- 1Department of Textile Economics and Management, Zhejiang Sci Tech University, Hangzhou, China
- 2Department of Economics, Faculty of Business and Technologies, International Black Sea University (IBSU), Tbilisi, Georgia
- 3Innovative Research in Economics and Governance-IREG Cotonou (Benin), Porto-Novo, China
This research provides an in-depth understanding of cotton production in Benin. The analysis explored the effects of cotton production on economic growth using a vector error correction model (VECM). Prior to the VECM, a descriptive analysis was conducted on a time series database collated over 56 years (1965–2021) from national and international organizations including World Bank, the United States Department of Agriculture, and the National Accounts. Depending on the availability of the information over this period, the data were trimmed down to enable a better overview of the trend for variable of interest. Thus, the review of the trend for agricultural land (hectares) for cotton observed from 1965 to 2021 revealed that the trend evolves over the years in three different patterns, while the yields demonstrate four patterns over the same period. For the VECM analysis that permits to understand the long- and short-term relation, the time bound covers 30 years from 1990 to 2019 given the availability of data for all the variables used for the model. The findings highlighted that a strong and positive connection is found between cotton export and economic growth and a long-term relation between the human capital and the economic growth in Benin. A rapid human capital development will increase quality of the employment generation, and the country’s economy will adjust upward. Furthermore, a short-run coefficient unveils feedback necessary in a relative level of investment to bring back the economic growth to equilibrium. From these findings discussed in this study, the government of Benin is commended to diversify the production of cotton through a town target policy.
1 Introduction
Environmental degradation and energy poverty are major issues in the current world scenario (Sun et al., 2022). Agriculture plays a very important role in some major economies (United States, Canada, and Russia) and in the reduction of famine worldwide. Also, it demonstrates a tremendous impact on the industrialization process through the supply of raw materials (Cotton, Rubber, Sugarcane, Cereals, and many others) to many transformation industries. Agroforestry and agriculture demonstrate a significant part in the economies of West African countries. They account for about 40% (with cotton being the most important contributor) of the gross domestic product (GDP) and provide a wide range of ecosystem benefits to local residents (Djihouessi et al., 2022).
Cotton production plays key role in many economies across the world. With an estimated $600 billion annual economic impact worldwide, cotton is the leading natural fiber produced and commercialized in the world (Ahmad, 2014). The industrialization of cotton sector involves about 150 countries and provides income to about 100 million families (Tarazi et al., 2019; Meyer, 2020). Cotton output in 2019 was estimated to be worth USD 46 billion, with global trade worth USD 15 billion. In 2018/19, global cotton mill use reached 26.7 million tons, the same amount as in 2007/08.
In Sub-Saharan Africa (SSA), cotton, also known as white gold, gained attention from government and through scientific research, and it is considered as an essential commodity crop that is expected to grow by 14% by 2025 and about 15% of the world’s cotton lint exchanges, according to SSA (OCDE/FAO, 2016). Cotton is essential to the economies of several African countries, providing livelihoods to lots of small farmers around the world. Cotton is experiencing a revival in other countries, such as Ethiopia, and as well as voluntary certification programs like the global organic textile standard to encourage and ensure the sector’s sustainability (Partzsch and Kemper, 2019; Partzsch et al., 2019).
Cotton development in SSA faces a significant challenge in terms of environmental sustainability. In recent time, studies have been undertaken in the sector to revamp the value of African countries’ cotton production. For instance, study led by the World Bank in 2008 found out the economic advantages of cotton exports to developing countries and the disadvantages faced by producing countries due to lack of key technologies (Estur and Gergely, 2010). Next, the cotton sector plays a major role in the growth of economies and the improvement of living standards as well as purchasing power of the rural population. Also, (Morris, 1989) indicated that countries that grow and process cotton fiber emerged as a major provider of foreign exchange in nine emerging countries, including Benin.
However, in the past 5 years, Benin’s cotton industry has been in a major crisis resulting in a dramatic drop in the crop production. Looking at the process, cotton production in the country is heavily dependent on imported inputs, which increases the cost in production and is most time challenging for peasants and producers. Given the importance of the sector, many studies focused on ways to improve and stimulate the sector. Thus, according to (Togbé et al., 2012), the pest control technique in the production chain improved the cotton yields and its quality. As a main source of foreign exchange in Benin, the production of cotton, over time, evolved in a saw path before gaining since 2016, a remarkable and steady increase with the highest record tolled at 597,985 tons at the end of the 2020 agronomical season. Indeed, after 4 years (2012–2016) of crisis due to the extreme interference of politics, which has dropped its performances, the cotton sector recovered with the Inter-professional Association of Cotton (AIC) bouncing back within the system as the system core managerial body and structural umbrella. The progressive disengagement of the state from the provision and distribution of inputs accelerated and reinforced the liberalization of the cotton commodity chain under this umbrella. Thus, private national operators increasingly took over import and distribution operations, and their numbers grew over time. As a result, AIC has become the executing agency for key chain operations such as cotton research, seed production, producer training and supervision, cotton grain quality control, fiber grading, and road maintenance. Some of these tasks are delegated to government-run technical services and service companies. Along the line, the functioning of the AIC1 and some of quantified results are rewarding. For example, Benin’s five-year average production is 435 kg/ha, which is significantly higher than that of the other franc zone’s countries’ five-year average output of 393 kg/ha.
Researchers estimated that the cotton industry employs 30% of Benin’s workforce. In the past, previous studies focused mainly on the impact of agricultural activities on the economy of developing countries in general. An in-depth study to analyze the contribution of cotton production to the economic growth of Benin will be vital for our research. However, as these recent performances remain visible, it is worth revisiting the nexus between cotton agricultural production and the economic growth in Benin. In addressing this aim, the present research develops two hypotheses related to the interaction between cotton production and economic growth, as follows: (H1) the impact of increasing production of cotton on economic growth is positive; (H2) the gains from improving human capital affect in long run both cotton production and economic growth. The findings from testing these hypotheses through a vector error correction model (VECM) will feed into the existing literature. Thus, while the next section discusses the relevant literature, the third section will detail the methodology and data. The final section will present and discuss the results and wrap up the study with socioeconomic policy implications.
2 Literature Review
Agriculture exhibits a crucial contribution to the economic development of a nation (Gollin, 2010; Brückner, 2012; Cao and Birchenall, 2013; Fayçal and Ali, 2016). A case study of Benin as a WAEMU member state (Amoussouga Gero and Egbendewe, 2020) showed that enhancing agricultural productivity could boost the overall economic growth through a mechanism that reduces the trade deficit and subsequently increases household income and government revenues. According to (Awokuse and Xie, 2015), a strong agricultural contribution is a driving factor of economic growth in a country. Also, (Los and Gardebroek’s (2015) study implies that the agricultural sector performs different roles in different stages of economic development. Along with investment and the private sector, the share of agriculture demonstrates a significant added value to the country’s GDP (Mijiyawa, 2013). Similar to this, (Amao et al., 2021) recommended that reforms designed to improve the accessibility to loans and the productivity of cash crops, including cotton, will boost the country’s export and economic growth. Güzel and Akin (2021) found that increased agricultural production during the industrialization process in middle-income countries can stimulate a growing economy. However, the research findings did not conclude a significant connection between trade openness and a growing economy. Furthermore, other agricultural products such as tea, cereals, and tobacco significantly impact the GDP of the Indian economy. In contrast, some agricultural products, such as coffee and sugarcane, demonstrate the reverse effect (Urmi Pattanayak and Minati Mallick, 2019). In support of agriculture’s role in economic growth, (Agboola et al., 2020) explained unidirectional causality from various sub-sectors of agriculture such as forestry, agricultural production, fisheries, and life stock to economic growth.
Besides this thesis, other scholars concluded that agriculture and economic growth are related to the sector’s profitability. Boosting agricultural profitability could be an essential pathway toward poverty reduction and socioeconomic development. Thus, according to (Matsuyama, 1992), such products can only be achieved in an unregulated environment where states demonstrate a comparable production benefit in agriculture. However, the rural income could not increasingly improve alongside the productivity increase over the years because of the poor quality of commercial transactions in the agricultural sector (Bautista, 1986). The theoretical and empirical research findings of (Mellor, 1983) provide useful indications of how increased agricultural production sustains economic development and poverty reduction. Thus, the agricultural sector is considered a labor-intensive sector but with very low wages (Khor and Feike, 2017).
It is worth noting that the relationship between agriculture and economic growth has lately been re-examined. Using analytical and dynamic models, (Yang and Zhu, 2013) employed the double-sector dynamic United Kingdom model and demonstrated that in the absence of improved agricultural output, an agricultural economy could not compensate for the restrictions of natural assets. Similar to this, (Irz and Roe, 2000); (Ogunlesi, 2018) concluded on a minimum growth rate at which agricultural performance is required to overcome the growing population and the subsequent Malthusian trap.
On the other hand, various studies from developing countries (Dorosh and Thurlow, 2014) show evidence of aggregate improvements in economic performance and a decline in poverty that can be accomplished with agricultural productivity expenditures. For instance, (McArthur and Sachs 2014) Uganda’s CGE mod showed how substantial economic growth might be achieved by focusing development aid on policy finance to improve agricultural production efficiency. Furthermore, with an auto-regressive distributed lag model, studies indicated that the sustainability of agricultural production would significantly affect agricultural growth, even though it will negatively impact the long run economic growth (Fayçal and Ali, 2016).
Cotton is seen as an integral part of the livelihoods of many countries in the world and as an important cash crop in West African countries, including Benin. Since 1960, world cotton production increasingly evolved, resulting in a slight annual improvement of 1.7%. Across the world, during the year 2018–2019, China was the world’s largest producer with nearly six million tons, followed by India with 5,879,000 tons and the United States with 4,004,000 tons. In 2016, USAID indicated that cotton contributed 3–10% of the GDP in Benin, Burkina Faso, Mali, and Chad, among other key producers of cotton, where the crop is heavily produced and seen as a main source of income and as a crucial contributing factor to poverty reduction (Sabesh and Prakash, 2018).
In particular, according to the USDA (2019), Benin was the largest cotton producer in the West African region in the 2019–2020 cotton season (1,450 thousand bales). As a result of this, it is expected to be the same this coming year. Within the West African franc zone, it is followed by Mali (1,425), Burkina Faso (1,200), Côte d’Ivoire (830), Cameroon (565), Togo (265), Chad (200), Senegal (35), and Niger (9). Looking at the volume of cotton trade (import and export operations), SSA is ranked as the third top with 12% of the overall cotton export. In addition, (Baffes, 2007) and (Gray and Moseley, 2008) argued that cotton total merchandise exports ranging from 25% to 45% contributed to West African countries’ GDPs and remains many households’ sources of income.
In Benin, according to World Bank. (2016), agriculture contributed for 4.33% to the country’s GDP from 1994 to 2014. For a decade, Benin’s economy is agriculture reliant, with cotton as its main cash crop (Gergely, 2009). The cotton sector directly or indirectly generated more than 40% of rural employment and provided for nearly 50% of the population. According to the National Institute of Statistics and Economic Analysis, it contributed 13% to the GDP. In the same vein, according to the platform of civil society actors in Benin (PASCiB, 2013), cotton was the major contributor to Benin’s rural producers’ incomes. In as much as this is attributable to the cotton sector, this study, in pursuing its keen interest, will drive into understanding how cotton production could affect the economic growth in Benin in the long run.
3 Data and Methodology
3.1 Data and Key Variables
This study utilizes macroeconomics data collated from 1965 to date. The dependent variable is “The Gross Domestic Product (GDP) per capita” drawn from the world development index database (World Bank. 2019). Other relevant variables include cotton production, the area under cotton production, and the cotton productivity assembled from production, supply, and distribution database of the United States Department of Agriculture. The gross fixed capital formation (GFCF), also called “investment,” as well as human capital to measure respectively investment in property developments, the total employment, or labor force in the cotton production chain are also used as independent variables.
As can be observed, regression models are frequently used to examine production and economic difficulties, and most research publications (Hollinger and Staatz, 2015; Nyamekye et al., 2021; Ngong et al., 2022) focus on the relationship between agriculture and other factors in developing countries.
It is good to add that other research employs a non-linear auto-regressive distributed lag framework to address non-linearity arising from abnormal data distribution (Sun et al., 2022).
However, little relevant research exists that specifically investigates that element of agriculture in cotton farmers in West African countries (Benin, Mali, and Burkina Faso). We used the VEC model to investigate the implications or influence of cotton output on economic growth in the Benin Republic.
3.2 Analytical Procedure
This study will use a VECM which can explore not only a long-term causal relationship between the two major variables but also gives the advantage to underscore the short-term impact. In the process, before running a VECM model, one may perform a unit root test to ensure the stability of each series in the model. This study will perform the augmented Dickey-Fuller (ADF) test. However, for the sake of robustness, the Phillips-Perron (PP) test will subsequently run to control the volatility of the series over the long period under review.
3.3 Empirical Model Specification
In reference to (Ren et al., 2020; Ai et al., 2021), the impact of cotton production on economic growth will be assessed through the following model.
The econometric expression of Eq. 1 is the following:
where β0, β1, β2, and β3 > 0, EXPCT is the quantity of cotton exported, HC denotes the human capital, and LAB is the labor employed in the labor market; they are all likely to be positively related to economic growth. Also, μ signifies the error term and represents the year period.
Taking log on Eq. 2 turns to Eq. 3:
3.3.1 Unit Root Test
As a macroeconomic long-term series, estimating the root unit and ensuring they are not integrated is essential. This test is necessary to evaluate variable stability and, thus, to avoid biased findings. With the ADF and the PP test, the following hypotheses are tested:
H0: β1 = β2 = β3 = 0 → the null hypothesis of this model is that co-integration is not available.
H1: β1≠β2≠β3≠0 → the alternative hypothesis asserts that co-integration exists.
If the F-statistics computed value is higher than the top critical limits, then the null hypothesis of no long-lasting link is rejected. Otherwise, it is accepted if the calculated F-statistics value is below the critical limit values. The PP test ensures reliability of the results.
3.3.2 Co-Integration Analysis
The co-integration test is performed to confirm whether the VECM will run with stationary time series. This leads to a discriminatory criterion that determines the best order of lag and evaluates the necessary amount of co-integration by co-integration testing. Also, the Engle Granger two-stage method and the Johansen maximal probability method were most frequently used (Shao et al., 2021).
3.3.3 Estimation
In addition to the descriptive analysis, the final equation that will be used to explore the casual long and short-term relations is given as follows:
4 Results and Discussion
4.1 Trend of Cotton Production: Yield and Exports
The descriptive analysis shows that the trend for agricultural land (hectares) for cotton evolves over the years in three different patterns, while the yield demonstrates four patterns over the same period. Looking at the yield, after a challenging decade, the trend shows, around 2010, a noticeable relaunch of the sector with the arrival of AIC and the settlement of new leadership, which offers, until 2020, a positive outlook for Benin’s cotton future (Figures 1, 2).
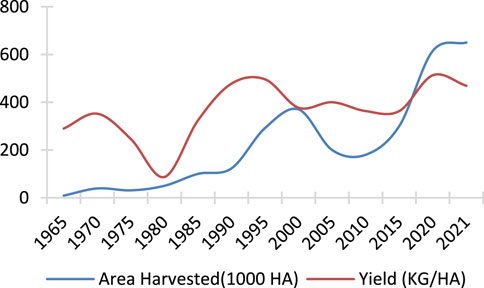
FIGURE 1. Cotton yield and agricultural land in Benin. Source: Authors with data from USDA, https://apps.usda.gov/psdonline.
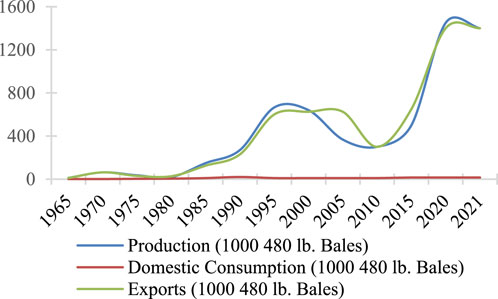
FIGURE 2. Cotton production in Benin. Source: Authors with data from USDA, https://apps.usda.gov/psdonline.
Also, this period marks the period where the government and private business engaged in modernizing the entire cotton supply chain and providing more timely seed and fertilizer inputs. Thus, Benin’s five-year average yield is 435 kg/ha, which is much higher than the franc zone’s five-year average production of 393 kg/ha. Moreover, looking at the production and exports, the graph suggests similar progress with a larger part being exported. Indeed, since 2010, reforms primarily included the privatization of input supply, the introduction of private ginners, and the formation of inter-professional bodies to take over sector management. This resulted in an increase in cotton production and export of up to 1,450 thousand bales.
In sum, this recent trend reinforces the assertion that cotton is the main cash crop for exports, even though the yield does not demonstrate a stable trend over time. Therefore, to bridge the gap of missing years, the government of Benin2 commenced, since 2016, to diversify the agriculture sector and consider new crops such as cashew nuts, rice, and pineapple. This enables investment of agro-value chains, safety standards, and promoting research and development through the National Program for Agricultural Research known as INRAB.3
4.2 Emergence of Economic Growth
Economic growth is the continuing increase of GDP per capita which is determined by dividing the gross domestic product by the mid-year population, according to the World Development Indicators. It is the sum of the gross value created in the economy by all residential manufacturers, plus product taxes, minus subsidies not included but estimated without taking into consideration depreciation of produced items or natural resource depletion and deterioration. Next, in line with this definition, the Benin National account reveals that structural characteristics of the Benin economy are predominantly agricultural. Regarding the structure of Benin economics, agriculture is the main contributor of the GDP, followed by trade, transport, and key services. For instance, the analysis of percentage of contribution reveals an increasing trend for agriculture (Table 1).
However, the main concern is how does it link to the overall average variation of economic growth. The GDP per capita yearly growth rate based on constant local currency varied considerably across the years, and stable growth has not occurred. Thus, from −0.06 in 1970, the GDP per capita growth (annual %) evolved at an average of 0.30 between 1970 and 1990, while the GDP per capita changed at an average rate of 1.10 since then to 2010 before an average annual rate of 2.09% during the last decade (2011–2022) (Figure 3).
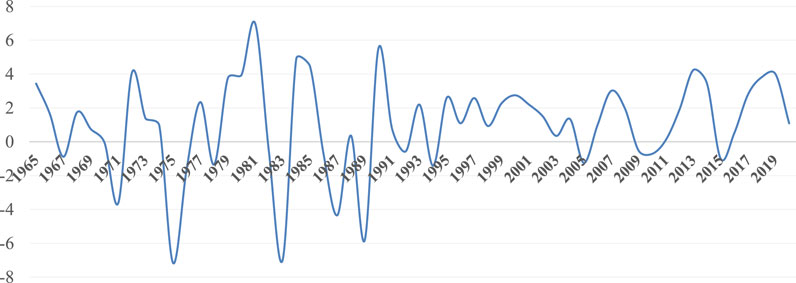
FIGURE 3. Trend of the annual percentage growth rate of gross domestic product per capita. Source: World Development indicator, https://data.worldbank.org/indicator/NY.GDP.PCAP.KD.ZG?locations=BJ.
Real GDP growth has been highly volatile throughout most of the country’s post-independence history, related to its dependence on commodity exports (cotton) and transit trade with Nigeria. However, between 2015 and 2019, Benin recorded a solid economic outcome, which is depicted by a steady but robust GDP per capita growth regardless the Covid-19 pandemic and the border closure of Benin’s main economic partner. Politics put in place showed the country’s relative resilience, and though the economic activity, mainly export-import slowed down and yielded a slight drop-down of GDP annual growth at 1.01%, as shown in Figure 3.
4.3 Analysis of the Empirical Results
In the subsequent analysis, the time bound covers 30 years from 1990 to 2019 given the availability of data for all the variables used for the VECM analysis. The summary of the data for the variables utilized in this investigation showed that the average value for ln GDP is 6.99, which means that the estimated average of the economic growth over the period under review is 991.53 USD (constant 2010 US$) (Table 2).
Similar to this, the estimated value for investment, human capital, and export are, respectively, and in the same order equal to 17.51% GDP: 3,106,912.42 of the total population and 546,844.63 480 lb. Bales.
The bi-variate relationship between each of the independent variables and economic growth over the period under review indicates that there exists a strong positive relationship between cotton exportation, the quality of people (farmers directly for cultivation and harvest of the cotton crop and indirectly those somehow on the industrial labor force) involves in the cotton value chain, the type of equipment or investment done to support the value chain, and the economic growth of the country.
4.3.1 Unit Root Tests
The unit ratio could lead to a misleading conclusion in the underlying variable or lead to a fake regression (Vau and Bourlès, 2021). To avoid this, check first and foremost whether the sequence contains a unit root. If a unit root is discovered, it means the sequence is non-stationary, necessitating the use of first differences. In this study, the ADF and PP tests are used simultaneously to examine the dropout rate separately from the time trend (Table 3).
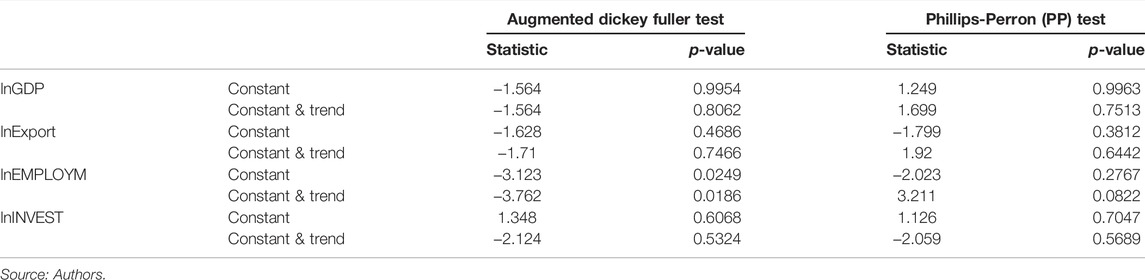
TABLE 3. The model variables were subjected to augmented Dickey-Fuller (ADF) and Phillips-Perron (PP) unit root tests.
Apart from EMPLOYM, the remaining original series failed the ADF test, regardless of whether either intercept terms or the time trend was taken into account, indicating a non-stationary time series, which was confirmed by the PP test. As a result, we cannot reject the null hypotheses for all series. EMPLOYM is a stationary time series that does not need to be lagged.
4.3.1.1 Identification of Optimal Lag Order
The criterion test for selecting the optimal lag length required to differentiate our series is presented in the table below.
It includes the Akaike criterion for information (AIC), the SBIC, the Hannan-Quinn information criterion, and the log-likewood criterion. The SBIC is the final prediction error (FPE) (LIK). This document based its judgment on SBIC, although the function of discrimination differs from criterion to criterion (Table 4). Also, the probability ratio test shows that there are four in the best possible lag order, although, in addition to Akaike, Hannan-Quinn, and Schwartz (SBIC) data criterion, one in the final prediction error seems the most appropriate.
4.3.1.2 Johansen Co-Integration Test
Before running the co-integration test, the unit ratio will be re-run on the lagged original variables based on the selected ordered lag. The following table that synthesizes the results suggests that all series are now stationary series. The ADF and PP tests show that the calculated value of the statistics for each of the three variables is greater than the upper critical bound values (see annex) and is associated with statistically significant (1 and 5%) p-values (Table 5). Therefore, the neutral hypothesis of no long-term relationship is denied, and the Johansen test can be performed easily.
After the unit root properties have been satisfied, the relationship of long-term co-integration of variables is studied. A wide range of tests exist to analyze the co-integration (Engle and Granger, 1987; Juselius and Johansen, 1990; Pedroni, 2000; Pradhan et al., 2014). (Engle and Granger, 1987) presented a two-step test to detect co-integration, but this test is incapable of handling circumstances in which many co-integrating relationships are feasible. This issue is addressed with (Juselius and Johansen, 1990), who introduced two distinct likelihood ratio tests that allow linearly independent co-integrating vectors to be determined. In this study, we apply this latter technique (Table 6). It will help underpin the co-integrating rank of a VECM. The study looked for co-integration links between variables using the Johansen test with the optimal first-order lag.
Only when ranked, 1 does the trace statistic fall below the crucial value under 95% confidence, indicating that the null hypothesis is accepted at a 5% threshold, whereas all others reject the null hypothesis with co-integration connection, as seen in the (Table 6). As a result, the Johansen Co-Integration test reveals that the three variables demonstrate only one co-integration relationship.
4.3.2 Model for Vector Error Correction
Co-integration is a valuable method for the modeling of time series data long-term relationships, as explained by many studies (McGowan and Ibrahim, 2012; Andrei and Andrei, 2015; Loves et al., 2021). It indicates that time series are connected with an error correction model. The relationship between the first-order difference hysteresis of each variable is then better understood, allowing for a better understanding of long run equilibrium dynamics. Also, it highlights a short-term method for dynamic adjustment which illustrates how variables adapt when out of balance.
It helps to measure the factors that push the connection to a long-term balance more easily with the adjustment coefficients. In reference to empirical model developed, the estimated long-term and short-term relationship through the VECM estimation provides the findings synthesized in the (Table 7) In the equations, the L1. _ce1 term is the lagged error correction term that presents the long-term variation, while the models constructed under model (1)–(4) represent the short-term variations. The fitness of the VCEM regressions provided by the adjusted R squared reveals that model (4) demonstrates the highest good fit with a value 0.99 greater than 75%. To a certain extent, it is followed by models (1), while models (2) and (3) appear with a low fit. Model (4) exhibits a positive and large (at the 1% level) error correction coefficient, and (Table 7) shows that if human capital increases rapidly, the country’s economy will adapt higher as well, and growth will finally return to the long-term equilibrium state. In addition, only the short-run coefficients for first lagged reveal a negative correlation in the same equation with a value significantly different from zero. In the other models, the negative and significant but at a different level of the error correction coefficient indicates the negative feedback necessary in relative level of exports and investment to bring back the economic growth equilibrium. The short-run relations are insignificant.
4.3.2.1 Vector Error Correction Model Stability Test
To investigate the impulse response results, the VEC model was subjected to a stability test (Figures 4, 5). The following are the results of a typical root test (Table 5). The diagram represents its own values of the matrix accompanying the real part at x-axis and the imaginary part at y-axis. All the adjacent unit values are included in the unit circle, with the exception of the VECM three-unit roots. Thus, the model is stable, meaning that an examination of the pulse response can be performed by a study.
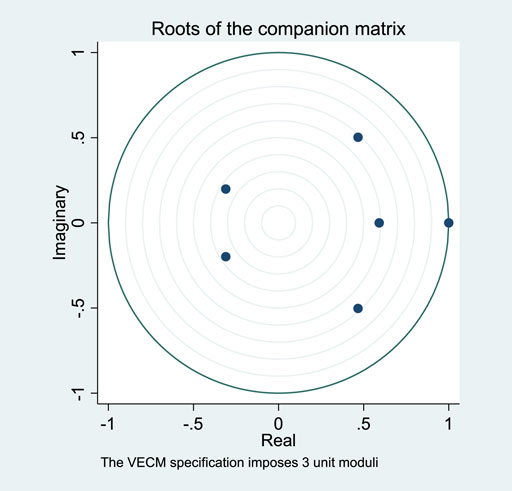
FIGURE 5. System stability discrimination diagram of the vector error correction model. Source: Authors.
4.3.2.2 Error Residual Normality Test
After performing a VECM, checking the null hypothesis that the disturbance in each equation is normally distributed is important. In so doing, this study run three different tests (Table 8). In light with the statistics for which the probabilities are displayed in Table 8 it is clear that the residuals do not suggest abnormality, and therefore, we cannot reject the null hypothesis.
5 Conclusion, Policy, and Future Prospects for Study
With a VECM, this study empirically examined the nexus between cotton production and the economic growth in Benin. Before the model, a descriptive study was conducted utilizing macroeconomic data collected by national and international agencies such as the World Bank, the US Department of Agriculture, and the National Accounts, generally from 1965 to 2021. This study utilized the GDP per capita as a proxy for economic growth. At the same time, other major explanatory variables included cotton productivity, investment in terms of GFCF, and employment and export levels in the country. The analysis revealed a strong positive connection between cotton export and economic growth. In addition, the results suggest a long-term relationship between human capital and economic growth in Benin. Indeed, a rapid human capital development will increase the quality of employment, and the country’s economy will adjust upward.
Furthermore, a short-run coefficient unveils feedback necessary in the relative level of investment to bring back the economic growth to equilibrium. In the same vein, in some studies in Nigeria, the least-squares results revealed that cash crops and food crops demonstrated a positive and significant impact on economic growth in Nigeria. In contrast, livestock demonstrated a positive and insignificant impact on Nigeria’s economic growth (Igoni and Anthony Nwadioha, 2021). Also, a recent study conducted in another cotton-dependent country (Burkina Faso) showed that the value-added of agriculture for households constitute a vector of development and will contribute to 57.33% of final household consumption expenditures, while other sectors such as industry and services contribute only 2.03% and 4.42% (Traore et al., 2021).
These findings nurture a couple of suggestions enlightening henceforth decision making for economic growth in Benin.
The first policy suggestion urges the government of Benin to address the agricultural sector of cotton. The deteriorating performance of this crop (in terms of productivity) will affect the largest value chain, especially the export, which demonstrates a strong long run implication on growth. In addition, as a low-middle country, the government of Benin, to fast track its steps towards a developed country, needs to diversify the production of cotton through a town or district target policy. Indeed, this policy may consist of identifying towns in Benin that could enable large-scale cotton production using advanced technology and cotton seeds. Similar to this, the enhancement of human capital involved in the cotton production chain is key in scaling up production and reducing the time of human resources in the process. Therefore, it is also recommended that the government make coordinated attempts to ensure that farmers, especially small-scale farmers, receive easy access to the financial aids and grants provided. The funds should be disbursed appropriately and adequately without any hitch. Also, we recommend policy by improving environmental quality and fiscal management, as explained in a recent study (Sun and Razzaq, 2022).
To conclude, albeit this study did not perform a detailed comparative and cross-section analysis across the countries in the region, it is worth that the government pays attention to countries’ comparison analysis to learn from others and leverage the strengths, challenges, and achievements of other countries in the relations between cotton production and economic growth. Nevertheless, although production increased in recent years in Benin, the cotton sector is still confronted with certain shortcomings that need to be addressed externally. Then, it will be good for future research to focus on climate change in national and regional level policies as a serious threat to socioeconomic development and agricultural productivity in West Africa.
Data Availability Statement
The original contributions presented in the study are included in the article/Supplementary Material, and further inquiries can be directed to the corresponding author.
Author Contributions
DN: conceived the idea, performed the data collection, statistical analysis contributes to the writing of the manuscript. CF: Proofread the manuscript and gave guidance throughout the process of this study. AD: Formal analysis, Writing-review and editing. SR: Proofread, statistical analysis contributes to the writing of the manuscript.
Conflict of Interest
The authors declare that the research was conducted in the absence of any commercial or financial relationships that could be construed as a potential conflict of interest.
Publisher’s Note
All claims expressed in this article are solely those of the authors and do not necessarily represent those of their affiliated organizations, or those of the publisher, the editors, and the reviewers. Any product that may be evaluated in this article, or claim that may be made by its manufacturer, is not guaranteed or endorsed by the publisher.
Footnotes
1In recent years, Benin’s yield trend grew following investments in cotton supply chain modernization by government and private business and timely provision of seeds and fertilizers
2Programme d’Action du Gouvernement- PAG
3Implementation Completion Report ICR00004927, July 2020
References
Agboola, M. O., Bekun, F. V., Osundina, O. A., and Kirikkaleli, D. (2020). Revisiting the Economic Growth and Agriculture Nexus in Nigeria: Evidence from Asymmetric Cointegration and Frequency Domain Causality Approaches. J. Public Aff. 22. doi:10.1002/pa.2271
Agrawal, K. K., and Mallick, Minati (2019). An Econometric Analysis of Agricultural Production and Economic Growth in India. Indian J. Mark. 49 (11), 56–65. doi:10.17010/ijom/2019/v49/i11/148276
Ahmad, S. (2014). Cotton Production and Uses Agronomy. Crop Protection and Postharvest Technologies, Springer Nature Singapore. doi:10.1002/14356007.a22
Ai, H., Guan, M., Feng, W., and Li, K. (2021). Influence of Classified Coal Consumption on PM2.5 Pollution: Analysis Based on the Panel Cointegration and Error-Correction Model. Energy 215, 119108. doi:10.1016/j.energy.2020.119108
Amao, O. D., Antwi, M. A., Oduniyi, O. S., Oni, T. O., and Rubhara, T. T. (2021). Performance of Agricultural Export Products on Economic Growth in Nigeria. 5005 11 (1), 47–52. doi:10.18488/journal.ajard.2021.111.47.52
Amoussouga Gero, A., and Egbendewe, A. Y. G. (2020). Macroeconomic Effects of Semi-subsistence Agricultural Productivity Growth: Evidence from Benin and Extension to the WAEMU Countries, Scientific African 7. Elsevier B.V, e00222. doi:10.1016/j.sciaf.2019.e00222
Andrei, D. M., and Andrei, L. C. (2015). Vector Error Correction Model in Explaining the Association of Some Macroeconomic Variables in Romania. Procedia Econ. Finance 22, 568–576. doi:10.1016/s2212-5671(15)00261-0
Awokuse, T. O., and Xie, R. (2015). Does Agriculture Really Matter for Economic Growth in Developing Countries? Can. J. Agric. Economics/Revue Can. d'agroeconomie 63 (1), 77–99. doi:10.1111/cjag.12038
Baffes, J. (2007). The "Cotton Problem, West and Central Africa: The Case for Domestic Reforms, 11. Online.
Bautista, R. (1986). Effects of Increasing Agricultural Productivity in a Multisectoral Model for the Philippines. Agric. Econ. 1 (1), 67–85. doi:10.1016/0169-5150(86)90007-1
B. McGowan, C., and Ibrahim, I. (2012). Demonstrating the Use of Vector Error Correction Models Using Simulated Data. Jbcs 8 (4), 377. doi:10.19030/jbcs.v8i4.7031
Brückner, M. (2012). Economic Growth, Size of the Agricultural Sector, and Urbanization in Africa. J. Urban Econ. 71 (1), 26–36. doi:10.1016/j.jue.2011.08.004
Cao, K. H., and Birchenall, J. A. (2013). Agricultural Productivity, Structural Change, and Economic Growth in Post-reform China. J. Dev. Econ. 104, 165–180. doi:10.1016/j.jdeveco.2013.06.001
Djihouessi, M. B., Degan, A., Mpo Yekanbessoun, N. t., Sossou, M., Sossa, F., Adanguidi, J., et al. (2022). Inventory of Agroecosystem Services and Perceptions of Potential Implications Due to Climate Change: A Case Study from Benin in West Africa. Environ. Impact Assess. Rev. 95, 106792. doi:10.1016/j.eiar.2022.106792
Dorosh, P., and Thurlow, J. (2014). Can Cities or Towns Drive African Development? Economywide Analysis for Ethiopia and Uganda, World Dev., 63. 113–123. doi:10.1016/j.worlddev.2013.10.014
Engle, R. F., and Granger, C. W. J. 1987, Co-Integration and Error Correction: Representation, Estimation, and Testing). Econometrica ,. 55(2), 251–276. doi:10.2307/1913236
Estur, G., and Gergely, N. (2010). The Economics of Roller Ginning Technology and Implications for African Cotton Sector. Econ. Roll. Ginning Technol. Implic. Afr. Cotton Sect. 129 (129). doi:10.1596/27585
Fayçal, M., and Ali, H. M. (2016). Economic Growth and Government Subventions for Agriculture Sector in Algeria: An ARDL ModelArab Economic and Business Journal. Arab Econ. Bus. J. 11 (Issue 2), 105–114. doi:10.1016/j.aebj.2016.10.001
Gergely, Nicolas. (2009). The Cotton Sector in Benin. World Bank, Africa Region Working Paper Series 2009 No.125 pp.vi + 45 pp.
Gollin, D. (2010). Chapter 73 Agricultural Productivity and Economic Growth. In Handbook of Agricultural Economics (1st ed., Vol. 4, Issue 09). Elsevier. doi:10.1016/S1574-0072(09)04073-0
Gray, M., and Moseley, W. G (2008). Hanging by a Thread: Cotton, Globalization, and Poverty in Africa.
Güzel, A. E., and Akin, C. S. (2021). The Role of Agricultural Productivity in Economic Growth in Middle-Income Countries: An Empirical Investigation. Ejem 13 (1), 13–26. doi:10.20885/ejem.vol13.iss1.art2
Hollinger, F., and Staatz, J. M. (2015). Agricultural Growth in West Africa. www.org/publications.
Igoni, S., and Anthony Nwadioha, N. (2021). Impact of Agricultural Credits on the Nigerian Economic growthQuest Journals Journal of Research in Business and Management. www.questjournals.org, 9, Issue 7. doi:10.21474/ijar01/13816
Irz, X., and Roe, T. (2000). Can the World Feed Itself? Some Insights from Growth Theory. Agrekon 39 (4), 513–528. doi:10.1080/03031853.2000.9523669
Johansen, S., and Juselius, K., 1990. (1990a). Maximum Likelihood Estimation and Inference on Cointegration - with Applications to the Demand for Money. 52(55), 169–210. doi:10.1111/j.1468-0084.1990.mp52002003.x
Khor, L. Y., and Feike, T. (2017). Economic Sustainability of Irrigation Practices in Arid Cotton productionWater Resources and Economics. Water Resour. Econ. 20 (Issue September), 40–52. doi:10.1016/j.wre.2017.10.004
Los, E. J., and Gardebroek, C. (2015). Unravelling the Links between Agriculture and Economic Growth: A Panel Time Series Approach for Post-WW II Africa. in“10th New Frontiers in African Economic History Workshop -Is Africa Growing out of Poverty?”, March, 20–31.
Loves, L., Usman, M., Warsono, Widiarti, Widiarti, E., and Russel, E. (2021). Modeling Multivariate Time Series by Vector Error Correction Models (VECM) (Study: PT Kalbe Farma Tbk. And PT Kimia Farma (Persero) Tbk). J. Phys. Conf. Ser. 1751 (1), 012013. doi:10.1088/1742-6596/1751/1/012013
Matsuyama, K. (1992). Agricultural productivity, comparative advantage, and economic growth. J. Econ. Theory 58 (2), 317–334. doi:10.1016/0022-0531(92)90057-O
McArthur, J. W., and Sachs, J. D. (2014). A General Equilibrium Model for Analyzing African Rural Subsistence Economies and an African Green Revolution. SSRN J. doi:10.2139/ssrn.2450842
Mellor, J. W. (1983). Agricultural Growth-Structures and Patterns. Growth Equity Agric. Dev. 216–228. http://ageconsearch.umn.edu/bitstream/182449/2/IAAE-CONF-142.pdf.
Mijiyawa, A. G. (2013). Africa's recent economic growth: What are the contributing factors? Afr. Dev. Rev. 25 (3), 289–302. doi:10.1111/j.1467-8268.2013.12030.x
Morris, D. (1989). The importance of Cotton to developing countries. Seminaire d’Economie de Sociol. II–15, 135–146.
Ngong, C. A., Onyejiaku, C., Fonchamnyo, D. C., and Onwumere, J. U. J. (2022). Has bank credit really impacted agricultural productivity in the Central African Economic and Monetary Community? Ajeb. doi:10.1108/ajeb-12-2021-0133
Nyamekye, A. P., Tian, Z., and Cheng, F. (2021). Analysis on the Contribution of Agricultural Sector on the Economic Development of Ghana. Ojbm 09 (03), 1297–1311. doi:10.4236/ojbm.2021.93070
Ogunlesi, A. (201890202). Agricultural Productivity , Fiscal and Trade Policies Nexus in Sub-saharan Africa : A Panel Structural Vector Error Correction Model Analysis. Munich Personal RePEc Archive.
Partzsch, L., and Kemper, L. (2019). Cotton certification in Ethiopia: Can an increasing demand for certified textiles create a 'fashion revolution'? Geoforum 99 (December 2018), 111–119. doi:10.1016/j.geoforum.2018.11.017
Partzsch, L., Zander, M., and Robinson, H. (2019). Cotton certification in Sub-Saharan Africa: Promotion of environmental sustainability or greenwashing? Glob. Environ. Change, 57(June 2019), 101924. doi:10.1016/j.gloenvcha.2019.05.008
PASCiB, (2013). La filière coton au Bénin. La Filière Cot. Au Bénin, Regard Analyses Prospect. de La Société Civile Plateforme Des Acteurs de La Société Civile Au Bénin (PASCIB). Cotonou Bull. 4.
Pedroni, P. (2000). Fully modified OLS for heterogeneous cointegrated panels. Adv. Econ. 15. doi:10.1016/S0731-9053(00)15004-2
Pradhan, R. P., Arvin, M. B., Norman, N. R., and Bele, S. K. (2014). Economic growth and the development of telecommunications infrastructure in the G-20 countries: A panel-VAR approach. Telecommun. Policy 38, 634–649. doi:10.1016/j.telpol.2014.03.001
Ren, X., Shao, Q., and Zhong, R. (2020). Nexus between green finance, non-fossil energy use, and carbon intensity: Empirical evidence from China based on a vector error correction model, Journal of Cleaner Production, 277. 122844. doi:10.1016/j.jclepro.2020.122844
Sabesh, M., and Prakash, A. H. (2018). Higher cotton productivity in Africa – a socio economic analysis. ICAC Rec. 36 (4), 1–34.
Shao, Q., Chen, L., Zhong, R., and Weng, H. (2021). Marine economic growth, technological innovation, and industrial upgrading: A vector error correction model for China. Ocean Coast. Manag. 200 (December 2020), 105481. doi:10.1016/j.ocecoaman.2020.105481
Sun, Y., and Razzaq, A. (2022). Composite fiscal decentralisation and green innovation: Imperative strategy for institutional reforms and sustainable development in OECD countries. Sustainable Development. doi:10.1002/sd.2292
Sun, Y., Razzaq, A., Sun, H., and Irfan, M. (2022). The asymmetric influence of renewable energy and green innovation on carbon neutrality in China: Analysis from non-linear ARDL model. Renew. Energy 193, 334–343. doi:10.1016/j.renene.2022.04.159
Tarazi, R., Jimenez, J. L. S., and Vaslin, M. F. S. (2019). Biotechnological solutions for major cotton (Gossypium hirsutum) pathogens and pests. Biotechnol. Res. Innovation 3, 19–26. doi:10.1016/j.biori.2020.01.001
Togbé, C. E., Zannou, E. T., Vodouhê, S. D., Haagsma, R., Gbèhounou, G., Kossou, D. K., et al. (2012). Technical and institutional constraints of a cotton pest management strategy in Benin. NJAS Wageningen J. Life Sci. 60-63 (September 2009), 67–78. –63. doi:10.1016/j.njas.2012.06.005
Traore, O., Wei, C., and Rehman, A. (2022). Investigating the performance of agricultural sector on well-being: New evidence from Burkina Faso. J. Saudi Soc. Agric. Sci. 21, 232–241. doi:10.1016/j.jssas.2021.08.006
Vau, B., and Bourlès, H. (2021). A pseudo-linear regression algorithm in discrete-time for the efficient identification of stiff systems. IFAC-PapersOnLine 54 (7), 322–327. doi:10.1016/j.ifacol.2021.08.379
Keywords: Benin republic, cotton production, economic growth, vector error correction, technological innovation
Citation: Chabi Simin Najib D, Fei C, Dilanchiev A and Romaric S (2022) Modeling the Impact of Cotton Production on Economic Development in Benin: A Technological Innovation Perspective. Front. Environ. Sci. 10:926350. doi: 10.3389/fenvs.2022.926350
Received: 22 April 2022; Accepted: 16 June 2022;
Published: 22 July 2022.
Edited by:
Asif Razzaq, Dalian University of Technology, ChinaReviewed by:
Shahid Ali, Nanjing University of Information Science and Technology, ChinaYunfeng Shang, Zhejiang Yuexiu University of Foreign Languages, China
Copyright © 2022 Chabi Simin Najib, Fei, Dilanchiev and Romaric. This is an open-access article distributed under the terms of the Creative Commons Attribution License (CC BY). The use, distribution or reproduction in other forums is permitted, provided the original author(s) and the copyright owner(s) are credited and that the original publication in this journal is cited, in accordance with accepted academic practice. No use, distribution or reproduction is permitted which does not comply with these terms.
*Correspondence: Chen Fei, chenfei1208@sina.com