complete membership points, intersection points, and complete non-membership points. The original data is converted into the fuzzy score by Calibrate formula. It is useful to check necessary and sufficient conditions before performing truth table analysis of fuzzy sets. The results in table 3 show that the adequacy consistency rate and necessity coverage rate of each antecedent condition is lower than the identification standard of 0.9, that is, a single antecedent condition does not constitute a sufficient and necessary condition for IAE, which means that the influence of condition configuration on IAE needs to be investigated.
Second, we then performed a sufficiency analysis using the fsQCA algorithm to produce the truth table (Ragin, 2014). To avoid including less significant configurations, a five-observation frequency threshold was adopted, which caused the exclusion of thirty-four cases in the sample. Our analysis produced four possible solutions leading to a high level of IAE. The complex solution is the solution without any simplification, so there may be multiple paths, which is not conducive to the subsequent path analysis; the parsimonious solution may ignore the necessary conditions that lead to the result; the intermediate solution is the solution between the complex solution and the parsimonious solution, most scholars prefer to use the intermediate solution for the path analysis, and the intermediate solution is also used for the path analysis in this study. According to the operation results of fsQCA 3.0 software shown in Table 4, Our analysis produced four possible solutions leading to a high level of IAE., and the overall solution coverage reaches 0.737, indicating that the coverage rate of the four configurations has a high explanatory power to the results.
Solution 1, with high levels of ∼PU, SN, BA, and PBC, had the lowest consistency (0.912) and explained the lowest number of cases (coverage = 0.406). It had the highest unique coverage (0.109), indicating that the combination of high levels of SN, BA, and PBC mostly contributed to high levels of IAE in the absence of PU compared to all other solutions.
Solution 2, with high levels of PU, PEOU, SN, and BA, had the lowest unique coverage (0.031) and explained the lower number of cases (coverage = 0.538). It had the higher Consistency (0.938), indicating that the combination of high levels of PU, PEOU, SN, and BA mostly contributed to high levels of IAE compared to all other solutions.
Solution 3, with high levels of PU, PEOU, SN, and PBC had the highest consistency (0.945) and explained the highest number of cases (coverage = 0.558). It also had higher unique coverage (0.051), indicating that the combination of high levels of PU, PEOU, SN, and PBC mostly contributed to high levels of IAE compared to all other solutions.
Solution 4, with high levels of PU, PEOU, BA, and PBC had the higher consistency (0.938) and explained the higher number of cases (coverage = 0.546). It also had higher unique coverage (0.039), indicating that the combination of high levels of PU, PEOU, BA, and PBC mostly contributed to high levels of IAE compared to all other solutions.
The combination of perceived usefulness and perceived ease of use in TAM and any other two factors in TPB can constitute a path to high IAE for farmers, as found by path comparison combinations 2–4. When farmers perceive EAT to be useless, the path to high IAE can only be strengthened by the three factors in TPB, regardless of whether EAT is easy or not. Therefore, the TAM and TPB frameworks constructed in this study can mutually promote high IAE. The comparative analysis reveals that the formation of high IAE in the context of green agriculture is caused by multiple different antecedent condition configurations, and whether a single causal condition exists in a particular configuration depends on other causal conditions. In addition, each pathway often does not exist in isolation, and different equivalent causal chains are complementary and alternate.
Based on the extended TAM-TPB integrated analysis framework, PLS-SEM and fsQCA were used to analyze the influence mechanism of agroecological technology adoption willingness from two perspectives of technology acceptance-planning behavior and configuration influence, and the following main findings were obtained.
Firstly, by integrating TAM and TPB into a unified analytical framework, we explained the formation mechanism of farmers’ willingness to adopt agroecological technologies from the dual perspectives of farmers’ technology acceptance and technology adoption behavior, and found that the formation of farmers’ willingness to adopt agroecological technologies is influenced by the dual motives of technology ease of use and technology usefulness, and that ease of use indirectly influences farmers’ attitude toward using and thus their willingness to use.
Secondly, in TPB, attitudes, perceived behavioral control, and subjective norms all have positive effects on farmers’ willingness to adopt agroecological technologies, but there are differences in the magnitude of their effects, with subjective norms having the greatest effect on willingness and perceived behavioral control having a higher effect than attitudes.
Thirdly, the fsQCA study identified four pathways that generate high willingness to adopt agroecological technologies. Also, it was demonstrated that perceived usefulness, perceived ease of use, subjective norms, perceived behavioral control, and attitudes have a direct effect on willingness to adopt technology.
Drawing on TAM and TPB, this study proposes a research model in which BA mediates the effect of PEOU on IAE, thus contributing to the understanding of the feasibility of the technology to develop green agriculture (Balafoutis et al., 2020). Therefore, we propose seven hypotheses, including five direct effects, namely SN on IAE, BA on IAE, PBC on IAE, PU on IAE, and PEOU on IAE. Similarly, we assume that PPU and PEOU have two indirect effects on IAE through BA. In addition to PLS-SEM analysis, we applied fsQCA to investigate how the antecedents of IAE (PU, PEOU, SN, BA, PBC) contribute to IAE. The findings of PLS-SEM and fsQCA emphasize that farmers’ external technical awareness (TAM) and internal use understanding (TPB) of EAT, and are alternative and complementary to each other.
The results show that SN positively influences IAE, thus supporting Hypothesis H1. This signifies that the more farmers perceive that they can master the technology, the easier they perceive it to be to use, and the more intention they are to adopt it. On the one hand, the population in rural areas of China has a strong sense of kinship and neighborhood ties, so mutual communication and observational learning can form a certain group perception, which can reduce the difficulty and risk uncertainty of technical mastery and generate intra-group compliance motivation. On the other hand, communication among farmers and relatives, and friends in the village can bring each other a sense of pleasure in communication, which can generate a sense of self-efficacy and enhance the intention to adopt.
The results show that BA positively influences IAE, thus supporting Hypothesis H2. The more farmers understand the technology, the more positive their attitudes, the stronger their behavioral attitudes, the more likely they are to adopt it. The behavioral attitudes of farmers towards rice and shrimp crop technology include the evaluation of the economic, ecological, and social aspects, and only based on sufficient understanding of the technology, they can form a rational understanding and make an objective and comprehensive evaluation, thus forming a strong belief in the results. Even though the technology is profitable, farmers will not try things they do not understand, and their attitudes towards things they do not understand tend to be ignorant or negative.
The results show that PBC positively influences IAE, thus supporting Hypothesis H3. The more positive the farmers’ condition assessment, the stronger their perceived ability, the stronger their perceptual behavior control, and the stronger their intentions to adopt. For farmers who depend on land for their livelihood, the most important resource is land, the direct condition for maximizing the allocation of land resources is the labor force itself, and one of the subjective influences on how to use land resources is the farmers’ risk perception. One of the subjective influences that determine how land resources are used is farmers’ perception of risk. Risk is often accompanied by reward, and only when these conditions are present will farmers’ perceived behavioral control be stronger and their intentions to adopt be enhanced.
The results show that PU positively influences IAE, thus supporting Hypothesis H4. The higher the perceived benefits of the technology, the stronger the perceived usefulness, and the stronger the intention to adopt it. In recent years, the government has been increasing its efforts to promote the ecological agriculture technology of rice and shrimp farming, so that farmers feel the great benefits of this technology and form a sense of recognition, and their perceived usefulness is also greatly improved, and the stronger the perceived usefulness of farmers, the stronger their intention to adopt it.
The results show that PEOU positively influences IAE, thus supporting Hypothesis H5. The more farmers perceive themselves to be able to master the technology, the stronger the perceived ease of use, and the stronger their intentions to adopt it. Since rice-shrimp farming technology is subject to high natural risks, it requires farmers to have certain farming and cultivation experience. When farmers believe that they can fully master rice-shrimp farming technology or receive technical support, the greater the perceived ease of use, the higher the intention to adopt it.
Hypothesis H6 was not validated. When farmers believe that the technology has benefits, they will be influenced by other farmers’ evaluations of the technology and become anxious and skeptical, which will have a negative impact on the farmers’ behavior and attitude toward IAE.
Hypothesis H7 was validated. Due to curiosity, when farmers perceive that the technology is easy to master, they will learn more about the technology and thus have an impact on behavioral attitudes, which in the previous analysis had a significant positive effect on the intention to adopt. Based on the above analysis, perceived ease of use has an indirect effect on the intention to adopt and has a direct effect on behavioral attitude with behavioral attitude as a mediating variable. Perceived usefulness does not have an indirect effect.
More specifically, we applied fsQCA to gauge the combinatory conditions by which PU, PEOU, SN, BA, and PBC interact to predict IAE. The findings of fsQCA (asymmetric) analysis revealed that the study s independent variables (PU, PEOU, SN, BA, and PBC) were all necessary to escalate IAE, but they were not in themselves sufficient.
This study has been able to theorize the SN, BA, and PBC in farmers to unlock the development path of sustainable EAT. This study has also theorized that such EAT is compatible in the context of green development (Shen et al., 2021; Ataei et al., 2022). For this, the help of a hybrid model, i.e., the integrated TAM-TPB model, has been taken. This hybrid model has been successfully used to explore farmers’ intention to adopt EAT. Since few research studies are available to nurture the use of EAT in farmers. This study provides a novel contribution in this context. The present study also demonstrates the research in the face of new technology acceptance models (Chaiyasoonthorn et al., 2019; Chaveesuk et al., 2020). This study has examined how BA poses an impediment to adopting new technology in farmers, and it has been explained through this study how the TAM-TPB framework will help to interpret the situation. The reasons for using the TAM-TPB-based integrated model have been explained in the theoretical background section. This study aims to explain what factors could influence the intention to adopt EAT, a component of green development. This study analyses the adoption of EAT in farmers. In this context, this study could have used an updated standard adoption model. However, the study has ventured to select better-suited antecedents from TAM-TPB-based integrated models.
First, it is suggested that relevant departments link up to jointly promote knowledge of ecological agriculture technology, introduce to farmers the learning steps and expected benefits of ecological agriculture technology, enhance farmers’ awareness of the economic, social and ecological benefits of the technology, improve farmers’ perceived utility, and achieve the effect of changing farmers’ perception of ecological agriculture technology, thus improving farmers’ behavioral attitudes toward ecological agriculture technology.
Second, it is recommended that relevant technical departments establish a communication network with farmers in villages for regular contact, and provide farmers with timely technical interpretation and assistance through agricultural extension staff to reduce the perceived difficulty of farmers in learning eco-agricultural technology. Build a production exchange platform for farmers, strengthen the role of neighborhood demonstration, and form a rural mutual aid system to solve the problems of agricultural sources and technology learning, improve perceived ease of use, and enhance farmers’ confidence in mastering technology. Thirdly, it is recommended to improve the level of farmers’ social networks and optimize the structure of farmers’ resource endowment by category. In terms of social endowment, social institutions and social resources should be adopted to strengthen the social network of farmers; in terms of economic endowment, government subsidies for ecological agriculture technology should be strengthened to increase the economic benefits of farmers’ adoption of ecological agriculture technology; in terms of human endowment, the government and social institutions can provide free personnel to explain the means to enrich farmers’ resource endowment and enhance the control of farmers’ perceptual behavior. By increasing the level of various types of endowments of farmers to enrich their resources and enhance their risk resistance, farmers can improve their perceptual behavior control and exert the influence of subjective norms.
The specificity of the sample. The sample selected for this study is mainly from villages in the middle and lower reaches of the Yangtze River, which is reasonable but has some limitations. For example, the sample does not cover a wide range of farmers who do not use agroecological technologies, and the sample does not fully represent the psychological dynamics of farmers’ intention to adopt ecological agricultural technologies. Therefore, we need to accumulate valid samples and conduct a more extensive survey to make the research findings more generalizable.
In the future, this study will be conducted in the present agricultural complex in the form of an organization using the Technology-Organization-Environment (TOE) framework to conduct a complementary study to deeply explore the willingness of the organization and individuals in the organization to adopt eco-agricultural technologies.
The original contributions presented in the study are included in the article/Supplementary Material, further inquiries can be directed to the corresponding author.
Ethics review and approval/written informed consent was not required as per local legislation and institutional requirements
HD and JCH; formal analysis, HD and HYW; resources and data curation, HD and JCH; investigation, HD; writing-original draft preparation, HYW; writing-review and editing, HD and JCH; supervision and project administration, HD; All authors have read and agreed to the published version of the manuscript.
This research was funded by the Humanities and Social Sciences Project of the Ministry of Education in 2019: A comparative research on Sino-German vocational education integration of industry and education and inheritance of craftsman spirit (19YJC880010, Ministry of Education, China).
Author HD is employed by Shaanxi Provincial Land Engineering Construction Group Co., Ltd.
The remaining authors declare that the research was conducted in the absence of any commercial or financial relationships that could be construed as a potential conflict of interest.
All claims expressed in this article are solely those of the authors and do not necessarily represent those of their affiliated organizations, or those of the publisher, the editors and the reviewers. Any product that may be evaluated in this article, or claim that may be made by its manufacturer, is not guaranteed or endorsed by the publisher.
The Supplementary Material for this article can be found online at: https://www.frontiersin.org/articles/10.3389/fenvs.2022.927668/full#supplementary-material
Adnan, N., Nordin, S. M., and Ali, M. (2018). A Solution for the Sunset Industry: Adoption of Green Fertiliser Technology Amongst Malaysian Paddy Farmers. Land Use Policy 79, 575–584. doi:10.1016/j.landusepol.2018.08.033
Adnan, N., Nordin, S. M., Bahruddin, M. A., and Tareq, A. H. (2019). A State-Of-The-Art Review on Facilitating Sustainable Agriculture through Green Fertilizer Technology Adoption: Assessing Farmers Behavior. Trends Food Sci. Technol. 86, 439–452. doi:10.1016/j.tifs.2019.02.040
Ajzen, I. (1991). The Theory of Planned Behavior. Organ. Behav. Hum. Decis. Process. 50, 179–211. doi:10.1016/0749-5978(91)90020-T
Arli, D., Tan, L. P., Tjiptono, F., and Yang, L. (2018). Exploring Consumers' Purchase Intention towards Green Products in an Emerging Market: The Role of Consumers' Perceived Readiness. Int. J. Consum. Stud. 42, 389–401. doi:10.1111/ijcs.12432
Ataei, P., Gholamrezai, S., Movahedi, R., and Aliabadi, V. (2021). An Analysis of Farmers' Intention to Use Green Pesticides: The Application of the Extended Theory of Planned Behavior and Health Belief Model. J. Rural Stud. 81, 374–384. doi:10.1016/j.jrurstud.2020.11.003
Ataei, P., Karimi, H., Moradhaseli, S., and Babaei, M. H. (2022). Analysis of Farmers' Environmental Sustainability Behavior: the Use of Norm Activation Theory (A Sample from Iran). Arab. J. Geosci. 15, 1–13. doi:10.1007/s12517-022-10042-4
Atkins, L., Francis, J., Islam, R., O’Connor, D., Patey, A., Ivers, N., et al. (2017). A Guide to Using the Theoretical Domains Framework of Behaviour Change to Investigate Implementation Problems. Implement. Sci. 12, 77. doi:10.1186/s13012-017-0605-9
Bakker, L., Sok, J., van der Werf, W., and Bianchi, F. J. J. A. (2021). Kicking the Habit: What Makes and Breaks Farmers' Intentions to Reduce Pesticide Use? Ecol. Econ. 180, 106868. doi:10.1016/j.ecolecon.2020.106868
Balafoutis, A. T., Evert, F. K. V., and Fountas, S. (2020). Smart Farming Technology Trends: Economic and Environmental Effects, Labor Impact, and Adoption Readiness. Agronomy 10, 743. doi:10.3390/agronomy10050743
Bamberg, S., Ajzen, I., and Schmidt, P. (2003). Choice of Travel Mode in the Theory of Planned Behavior: The Roles of Past Behavior, Habit, and Reasoned Action. Basic Appl. Soc. Psychol. 25, 175–187. doi:10.1207/S15324834BASP2503_01
Bayes, R., and Druckman, J. N. (2021). Motivated Reasoning and Climate Change. Curr. Opin. Behav. Sci. 42, 27–35. doi:10.1016/j.cobeha.2021.02.009
Chaiyasoonthorn, W., Khalid, B., and Chaveesuk, S. (2019). “Success of Smart Cities Development with Community's Acceptance of New Technologies,” in Proceedings of the 9th International Conference on Information Communication and Management-ICICM 2019, August 23–26, 2019 (Prague: Czech Republic). doi:10.1145/3357419.3357440
Chaveesuk, S., Chaiyasoonthorn, W., and Khalid, B. (2020). “Understanding the Model of User Adoption and Acceptance of Technology by Thai Farmers,” in Proceedings of the 2020 2nd International Conference on Management Science and Industrial Engineering, April 7–9, 2020 (Osaka, Japan: ACM), 279–285. doi:10.1145/3396743.3396781
Chih Chung, C. (2013). The Exploration on Network Behaviors by Using the Models of Theory of Planned Behaviors (TPB), Technology Acceptance Model (TAM) and C-TAM-TPB. Afr. J. Bus. Manage. 7, 2976–2984. doi:10.5897/AJBM11.1966
Conner, M., and Armitage, C. J. (1998). Extending the Theory of Planned Behavior: A Review and Avenues for Further Research. J. Appl. Soc. Pyschol 28, 1429–1464. doi:10.1111/j.1559-1816.1998.tb01685.x
Connor, M., Tuan, L. A., DeGuia, A. H., and Wehmeyer, H. (2021). Sustainable Rice Production in the Mekong River Delta: Factors Influencing Farmers' Adoption of the Integrated Technology Package "One Must Do, Five Reductions" (1M5R). Outlook Agric. 50, 90–104. doi:10.1177/0030727020960165
Cook, B. R., Satizábal, P., and Curnow, J. (2021). Humanising Agricultural Extension: A Review. World Dev. 140, 105337. doi:10.1016/j.worlddev.2020.105337
Damalas, C. A. (2021). Farmers' Intention to Reduce Pesticide Use: the Role of Perceived Risk of Loss in the Model of the Planned Behavior Theory. Environ. Sci. Pollut. Res. 28, 35278–35285. doi:10.1007/s11356-021-13183-3
de Lima Vieira, J., dos Santos Nunes, L., Rodrigues de Menezes, F. G., Vieira de Mendonça, K., and Viana de Sousa, O. (2021). An Integrated Approach to Analyzing the Effect of Biofloc and Probiotic Technologies on Sustainability and Food Safety in Shrimp Farming Systems. J. Clean. Prod. 318, 128618. doi:10.1016/j.jclepro.2021.128618
Delmas, M. A., and Pekovic, S. (2018). Organizational Configurations for Sustainability and Employee Productivity: A Qualitative Comparative Analysis Approach. Bus. Soc. 57, 216–251. doi:10.1177/0007650317703648
Fiss, P. C. (2011). Building Better Causal Theories: A Fuzzy Set Approach to Typologies in Organization Research. Acad. Manag. J. 54, 393–420. doi:10.5465/amj.2011.60263120
Floress, K., García de Jalón, S., Church, S. P., Babin, N., Ulrich-Schad, J. D., and Prokopy, L. S. (2017). Toward a Theory of Farmer Conservation Attitudes: Dual Interests and Willingness to Take Action to Protect Water Quality. J. Environ. Psychol. 53, 73–80. doi:10.1016/j.jenvp.2017.06.009
Fornell, C., and Larcker, D. F. (1981). Evaluating Structural Equation Models with Unobservable Variables and Measurement Error. J. Mark. Res. 18, 39. doi:10.2307/3151312
Frohlich, M. T., and Westbrook, R. (2002). Demand Chain Management in Manufacturing and Services: Web-Based Integration, Drivers and Performance. J. Operations Manag. 20, 729–745. doi:10.1016/S0272-6963(02)00037-2
Greckhamer, T. (2016). CEO Compensation in Relation to Worker Compensation across Countries: The Configurational Impact of Country-Level Institutions. Strat. Mgmt. J. 37, 793–815. doi:10.1002/smj.2370
Hair, J. F., Ringle, C. M., and Sarstedt, M. (2011). PLS-SEM: Indeed a Silver Bullet. J. Mark. Theory Pract. 19, 139–152. doi:10.2753/MTP1069-6679190202
Hair, J. F., Sarstedt, M., Ringle, C. M., and Mena, J. A. (2012). An Assessment of the Use of Partial Least Squares Structural Equation Modeling in Marketing Research. J. Acad. Mark. Sci. 40, 414–433. doi:10.1007/s11747-011-0261-6
Hair, J. F., Risher, J. J., Sarstedt, M., and Ringle, C. M. (2019). When to Use and How to Report the Results of PLS-SEM. Eur. Bus. Rev. 31, 2–24. doi:10.1108/EBR-11-2018-0203
Henseler, J., Ringle, C. M., and Sarstedt, M. (2015). A New Criterion for Assessing Discriminant Validity in Variance-Based Structural Equation Modeling. J. Acad. Mark. Sci. 43, 115–135. doi:10.1007/s11747-014-0403-8
Hossain, R., Hasan Mahmud, S. M., Hossin, M. A., Bhuiyan, T., and Hua, Y. X. (2019). “Effects of Cognitive Ability, Trust and Time-Saving: Predicting Further Amelioration and Successive Usage of E-Ticketing with TAM, TPB and Cognitive Frameworks,” in Information and Communication Technology for Competitive Strategies (Springer), 41–51. doi:10.1007/978-981-13-0586-3_5
Huang, J. (2021). “Innovations in Agricultural Technologies in China,” in From Food Scarcity to Surplus (Springer), 83–135. doi:10.1007/978-981-15-9484-7_4
Ji, C., Jin, S., Wang, H., and Ye, C. (2019). Estimating Effects of Cooperative Membership on Farmers' Safe Production Behaviors: Evidence from Pig Sector in China. Food Policy 83, 231–245. doi:10.1016/j.foodpol.2019.01.007
Lee, Y., Kozar, K. A., and Larsen, K. R. T. (2003). The Technology Acceptance Model: Past, Present, and Future. Commun. Assoc. Inf. Syst. 12, 1250. doi:10.17705/1CAIS.01250
Li, B., Ding, J., Wang, J., Zhang, B., and Zhang, L. (2021). Key Factors Affecting the Adoption Willingness, Behavior, and Willingness-Behavior Consistency of Farmers Regarding Photovoltaic Agriculture in China. Energy Policy 149, 112101. doi:10.1016/j.enpol.2020.112101
Lynne, G. D., Franklin Casey, C., Hodges, A., and Rahmani, M. (1995). Conservation Technology Adoption Decisions and the Theory of Planned Behavior. J. Econ. Psychol. 16, 581–598. doi:10.1016/0167-4870(95)00031-6
Nguyen, T. P. L., Doan, X. H., Nguyen, T. T., and Nguyen, T. M. (2021). Factors Affecting Vietnamese Farmers' Intention toward Organic Agricultural Production. Int. J. Soc. Econ. 48, 1213–1228. doi:10.1108/IJSE-08-2020-0554
Ragin, C. C. (2014). The Comparative Method: Moving beyond Qualitative and Quantitative Strategies. University of California Press. doi:10.1525/9780520957350
Rezaei, R., Safa, L., Damalas, C. A., and Ganjkhanloo, M. M. (2019). Drivers of Farmers' Intention to Use Integrated Pest Management: Integrating Theory of Planned Behavior and Norm Activation Model. J. Environ. Manag. 236, 328–339. doi:10.1016/j.jenvman.2019.01.097
Rezaei, R., Safa, L., and Ganjkhanloo, M. M. (2020). Understanding Farmers' Ecological Conservation Behavior Regarding the Use of Integrated Pest Management- an Application of the Technology Acceptance Model. Glob. Ecol. Conservation 22, e00941. doi:10.1016/j.gecco.2020.e00941
Ruzzante, S., Labarta, R., and Bilton, A. (2021). Adoption of Agricultural Technology in the Developing World: A Meta-Analysis of the Empirical Literature. World Dev. 146, 105599. doi:10.1016/j.worlddev.2021.105599
Sapbamrer, R., and Thammachai, A. (2021). A Systematic Review of Factors Influencing Farmers' Adoption of Organic Farming. Sustainability 13, 3842. doi:10.3390/su13073842
Savari, M., Zhoolideh, M., and Khosravipour, B. (2021). Explaining Pro-environmental Behavior of Farmers: A Case of Rural Iran. Curr. Psychol. doi:10.1007/s12144-021-02093-9
Shang, L., Heckelei, T., Gerullis, M. K., Börner, J., and Rasch, S. (2021). Adoption and Diffusion of Digital Farming Technologies - Integrating Farm-Level Evidence and System Interaction. Agric. Syst. 190, 103074. doi:10.1016/j.agsy.2021.103074
Shen, S., Li, J., and Xu, R. (2021). Agricultural Ecological Environment Protection Based on the Concept of Sustainable Development. Acta Agric. Scand. Sect. B - Soil & Plant Sci. 71, 920–930. doi:10.1080/09064710.2021.1961852
Spanaki, K., Sivarajah, U., Fakhimi, M., Despoudi, S., and Irani, Z. (2022). Disruptive Technologies in Agricultural Operations: a Systematic Review of AI-Driven AgriTech Research. Ann. Oper. Res. 308, 491–524. doi:10.1007/s10479-020-03922-z
Sun, H., Awan, R. U., Nawaz, M. A., Mohsin, M., Rasheed, A. K., and Iqbal, N. (2021a). Assessing the Socio-Economic Viability of Solar Commercialization and Electrification in South Asian Countries. Environ. Dev. Sustain 23, 9875–9897. doi:10.1007/s10668-020-01038-9
Sun, H., Edziah, B. K., Kporsu, A. K., Sarkodie, S. A., and Taghizadeh-Hesary, F. (2021b). Energy Efficiency: The Role of Technological Innovation and Knowledge Spillover. Technol. Forecast. Soc. Change 167, 120659. doi:10.1016/j.techfore.2021.120659
Suvedi, M., Ghimire, R., and Kaplowitz, M. (2017). Farmers’ Participation in Extension Programs and Technology Adoption in Rural Nepal: a Logistic Regression Analysis. J. Agric. Educ. Ext. 23, 351–371. doi:10.1080/1389224X.2017.1323653
Tama, R. A. Z., Ying, L., Yu, M., Hoque, M. M., Adnan, K. M., and Sarker, S. A. (2021). Assessing Farmers' Intention towards Conservation Agriculture by Using the Extended Theory of Planned Behavior. J. Environ. Manag. 280, 111654. doi:10.1016/j.jenvman.2020.111654
Vu, T., Magis-Weinberg, L., Jansen, B. R. J., van Atteveldt, N., Janssen, T. W. P., Lee, N. C., et al. (2022). Motivation-Achievement Cycles in Learning: a Literature Review and Research Agenda. Educ. Psychol. Rev. 34, 39–71. doi:10.1007/s10648-021-09616-7
Wang, H., Wang, X., Sarkar, A., and Zhang, F. (2021). How Capital Endowment and Ecological Cognition Affect Environment-Friendly Technology Adoption: A Case of Apple Farmers of Shandong Province, China. Int. J. Environ. Res. Public Health 18, 7571. doi:10.3390/ijerph18147571
Xue, Y., Guo, J., Li, C., Xu, X., Sun, Z., Xu, Z., et al. (2021). Influencing Factors of Farmers' Cognition on Agricultural Mulch Film Pollution in Rural China. Sci. Total Environ. 787, 147702. doi:10.1016/j.scitotenv.2021.147702
Zeweld, W., Van Huylenbroeck, G., Tesfay, G., and Speelman, S. (2017). Smallholder Farmers' Behavioural Intentions towards Sustainable Agricultural Practices. J. Environ. Manag. 187, 71–81. doi:10.1016/j.jenvman.2016.11.014
Keywords: Ecological agricultural technology (EAT)1, TAM-TPB framework2, fsQCA3, Intention to adopt4, China5
Citation: Dong H, Wang H and Han J (2022) Understanding Ecological Agricultural Technology Adoption in China Using an Integrated Technology Acceptance Model—Theory of Planned Behavior Model. Front. Environ. Sci. 10:927668. doi: 10.3389/fenvs.2022.927668
Received: 24 April 2022; Accepted: 23 May 2022;
Published: 30 June 2022.
Edited by:
Huaping Sun, Jiangsu University, ChinaReviewed by:
Wornchanok Chaiyasoonthorn, King Mongkut’s Institute of Technology Ladkrabang, ThailandCopyright © 2022 Dong, Wang and Han. This is an open-access article distributed under the terms of the Creative Commons Attribution License (CC BY). The use, distribution or reproduction in other forums is permitted, provided the original author(s) and the copyright owner(s) are credited and that the original publication in this journal is cited, in accordance with accepted academic practice. No use, distribution or reproduction is permitted which does not comply with these terms.
*Correspondence: Jichang Han, aGFuamNfc3hkakAxMjYuY29t
Disclaimer: All claims expressed in this article are solely those of the authors and do not necessarily represent those of their affiliated organizations, or those of the publisher, the editors and the reviewers. Any product that may be evaluated in this article or claim that may be made by its manufacturer is not guaranteed or endorsed by the publisher.
Research integrity at Frontiers
Learn more about the work of our research integrity team to safeguard the quality of each article we publish.