- 1Faculty of Geography, Babes-Bolyai University, Extension of Sighetu Marmatiei, Sighetu Marmației, Romania
- 2Faculty of Geography, Babes-Bolyai University, Cluj-Napoca, Romania
- 3Faculty of Geography, Tourism and Sport, University of Oradea, Oradea, Romania
- 4Department of Digital Economy, Samarkand State University, Samarkand, Uzbekistan
- 5Faculty of Geography, University of Belgrade, Belgrade, Serbia
- 6Faculty of Natural Sciences, L.N. Gumilyov Eurasian National University, Nur-Sultan, Kazakhstan
- 7Department of Geography, Tourism and Hotel Management, Faculty of Sciences, University of Novi Sad, Novi Sad, Serbia
- 8Faculty of Geology, Geography, Recreation and Tourism, V.N. Karazin Kharkiv National University, Kharkiv, Ukraine
- 9Faculty of Sciences and Mathematics, University of Priština in Kosovska Mitrovica, Mitrovica, Serbia
Downscaling and modeling natural disturbances such as the extratropical storms are important for understanding and predicting the behavior of the air flow over complex forested areas, especially in high wind instances that cause damages. Therefore, the rationale of the research was that the windscape of a storm could be reconstructed based on the features of consequent forest windthrows. Main input data for the model were derived from data on forest damages (extension, orientation, and depth of windthrows) retrieved from combined UAV imagery, high-resolution imagery, and field survey, integrated with terrain, wind data at stations within the study area, land cover, overturning and breaking point conditions for tree species, and human interventions. Using a GIS environment, a critical wind speed index was calculated, as well as the main wind direction on each damaged stand, considering the factors that lead to windthrows. This model was then compared to a computational fluid dynamics wind model built with WindNinja app, using the conservation of mass and momentum solver, at the moment of the storm, and adjusted. Ultimately, maps and 3D models were presented to the main stakeholders in the area, namely, forest management, protected area operatives, and road and tourist infrastructures’ management in order to enhance the resilience strategies and environmental protection.
1 Introduction
Wind gusts produced during high-intensity storms represent one of the most frequent hazards with destructive potential in the temperate zone, affecting both human infrastructure elements and forest lots. They are considered the number one hazard factor in Europe by the volume of timber affected during extratropical storms or convective storms, being responsible for more than half of the damage caused. The main risk factor during the manifestation of high-intensity storms is represented by trees that fail by breaking and uprooting, under the pressure of wind gusts (Schelhaas, 2008). The complexity of the relief configuration can significantly influence the parameters of a storm when it passes over topographical obstacles, by increasing the wind speed near the surface and implicitly the wind stress on forest and built environment. Unfortunately, the occurrence of such episodes causes significant material damage and loss of human life.
Regarding wind damage to forest surfaces, over the past 150 years, reported storm damage appears to have increased in both frequency and magnitude. For the period 1950–2000, the annual average of storm damage was estimated to be 18.7 million cubic meters of wood (Schelhaas, 2008), hence the importance socio-economic and environmental consequences.
Understanding the behavior of air flow in high wind situations over complex topographic surfaces, covered by forest vegetation, is essential in various practical domains, from forest and road infrastructure management to tourism (Gardiner et al., 2017). The evolution of storms and their impact on human society explain the great interest to study their frequency, intensity, trajectory, etc., objectifying the need to compute and display large datasets in a fast and accurate way (Gardiner et al., 2010, 2013; Schindler et al., 2012; Suvanto et al., 2016; Szwagrzyk et al., 2018; Lukić et al., 2021; Ponjiger et al., 2021). These efforts intensified especially after the storms Lothar and Martin (December 26 and 27, 1999 respectively), some of the most catastrophic events of the last century in Europe. Even though the study area is not susceptible to wild fire, the risk of human-generated forest fire is correlated with different types of high wind situations. Hence, the assessment and predictor tools were first developed in this area.
A relatively new approach involves the analysis of the evolution of the exposure to this type of risk of different humanized spaces. At the level of the European Union at least, attempts are being made to harmonize databases containing historical time series of damage caused by storms in the forest sector that would allow adequate risk analyses. For example, starting in 2018, within the framework of the Copernicus program, the WISC (Windstorm Information Service) project started, through which a new operational service will be implemented that will make new datasets available to decision-makers that will allow understanding the occurrence mode and the impact of wind gusts (https://wisc.climate.copernicus.eu/wisc/#/). Technological advances were connected to assessing forest damages either through remote sensing as an efficient tool involving high resolution optical EO data (Einzmann, 2017) or through a two-stage sampling plan using single date, post-event ALS data (airborne laser scanning) (Chirici et al., 2018). At the same time, integrating logistic regression into GIS to assess wind disturbance forest vulnerability (Lukas Krejci et al., 2018) was part of the process.
That is why, the first rationale of this research is linked to the idea of visualizing the features of a storms’ windscape, post-event, downscaled to the Gutai Mountains. We also acknowledged that it is complicated to develop such a model in mountain forested areas, knowing that general wind models did not perform well in some complex areas or at this scale (Lindsay and Rothwell, 2008; Achim and Nicoll, 2009; Brunet et al., 2009; Petersen, 2013; Wagenbrenner et al., 2016; Dupont et al., 2018). In addition, this paper does not tackle prediction of forest damage due to strong winds. It is mainly about the reconstruction of the windscape of 76 a storm that caused forest damage in terms of speed and wind direction.
Communicating to stakeholders using maps and simulations is important for a sustainable environmental management of the forested area, having in mind the features of national roads crossing the study area toward a renowned tourist destination such as Maramures and its protection status. This also linked to the resilience scenarios for the post-event reaction plans and to the publicly conveyed messages (Ilies et al., 2010; Ilies et al., 2017a; Ilies et al., 2017b; Bou-Belda et al., 2020; Ilies et al., 2021a; Ilies et al., 2021b; Caciora et al., 2021; Marcu et al., 2021). Gutai Mountains are involved in two different narratives regarding the forest: the eco-tourism destination status of the area is promoting sustainable tourism integrated with cultural and community resilience messages, on one hand, and it is one of the areas most affected by illegal wood harvesting in Maramures County, on the other (Cicort-Lucaciu et al., 2011; Ilies et al., 2012; Kelfaoui et al., 2021; Nigmatova et al., 2021; Durlević et al., 2022). The situation in the aftermath of the extratropical storm was poorly communicated to the public, leading to negative inferences about the degree of deforestation and devastation, also degrading the tourist destination image by the presence of uncleared windsnaps and windthrows 3–4 years after the event. Nevertheless, these remains from 2017 laid the ground for ulterior forest damages by the following wind gusts, although weaker in wind speed (in April 2019 and August 2021).
Therefore, the main research objective was to explore the prospects of reconstructing the windscape during the storms, based on the features of the forest damage that it produced, using GIS and modeling, by integrating different types of imagery with a consecrated computational fluid dynamic wind model and field data retrieval. The subsequent aim was to substantiate the visualization products to be conveyed to the main operators within the study area, namely, road management, park management, tourist accommodation, and ski-related cable transportation installations.
2 Study area and context
Extratropical storms are rare events in this part of Romania, occurring mostly in the June to September season. Between 1950 and 2020, the average occurrence of wind gusts over 25 m/s was 0–4 times per year, with the year 2017 being a reference within the study area (www.eswd.eu). The high wind situation is used as the baseline for the present study—a derecho storm touched the north-western part of Romania and adjacent areas in Hungary, Ukraine, and Slovakia on 17 September 2017; it was the strongest since 1950 in terms of wind speed and forest damages. It reached the Gutai Mountains area (Maramures, Romania) around 5.00 p.m. EET, with an average wind speed of 25 m/s and gusts of 28 m/s, over a 20-min window, has a general direction from west (Magnusson 2021, https://confluence.ecmwf.int/display/FCST/201709+-Windgusts+-+Serbia+Romania). The national forest management evaluated consistent damages to forests (270.634 m3 of windthrow and windsnaps on 36.921 ha), 1,200 dwellings, and 78 businesses on that date (Regia Nationala a Padurilor-ROMSILVA, 2017).
First-hand observations revealed that the storm impacted the forest unevenly, with devastation along several channels across the mountains. Detailed ground survey on the damaged stands correlated with available high-resolution imagery and our UAV imagery showed an overturning pattern of windthrows, which is partly consistent with the scientific research corpus in the field. Flows across the forest stands, at breaking levels of 8–10 m above the ground, could be observed. At that point, computational fluid dynamics modeling (CFD) was taken into consideration for a seemingly straight-forward windscape visualization project, due to the low computing requirements of the applications we intended to use and the interoperability of its outputs with the GIS environment.
Specific CFD modeling for wind flows use complex solvers, which require large computing capabilities. In order to overcome these shortcomings, we used an open-source solver (WindNinja 3.5.3) to generate a model for the storm windscape. Two-dimensional and 3D visualizations of the output model at the scale of the study area revealed some differences in speed and orientation of the flows, compared to the surveyed data. Albeit not new, these differences occurred, so many related factors were documented or resulted from the related research articles and measurements for other regions (Kamimura et al., 2019). That is why we adjusted the general model with data derived from GIS treatment of the field survey and high-resolution images in order to present/explain the features of the windscape to the stakeholders.
The study area, the Gutai Mountains, is located in the northern part of the Romanian Carpathian range, forming the limit of Maramures Lowland (Figure 1). Gutai Massif (1,400 m a.s.l.) has a semicone shape facing north, due to its volcanic origin. Southward, lower mountain ranges, around 1,000 m, are shaped by a rectangular hydrographic network. At the periphery of these mountains, the altitudes reach 400–500 m. The territory has over 80% forest cover, with deciduous (Fagus sylvatica) and coniferous (Picea abies) species. The forest areas are interspersed with meadows (12%) and juniper bushes (Pinus mugo and Juniperus communis) at altitudes above 1,200 m (Ilies et al., 2017a). From the environmental perspective, the entire area is protected by the means of a Natura 2000 (Ministerul Mediului and Apelor și Pădurilor, 2021) site—ROSPA0134 site with ROSCI0089 Gutai—Creasta Cocosului and ROSCI0092 Ignis, and several national- or regional-level reserves (https://natura2000.eea.europa.eu/Natura2000/SDF.aspx?site=ROSCI0089). The area is served by two main roads, DN 18 (along 13 km) and DJ 184 (19 km), connecting the county capital Baia Mare with the Maramures Lowland across the mountains. Nevertheless, the tourist infrastructure is represented by the ski resorts of Cavnic and Suior, with 360 bed places, a total of 12.3 km of ski slopes, and 3.6 km long cable transport systems, all prone to high wind impact.
The areas affected by the storm and included in the present study are located on two valleys related to the mountain passes of Gutâi P. (987 m) and Neteda P. (1,054 m), managed by the Baia Sprie forest district, comprising an area of approximately 3,000 ha. We selected the study area due to 1) the extent of forest damage due to the storm on 17.09.2017, 2) the general aspect of the terrain, and 3) the important communication infrastructures that cross the mountain affected by the windthrows (Figure 1; Table 1).
The high wind situation within the study area was captured accurately by the NDVI (normalized difference vegetation index), before and after the storm. Sentinel 2L 2A produced relevant images for the AOI on 16 September 2017 (1 day before the storm) and on 09 October 2017 (2 weeks after), available at Landviewer (2021), https://eos.com/landviewer (Figure 2).
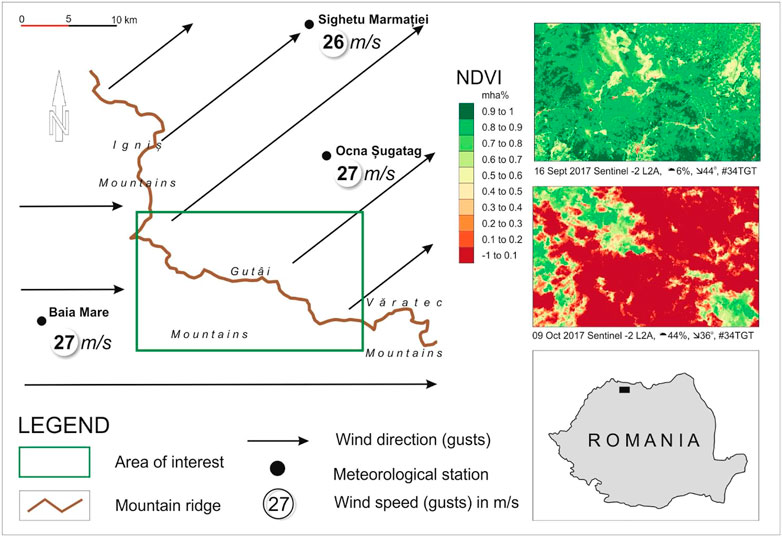
FIGURE 2. NDVI for the AOI of the study area, before (16.09.2017) and after the storm (09.10.2017), Sentinel 2L 2A at https://eos.com/landviewer.
3 Literature review
The varied environmental conditions and the structural and allometric diversifications of forest stands make it difficult to build highly accurate wind models at small scales. Therefore, researchers try to fully understand the behavior of air masses above complex topographic surfaces covered with forest vegetation using field or in situ research, simulations, and laboratory experiments through which territorial management practices in general and forestry in particular can be developed, increasing the degree of resilience and preventing material damage, injuries, or deaths (http://ec.europa.eu/agriculture/analysis/external/euroforests/fullreporten.pdf).
Therefore, windscape modeling has been a research subject for a number of studies in order to explain the impact, to substantiate ecological or green management practices (Morar et al., 2022), to consolidate resilience, etc. (Gardiner, et al., 2017). The complexity of the air flow over forested areas raised the interest of interdisciplinary research, mainly regarding those extreme events and the resulting wind damages or floods (Ilieș and Grama, 2010). Resulting models include predictive statistical models (Jackson and Hunt, 1975; Kim et al., 1997; Bitsuamlak et al., 2004; Balogh et al., 2012; Hannon Bradshaw, 2017), wind risk assessment models (Hale et al., 2015; Locatelli et al., 2017), machine learning wind calculators (Hart et al., 2019; Wagenbrenner et al., 2019), finite element modeling (FEM) (Yang et al., 2014), and computational fluid dynamics (CFD) wind models (Zhang et al., 2011; Kim and Kim, 2012).
Nevertheless, displaying information through high-quality visualization became available due to the increasing computing power, internet mobile connectivity, and overall technological advances (Valjarević et al., 2014; Niemets et al., 2018; Stankov et al., 2019; Valjarević et al., 2020; Morar et al., 2021; Valjarević et al., 2021).
Over the past 10 years, progress was made in the online application design for windscape modeling and a number of GIS plugins and algorithms for wind and forest including mixed method modeling (Grebhan et al., 2009; Kim and Kim, 2012; Laib and Kanevski, 2016; Wagenbrenner et al., 2016; Pintilii et al., 2017). Using open-source solutions to solve CFD data are relatively new, which were developed for simulating different essential conditions that impact the wind flow over a vegetated area, with a variety of terrains, soil, and vegetation features (Zhang et al., 2011; Gardiner et al., 2017).
Regarding the potential factors that influence the air flow in forested areas, more specific studies summarize research results as groups of predictor variables, offering detailed insights for the analysis of storm damage, including stand data, terrain data, wind data, and species data (Albrecht et al., 2009; Wellpott et al., 2009).
In this respect, several online and desktop applications offer tools which support decisions of forest managers by integrating the aforementioned data through machine learning and webGIS—for example, Forest GALES (Albrecht et al., 2019) or CFD wind modeling—for example, WindNinja (Wagenbrenner et al., 2019).
Forest GALES uses terrain and tree data correlated via algorithms to determine the critical wind speed at breaking point and overturning, which have been proved to be useful for modeling the air flow (Locatelli et al., 2019). It is essentially a computer-based decision support tool that enables forest managers to estimate the probability of wind damage to any conifer stand in Britain by calculating the wind speed that would be expected to damage a stand (https://www.forestresearch.gov.uk/tools-and-resources/fthr/forestgales/). The documentation indicates a set of variables and code that could be adapted for other regions (Locatelli et al., 2019).
WindNinja is an open-source application developed and maintained by the USFS Missoula Fire Sciences (Kim et al., 1997; Yang et al., 2014). It is essentially a CFD mass and momentum conserving diagnostic wind model, initially used for forest fire prediction, but ultimately ended up being used to downscale wind fields in areas where large weather models do not perform well. Current research on windscapes uses the outputs of ninjaFOAM solvers in order to confirm or to adjust wind data for hilly or low mountain areas (Forthofer et al., 2014; Quill et al., 2019; Wagenbrenner et al., 2019). The output can be visualized in QGIS, ArcGIS, and Google Earth and covers any given area of the world. The available algorithms for data analysis (wind speed and wind direction) for a mountain region such as our study area integrate data on forest/forest damage in order to adjust the windscape output of a CFD model, considering the strongest high wind situation.
4 Data and methods
In order to reconstruct the windscape downscaled to the Gutai Mountains, we used the storm on 17 September 2017 as the baseline reference. The logical data flow includes 1) terrain, 2) storm, 3) forest, and 4) forest damage (Table 2). Other important factors (soil, deforestation, forest management, and infrastructures) were considered at the relevant moment of analysis.
In the aftermath of the storm, the resultant forest damage suggested that the mechanical effects of the terrain on the flow are essential under high wind speed conditions, as also shown in studies by Wagenbrenner et al. (2019) and Forthofer et al. (2014). Therefore, a first windscape model could be derived from the forest damage assessment and terrain (Figure 3). Verification and validation processes directed the research toward the need to compare the model to a consecrated model; hence, the second model is a CFD wind model derived using WindNinja 3.5.3 application for the area of interest. This second model has, as input variables, wind data measured at the closest meteorological station during the storm, terrain, and land cover at an appropriate resolution (Table 2). The validation includes the assessment of the differences between the two models in terms of wind speed and direction within five observed stances (main channel, upward, leeward slopes, saddles, and ridges), and the correlation between the two types of channels (terrain and CFD derived) and the occurrence of the observed damaged stands. Ultimately, the outputs were treated for visualization used during the meetings with the main stakeholders like park management, road management, and tourist resort business representatives. These materials explain the features of the windscape and the behavior of the air flow during high wind situation and lay the foundation for further resilience plans and practices.
Figure 3 shows the workflow details and the input data from Table 2 as a three-step design: 1) modeling the critical wind speed; 2) modeling the air flow using a CFD wind model; and 3) downscaling, validation, and visualization of the windscape for the exact moment of the storm.
• Step 1—first wind model: processing field survey, integrating imagery data, and determining the critical wind speeds for each damaged stand.
In the aftermath of the storm, from October to November 2017, our research team carried out the field research using specific mapping procedures with GPS, observations, and measurements on the damaged forest stands. These were correlated with UAV imagery retrieved in November 2017 and September 2019 and high-resolution imagery on the forest: NDVI and deforestation index, before and after the storm. Consequently, we built the spatial database for the field survey data and derived the CWS index for the study area, using built-in algorithms available with QGIS 3.16.
In addition to the data on trees, considered essential, we also input data related to tree cover density (TCD), mean canopy values, soil type, rooting depth, and the nature of stand edges in order to calculate a more accurate output for the critical wind speeds. Thus, we calculated a CWS index at a breaking point and overturning, from surveyed stands’ features using the formulas described by Locatelli et al. (2019). CWS data were validated against the Forest GALES 2.5. application and also acknowledged the limitations of the output related to the data for trees that are available for Norway spruce (Fonweban et al., 2012).
• Step 2—second wind model: generating the CFD model for the area at the moment of the storm
We calculated the wind directions and speed for the CFD model with WindNinja 3.5.3, using the conservation of mass and momentum solver, with initial input data from the Baia Mare meteorological station and from the Baia Mare Airport automated meteorological station. Available data were filtered for 30-min intervals, from 3.00 to 6.00 p.m. (Weather underground, 2021, https://www.wunderground.com/history/daily/ro/t%C4%83u%C8%9Bii-m%C4%83gher%C4%83u%C8%99/LRBM/date/2017-9-17).
We ran the solver for all six stances, focusing on the data at 5.00 p.m., having the highest hourly mean wind speed and the lowest hourly air temperature. The solver outputs were then loaded in QGIS 3.16, according to the guidelines provided by the tool developers. Furthermore, the wind speed data were downscaled for the AOIs, and the air flow channels were derived (CFD channels).
• Step 3—validation: model adjustment and visualization.
Third, the validation processes used for the present study can be categorized into several types: comparison to other similar models, parameter variability, and predictive validation, only to some extent. We compared the two models (CFD and CWS) and correlated the differences with a set of factors, highlighting the areas requesting adjustments, determined mainly by the details of the terrain, the correlation with air flow channels, and junction points. High-resolution imagery on NDVI and TCD was treated in order to determine the damaged stand features outside the two field study areas (Gutai West and Gutai East) in terms of wind speed and direction. Also, graphic visualization of the windthrow direction on the main CFD channels was tested for dominant angles: 45, 90, 135, and 315 degrees derived after the storm TCD layer, in order to identify the locations with two or more overlapping factors. Finally, the data were prepared for visualization in the 2D and 3D environments (GIS, ParaView, and Google Earth).
Field survey of the damaged stands covered 41.5 ha, comprising 28.4% of the total study area of 146 ha. We selected the position of the two sampling areas with a variety of stances: windthrows along the narrow channels, within a vortex structure, uphill on a slope, and windsnaps at 10 m above the soil within the basinets and along the large valleys (Figures 4, 5).
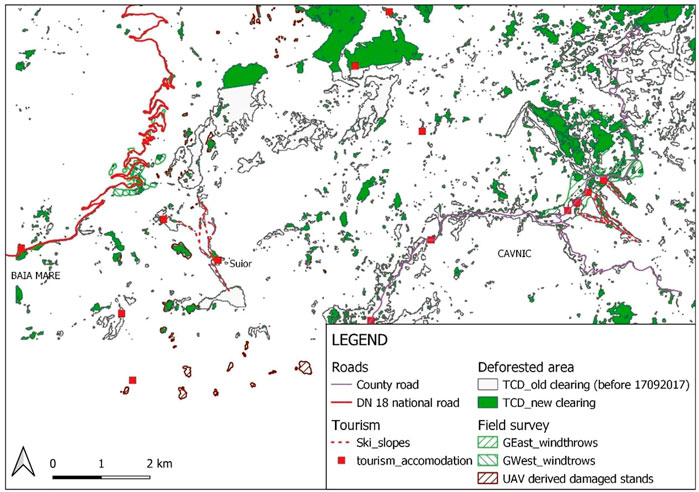
FIGURE 5. Tree cover density (TCD 2015 and 2018 under 25), field survey samples for the windthrows, and human infrastructure.
Regarding the specifics of the damages, the Norway spruce stands were affected mostly by windsnaps, and beech stands were mostly overturned (Table 3). The stands included in the field survey suffered massive damages, counting 4,000 trees, with differences within the two studied sectors: Gutai East 91.7% Norway spruce, and Gutai West 64% spruce/36% beech. From the morphological perspective, significant variables needed to be input in the model are shown in Table 3, correlated with the age of the stands and altitudinal disposition originating from reforestation projects during the 50s and 70s of the last century, lower locations for Norway spruce, geometric patterns and fixed distances between the trees, etc. Recent deforestation activity was also present before the storm, and that is why determining the area of the damage caused by the extratropical storm was confirmed with the UAV imagery and the field survey within the two sampling areas: Gutai West (further identified as GW) and Gutai East (GE).
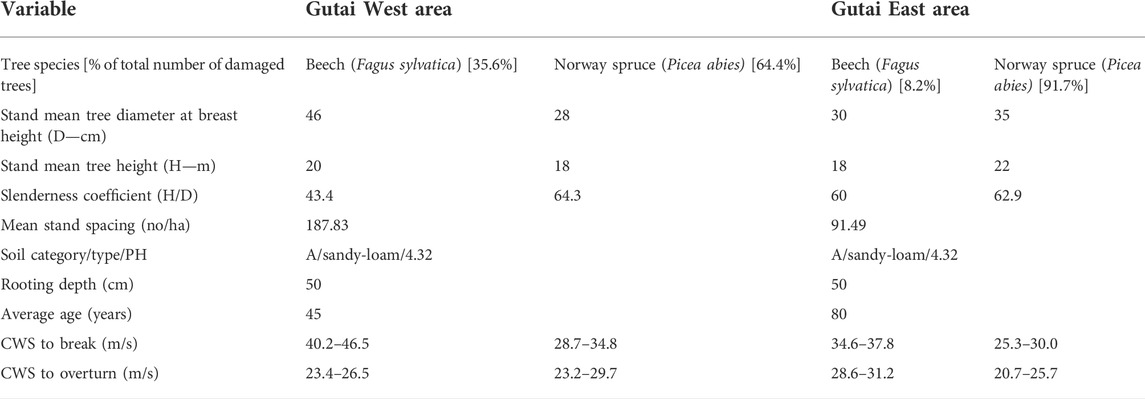
TABLE 3. Variables to calculate the critical wind speed (CWS) for windthrow and windsnap at 10 m (data source: NFM 2017 Report, OSPA Cluj, Forest GALES for conifers).
5 Results
5.1 Windscape reconstructed from the forest damage assessment
The general windscape of an area of interest is described mainly by the wind speed and the air flow direction in specific situations.
First model rationale: since windthrows have been produced, an index of critical wind speed could be calculated for the storm and integrated within a model. A number of studies assert that damage probability depended on forest stand characteristics, cuttings, soil, and topography, regardless of storm type, presenting a certain critical wind speed needed to produce breaking or overturning of the trees (Suvanto et al., 2016). Therefore, for each damaged stand, a critical wind speed was calculated and interpolated using QGIS 3.16, integrating the variables shown in Table 3, with spatial data derived from field survey and our own UAV imagery (Step 1 in Figure 3). Wind directions were estimated according to the mean direction of the windthrow. Critical wind speed (CWS) for the present study is considered as the minimum wind speed that could determine windthrows and windsnaps within the study area based on the model provided by Forest GALES for conifers, which is “a hybrid mechanistic model” used where climate data are available (Albrecht et al., 2019; Hart et al., 2019, p. 20). Therefore, Figure 6 shows the estimated CWSs for each surveyed stand and a general value consistent with data provided by meteorological stations at the moment of the storm, 26–28 m/s modulated by the terrain and air flow channeling (https://confluence.ecmwf.int/display/FCST/201709+-Windgusts+-+Serbia+Romania).
Looking at the details regarding the wind speed calculation method for the two surveyed areas, (Figure 6) the factors regarding species, height, diameter, and age were determined from field survey, specific data on the forest, and every damaged stand in particular. The next stage was to determine the soil type, rooting depths, spacing, and thinning procedures in order to input the data for the first part of the Forest GALES tool, also at a resolution of damaged forest lot. For the present study, we did not run the algorithms for predictions and occurrence probability, since the data are calibrated for Britain. Another limitation is that there is a small range of tree species available for the research mode of the tool. The specifics for the Norway spruce were considered of main importance due to the type and extent of the damages within the two surveyed area (Table 3). Data for beech were estimated according to a 2017 Report of the National Forest Management—NFM (Regia Nationala a Padurilor-ROMSILVA, 2017) and discussions with specialists from the Baia Sprie forest district. The result is a database with CWS for every damaged forest stand, and the attributes are mentioned in Table 3. Using GIS treatment (point generation and interpolation), the calculated wind speed depicts different types of correlations with the main findings in the field, further discussed within Section 5.3 used for identifying other damaged stands and their attributes which could contribute to the windscape reconstruction for the larger area.
5.2 Computational fluid dynamics model
The second windscape model is a consecrated CFD model based on the dataset containing the output of the WindNinja 3.5.3 application (Windninja firelab, 2021, https://weather.firelab.org/windninja/) for the speed and wind directions occurred during the storm on 17 September 2017 at the level of the study area. The modeling process is described by the step 2 of the Figure 3. It consists mainly in:
- selecting the area of interest with data on terrain with a variety of accepted file types: ASCII raster (*.asc), FARSITE landscape file (*.lcp), GeoTiff (*.tif), and ERDAS IMAGINE (*.img);
- setting the dominant vegetation type allowing to approximate the drag effect;
- mesh resolution selection directing the details of the output files and also the time needed to solve the model. For the present study, we selected the medium resolution due to the extension of the study area;
- input wind speed and direction, an average value for the wind; for this stance, the data measured at Baia Mare station at the time of the storm were used, although the application allows multiple values.
The model outputs are very versatile, optimized for several types of visualizations: Google Earth (*kmz files), shape files (at the same projection as the initial mesh), geospatial PDF files, and VTK files. This widens the presentation possibilities to the stakeholders, especially in GIS environments, Google Earth, or 3D environments as ParaView.
5.2.1 Computational fluid dynamics windscape visualized in QGIS 3.16
The output data for the model used here were the shapefile package (*.shp, *.shx, *.dbf, and *.prj) visualized in GIS. Conditional and unconditional wind directions were derived from the diagnostic model offered by WindNinja 3.5.3 application, after running the conservation of mass and momentum (CMM) solvers for the data provided by meteorological stations, within the “domain average wind” section. We input six series of 30-min wind data (speed, direction, time, date, cloud cover, and air temperature), around the main wind gust occurrence (3–6 p.m.), on the date of the storm. Also, standard 10 m height and tree settings were used. This generated a wind field at a resolution of 50 m for each run, analyzed in QGIS and transformed into a general windscape of the storm. Another output is a ninjaFOAM file, which was assimilated with an elevation raster. This output raster was treated with channel and drainage tools from SAGA: fill sinks with the Wang &Liu algorithm in order to derive channels with the Channel and network drainage basin. The main result of this stage is a channel network, further used to visualize the windscape for the larger study area.
Figure 7 shows the features of the wind (speed and direction) at 5.00 p.m. combined with the air flow channel data derived in QGIS 3.16 based on the ninjaFOAM outputs. The output files are originally formed by point data with two main attributes: wind speed (mph) and direction (degrees, with default 270o representing wind from west), and in order to facilitate comparison with the first model (the CWS windscape), data were interpolated.
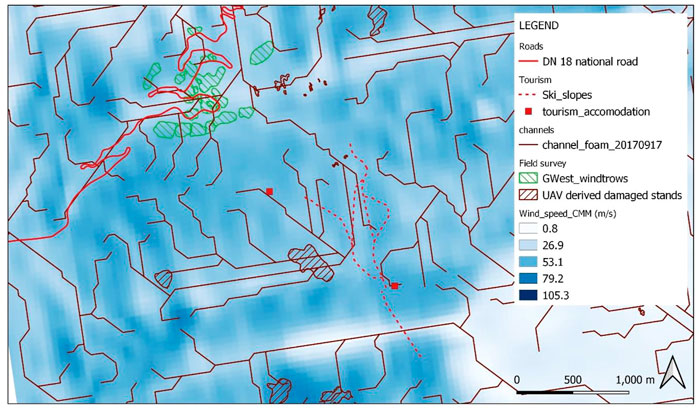
FIGURE 7. Initial CFD model of the storm on 17.09.2017, with channels derived from the WindNinja dataset, treated with tools from SAGA in QGIS 3.16.
5.2.2 Computational fluid dynamics model visualized using Google Earth
A general image of the CFD model is formed by representing the data through a *.kmz file using Google Earth (from http://earth.google.com/). The vectors’ appearance, the classification method, and the resolution can be adjusted in order to explain or to demonstrate the features of the wind within the study area. In addition, it is easy to change the scale or the perspective when analyzing the main stances, where the two models do not correlate well (see Section 5.3).
Figure 8 is a raw representation of the windscape based on the output of the WindNinja application, with built-in data optimized for Google Earth. Although the measured general wind speed was 25–28 m/s and the direction was west, terrain and air flow channels were formed speeding up or slowing down the gusts. First, in the western part of the mountain, the storm entered along the valley, confining the forest stands between two channels, causing damages. Nevertheless, the confluence created the premises for recirculation, confirmed by the observations on the windthrows’ orientation. Second, in the eastern part, most of the forest damages were along the channels with lower wind speed and forced direction changes. Using this pattern, we tried to find if there are similar correlations within the larger study area. From the UAV images, we derived the location of other damaged forest stands and overlapped the data with our CDF model, confirming that the nodes of the channel networks are more prone to producing forest windthrows and windsnaps.
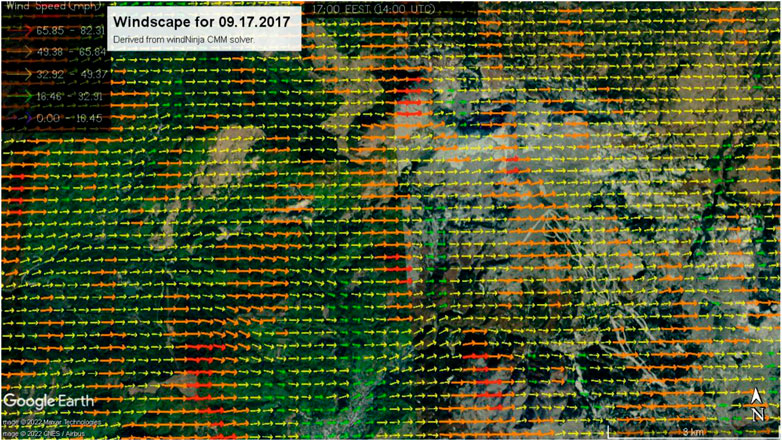
FIGURE 8. Initial CFD model of the storm on 17.09.2017, derived from the WindNinja dataset for Google Earth.
5.3 Validation, downscaling, and visualization
5.3.1 Validation and downscaling
Verification and validation are of crucial importance when building a model. The verification processes used for the present study are implemented in order to see if each step is complete, consistent, and correct (Law, 2014). First, we needed to answer several questions about the models:
- Do we know what is our model doing? Are there any hidden assumptions?
- Do we overcome the math issues? What data could be causing strange behaviors?
- Does verification and validation of each model guarantee credibility? What if each model produces valid results and they integrate well but the simulation produces invalid results?
The answers to these questions were tackled along the development of each model (Step 1 and 2 in Figure 3), by stating the rationale, using the data and the IT resources correctly, and by using multiple validation methods.
Second, the validation stage tries to answer the question about the relevance of the model we built. The validation strategy is based on the comparison to other similar models, parameter variability, and predictive validation, only to some extent.
Integrating the models resulting from CFD, the windthrow critical wind speed model and remote sensing data in GIS environment allowed us to reconstruct the windscape using a finer tuned approach. The general wind direction model output from WindNinja application is considered a consecrated wind model, even though it is mainly used in forest fire prevention. The research corpus using the output of this application stresses on a series of limitations regarding the leeward slope, working well on the windward side and ridgetops. Also, there are limited datasets available for evaluating high-resolution wind models in correlation with terrain complexity, spatial density, and meteorological conditions, hence the need to downscale the large-scale model is needed (Wagenbrenner et al., 2016). Although the location and magnitude of forest damages mostly confirm the existing theory in the field, the derived data offer a much higher correspondence with the observed features of the windthrow. Therefore, the direction output was corrected by the angle of the channels with simple empirical measurements. Channeling around the edges of damaged forest stands was observed, with two exemptions, located at the higher end of the channels, within the saddles. Wind speed is rather accurate, but wind directions are to be corrected according to the channel flow layer.
Post-event surveys and measurements confirm that the values of the critical wind speed calculated and represented using complex criteria described earlier are correlated with the channeling effects, the recirculation processes, and outlined the leeward effects, missed by the CFD model. Regarding the wind speed values, observed differences were between 0.9 and 14 m/s, lower values corresponding to the main channels and the higher ones along the ridges (Table 4). Moreover, the wind direction was evaluated with higher differences within the vortex areas and on upward slopes. This may be due to the features of the overthrown trees in terms of volume, height, and root system.
Regarding the validation of channeling effects used to re-construct the windscape, three hypotheses were formulated concordant with methods described as parameter variability and predictive validation, only to some extent:
• H1: damages occurred at the intersections of two types of channels: terrain and CFD generated (case A)
• H2: damages occurred at the confluences of CFD channel network segments (case B)
• H3: damages occurred where the direction changes of the air flow are higher than 45o
GIS analysis on windthrows and air flow showed that even though it is observable, it does not confirm the first hypothesis (Figure 9). At least three types of situations can be derived from the analysis of case A: (1) damaged stands are located exactly at the intersection of the channels derived from terrain and from air flow; (2) damaged stands can be found within a short distance, after the intersection; and (3) intersections without surveyed or derived damaged stands. First and second stances represent only 23.4% of the total number of channel intersections within the area Gutai West (Figure 9).
Moreover, topography aspect influences the magnitude, profile, and speed of the wind in the atmospheric layer in the immediate vicinity of the surface; the effects are more obvious in the area of mountain passes, on slopes directly exposed to the wind and on the saddles.
Stronger differences between the model and the observations occurred on SW–NE oriented valleys, compared to the valleys arranged on N–S or E–W. Along the main mountain ridge, around the altitude of 1,000 m, the wind was channeled and caused damage through saddles, without affecting the mounts between them. When switching from a flat or slightly sloping, deforested or a very young tree growing, to a steep (60°) leeward slope, the wind has strong effects on the inclined surface, particularly in the first 20–30 m. This situation is confirmed by the intersections of potential damaged stands with the CFD-generated channels (noted here as Case B), by wind directions (45, 90, 135, and 315°). Figure 10 shows that direction changes, and CFD channel confluences are good predictors for damages occurrence (H2 and H3); hence, they could be considered in the windscape modeling process.
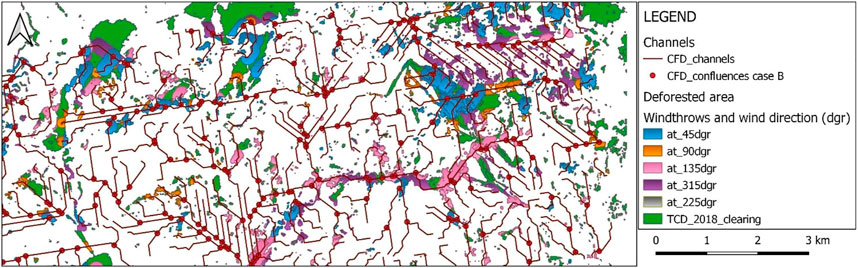
FIGURE 10. Case B—wind direction changes and CFD channel confluences and windthrows at different wind directions.
On the exposed slopes, with a reduced angle and on plateau-type surfaces, a higher incidence of damage was found, thus higher wind speeds and vortex movements. This accounts for the damages within the basinets and along the large valleys.
Supplementary predictive validation is based on the stand and species characteristics against data on other windthrow situations in Romanian mountains. Critical wind speed modeling asserts that stands of Norway spruce (Picea abies) are up to three times more prone to storm damage than beech (Fagus sylvatica). Nevertheless, the spruce forests in the area are the result of reforestation in the last 50 years, and are arranged at lower than natural altitude also showing a certain alignment of the trees within the stands.
The spruce trees were broken from the stem, about 10 m from the ground, while the majority of beech was overthrown. The integrity of a forest plot in the area can be affected at wind speeds of over 25 m/s (Săvulescu and Bogdan, 2012) for felling/breaking trees, over 45 m/s for penetration of a compact forest structure by a descendant vortex-type movement. The wind field derived from these data was consistent with the CFD model as general aspect, but displayed higher values for the actual wind speed. From this perspective, several typical situations are observable. Further modeling of the channels and flows in a mountain could also be developed to determine the areas prone to forest damage.
5.3.2 Visualization and presentation of the results to the stakeholders
Finally, in order to present the windscape of the storm to the stakeholders in the affected area, the resultant data were organized in a spatial database used to design the maps and the simulations (Figures 11). The goals of the visualization were linked to the following assumptions:
- The main stakeholders in the area need to fully understand what happened on the day of the storm, beyond the simplified explanations developed by media;
- The actors of resilience issues need the simulation models in order to scientifically ground their intervention plans and strategies;
- Research and development projects related to windscapes or to windthrows/windsnaps could consider the results as study case.
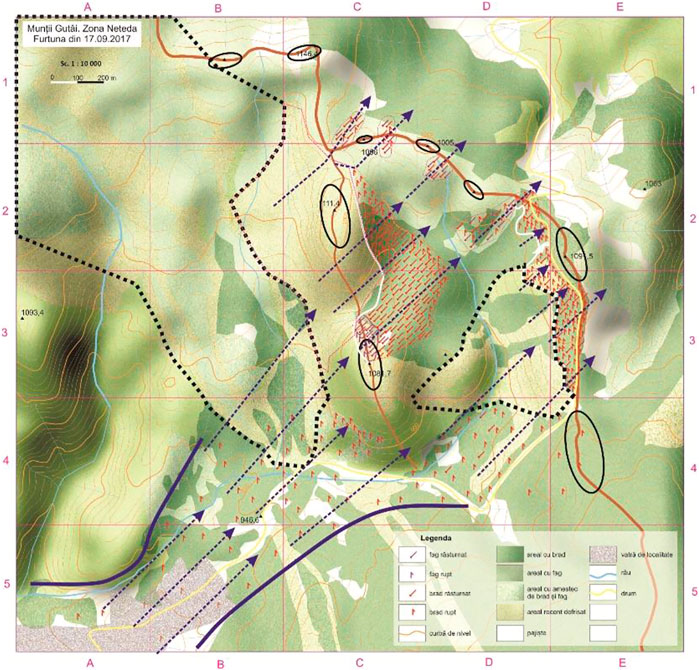
FIGURE 11. Gutai East area. Reconstructed windscape in QGIS treated with graphic design tools for the main stakeholders.
For the use of the main stakeholders, the map of the storm-related damages with the general wind directions was optimized in GDS in order to offer a better understanding of the main channels and flows, considering the impact on the road and tourist infrastructures (Figures 11, 12). For the more specialized stakeholders (forest and protected site management authorities), we reconstructed the windscape of the storm in a 3D environment.
6 Discussions and conclusion
Integrative reconstruction and visualization of rare event windscapes is crucial for an accurate understanding of the effects of damaging occurrences within a protected area, also involving road and tourism infrastructure.
The theoretical literature corpus on forest windthrows and windsnaps approaches the windscape concept in relation with a complex set of factors. Therefore, for the stakeholders within the study area, it is hard to grasp the specific context. Using as pretext a rare event such as the extratropical storm on 17.09.2017, the study highlights the main tools that could be used for detailed modeling and to display the potential risk areas. Model evaluation for the scope of the study is performed considering both mathematical and domain-specific criteria: the accuracy of the solver against the declared limitations of the WindNinja application, the stances observed in the literature regarding high wind situation in mountain forested environments, and several case studies for storms and mountain areas in Romania and abroad.
Using the windthrow—the terrain model—against a simple CFD model has been proved effective from the vantage point of computing capacity and visualization options. First, the model highlights the channeling effects and the trajectories of the channels. The GIS treatment of the datasets showed that the forest damages mostly occurred at the junctions of the CFD generated channels and in the area confined between the two types of channels doubled by changes in wind direction.
Second, downscaling the windscape allows us to analyze the finer features of the factors that contributed to the windthrows and windsnaps at different scales. The CFD model generally uses a 100-m resolution for the solver. This is considered finer than the general resolution of a weather model, which is usually 3 km (https://www.firelab.org/project/windninja). Moreover, combined with a standard type of forest and a 25-m DEM, it produces a detailed image on the windscape, for both speed and directions. Also, high-resolution imagery and derived indexes as NDVI and deforestation index time series available for −1 day and +22 days (16.09 and 09.10.2017) easily rule out other types of deforestation processes, considering the resilience matters (affected primary roads and infrastructure). Nevertheless, the tree cover density index available for 2018 was considered accurate for modeling with a 10 m resolution.
Third, the entire dataset used for modeling is publicly available, and the applications are open source, which opens a range of further developments in the field of windscape visualization for rare events. Exact measurements during the high wind situations are difficult to achieve when this type of event rarely occurs. Therefore, a post-factum analysis could be considered, capitalizing on an inverse rationale: to reconstruct the windscape based on the damages on the forest, knowing that there is a correlation between complex set of features and the windthrows/windsnaps, including the critical wind speed (CWS).
Ultimately, the reconstruction can be visualized in professional environments, such as GIS or ParaView, and more accessible platforms such as Google Earth. This model confirms most of the research conclusions about the prerequisite conditions for windthrows/windsnaps in mountain areas and can be useful in forest management, protected area operations, road, and tourist infrastructure management in order to enhance the resilience strategies and environmental protection.
In conclusion, the article is focused on a case study with an inverse rationale—the reconstruction of a windscape after a storm that caused forest damage. It was conceived based on forest and forest damage data retrieved from field research, high-resolution imagery, and UAV imagery. It does not tackle prediction of forest damage due to strong winds, even if, during the process of modeling, predictor factors were considered. At a wider scale, this study tried to demonstrate that forest damage assessment can be an important aspect to integrate in the windscape reconstruction models. For a finer approach, the study has several limitations in terms of data retrieval and validation. Data retrieval is not completely automated; it has to be confirmed with field survey and UAV for those areas with unavailable imagery or with higher deforestation rates. The validation process of the model for the leeward slopes and channels could be improved by a more accurate CFD model, designed for a larger variety of stances and not only for the extremes. This requires larger research capabilities in order to organize the in-field measurements during a high wind situation, starting from the moment the warning was issued, so post-event evaluation is an option to be developed.
Data availability statement
The original contributions presented in the study are included in the article/Supplementary Material; further inquiries can be directed to the corresponding author.
Author contributions
Conceptualization, GI, MI, and MH; data curation, GI, MI, and MH; formal analysis, GI, MI, MH, S-VB, D-CI, TC, BS, and CM; methodology, GI and MI; project administration, GI and MI; visualization, GI, MI, and MH; writing—original draft, GI, MI, MH, S-VB, D-CI, TC, BS, CM, AV, TL, MM, PV, NH, ZB, and NL; writing—review and editing, GI, MI, MH, S-VB, D-CI, TC, BS, CM, AV, TL, MM, PV, NH, ZB, and NL. All authors have read and agreed to the published version of the manuscript. All authors have contributed equally.
Acknowledgments
We acknowledge the partial contribution of University of Oradea Grants, Competition “Scientific Research of Excellence Related to Priority Areas with Capitalization through Technology Transfer: INO–TRANSFER–UO”, Project Nos. 329/2021 and 317/2021. The research undertaken was made possible by the equal scientific involvement of all the authors concerned.
Conflict of interest
The authors declare that the research was conducted in the absence of any commercial or financial relationships that could be construed as a potential conflict of interest.
Publisher’s note
All claims expressed in this article are solely those of the authors and do not necessarily represent those of their affiliated organizations, or those of the publisher, the editors, and the reviewers. Any product that may be evaluated in this article, or claim that may be made by its manufacturer, is not guaranteed or endorsed by the publisher.
References
Achim, A., and Nicoll, B. (2009). Modelling the anchorage of shallow-rooted trees. Forestry 82 (1), 273–284. doi:10.1093/forestry/cpp004
Albrecht, A., Jung, C., and Schindler, D. (2019). Improving empirical storm damage models by coupling with high-resolution gust speed data. Agric. For. Meteorology 268 (1), 23–31. doi:10.1016/j.agrformet.2018.12.017
Albrecht, A., Kohnle, U., Hanewinkel, M., and Bauhus, J. “Empirical modeling of long-term storm damage data in forests of Southwestern Germany: Judging the impact of silviculture,” in Proceedings of the 2nd International Conference Wind Effects on Trees Albert-Ludwigs-University of Freiburg, Germany, October 2009. Editors H. Mayer, and D. Schindler (Freiburg: Albert-Ludwigs-University), 103–104.
Balogh, M., Parente, A., and Benocci, C. (2012). RANS simulation of ABL flow over complex terrains applying an enhanced k–ε model and wall function formulation: Implementation and comparison for fluent and OpenFOAM. J. Wind Eng. Industrial Aerodynamics 104-106, 360–368. doi:10.1016/j.jweia.2012.02.023
Bitsuamlak, G., Stathopoulos, T., and Bedard, C. “Numerical modelling of wind flow over different types of topography,” in Structures Congress 2004, Nashville, Tennessee, United States, May 2004 (American Society of Civil Engineers).
Bou-Belda, Eva, Indrie, Liliana, Camelia Ilies, Dorina, Hodor, Nicolaie, Berdenov, Zharas, Herman, Grigore, et al. (2020). Chitosan – a non-invasive approach for the preservation of historical textiles. Ind. Textila 71 (6), 576–579. doi:10.35530/it.071.06.1756
Brunet, Y., Dupont, S., Roux, P., and Sellier, D. (2009). “Tree motion in heterogeneous forests: A coupled flow-tree simulation study,” in Proceedings of the 2nd International Conference Wind Effects on Trees Albert-Ludwigs-University of Freiburg, Germany, October 2009. Editors H. Mayer, and D. Schindler (Freiburg: Albert-Ludwigs-University), 75–80.
Caciora, T., Herman, G. V., Ilieș, A., Baias, Ș., Ilieș, D. C., Josan, I., et al. (2021). The use of Virtual Reality to Promote sustainable tourism: A case study of wooden Churches historical Monuments from Romania. Remote Sens. (Basel). 13 (9), 1758. doi:10.3390/rs13091758
Chirici, G., Bottalico, F., Giannetti, F., Del Perugia, B., Travaglini, D., Nocentini, S., et al. (2018). Assessing forest windthrow damage using single-date, post-event airborne laser scanning data. For. An Int. J. For. Res. 91 (1), 27–37. doi:10.1093/forestry/cpx029
Cicort-Lucaciu, A. Ş., Cupşa, D., Ilieş, D., Ilieş, A., Baiaş, Ş., and Sas, I. (2011). Feeding of two amphibian species (Bombina variegata and Pelophylax ridibundus) from artificial habitats from Pădurea Craiului Mountains (Romania). North-Western J. Zoology 7 (2), 297–303.
Copernicus, (2022). Climate. Available at: https://wisc.climate.copernicus.eu/wisc/#/.
Copernicus Programme, (2021). Copernicus land Monitoring service at a glance. Available at: https://land.copernicus.eu.
Dupont, S., Defossez, P., Bonnefond, J. M., Irvine, M. R., and Garrigou, D. (2018). How stand tree motion impacts wind dynamics during windstorms. Agric. For. Meteorology 262 (1), 42–58. doi:10.1016/j.agrformet.2018.06.022
Durlević, U., Valjarević, A., Novković, I., Ćurčić, N. B., Smiljić, M., Morar, C., et al. (2022). GIS-based spatial modeling of snow avalanches using analytic hierarchy process. A case study of the Šar mountains, Serbia. Atmosphere. 13 (8), 1229. doi:10.3390/atmos13081229
Einzmann, K., Immitzer, M., Bock, S., Bauer, O., Schmitt, A., and Atzberger, C. (2017). Windthrow Detection in European forests with very high-resolution optical data. Forests 8, 21. doi:10.3390/f8010021
European Commission, (2022). Agriculture and rural development Supporting EU farmers, food security, the environment and rural areas. Available at: http://ec.europa.eu/agriculture/analysis/external/euroforests/fullreporten.pdf.
European Severe Weather Database, (2021). European Severe weather database. Available at: www.eswd.eu.
Fonweban, J., Gardiner, B., and Auty, D. (2012). Variable-top merchantable volume equations for Scots pine (Pinus sylvestris) and Sitka spruce (Picea sitchensis (Bong.) Carr.) in northern Britain. Forestry 85 (2), 237–253. doi:10.1093/forestry/cpr069
Forest Research, (2022). Forestry and tree Health resources. Available at: https://www.forestresearch.gov.uk/tools-and-resources/fthr/forestgales/.
Forthofer, J., Butler, B., and Wagenbrenner, N. (2014). A comparison of three approaches for simulating fine-scale surface winds in support of wildland fire management: Part I. Model formulation and comparison against measurements. Int. J. Wildland Fire 23 (7), 969–981. doi:10.1071/wf12089
Gardiner, B., Alexis, A., Bruce, N., and Ruel, J.-C. (2017). Understanding the interactions between wind and trees: An introduction to the IUFRO 8th wind and trees Conference (2017). For. An Int. J. For. Res. 92 (4), 375–380. doi:10.1093/forestry/cpz044
Gardiner, B., Blennow, K., Carnus, J. M., Fleischer, P., Ingemarson, F., Landmann, G., et al. (2010). Destructive storms in European forests: Past and forthcoming impacts. Joensuu: European Forest Institute. Final report to DG Environment (07.0307/2009/SI2.540092/ETU/B.1).
B. Gardiner, A. Schuck, M. J. Schelhaas, Ch. Orazio, Blennow Kristina, and B. Nicoll (Editors) (2013). Living with damage to forests (European Forest Institute).
Grebhan, K., Schindler, D., and Mayer, H. “GIS-based modeling for evaluation of wind damage probability in forests in Southwest Germany,” in Proceedings of the 2nd International Conference Wind Effects on Trees Albert-Ludwigs-University of Freiburg, Germany, October 2009. Editors H. Mayer, and D. Schindler, 264.
Hale, S., Gardiner, B., Peace, A., Nicoll, B., Taylor, P., and Pizzirani, S. (2015). Comparison and validation of three versions of a forest wind risk model. Environ. Model. Softw. 68 (1), 27–41. doi:10.1016/j.envsoft.2015.01.016
Hannon Bradshaw, L. (2017). Developing a model to predict storm damage to forests in Kronoberg county, Sweden, Masters diss. Lund: Department of Physical Geography and Ecosystem Science, Lund University.
Hart, E., Sim, K., Kamimura, K., Meredieu, C., Guyon, D., and Gardiner, B. (2019). Use of machine learning techniques to model wind damage to forests. Agric. For. Meteorology 265 (1), 16–29. doi:10.1016/j.agrformet.2018.10.022
Ilieş, A., Dehoorne, O., and Ilieş, D. C. (2012). The cross-border territorial system in Romanian-Ukrainian Carpathian Area. Elements, mechanisms and structures generating premises for an integrated cross-border territorial system with tourist function. Carpathian J. Environ. Sci. 7 (1), 27–38.
Ilieş, A., and Grama, V. (2010). The external Western Balkan border of the European Union and its borderland: Premises for building functional transborder territorial systems. Ann. Ann. Istrian Mediterr. Stud. Ser. Hist. Sociol. 20 (2).
Ilies, A., Hurley, P., Ilies, D., and Baias, S. (2017a). Tourist animation – a chance adding value to traditional heritage: Case studys in the land of Maramures (Romania). Rev. Etnogr. și Folc. (1–2), 131–151.
Ilies, D., Hodor, N., Indrie, L., Dejeu, P., Ilieș, A., Albu, A., et al. (2021b). Investigations of the surface of heritage Objects and green Bioremediation: Case study of Artefacts from Maramureş, Romania. Appl. Sci. 11 (14), 6643. doi:10.3390/app11146643
Ilies, D., Marcu, F., Caciora, T., Indrie, L., Ilieș, A., Albu, A., et al. (2021a). Investigations of Museum Indoor Microclimate and air quality. Case study from Romania. Atmosphere 12 (2), 286. doi:10.3390/atmos12020286
Ilies, M., Ilies, D. C., Ilies, A., Josan, I., and Ilies, G. (2010). The Gateway of Maramureş land. Geostrategical Implications in Space and time. Ann. Istrian Mediteranian Stud. Ser. Hist. Sociol. 20 (2), 469–480.
Ilies, M., Ilies, G., Hotea, M., and Wendt, J. (2017b). Geomorphic attributes involved in sustainable ecosystem management scenarios for the Ignis-Gutai Mountains Romania. J. Environ. Biol. 38 (5), 1121–1127. doi:10.22438/jeb/38/5(si)/gm-32
Jackson, P., and Hunt, J. (1975). Turbulent wind flow over a low hill. Q. J. R. Meteorol. Soc. 101 (1), 929–955. doi:10.1002/qj.49710143015
Kamimura, K., Gardiner, B., Dupont, S., and Finnigan, J. (2019). Agent-based modelling of wind damage processes and patterns in forests. Agric. For. Meteorology 268 (1), 279–288. doi:10.1016/j.agrformet.2019.01.020
Kelfaoui, A., Rezzaz, A. M., and Kherrour, L. (2021). Revitalization of mountain rural tourism as a tool for sustainable local development in Kabylie (Algeria). The case of Yakouren municipality. Geoj. Tour. Geosites 34 (1), 112–125. doi:10.30892/gtg.34115-626
Kim, D., and Kim, B.-Y. “Development and validation of computational wind field model (wind scape),” in Proceedings of 4th Asian Joint Workshop on Thermophysics and Fluid Science, Busan, October 2012.
Kim, H., Lee, C., Lim, H., and Kyong, N. (1997). An experimental and numerical study on the flow over twodimensional hills. J. Wind Eng. Industrial Aerodynamics 6 (1), 17–33. doi:10.1016/s0167-6105(97)00007-x
Laib, M., and Kanevski, M. (2016). Spatial modelling of extreme wind speed distributions in Switzerland. Energy Procedia 97 (1), 100–107. doi:10.1016/j.egypro.2016.10.029
Landviewer, (2021). Landviewer. Hingoli, India: EOS. Available at: https://eos.com/landviewer.
Lindsay, B., and Rothwell, J. (2008). “Modelling channeling and deflection of wind by topography,” in Advances in Digital terrain analysis. Editors Q. Zhou, B. Lees, and G. Tang (Springer), 383–486.
Locatelli, T., Hale, S., Nicoll, B., and Gardiner, B. (2019). ForestGALES and fgr, documentation and manual. Available at: https://github.com/tom-locatelli/fgr/blob/master/ForestGALES%20and%20fgr%20manual%2016092020.pdf.
Locatelli, T., Tarantola, S., Gardiner, B., and Patenaude, G. (2017). Variance-based sensitivity analysis of a wind risk model - model behaviour and lessons for forest modelling. Environ. Model. Softw. 87 (1), 84–109. doi:10.1016/j.envsoft.2016.10.010
Lukas Krejci, L., Kolejka, J., Vozenilek, V., and Machar, I. (2018). Application of GIS to empirical windthrow risk model in mountain forested landscapes. Forests 9 (2), 96. doi:10.3390/f9020096
Lukić, T., Micic Ponjiger, T., Basarin, B., Sakulski, D., Gavrilov, M., Markovic, S., et al. (2021). Application of Angot Precipitation index in the assessment of Rainfall Erosivity: Vojvodina region case study (north Serbia). Acta Geogr. Slov. 61 (2), 123–153. doi:10.3986/ags.8754
Magnusson, Linus (2021). Windgusts-Serbia Romania Skip to end of metadata. 201709. Available at: https://confluence.ecmwf.int/display/FCST/201709+-Windgusts+-+Serbia+Romania (Accessed July 10, 2019).
Marcu, F., Hodor, N., Indrie, L., Dejeu, P., Ilieș, M., Albu, A., et al. (2021). Microbiological, Health and Comfort aspects of Indoor air quality in a Romanian historical wooden Church. Int. J. Environ. Res. Public Health 18 (18), 9908. doi:10.3390/ijerph18189908
Ministerul Mediului, , and Apelor și Pădurilor, (2021). Gutâi - Creasta Cocoșului. ROSCI0089. Available at: https://natura2000.eea.europa.eu/Natura2000/SDF.aspx?site=ROSCI0089.
Morar, C., Lukic, T., Basarin, B., Valjarevic, A., Vujicic, M., Niemets, L., et al. (2021). Shaping sustainable urban environments by Addressing the Hydro-meteorological factors in Landslide occurrence: Ciuperca hill (Oradea, Romania). Int. J. Environ. Res. Public Health 18 (9), 5022. doi:10.3390/ijerph18095022
Morar, C., Lukic, T., Valjarevic, A., Niemets, L., Kostrikov, S., Sehida, K., et al. (2022). Spatiotemporal analysis of urban green areas using change Detection: A case study of Kharkiv, Ukraine. Front. Environ. Sci. 10, 823129. doi:10.3389/fenvs.2022.823129
Niemets, L., Mezentsev, K., Sehida, K., Morar, C., Husieva, N., Peresadko, V., et al. (2018). Innovation and Investment potential of region as a factor of its” Smart Transformation”: Kharkiv region case study (Ukraine). Visnyk V. N. Karazin Kharkiv Natl. Univ. Ser. "Geology. Geogr. Ecol. 49 (1), 137–159.
Nigmatova, S., ZhamangarA, A., Bayshashov, B., Abubakirova, N., Akmagambet, S., and Veerdenov, Z. (2021). Canyons of the Charyn river (South – East Kazakhstan): Geological history and Geotourism. Geoj. Tour. Geosites 34 (1), 102–111. doi:10.30892/gtg.34114-625
Petersen, G. (2013). Wind tunnel modelling of atmospheric boundary layer over hills, PhD diss. Hamburg: Universität Hamburg.
Pintilii, R., Andronache, I., Diaconu, D., Dobrea, R., Zelenakova, M., Fensholt, R., et al. (2017). Using Fractal analysis in modeling the dynamics of forest areas and economic impact assessment: Maramureș county, Romania, as a case study. Forests 8 (1), 25. doi:10.3390/f8010025
Ponjiger, M. T., Lukic, T., Basarin, B., Jokic, M., Wilby, R. L., Pavic, D., et al. (2021). Detailed analysis of spatial–Temporal variability of Rainfall Erosivity and Erosivity density in the Central and Southern Pannonian basin. Sustainability 13, 13355. doi:10.3390/su132313355
Quill, R., Sharples, J. J., Wagenbrenner, N. S., Sidhu, L. A., and Forthofer, J. M. (2019). Modeling wind direction distributions using a diagnostic model in the context of probabilistic fire spread prediction. Front. Mech. Eng. 5. doi:10.3389/fmech.2019.00005
Regia Nationala a Padurilor-Romsilva, (2017). Adresa 12002 din 08.06.2018 privind volumul estimat, inventariat, exploatat al produselor accidentale din doboraturile de vant inregistrate.
Săvulescu, I., and Bogdan, M. (2012). Geographic information system application for windthrow mapping and management in Iezer Mountains, Southern Carpathians. J. For. Res. 23 (2), 175–184.
Schelhaas, M. J. (2008). Impacts of natural disturbances on the development of European forest resources: Application of model approaches from tree and stand levels to large-scale scenarios. Diss. For., 28. doi:10.14214/df.56
Schindler, D., Grebhan, K., Albrecht, A., Schönborn, J., and Kohnle, U. (2012). GIS-Based estimation of the winter storm damage probability in forests: A case study from Baden-Wuerttemberg (Southwest Germany). Int. J. Biometeorol. 56, 57–69. doi:10.1007/s00484-010-0397-y
Stankov, U., Vasiljevic, Ð., Jovanovic, V., Kranjac, M., Vujicic, M. D., Morar, C., et al. (2019). Shared Aerial Drone Videos — prospects and Problems for Volunteered Geographic information research. Open Geosci. 11 (1), 462–470. doi:10.1515/geo-2019-0037
Suvanto, S., Henttonen, H., Nojd, M., and Pekka Makinen, H. (2016). Forest susceptibility to storm damage is affected by similar factors regardless of storm type: Comparison of thunder storms and autumn extra-tropical cyclones in Finland. For. Ecol. Manag. 381 (1), 17–28. doi:10.1016/j.foreco.2016.09.005
Szwagrzyk, J., Maciejewski, Z., Maciejewska, E., Tomski, A., and Gazda, A. (2018). Forest recovery in set-aside windthrow is facilitated by fast growth of advance regeneration. Ann. For. Sci. 75 (80). doi:10.1007/s13595-018-0765-z
Valjarević, A., Morar, C., Zivkovic, J., Niemets, L., Kicovic, D., Golijanin, J., et al. (2020). Long term Monitoring and connection between topography and cloud cover Distribution in Serbia. Atmosphere 12 (1), 964. doi:10.3390/atmos12080964
Valjarević, A., Zivkovic, D., Valjarevic, D., Stevanovic, V., and Golijanin, J. (2014). GIS analysis of land cover changes on the territory of the Prokuplje municipality. Sci. World J. 12 (1), 1–8. doi:10.1155/2014/805072
Valjarević, V., Morar, C., Zivkovic, J., Niemets, L., Kicovic, D., Golijanin, J., et al. (2021). Long term Monitoring and connection between topography and cloud cover Distribution in Serbia. Atmosphere 12 (8), 964. doi:10.3390/atmos12080964
Wagenbrenner, N., Forthofer, J., Butler, B., and Butler, (2019). Development and evaluation of a Reynolds-averaged Navier–Stokes solver in WindNinja for operational wildland fire applications. Atmosphere 10 (11), 672. doi:10.3390/atmos10110672
Wagenbrenner, N., Forthofer, J. M., Lamb, B. K., Shannon, K. S., and Butler, B. W. (2016). Downscaling surface wind predictions from numerical weather prediction models in complex terrain with WindNinja. Atmos. Chem. Phys. 16 (1), 5229–5241. doi:10.5194/acp-16-5229-2016
Weather underground, (2021). Romania weather history. Tăuții-Măgherăuș, Maramureș. Available at: https://www.wunderground.com/history/daily/ro/t%C4%83u%C8%9Bii-m%C4%83gher%C4%83u%C8%99/LRBM/date/2017-9-17.
Wellpott, A., Gardiner, B., and Ruel, J.-C. “Modelling wind damage for managed and old-growth stands in Québe,”, Germany, October 2009. Editors H. Mayer, and D. Schindler (Freiburg: Albert-Ludwigs-University), 116.Proceedings of the 2nd International Conference wind effects on trees Albert-Ludwigs-University of Freiburg.
Windninja firelab, (2021). Windninja. Available at: https://weather.firelab.org/windninja/.
Yang, M., Défossez, P., Danjon, F., and Fourcaud, T. (2014). Tree stability under wind: Simulating uprooting with root breakage using a finite element method. Ann. Bot. 114 (4), 695–709. doi:10.1093/aob/mcu122
Keywords: windthrows, windscape reconstruction, integrative modeling, Gutai Mountains, 3D models (three-dimensional)
Citation: Ilies G, Ilies M, Hotea M, Bumbak S-V, Hodor N, Ilies D-C, Caciora T, Safarov B, Morar C, Valjarević A, Berdenov Z, Lukić T, Mihajlović M, Liudmyla N and Vasić P (2022) Integrating forest windthrow assessment data in the process of windscape reconstruction: Case of the extratropical storms downscaled for the Gutai Mountains (Romania). Front. Environ. Sci. 10:926430. doi: 10.3389/fenvs.2022.926430
Received: 22 April 2022; Accepted: 22 September 2022;
Published: 12 October 2022.
Edited by:
Shuisen Chen, Guangzhou Institute of Geography, ChinaReviewed by:
Pratheesh C. Mammen, Kerala State Disaster Management Authority (SDMA), IndiaSaleh Yousefi, Agricultural Research, Education and Extension Organization (AREEO), Iran
Copyright © 2022 Ilies, Ilies, Hotea, Bumbak, Hodor, Ilies, Caciora, Safarov, Morar, Valjarević, Berdenov, Lukić, Mihajlović, Liudmyla and Vasić. This is an open-access article distributed under the terms of the Creative Commons Attribution License (CC BY). The use, distribution or reproduction in other forums is permitted, provided the original author(s) and the copyright owner(s) are credited and that the original publication in this journal is cited, in accordance with accepted academic practice. No use, distribution or reproduction is permitted which does not comply with these terms.
*Correspondence: Cezar Morar, cmorar@uoradea.ro